Data Portability and AI Workloads with Airbyte using Iceberg
Moving AI workloads between clouds is a nightmare for data teams, highlighting the critical importance of data portability. Massive datasets create “data gravity” that locks you into a single cloud provider, limiting flexibility and innovation.
Each major cloud—AWS, Azure, and Google Cloud—has its own architecture, APIs, and services. Build around Lambda or BigQuery, and you’re trapped. As CloudTweaks points out, these incompatibilities constitute a significant blocker to multi-cloud strategies.
Switching environments means costly, time-consuming rebuilds. Proprietary formats, schema mismatches, and slow, expensive transfers make it worse. According to Intelligent CIO, these factors quickly drain AI budgets at scale.
Worse, ML models trained on one provider’s infrastructure can underperform elsewhere, tying your stack to a single vendor.
Cloud sprawl is inevitable. Data portability isn’t optional—it’s the foundation for adapting and scaling AI workloads. The European Union’s General Data Protection Regulation (GDPR) underscores this by establishing the framework for individuals to access, move, and manage their personal data across different services. It emphasizes compliance and the implications for both data processors and users within the EU.
That’s where Apache Iceberg comes in. It was built to solve exactly this by leveraging an application programming interface (API) to enable data exchanges and enhance interoperability between different software systems.
Understanding Data Portability
Data portability refers to the ability to move data seamlessly among different applications, programs, computing environments, or cloud services. It is applicable when the processing of personal data is executed by automated means, highlighting the necessity of consent or a contractual basis between the data subject and the data controller. In the context of cloud computing, it allows customers to migrate their data and applications between or among cloud service providers (CSPs) without losing access to their valuable information.
This capability is crucial for organizations that store large quantities of data in the cloud. It ensures they can switch between CSPs as needed, maintaining continuous access to their data and services.
By enabling this fluid movement, data portability supports flexibility and adaptability in managing AI workloads and other data-intensive operations.
Benefits of Data Portability
Data portability offers several significant benefits that can enhance the efficiency and effectiveness of data management in cloud computing environments:
- Increased Flexibility: With data portability, organizations can switch between different CSPs, allowing them to choose the best service for their needs at any given time. This adaptability is essential for optimizing performance and cost-efficiency.
- Improved Data Management: The ability to move data between various applications and services allows organizations to manage their data more effectively. They can leverage the strengths of different platforms to optimize their data processing and storage strategies.
- Enhanced Collaboration: Data portability facilitates easier data sharing and collaboration between different teams and organizations. Enabling seamless data exchange supports more efficient and productive collaborative efforts.
- Reduced Vendor Lock-In: One of the most significant advantages of data portability is the reduction of vendor lock-in. Organizations are not tied to a single CSP, which means they can avoid the risks and limitations of dependence on one provider.
- Improved Data Security: Data portability allows organizations to move their data to more secure environments as needed. This capability is vital for maintaining high data protection standards and compliance with security regulations.
- Enhanced Consumer Protection: Data portability also plays a crucial role in improving consumer protection by ensuring compliance with data privacy regulations like the GDPR and CCPA. These regulations empower users to make data requests and control their personal information, promoting a broader landscape of consumer rights in the digital space.
What Is Apache Iceberg and How It Enhances Data Portability?
Apache Iceberg is an open table format Netflix created to fix the problems with traditional data lakes, focusing on better data organization, scalability, and metadata management for modern cloud workloads. It supports various data formats, including Extensible Markup Language (XML), for structured data representation.
It enables individuals to transfer their reputation or credibility between various contexts, such as different online communities and professional networks, underscoring the importance of maintaining a consistent reputation across multiple platforms to build trust and reduce reliance on a single community. It plays a significant role in facilitating data portability across different platforms.
Traditional data lakes break down when handling massive AI datasets. Iceberg solves this with features that keep your data consistent, accessible, and portable:
ACID Transactions That Actually Work
When multiple software applications write to your data simultaneously, Iceberg keeps everything consistent—essential for AI pipelines pulling from diverse sources and ensuring data portability across environments.
