What is Master Data Management (MDM): Benefits & Best Practices
In the intricate web of modern businesses, where data flows from several sources and systems, ensuring data accuracy and consistency can be daunting. Data collected at the source can be fragmented, outdated, or inconsistent, leading to missed opportunities. Therefore, maintaining up-to-date and consistent data across all touchpoints is imperative.
Master data management (MDM) is a crucial solution to these challenges. MDM helps consolidate key data entities such as customers, products, and suppliers into a single reliable source of information. The process ensures that all critical business data is precise and uniform, enabling better decision-making and improved operational efficiency.
This article lays out the core concepts of master data management (MDM) and explains how to implement it, its benefits, and more.
What is Master Data Management (MDM)?

Master data management (MDM) is a comprehensive method for managing enterprise data to provide a single reference point. MDM ensures the data is reliable, uniform, and easily accessible across the enterprise, supporting various functions and processes.
Through MDM, you can collect, integrate, maintain, and enforce governance practices on master data, including essential business entities such as customers, products, and suppliers. An effective MDM solution helps you eliminate data silos, reduce errors and improve data quality leading to better outcomes.
Benefits of Master Data Management
Master data management provides you with several advantages; here are some of them:
Data Quality and Consistency
You can consolidate data from multiple sources into an authoritative repository using MDM. This data standardization eliminates discrepancies and redundancies, making data more reliable and improving data quality.
Operational Efficiency
MDM helps you eliminate duplicate data records and streamline data access. By providing a unified and reliable dataset, MDM reduces the need for manual data reconciliation, leading to increased productivity and lower operational costs.
Provides a 360-degree View of Customers
Master data management integrates customer data, such as sales, support, and marketing, from various touchpoints into a unified repository. This integration consolidates information into a comprehensive view, enabling a better understanding of customer’s preferences and improved customer service.
Helps Meet Data Governance
MDM helps you establish data governance policies and ensure data integrity and compliance with organizational standards. It supports data stewardship, lineage, and quality management through structured practices, enhancing governance and accountability.
Helps with Data Compliance
MDM helps meet regulatory and industry compliance requirements by establishing robust data governance frameworks and enforcing organizational standards. It supports the tracking and documentation of data lineage, ensuring data is secure, consistent, and managed according to regulations. Compliance with regulatory standards and industry best practices reduces non-compliance risk and associated penalties.
Core Concepts of Master Data Management (MDM)
Master data management revolves around several key concepts that ensure the effective management and utilization of a business’s master data. Understanding these core concepts is essential for implementing a successful MDM strategy.
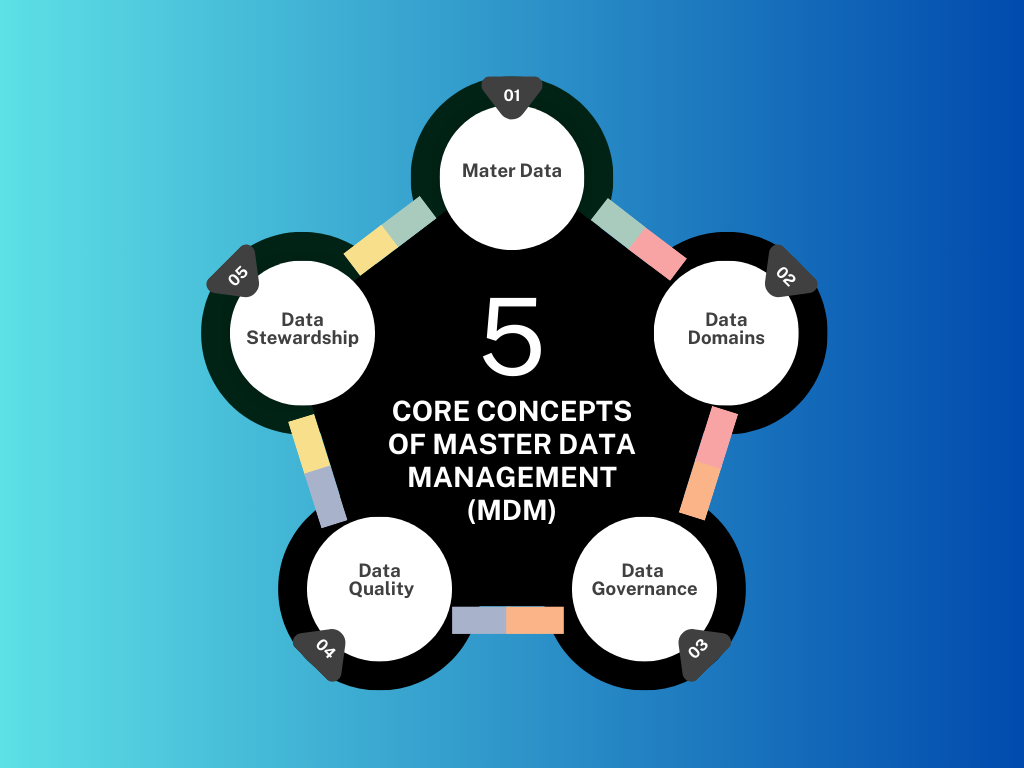
Master Data
Master data is the foundational and critical information about the key business entities. These entities include information about customers, products, and locations used across different systems and processes within an organization.
Data Domains
