Organizations increasingly rely on data to make informed business decisions, drive innovation, and achieve their goals. However, to leverage the data’s full potential, you would need a robust data strategy. A data strategy outlines the vision, guidelines, and tools to manage your organization’s information assets.
This article answers the question, “What is data strategy?” and explains its importance, key components, and the approach you should take when creating one. This will help you align your data with your organization’s goals, optimize operational efficiencies, enhance customer experience, and maintain a competitive edge in the market.
What Is a Data Strategy?
A data strategy is a comprehensive long-term plan defining data collection, storage, sharing, and utility while aligning with your organization’s business strategy. It integrates data management practices, technologies, and policies to ensure your data is accurate, accessible, and actionable.
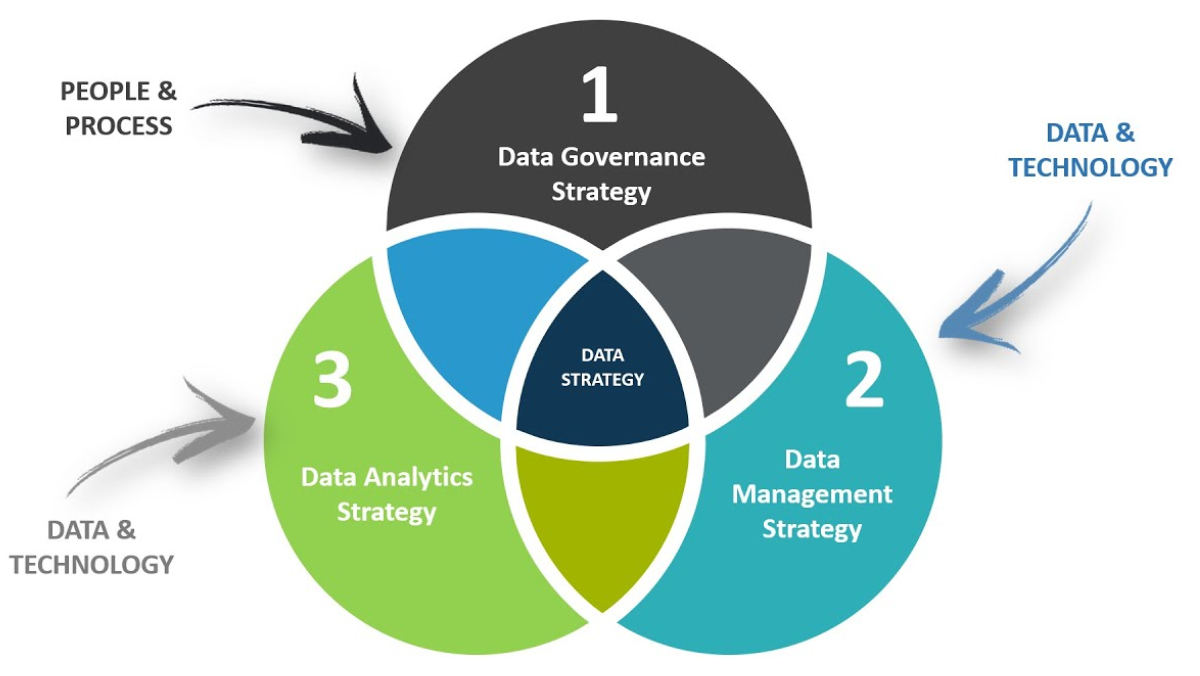
A well-defined data strategy considers the entire data lifecycle and provides a roadmap for governance, architecture, and analytics. This turns your data into a powerful asset you can leverage to design targeted marketing campaigns, identify emerging trends, and capitalize on them.
You can also develop machine learning (ML) and artificial intelligence (AI) applications trained on your data to automate repetitive tasks and improve overall cost efficiency.
Importance of a Data Strategy
Data strategies are essential for your organization to stay relevant in the continuously changing market. It helps you understand your current data infrastructure, identify areas for improvement, and define the steps to address shortcomings. Here are some more reasons explaining the importance of having a data strategy:
- Improved Task Prioritization: A data strategy helps you prioritize projects that give the most business value. It ensures all data-related activities are focused on extracting valuable insights from market research, trends, and customer behavior. Leveraging AI tools for market research can further enhance the accuracy and efficiency of these insights, helping you streamline high-value initiatives.
- Enhanced Data Quality and Consistency: A data strategy establishes standards and processes for data collection, storage, and management, ensuring high-quality and consistent data across your organization. This minimizes biases during analysis, prevents poor decision-making, and saves time and resources by reducing the need for rework.