It is also crucial to consider the rights and agreements of all parties involved in data transactions to ensure compliance and protect individual rights.
Time Travel for Experiment Reproducibility
Need to recreate the exact dataset version from last week’s training run? Iceberg lets you access precise historical snapshots without complex backup systems, enhancing data portability by simplifying data versioning.
This capability also facilitates interoperability, allowing different systems to seamlessly exchange data and promoting competition by allowing users to switch services without losing their valuable data. Additionally, time travel capabilities allow for the reuse of historical data snapshots, further enhancing data portability.
Hidden Partitioning for Optimized Performance
Iceberg handles complex data organization behind the scenes, including transaction data management, while keeping queries lightning fast—no more manual partition management, which streamlines data portability efforts.
Additionally, data is stored in structured formats on computer disks, facilitating effective data extraction and usability.
Schema Evolution Without Breaking Changes
Add, rename, or change columns without breaking existing queries or pipelines. This flexibility is crucial when your AI datasets constantly evolve, supporting seamless data portability and ensuring compliance with data protection regulations.
As a data controller, you provide personal data in a structured format and ensure its secure transmission to another controller upon request. Well-defined data formats like CSV and XML ensure compatibility and ease of use in data management.
Iceberg offers significant advantages compared to Apache Hive, which struggles with massive datasets. Hive’s chunky partitions don’t scale well, its metadata handling breaks down with large tables, and it can’t handle frequent updates AI workflows demand.
Even compared to Delta Lake, Iceberg provides more flexibility, avoiding Delta’s frequent checkpointing, which slows down complex AI jobs, and its limitations, mostly to the Parquet format.
For machine learning teams, Iceberg delivers reproducible experiments, flexible schemas, compatibility with diverse query engines, and strong performance at scale.
Using Airbyte with Iceberg: A Future-Proof Stack for Data Portability
Data engineers waste countless hours building fragile pipelines that break when source schemas change or when moving between environments. Combining Airbyte with Apache Iceberg solves this headache by creating a resilient foundation for your AI data flows and enhancing data portability.
While there is no universal right to data portability, regulations like the California Consumer Privacy Act (CCPA) define its parameters, emphasizing the importance of security and compliance in the data sharing process.
Airbyte’s 550+ source connectors and 50+ destination connectors (including Apache Iceberg) eliminate custom coding for data movement. With just a few clicks, you can pull information from virtually any source into your Iceberg tables, ensuring your data remains portable across systems.
Setting up this pipeline takes minutes:
- Configure your source connector (e.g., PostgreSQL, Salesforce, Google Analytics)
- Set up Apache Iceberg as your destination connector
- Define your sync settings, including table mapping, scheduling, and transformation rules
The real power emerges when Airbyte’s incremental updates work with Iceberg’s transactional guarantees. Instead of full table reloads, Airbyte tracks and replicates only what’s changed since the last sync, paired with Iceberg’s ACID transactions to ensure consistency.
For AI teams, this means models always train on the latest information without processing unchanged data, which is critical when every training run counts against your compute budget.
Both technologies are fully open-source, giving you complete control over your data infrastructure. You avoid vendor lock-in, benefit from community improvements, and can deploy anywhere—on-premises, in the cloud, or hybrid environments, all while maintaining data portability.