Data domains are specific categories of master data managed individually. Each domain focuses on a particular type of information, such as customer, product, or finance. Proper management of these domains is essential for maintaining high data quality and reliability across organizations.
Data Governance
Data governance includes the policies, standards, and procedures that guide the management of master data. It ensures the master data is handled in a way that meets organizational standards and compliance requirements, promoting data integrity and proper use.
Data Quality
Data quality focuses on the completeness of data, ensuring all necessary information is present. The higher the data quality, the more informed decisions you can make. MDM efforts aim to continuously monitor the data to improve data quality across the organization.
Data Stewardship
Data stewardship involves managing and overseeing an organization’s data assets. It includes tasks such as ensuring data quality, enforcing governance policies, and maintaining data integrity. Data stewards are responsible for implementing practices that ensure all data-handling practices align with organizational and regulatory requirements.
How Does the MDM Process Work?
The master data management framework ensures that all the information across the organization is semantically consistent. Here is a step-by-step breakdown of how the MDM process works:
Data Profiling and Discovery
Data profiling and discovery help you examine your data to understand its structure, content, and quality. It involves identifying anomalies such as missing values, inconsistencies duplicates, and understanding data sources to ensure the information is relevant.
Data Cleansing and Standardization
Data cleansing and standardization are essential to transform the raw data into a high-quality, consistent dataset ready for integration and analysis. This stage involves removing errors and inconsistencies, handling missing values, eliminating duplicates, and standardizing data formats.
Data Integration
Integration involves consolidating data from databases, applications, and systems in a centralized repository. This step ensures that all relevant data is accessible and can be analyzed together for better decision-making.
Data Enrichment
Data enrichment involves enhancing the existing information by supplementing information from external sources. This can include demographic details, market data, or other relevant information, making your data more valuable and informative.
Data Governance and Stewardship
Data governance and stewardship ensure that data management policies, standards, and procedures are followed. You define and implement data usage, quality, and security rules in this step. It includes assigning roles and responsibilities for data management and establishing protocols for data access.
Data Distribution
The final step of the MDM process is data distribution, where the cleaned, standardized, and enriched data is made available to users across the organization. This ensures everyone can access reliable data to perform tasks and produce accurate insights.
Master Data Management Use Cases & Examples
MDM helps improve data quality, operational efficiency, and regulatory compliance, enabling better analytics and reporting. The use cases below highlight MDM’s importance in enhancing business processes across various domains.
Customer Data Management
MDM helps you consolidate customer data from multiple sources, such as CRM systems, marketing platforms, and transactional databases, providing a 360-degree view of your customer data. This comprehensive view lets you personalize marketing and sales campaigns, improving customer engagement and satisfaction.
