Data Readiness For AI: How to Ready Your Data For Gen AI
A recent McKinsey global survey highlights that employees are adopting generative AI (GenAI) faster than their organizations, as businesses have been slow to implement it at scale. While companies focus on strategy and risk management, 91% of users are already integrating GenAI into their daily work. Nine out of ten believe AI can improve their work experience and support skills like critical thinking and creativity.

Despite this, companies have been reluctant to build their own AI models to maximize business productivity. To keep up, businesses needed a clear plan to develop and use AI strategically. However, AI alone is not enough; AI-ready data is required to work well.
This article covers the concept of data readiness for AI and the factors that make your data ready for GenAI systems.
What Is Data Readiness For AI?

Data readiness for AI refers to the process of preparing and optimizing your data to support GenAI models seamlessly. For AI to deliver reliable insights, your data must meet the following criteria:
- Data should be well-structured and meaningful to provide the right context for correct interpretation.
- It has to be accurate, complete, consistent, relevant, and unique to ensure trustworthy AI outputs.
- Strong governance is necessary for ethical and compliant data usage.
- Data needs to be easily available, discoverable, and readily accessible for AI processing.
Without proper AI data readiness, models may produce biased and incorrect results, limiting their value and impact on decision-making.
What Is the Importance of AI-ready Data?
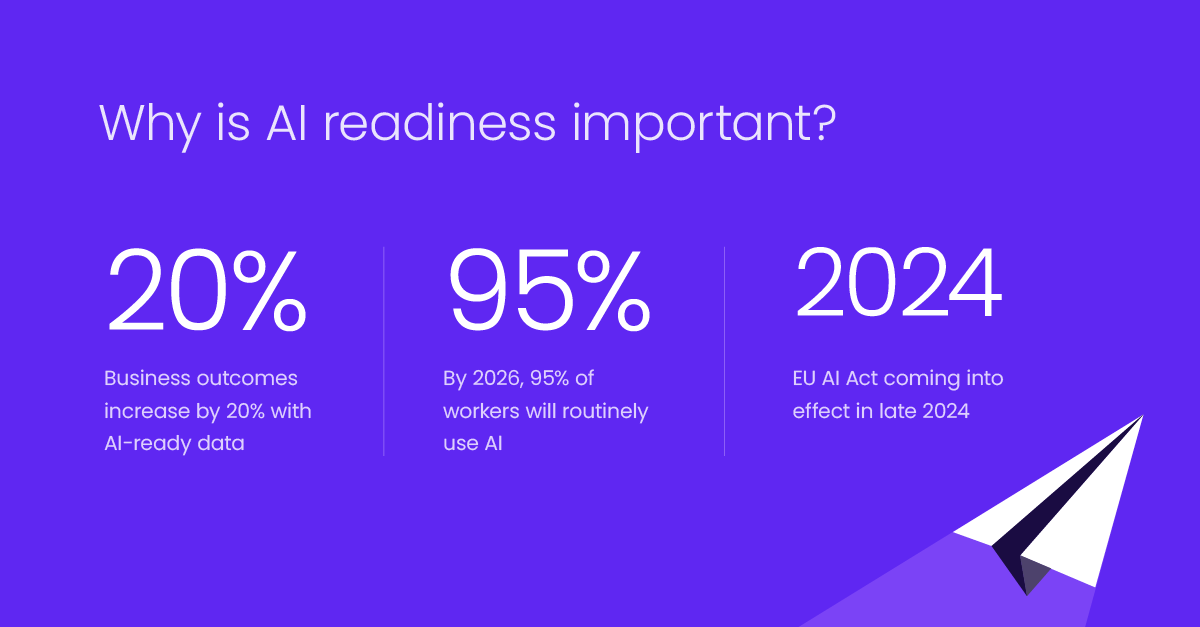
While the given statistical information highlights the significance of AI-ready data, here are a few key benefits that show its importance:
- Improve Model Precision: High-quality data enables AI models to extract relevant patterns, leading to generating more natural, trusted outcomes. This increases the model's precision, making it suitable for applications like fraud detection, healthcare diagnostics, and personalized recommendations, where precision directly influences outcomes.