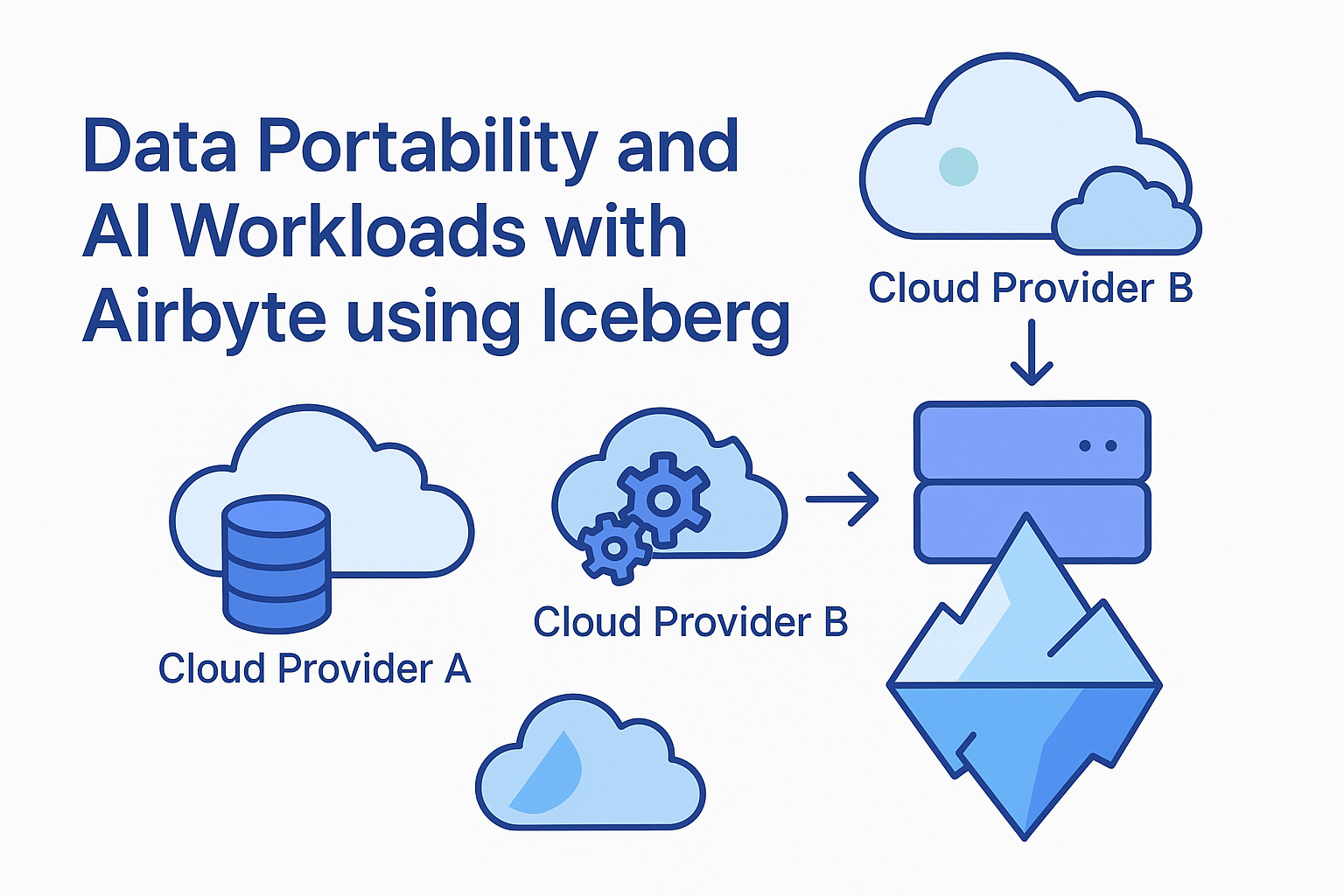
Data Portability in Practice: Use Cases
Teams must move data freely between platforms, tools, and clouds without facing friction or compliance issues. Done correctly, it saves time, improves model performance, and keeps you in line with regulations like GDPR.
These use cases show how companies make data portability work in the real world. With tools like Airbyte and Apache Iceberg, data portability is not just possible—it’s practical, scalable, and fast.
Single Source of Truth Across Multiple Clouds
Companies training AI models often face fragmented datasets across cloud providers, creating inconsistency nightmares.
A Fortune 500 company eliminated this problem by using Airbyte to move data from various SaaS applications into Apache Iceberg.
