Data is one of the most important assets you can use to expand your business and enhance customer experience. As the amount of your organizational data grows, it becomes increasingly crucial to manage the data appropriately and utilize it as effectively as possible. There are many factors that contribute to creating a data-driven environment, including data life cycle management.
Dividing the data life cycle into critical stages can help you achieve business goals. This blog discusses the key phases of a data life cycle and how managing them can benefit you and your organization.
What is the Data Life Cycle?
The data life cycle is a set of stages that the data undergoes in an organization, from its creation to the point where it is no longer considered valuable. Each stage involves specific tasks that ensure data integrity, reliability, and compliance throughout its lifecycle.
Although the arrangement of stages is serial, it is essential to understand that the path the data flows in is not linear. Depending on your company's specific requirements, some tasks under each stage might not occur at all.
The following section highlights the key stages of the data life cycle.
What are the Stages of the Data Life Cycle?

The above data life cycle diagram demonstrates the main stages involved in the life cycle of data. Each stage provides feedback for the next phase about the specific considerations. Here are the most crucial phases that you must consider to ensure efficient data utilization.
Phase 1: Data Generation
The first stage of a data life cycle is data generation, which sets the foundation for all subsequent phases. Data generation occurs continuously as the amount of data present on the internet increases. The data can come from multiple sources, such as internal applications, customer interactions, or even third-party applications.
If analyzed and interpreted correctly, this data can lead to robust insight, enabling you to enhance your business productivity and offer better customer service. You must also use tools like VoIP phone service that can help you analyze the call insights and make data-driven decisions.
Phase 2: Data Collection
Following data generation, the data collection stage involves gathering the data identified as potentially valuable for analysis needs. This data doesn’t necessarily undergo filtering or deleting at this point. The goal is just to collect potential data based on the outline designed.
There are various ways to collect data that cater to your business needs, including:
- Automated Data Extraction: Using tools to automatically extract data from databases, applications, or web services.
- Manual Data Collection: Manually entering data from forums, surveys, or other offline sources.
Phase 3: Data Processing
After the data collection, the next step is to process it and prepare it for further analysis. Multiple activities can help process data.
- Data Wrangling: The raw data is cleaned and transformed into an analysis-compatible format. Data wrangling can include merging data into a single dataset, filling out the missing values, and removing anomalies from the dataset.
- Data Compression: Reducing the data size is necessary as it might be in larger formats that are not easy to store. This step involves transforming the data into an easily usable format by encoding it while maintaining the essence of the information it represents.
- Data Encryption: This step involves encrypting data from one form to another. Converting data into a different format is often beneficial, ensuring security and privacy.
- Data Digitization: Converting analog data into digital data is also a part of data processing. This step formats data from its original form into discrete units.
Phase 4: Data Storage
After properly processing data and converting it into an accessible format, you can store it for further use. This data is mainly stored in databases or datasets, which you eventually upload to cloud storage. Physical storage is not the most convenient way to store data, with the release of new cloud technologies now and then.
An efficient way to store data in any organization is to create a central repository that contains all the accessible information. A single source of truth with all the data in a secure and private environment.
Phase 5: Data Management
Data management is the practice of organizing, storing, and retrieving data from the database to ensure its accuracy, accessibility, and usability throughout its data life cycle. It involves implementing data management tools, strategies, and processes to manage the entire data flow from the beginning to its deletion within an organization.
One of the main components of data management is archiving infrequently used data for long-term storage and compliance purposes. It comprises your organization's policies and standards for data creation, storage, and access.
Phase 6: Data Analysis
Data analysis is the process of analyzing data to generate valuable insights that can help make more informed decisions. You can incorporate various data analysis tools and strategies to enhance your data analytics process.
You can use techniques such as statistical modeling and machine learning algorithms to predict how to maximize your profit while enhancing customer experience. Multiple professionals, including data analysts, business analysts, and data scientists, can perform analysis and produce insights for your business.
Phase 7: Data Visualization & Interpretation
Representing the insights generated in the analysis stage can help you interpret the data more effectively. Various visualization tools, such as Power BI, Tableau, and many more, can be beneficial for communicating the analysis to an audience with or without technical expertise.
How business managers create compelling graphs depends on the data and the analysis goals. Once the data is visually represented, managers can easily interpret it. The most essential part of interpretation is finding patterns in the data and how the data varies in certain situations.
The interpretation stage demonstrates the message that the data implies. With this message, you can make informed decisions about the significant strategies your organization must incorporate to scale your business.
Phase 8: Data Destruction
The final stage of the data life cycle is deleting unnecessary data present within your organization. This involves removing data that is no longer useful to you, adhering to regulations, and preventing unnecessary storage costs. But before destroying data, you must ensure the data retention policy period is over.