- Achieve Data Consistency: AI applications might rely on multiple data sources, such as customer interactions, transactional records, and IoT sensor data. Ensuring these sources are consistent can assist you minimize discrepancies that typically arise during integration. By unifying reliable datasets, your AI models can seamlessly process information from different platforms to deliver context-aware responses.
Challenges in Achieving Data Readiness for AI
Ensuring data readiness for AI implementation comes with several limitations that can affect model accuracy and performance.
Data Silos
Scattered and disconnected datasets prevent AI models from accessing a complete and unified view of information. When data remains isolated across various departments or systems, it limits AI’s capability to derive meaningful trends and make correct predictions.
Breaking down these silos requires strong data integration and collaboration strategies.
Data Quality Issues
Training datasets with duplicates, missing values, or outdated records can compromise data reliability. This causes AI models to generate inaccurate, meaningless outputs.
If you utilize robust data cleaning processes, you can maintain high data quality and provide relevant AI-driven insights.
Security and Compliance Risks
AI-driven systems must adhere to strict data privacy laws, including GDPR and the EU AI Act, to prevent misuse and ensure safe AI deployment. Non-compliance can result in legal consequences, reputational damage, and financial losses.
To manage data securely, you need to establish strong security protocols and governance policies.
Limited Data Accessibility
AI models require smooth access to relevant and up-to-date data, but permission restrictions and outdated infrastructure may create obstacles. Inaccessible data slows down AI adoption and minimizes its effectiveness.
Following appropriate data management practices and modernizing infrastructure can improve accessibility.
Bias and Ethical Concerns
There is a possibility for the AI model to inherit biases from historical or unstructured data. This leads to unfair and discriminatory results. If biases go unaddressed, they can impact your business decisions, customer trust, and regulatory compliance.
You should implement bias detection, use diverse, large-scale datasets, and follow ethical AI practices to control these risks.
How to Ready Your Data For AI?
Making your data ready for AI involves several steps; each stage is crucial in enabling your AI models to learn and predict correct outputs.
Data Collection and Integration
Data readiness for AI begins with collecting data from diverse and relevant sources. When you gather data from trustworthy systems, the AI model receives varied and high-quality inputs for the learning process. While collecting data, you must ensure the data aligns with your specific AI use case.
For example, when developing a healthcare app, you must focus on relevant data like patient histories, physician records, and diagnostic images to generate meaningful results.
After you’ve gathered sufficient data, consider merging it into a unified storage system such as vector databases, data warehouses, or data lakes. This data consolidation can improve the effectiveness of your AI models. No-code data movement platforms like Airbyte can assist you in automating the integration process.
Data Cleaning and Transformation
The crucial aspects of data preprocessing are data cleaning and transformation. Data cleaning involves detecting and fixing errors, removing duplicates, and handling missing values. This can be done by using outlier detection, imputation, and validation checks.
Once your data is cleansed, you can apply several data transformation approaches, including min-max normalization, standardization, encoding, and data discretization. While this process can be time-consuming, it is essential to determine high-quality data that enhances AI model performance.
Data Reduction
When your data is clean, the next step is to minimize data volume while preserving its essential information. This stage aids you in eliminating redundancy, reducing storage requirements, and enhancing data quality. Several techniques, including feature engineering, dimensionality reduction, and data compression, can assist you in streamlining the data reduction process.
Data Labeling and Annotation
After data reduction, you can utilize data labeling and annotation tools to maintain the relevance of the refined dataset. While optional, this step remains useful for training supervised AI models.
Data labeling involves assigning predefined tags, categories, or labels to data points, enabling AI models to learn patterns and deliver correct predictions. In contrast, data annotation goes beyond labeling by adding metadata to raw data and providing contextual information for complex AI applications.
Data Splitting
Once the data is preprocessed, it must be divided into distinct sets to train, validate, and test the AI model. When you split the data, the AI model generalizes well to new data rather than memorizing patterns from the training set.
A popular method that assures balanced model training and evaluation is the 70/20/10 rule. 70% of the dataset is allocated for training, allowing the model to learn patterns and relationships within the data. The 20% is used for validation, helping improve the model prediction’s accuracy and prevent overfitting. The remaining 10% is reserved for testing the final model performance on completely unseen data.