- Facilitates Regulatory Compliance: A comprehensive data strategy includes compliance with relevant industrial regulations, such as HIPAA and GDPR, and anonymization of PII. It ensures that data is handled according to legal requirements, avoiding costly penalties and reputational damage.
- Optimizes Costs: Implementing a data strategy results in effective data management that reduces unnecessary data storage and processing costs. It helps you identify and fix operational inefficiencies, perform predictive maintenance, and prevent equipment failure and increased downtime. This also contributes to significant cost savings.
- Increases Efficiency and Productivity: Data strategy helps you streamline data operations, minimize manual tasks, reduce errors, and free up resources for strategic planning. It fosters a unified approach to data management, encouraging collaboration across your departments, breaking down data silos, and promoting data sharing.
- Facilitates Future-Proofing: Regularly revising and updating your data strategy can enhance your organization’s data capabilities. It helps you adapt to new technologies and trends, evolving market demands, and changing business environments. This proactive approach ensures long-term sustainability and growth.
Data strategies ensure your data initiatives are purposeful, efficient, and cost-effective, driving better outcomes for your organization’s success. This emphasizes the need to have a well-planned data strategy for your organization.
Why & How to Set Data Strategy Goals?
Setting clear data strategy goals is crucial for guiding your data initiatives, measuring their success, and making data-driven adjustments. These goals help you prioritize what data to collect, analyze, and leverage, maximizing your return on investment (ROI).
Well-defined goals motivate your teams and foster stakeholder buy-in, encouraging broader adoption of data-driven projects. These goals guide your step-by-step plan of leveraging data to achieve business objectives, mitigate risks in the early stages, and stay ahead of the curve.
You can follow the steps below to set your data strategy goals:
- Identify Business Priorities: Discuss the project’s expectations with the leadership and key stakeholders and map out how data can help achieve them while ensuring relevance and impact.
- Assess Current Data Capabilities: Conduct a thorough audit of your existing data assets and infrastructure. Identify gaps and opportunities for improvement. This will help you set realistic and achievable goals.
- Define SMART Goals: Ensure your goals are Specific, Measurable, Attainable, Relevant, and Time-bound (SMART). For example, improving data quality, increasing customer retention, or decreasing churn rate by analyzing purchase history.
- Communicate Effectively: Once you have outlined the goals, metrics, and action plan, communicate it to your teams and stakeholders. Assign roles and responsibilities while explaining the expected outcomes. This fosters transparency and accountability within the teams.
- Monitor and Adjust: Regularly review your team’s progress against goals and be flexible in pivoting or adapting new strategies based on your evolving business needs and data capabilities.
Key Components of a Data Strategy
In this section, you will learn about the core components of your database strategy. Understanding each component will help you build a solid foundation for growing as a company with a data-driven culture. You will learn how to effectively manage, analyze, and leverage your organization’s data, transforming raw information into actionable insights.
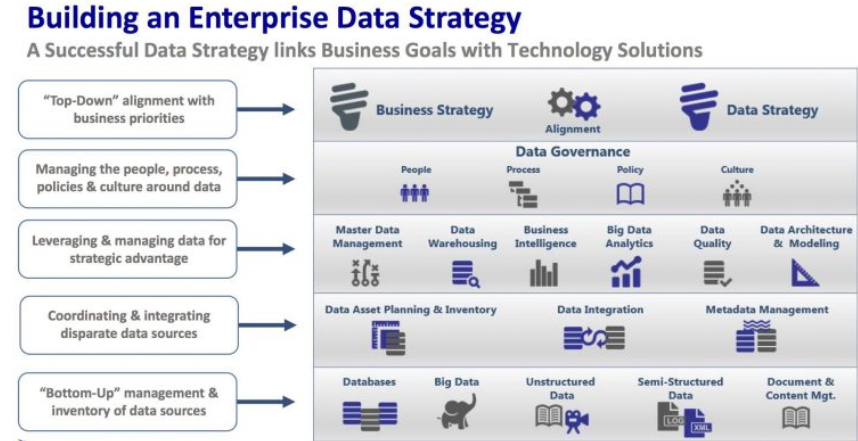
Business Strategy
Your data strategy should be closely aligned with your organization’s overall business goals. This ensures that your data initiatives support and drive your business goals, such as improved customer experience or new prototype development within the allotted budget.
You must also set clear, measurable objectives demonstrating how your data strategy contributes to business outcomes. These objectives provide evidence of progress and impact, facilitating stakeholder buy-in for your data-driven initiatives.