With Iceberg’s compatibility across analytics engines like Snowflake and Databricks, they created a unified data foundation accessible from any environment, demonstrating effective data portability.
This approach aligns with the “right to data portability” as established by the GDPR, ensuring that personal data can be efficiently managed and reused across different platforms while maintaining compliance. Data portability enables organizations to transfer data seamlessly across different cloud environments.
Reproducible AI Experiments with Time Travel
Recreating past experiment conditions can be a significant headache for data scientists, especially when datasets constantly change. Without versioned data, it's nearly impossible to reproduce results with confidence.
Airbyte’s reliable data synchronization, combined with Apache Iceberg’s time travel capabilities, solves this problem.
Teams can easily access historical snapshots of datasets, ensuring consistency across experiments and enabling faster iteration with accurate, auditable results.
Real-Time Model Retraining with Fresh Data
Models decay when they can’t access fresh data, but updating massive datasets efficiently challenges many teams.
The Airbyte-Iceberg combination enables continuous updates without compromising data integrity. This approach facilitates easier transfer and interoperability between systems by ensuring data is in a structured, commonly used, and machine-readable format.
Feature Stores for Consistent ML Features
API-driven data exchanges and interoperability are crucial for feature stores to ensure consistent ML features. Iceberg’s hidden partitioning ensures fast feature retrieval, while Airbyte’s connector ecosystem makes ingesting features from diverse sources straightforward.
APIs can connect different systems to facilitate data exchanges and interoperability, preventing vendor lock-in and enhancing consumer options.
Preparing for Data Portability Requests
Preparing for data portability requests involves several critical steps to ensure compliance with the General Data Protection Regulation (GDPR) and other relevant laws. First, data controllers must clearly understand the right to data portability and its implications for their organization.
A robust process for receiving and responding to data portability requests is essential. This includes a system for verifying the data subject's identity and ensuring the request's legitimacy. The process must be transparent, efficient, secure, and communicated to data subjects.
Technically, data controllers must be capable of transmitting personal data in a structured, commonly used, and machine-readable format, such as JSON, XML, or CSV. Implementing APIs or other technical solutions that enable secure and controlled data transfer is crucial. By ensuring these measures are in place, data controllers can effectively manage data portability requests and uphold the rights of data subjects.
Challenges of Implementing Data Portability
While data portability offers numerous benefits, implementing it can be challenging due to several factors:
- Standardization: Achieving data portability requires standardization of data formats and protocols. This standardization can be difficult to achieve, especially when dealing with diverse systems and applications.
- Interoperability: Ensuring interoperability between different systems and applications is crucial for data portability. However, achieving this interoperability can be challenging due to varying technologies and architectures.
- Security: Maintaining the security of data during transfer is a significant concern. Organizations must implement robust security measures to protect data from breaches and unauthorized access during the migration process.
- Governance: Data portability raises governance issues like ownership and control. Organizations need to address these issues to ensure clear policies and responsibilities are in place.
- Technical Feasibility: Implementing data portability requires technical feasibility, which can be particularly challenging for large datasets. Organizations must ensure that their infrastructure and resources can support the efficient transfer and management of data.
Information technology is crucial in facilitating data movement and managing the associated costs, which can vary among firms and sectors.
Data portability is a critical aspect of cloud computing that requires careful consideration of standardization, interoperability, security, governance, and technical feasibility. By addressing these challenges, organizations can fully leverage the benefits of data portability to enhance their AI workloads and overall data management strategies.
Best Practices for Building Portable AI Pipelines
Data teams struggle to maintain consistency when moving AI workloads between environments. You can create pipelines that work seamlessly anywhere by standardizing your approach with Airbyte and Apache Iceberg.
When managing data, it is crucial to ensure compliance with GDPR regulations, particularly in terms of the rights of data subjects.
This includes managing, transferring, and protecting personal data to uphold these rights. Using human-readable data formats like JSON and XML is important for data portability, as they are easily understood by humans and machine-readable.