Following these essential steps in data preparation, you can generate high-quality, well-structured data that optimizes your model functionality. Each stage, from data collection to final testing, enables you to provide relevant, clean, and formatted data for your AI agentic workflows.
How to Know Your Data is AI-Ready?
You can assess whether your data is truly AI-ready based on these three indicators:
Check If Your Data Is Centralized
AI models require a unified data source to function smoothly. If your data is dispersed in multiple systems, it can lead to inconsistencies. By centralizing the datasets into a single storage system, you can process and use the data required for model training effortlessly.
Verify For Right Data Contextualization
Raw data is not enough for AI systems to make actionable insights. If your data is contextually enriched by adding metadata or labeling, AI systems can interpret the information properly. For instance, in healthcare, a patient's records should include not just test results but also timestamps, doctor’s notes, and treatment history. This added context supports your AI models in understanding data relationships better, leading to more precise outputs.
Ensure Data Relevance and Timeliness
When you train AI systems on timely and relevant data, the model performs best. Outdated, incomplete, or unnecessary data can result in incorrect and biased outcomes. Regular updates, real-time data streaming, and validation processes let you maintain data relevance for AI-based workflows.
Tools to Help With Data Readiness For AI
Here are some tools that can assist you with data readiness for AI, categorized by their functions:
Data Integration
As mentioned earlier, Airbyte allows you to streamline data integration by extracting data from different sources and loading it into a desired target system.

Key features of Airbyte include:
- Extensive Connector Catalog: Airbyte offers 550+ pre-built connectors to assist you in combining data from varied sources into data warehouses, object storage, analytical tools, and many other destinations.
- Custom Connector Development: You can build personalized connectors using Airbyte’s no-code Connector Builder, low-code CDK, and language-specific CDKs. The Connector Builder features an AI assistant that auto-fills the mandatory fields during the connector setup.
- Vector Database Support: Airbyte offers compatibility with eight vector databases, including Pinecone, Milvus, and Weaviate. These vector stores enable you to facilitate efficient storage, retrieval, and processing of high-dimensional embeddings, ensuring data readiness for GenAI systems.
Data Cleaning and Transformation
OpenRefine is a powerful, open-source transformation tool for handling messy and noisy data. It offers two techniques—faceting and clustering, to bring more consistency to your data.
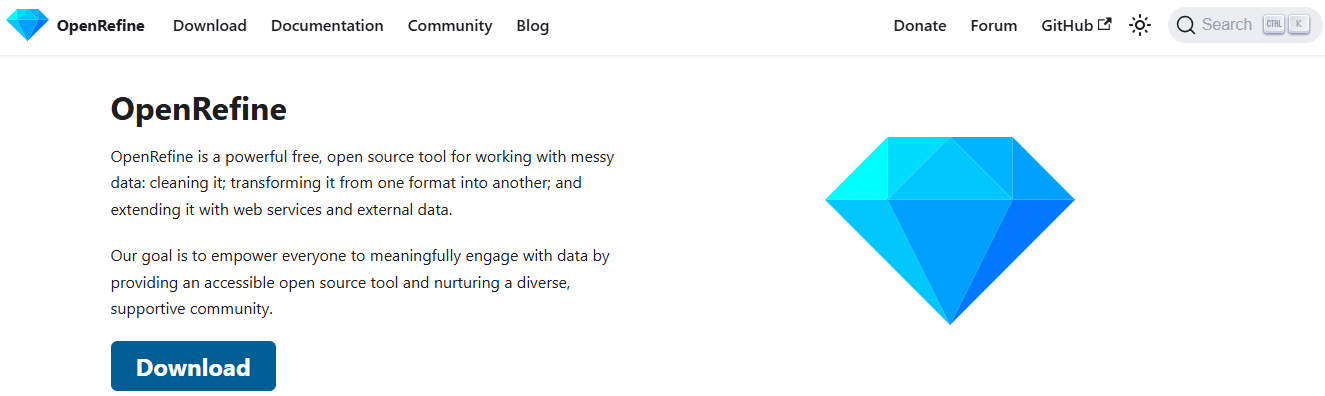
The former uses one of the essential features of OpenRefine, which is called facets. Facets aids you in gaining a clear overview of your data while improving data integrity. It comes in various forms, such as text, numeric, timeline, custom, and scatterplot. By leveraging a facet, you can group all values in a column, filter the data based on these values, and edit values across multiple records simultaneously.