Organizational Roles
Teamwork is crucial to making your data strategy a success. Defining clear roles and responsibilities involves identifying the key contributors, such as data engineers, analysts, scientists, architects, and business managers.
Each team member should own their work and communicate their progress, maintaining transparency, seamless collaboration, and avoiding duplication of efforts. This collective approach also fosters a data-driven culture.
Data Management
Data management involves establishing policies and practices that ensure data quality, security, and accessibility. This includes data governance frameworks to help you set standards for data handling, privacy, and regulatory compliance.
Based on your organization’s requirements, you can also invest in data management or governance tools that streamline and automate data monitoring. These tools alert you of unauthorized access or threats, allowing you to respond quickly and prevent further damage.
Data Architecture
Developing a comprehensive data architecture involves designing and organizing your data systems and infrastructure. This includes data storage solutions such as data warehouses and lakes, data pipelines for ingestion and transformation, and tools for data analysis.
You should ensure your data architecture is scalable and flexible, supports efficient data operations, and accommodates your growing data needs.
Data Analytics
Extracting valuable insights from your data requires implementing statistical and computational techniques. By employing data analytics tools, you focus on developing capabilities to analyze data effectively and leverage technologies like AI and ML that enable predictive and prescriptive analytics.
You can also use these tools to clean, filter, and transform data based on your business requirements and utilize that data to train ML/AI models. These data models help you perform advanced analytics, automate repetitive tasks, and increase operational efficiency.
Developing a data strategy is an ongoing process in which you continuously monitor and revise your approach based on metrics and feedback. By integrating all these components, you can create a cohesive plan that aligns with your business objectives and helps achieve sustainable growth.
Which Approach to Choose When Creating Data Strategy?
Businesses typically choose between a centralized and a decentralized approach when designing a data strategy. Each approach has its benefits and drawbacks, which makes choosing the right strategy highly dependent on your organization's specific needs, structure, and goals.
Centralized Approach
A centralized data strategy allows you to consolidate data management and governance under a unified system. There is one central repository for all your customer, sales, or revenue information.
This approach minimizes risks downstream by identifying, standardizing, and governing reliable data sources to ensure the consistency of the information flowing through your company's internal systems. A centralized data strategy prioritizes tasks like ensuring data integrity and regulatory compliance, detecting fraud through data analysis, and taking security measures to prevent cyber attacks.
Other advantages offered by centralized data strategy include:
- Consistency and Standardization: Standardized processes and definitions allow you to maintain your data’s quality, consistency, and reliability across the organization. This helps you perform data analysis with minimal bias and errors.
- Easier Integration: Integrating new data sources, tools, or systems is comparatively easier in a centralized environment. This significantly reduces the complexity of data handling across all departments of your organization.
- Improved Cost Efficiency: A centralized data management strategy can reduce redundancy and streamline operations, potentially lowering data storage and processing costs.
However, this approach can be less flexible and has a single point of failure. This implies that if your central data repository is compromised, it can affect the entire organization’s workflow and security. Centralized systems can also create a bottleneck if data access or processing overloads.
Decentralized Approach
Contrarily, a decentralized data strategy complements a centrally governed system by adding more flexibility. It acknowledges that data interpretations can differ across business units and distributes data management responsibilities across various departments or business units. However, it only allows for this leniency by permitting controlled data transformations that you can reliably trace back to a single source of truth.
The decentralized approach fosters a sense of ownership among your domain-specific teams, allowing them to experiment and build tailored data products, driving innovation. Additionally, the closer proximity to the data enables them to make faster decisions and stay agile while responding to evolving market trends or setbacks.
Other advantages offered by decentralized data strategy include:
- Local Optimization: Each team at your organization can fine-tune their data processes and systems independently to optimize performance and efficiency for their specific operations.
- Scalability: A decentralized approach can be more scalable as your organization grows. Individual departments can have the authority to expand their data capabilities based on their needs.
- Scope for Innovation: Your data teams can experiment with new technologies, tools, and methodologies without affecting other data systems. This can lead to innovative solutions and improvements that might otherwise be difficult to achieve.
A decentralized data strategy can create situations where departments develop data definitions or storage methods that are difficult for the rest of the organization to follow. This can lead to data fragmentation, making it harder to maintain a unified view of the organization’s data. Implementing consistent data governance across a decentralized system can also be challenging, potentially increasing the risk of errors and regulatory non-compliance.
Choosing the Right Approach
If you want your data strategy to be a success, you must incorporate both centralized and decentralized approaches. However, finding the right balance can be challenging. Centralized strategies offer consistency and control, making them suitable for teams that prioritize data quality and governance, such as legal, financial, compliance, and IT departments.