Example
A retail company used MDM to merge data from CRM systems, an email marketing platform, in-store sales, and online transactions. A consolidated view of each customer’s purchasing history, preferences, and interactions allows the company to analyze patterns, browning history, and engagement timing. The company can create personalized services, offers, and product recommendations by understanding these factors.
Product Data Management
Product data management is a sub-practice under MDM that involves organizing and maintaining product information throughout its lifecycle. It ensures all the information about the product is correct and uniform across multiple systems and channels within organizations.
Example
A global retail company can use MDM to manage its product catalog across multiple regions and sales channels. The company can set standard pricing and check logistics and inventory levels across multiple regions. This centralization of data streamlines operations and enhances overall product performance.
Compliance Data Management
It involves the processes and systems to ensure that an organization’s data handling practices meet legal, regulatory, and industry requirements. It focuses on maintaining data integrity, security, and privacy while adhering to relevant compliances.
Example
A healthcare organization uses MDM to manage compliance requirements by centralizing and standardizing patient data. The master data management systems help to ensure that all patient data is handled according to HIPAA and other regulatory necessary compliance, maintaining confidentiality and security across all systems.
Master Data Management Tools
The data management tools enable you to consolidate, clean, and manage master data across organizations, ensuring an accurate and up-to-date view of business-critical information. These tools also help you maintain data integrity and perform integration across various systems and applications.
Key Features of MDM Tools
- Data Integration: The tool should connect data from various sources and systems seamlessly.
- Data Cleansing: An MDM tool must have data cleansing functionalities to identify and resolve errors and duplicates to improve data quality and reliability.
- Scalability: A scalable tool can easily accommodate large datasets, ensuring that performance remains efficient as the data grows.
- Ease of Use: An MDM tool must have an intuitive user interface, including help resources, documentation, and training materials. Good support ensures that you can effectively navigate through and utilize the tool.
4 Best Master Data Management Tools
Airbyte
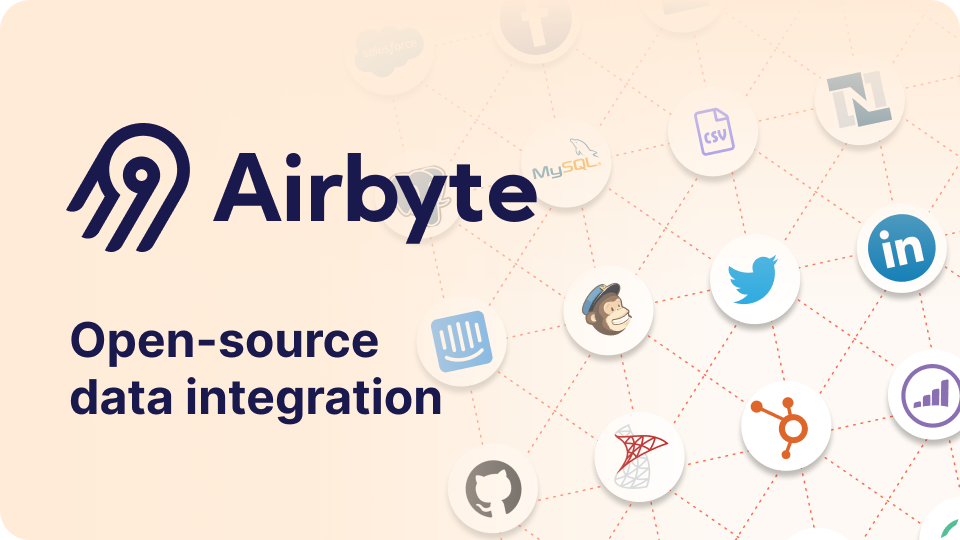
Airbyte is a robust and highly efficient data integration tool that streamlines MDM by consolidating data from different sources into a single platform. This makes the analysis and management of data effortless.