What are the Benefits of Understanding the Data Life Cycle?
- Understanding the data life cycle can benefit you even if you do not perform the data management tasks yourself. You can better understand how data works in any organization, enabling you to efficiently communicate with your data team.
- It can familiarize you with insights, allowing you to shift your focus on the key performance indicators (KPIs) to improve your business.
- The data life cycle sets out the basis for data governance, according to which an organization can function by following standard rules. These regulations help create, store, use, and share information for your organization.
- Knowledge about the data life cycle can create common ground between you and every member of your business so that you can interpret and utilize the data effectively.
Effortlessly Streamline Data Analysis Journey with Airbyte
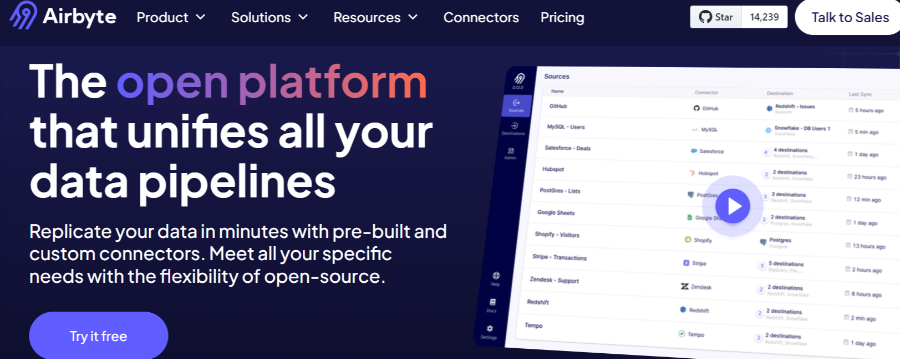
Following proper data life cycle measures is an important aspect to consider. One of the most crucial steps of the data life cycle is integrating data from various sources into a destination. This creates the basis for the data life cycle, allowing professionals working in your organization to access and work with the data.
However, data integration can become challenging if incorrectly executed, resulting in error production and time consumption. To overcome hurdles present in the data integration process, you can use Airbyte.
It is a data integration platform offering an easy-to-use user interface for moving data from different sources to a destination. Airbyte provides 350+ pre-built connectors you can connect to without stressing about the data formats involved. You can utilize the Connector Development Kit to create custom connectors that are flexible to your requirements.
Here are some of the key features provided by Airbyte:
- Integrating dbt (data build tool) with Airbyte allows you to perform robust data transformation techniques. This feature can enable you to develop an end-to-end data pipeline by performing complex transformations using SQL.
- Its Change Data Capture (CDC) feature allows you to capture the changes made to the source data and reflect them in the destination.
- PyAirbyte is a Python-based library that establishes connections with Airbyte connectors. It allows you to utilize Airbyte's strengths using Python programming.
- Airbyte secures the confidentiality and reliability of your data by complying with established security benchmarks, including HIPAA, SOC 2, GDPR, and ISO.
- Airbyte offers flexible pricing plans, including Airbyte Self-Managed, Airbyte Cloud, and Powered by Airbyte, that cater to your specific needs. Airbyte Self-Managed is open-source and free to use. Airbyte Cloud has a pay-as-you-go pricing model, and Powered by Airbyte offers pricing based on syncing frequency duration.
Key Takeaways
Understanding the data life cycle phases is necessary before using the data to make decisions. The data goes through eight main stages. You must know that data life cycle management is an ongoing process that starts during data creation and continues until the data destruction stage.
Proper data life cycle management enables a data-driven culture by helping you create a shared understanding of how data works in your organization. However, managing the data life cycle can become difficult as it requires challenging steps such as highly error-prone data integration.
Consider using a data integration platform like Airbyte to perform seamless data integration. Sign up today and explore its exciting features!
FAQs
What are the key phases in the data quality lifecycle?
The data quality lifecycle has eight primary phases—data generation, collection, processing, storage, management, analysis, visualization and interpretation, and destruction.
What is the life cycle of a data project?
The life cycle of a data project illustrates the stages involved in utilizing data's powers, understanding patterns between different datasets, and making data-driven decisions. The data life cycle is an ongoing process from data generation until destruction.
What is a good example of a data engineering lifecycle?
Consider the situation of an e-commerce platform that generates various data types, including transaction records. This data can be collected, processed, and stored in a data warehouse for further analysis. Analysts can then use this data to create visualizations to help understand customer behavior.
For example, analyzed data can provide insights into products that a particular customer buys frequently. Finally, you can archive or delete this data once it reaches the legal retention period or is no longer valuable.