The latter helps you identify and merge similar values using advanced heuristics. While it's useful for spotting errors, typos, and inconsistencies, clustering works only at the syntactic level and does not provide semantic awareness. To bridge this gap, OpenRefine allows you to integrate with external reconciliation services like Wikidata. This integration enables you to enhance data accuracy beyond syntax-level clustering.
Take a look at some of the most powerful data transformation tools here.
Data Annotation
SuperAnnotate is an AI data platform for image, video, text, and audio annotation. It lets you speed up your model development and evaluation with high-quality annotated training datasets.
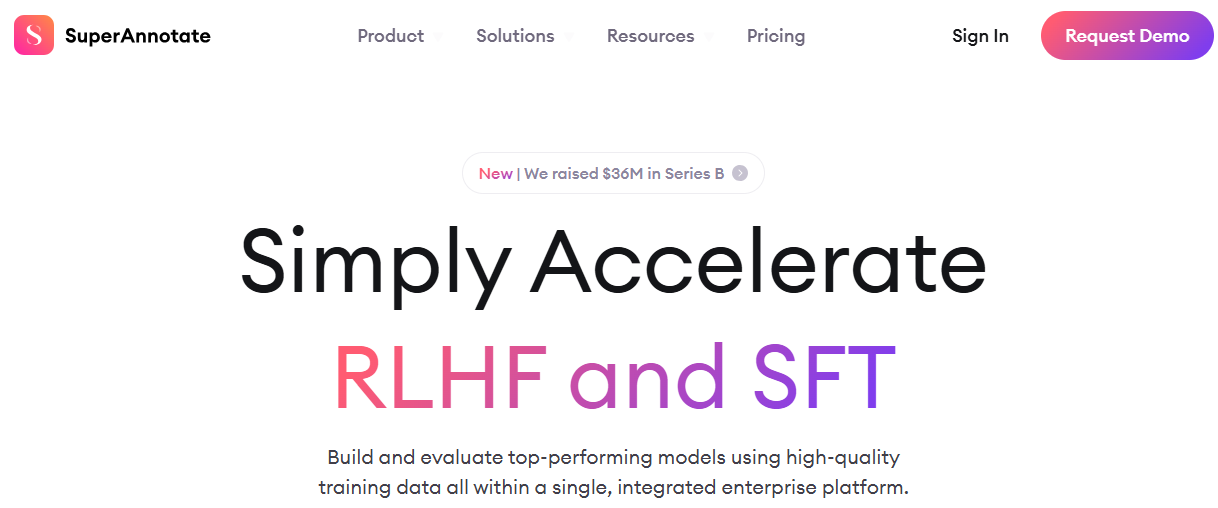
With SuperAnnotate, you can create annotations from scratch or by importing predefined annotations from existing models, known as pre-annotations. By utilizing pre-annotations, you can improve active learning and model-assisted labeling, accelerating the annotation process.
Besides this capability, SuperAnnotate enables you to automate the annotation tasks with a one-shot annotation approach called Annotate Similar. Rather than searching over each item in the dataset, Annotate Similar recommends a cluster of similar elements based on a single reference.
Data Governance
Alation is a data intelligence platform that allows you to manage, search, and leverage data effectively. It gives you the ability to transform metadata into a strategic asset through data cataloging, governance, and automated data lineage.

While data cataloging lets you organize and index metadata to improve data discoverability, Alation’s data governance framework ensures compliant data access for AI workflows.
Alation's data lineage capabilities are particularly valuable for professionals pursuing Gen AI certification. By providing a clear view of data flow and usage, the metadata summary pane enhances data trust and governance—key aspects when building AI models or driving data-driven business decisions.
Conclusion
Achieving data readiness for AI requires a structured approach, from data collection and integration to cleaning, transforming, labeling, and splitting. Appropriate data preparation ensures that AI models receive accurate and relevant inputs for generating valuable insights and making better predictions. With several tools highlighted in the article, you can streamline data preparation processes to enhance AI performance while maintaining data governance.