The platform offers a library of 350 pre-built connectors which you can use to build data pipelines without writing a single line of code. Airbyte also supports change data capture, allowing you to track and replicate changes in your source data. This feature helps to keep your master data updated at all times.
Key Features of Airbyte:
- GenAI Workflows: Airbyte simplifies AI workflows by allowing you to load unstructured data directly into vector store destinations. This helps in MDM by ensuring that data is systematically integrated and organized.
- Custom Connector Support: Using Airbyte’s Connector Development Kit, you can customize existing connectors or build new ones to meet your organization's unique requirements.
- Data Transformation: Airbyte supports data transformation using the ELT (Extract, Load, Transform) approach. It also offers integration with dbt for complex data transformations such as data cleaning and enrichment. Promptly applying transformations across all data sources helps maintain high-quality master data.
- Data Security and Governance: Airbyte adheres to important compliance standards in different deployments, including ISO 27001, GDPR, SOC2 HIPAA, and more. This assures you of data security and integrity while performing integration during MDM.
Hevo

Hevo is a no-code data integration platform that facilitates efficient MDM by ensuring seamless, real-time data transfer between sources and destinations. Its automated data pipeline simplifies the integration process, which helps in master data management by combining and transforming data across different systems, making your data analysis ready.
Key Features of Hevo:
- Flexible Integrations: Hevo supports many data sources and destinations, allowing easy and versatile data integration across different platforms. This flexibility ensures that all the relevant data is consolidated, aiding in creating a unified master data view.
- Data Transformation: Hevo offers robust transformation capabilities, which allow you to clean, enrich, and transform data as it moves through your pipeline. Through transformation, your master data can be optimized for analysis.
- Real-time Data Synchronization: Hevo provides real-time data synchronization between source and destination, ensuring timely and accurate data availability.
Boomi

Boomi is an industry-leading integration platform and service that connects applications and data across diverse environments. Its cloud-native architecture supports comprehensive data integration, API management, and workflow automation. Boomi aids master data management by helping you unify information from diverse sources, upholding quality, and providing real-time updates. It helps streamline data governance and enhance overall efficiency.
Key Features of Boomi:
- Comprehensive Integration: Boomi supports integration across multiple applications, databases, and systems, whether on-premises or in the cloud. This flexibility allows you to transfer master data between disparate systems quickly.
- API Management: The platform offers robust API management capabilities, allowing you to create, publish, and manage API securely for easy third-party integrations.
- Scalability: Boomi can handle businesses' growing needs, scaling seamlessly as data volumes and integration requirements increase.
Fivetran
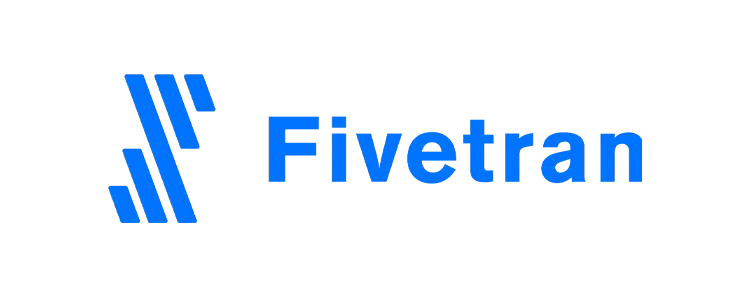
Fivetran is a data integration platform optimized to automate and simplify the management of data pipelines. It helps reduce the complexities of data integration, enabling you to move master data efficiently between different systems. By automating the ETL process, Fivetran minimizes the need for manual oversight and maintenance, making sure your data is current and updated. This enhances data reliability and usability across the organization, facilitating effective master data management.
Key Features of Fivetran:
- Automated Data Pipeline: Fivetran automates the end-to-end process of data extraction, transformation, and loading, minimizing the need for manual intervention.
- Pre-Built Connectors: The platform provides over 300+ pre-built connectors for various sources and destinations, simplifying integration and accelerating deployment.