Contrarily, decentralized strategies are well-suited for real-time operations in dynamic departments that need more flexibility, such as customer support and infrastructure maintenance teams. You can design a data strategy that fits your business model by carefully evaluating your requirements and capacity.
Who Builds Data Strategy?
Creating an effective data strategy requires a collaborative effort from all the data experts at your organization. Each role brings unique skills and perspectives to the table. Here’s a breakdown of the roles played by data engineers, data scientists, and data analysts in building a data strategy:
Data Engineers
Data engineers are responsible for designing, constructing, and maintaining the data architecture supporting your organization’s evolving needs, such as data collection, storage, and processing. They understand the capabilities of data storage and processing systems like data warehouse and ETL processes, aligning your strategy with what the technology can handle. Data engineers define the technical feasibility of your data strategy while ensuring data accessibility, reliability, and security.
They also streamline data integration by creating pipelines to extract data, transform it into a usable format, and load it into a central repository. They assist you in setting up automated data validation and monitoring processes that govern your data’s quality, integrity, and compliance. Data engineers ensure that your data stack can scale to handle large volumes of fast-flowing data while maintaining high performance.
Data Scientists
Data scientists help you identify the types of data needed to answer specific business questions outlined in the strategy. They also influence the strategy by suggesting potential applications of data analysis for achieving business goals. Data scientists apply advanced analytics like statistical methods, machine learning models, and AI algorithms to extract relevant insights from your data. They conduct experiments and develop prototype data solutions to validate hypotheses and test new approaches.
Data scientists push the boundaries of what data can achieve, contributing to strategic advancements at your organization. They closely work with business leaders, product managers, and other stakeholders to bridge the gap between technical capabilities and achieving business objectives.
Data Analysts
Data analysts translate raw data into understandable reports and visualizations. They leverage BI and other data analytics tools for trend analysis, performance monitoring, and identification of key metrics. Data analysts also create interactive and real-time data dashboards that allow you to make informed data-driven decisions. They work with various departments, understand their data needs, and define the key questions that data strategy should answer.
Data analysts continuously track KPIs for discrepancies or inconsistencies, interpret them, and create reports providing context. Explaining the implications of data findings guides strategic decisions and ensures effective data utilization.
Summing up the points above, data engineers build the infrastructure, data scientists define the analytical approach, and data analysts translate the results into actionable insights. All these teams collaborate to develop and implement a robust data strategy successfully across all the departments of your organization.
How Can Airbyte Help with Your Data Strategy?
Airbyte is a no-code ELT data integration platform that can significantly enhance your data strategy by streamlining the data movement process. Traditionally, integrating data from various sources, such as cloud services, databases, and applications, involves manual transfers and custom coding. This increases the possibility of errors, leading to the wastage of computational resources, time, and effort.

Airbyte cuts through the complexity by providing an intuitive and user-friendly interface with a library of over 350 pre-built connectors to build streamlined data pipelines. Its Connector Development Kit also provides the flexibility to create custom connectors within minutes based on your tailored needs. Using the schema change management feature, you can also configure Airbyte to propagate and reflect the schema changes made at the source in your destination databases.
Airbyte allows you to seamlessly integrate with platforms like dbt (Data Build Tool) and perform complex data transformations within your existing workflows. It optimizes efficiency by supporting incremental data synchronization, allowing you to transfer only new or updated data since the last successful sync.
Airbyte’s ease of use encourages even non-tech teams to get used to the platform and perform operations quickly. Its vibrant community of 15k members provides your data experts a platform to interact with other data experts and seek help in your data integration journey.
From custom connectors to security to automating data movement, Airbyte takes care of it all, saving you time to focus on other high-level activities. Owing to the above features, Airbyte is loved by data engineers, scientists, and analysts.
You can further explore Airbyte’s features and offerings by referring to the official documentation.
FAQs
Q. What is an example of a data strategy?
An example of a data strategy is an e-commerce platform that uses customer data to personalize product recommendations and improve ROI. This strategy involves goal setting, collecting relevant data, and implementing tools to utilize it.
Q. What are the five components of a data strategy?
The five components of a data strategy are business strategy, organizational roles, data management, data architecture, and data analytics.
Q. How to build a data strategy?
Building a data strategy involves aligning business objectives with data collection, analysis, and governance to extract insights and drive informed decisions.
Q. What are the pillars of data strategy?
The pillars of data strategy include data governance, fostering a data-driven culture, and utilizing the right tools and technology.