Standardize on Columnar Formats for Cross-Environment Performance
Standardizing on columnar formats like Parquet creates consistent optimization throughout your pipeline. It integrates smoothly with both Airbyte for data movement and Apache Iceberg for storage, boosting interoperability and enhancing data portability. This setup accelerates analytical queries and reduces compute costs for read-intensive AI workloads.
Another commonly used data format that supports data portability is CSV .
When configuring Airbyte to sync data into Iceberg tables, explicitly specify Parquet as your preferred storage format.
Track Data Lineage Across Environment Boundaries
- Integrate OpenLineage with Airbyte to automatically track data flows from source to destination, ensuring compliance and efficient data management with the help of artificial intelligence.
- Leverage Iceberg’s built-in metadata tables to track changes at both file and row levels:
SELECT * FROM my_catalog.my_db.my_table.history
- Centralize this metadata in tools like Amundsen or DataHub to make information discoverable across your organization.
Manage Schema Evolution Consistently
- Document changes explicitly when modifying schemas in Airbyte.
- Use Iceberg’s schema evolution capabilities to add, drop, or rename columns without rewriting data, ensuring users can manage data consistently and comply with regulations. Additionally, providing personal data in a structured, commonly used, and machine-readable format is crucial to comply with data portability requests.
- Add validation steps to verify that incoming data matches expected schemas before syncing to Iceberg tables.
Build Auditability into Every Pipeline Stage
- Create automated workflows with tools like Apache Airflow or Prefect that validate metadata at each pipeline stage.
- Use Iceberg’s time travel feature to access historical data states for auditing and debugging, ensuring that any changes do not adversely affect the rights and freedoms of third parties.
- Schedule Airbyte’s incremental syncs to regularly validate both data and metadata alignment.
Social media platforms must allow users to transfer their data seamlessly to ensure user control and compliance with data portability regulations.
Optimize Performance Consistently Across Environments
- Use Iceberg’s hidden partitioning to optimize query performance without adding complexity to your data model.
- Configure Airbyte to use CDC or incremental sync patterns to minimize data transfer volumes.
- When using dbt with Airbyte for transformations, ensure your models align with Iceberg’s query patterns and comply with data management requirements, including handling data portability requests as mandated by the UK GDPR. JavaScript Object Notation (JSON) is a widely used data format supporting portability.
Build Once, Run Anywhere with Data Portability
Moving AI workloads between environments shouldn’t require rebuilding your entire data foundation. By combining Airbyte and Apache Iceberg, you can create a data infrastructure that works consistently anywhere while giving you the control to adapt as your needs change.
Incorporating interoperable formats like JSON and XML can further enhance data management and compliance, ensuring seamless data exchange between different systems. The European Commission plays a crucial role in establishing data portability regulations and compliance standards, promoting interoperability and legal frameworks that benefit both EU and non-EU entities.
This approach solves real problems that data teams face daily:
- You eliminate vendor lock-in through Airbyte’s 400+ pre-built connectors and Iceberg’s compatibility with multiple query engines.
- Your AI pipelines run faster with Apache Iceberg’s efficient metadata management and optimized data layouts—speeding up analytical queries significantly.
- You maintain complete data governance with comprehensive lineage tracking, schema evolution, and time travel capabilities.
- You control where and how your data flows as both technologies are fully open-source.
What makes this approach particularly valuable is its resilience to change. As AI techniques evolve rapidly, a flexible foundation built on open standards ensures that you can incorporate new tools without rebuilding your entire infrastructure while supporting data portability.
The active communities around Airbyte and Apache Iceberg mean you’re not alone—you benefit from continuous improvements, extensive documentation, and shared knowledge as you scale.
Want to stop rebuilding pipelines every time you need to move workloads? Try integrating Airbyte with Apache Iceberg for your next AI project. Empowered by data portability, you can experience truly portable data infrastructure today.