- Real-time Data Sync: Fivetran utilizes incremental data loading, providing near real-time data updates to ensure that the data in your warehouse is as current as possible.
How to Implement MDM In Your Organization?
Here are some ways you can easily implement master data management in your organization:
Evaluate Existing Data Management Practices
Assess the current data management processes and identify gaps or inefficiencies. Understanding these practices will help you implement an MDM strategy addressing your organization's needs.
Gain Support from C-level Executives
Secure commitment from senior management to ensure you have adequate resources and strategic alignment. Executive support is crucial for driving the initiative and overcoming potential resistance.
Identify Focus Data Domains
Determine which data domains such as customer, product, supplier, sales, or others are essential for your business and prioritize them. Focusing on critical domains ensures that the MDM implementation will deliver value where it's most needed.
Define Roles and Responsibilities
Clearly outline the roles and responsibilities of managing the master data. Assigning specific roles such as data governance lead, MDM system administrator, integration specialist, and more helps ensure accountability and effective oversight throughout the MDM process.
Create Data Governance Policies
Develop data governance policies to establish data quality, usage, and management rules. Robust governance frameworks are essential for maintaining data integrity and compliance.
Design MDM architecture
Plan and design the MDM architecture to support data integration, quality, and access. It ensures that all components work together effectively and scale with organizational needs.
Evaluate and Select the MDM Tool
Assess MDM tools based on your organization’s requirements and select the best fit for your needs. The right tool is essential for successfully implementing the ongoing data management process.
Create a Unified Data Model for Master Data
Developed a unified data model to standardize and integrate master data across the organization. A cohesive model ensures consistency and accuracy in representing and using data.
Implement Data Quality Processes
In this step, you establish a process for monitoring and improving data quality. Regularly cleansing and validating data helps maintain its reliability and usefulness for decision-making.
Integrate Data Sources
Connect and consolidate data from multiple sources into a centralized MDM system. Effective integration facilitates a single source of truth and improves data accessibility.
Implement MDM in Phases
Roll out the MDM implementation in stages to manage complexity and address issues incrementally. The phased implementation allows for adjustments based on feedback and reduces the risk of widespread disruption.
Challenges with Master Data Management
Here are some challenges that one can encounter while implementing master data management:
- Data Standard Complexity: Establishing a standardized data format is challenging as it requires aligning diverse data types and sources with consistent criteria such as naming conventions and database fields.
- Project Scoping Issues: Defining and controlling the scope of an MDM project is crucial; a poorly managed project can become unwieldy, leading to delays and cost overruns.
- Integration of Data: The rise of big data adds to the difficulty of managing the master data, which is integrated from multiple business applications and sources. This complexity heightens the inclusion of unstructured and semi-structured data, which requires sophisticated management techniques.
- Data Stewardship: Poor data stewardship can hinder MDM deployment and long-term data management by failing to maintain data accuracy, leading to unreliable data.
Summary
Master data management is vital for maintaining accurate, consistent data across organizations, supporting better decision-making and operational efficiency. By consolidating key data entities into a single, reliable source, MDM enhances data quality and management, eliminating silos.
To implement an effective master data management process, you must follow the steps of data profiling, cleansing, integration, enrichment, and distribution. Utilizing tools like Airbyte can streamline this process, ensuring successful MDM implementation.
FAQs
What are the four styles of MDM?
Master data management can be implemented in four styles: the standard registry model, consolidation model, centralized model, and coexistence model.
What tech stack should you use when building a Master Data Management system?
A well-rounded tech stack ensures scalability, flexibility, security, and efficient data handling when building a master data management system. Tools like Airbyte, Boomi, and Hevo can be used to create a robust MDM system.
Who is responsible for master data management?
Master data management involves multiple roles and responsibilities, including those of the MDM program manager, data governance analyst, data steward, data architect, and more.
When do we need master data management?
Master data management is needed when an organization faces challenges with data accuracy, completeness, and consistency across systems. The process helps you consolidate critical data into a reliable source, improving decision-making.