Next-Gen AI Data Integration: Top 10 Platforms to Know
Generative AI has captured the spotlight for its creative applications—but it’s also reshaping how modern data teams build and manage pipelines. In the world of data integration, GenAI is being used not just to generate text or images, but to automate repetitive tasks, accelerate development workflows, and make systems smarter and more resilient.
Data integration platforms are starting to embed generative AI into their core features, offering capabilities like auto-generated connectors, SQL assistance, schema inference, and even natural language pipeline creation. This AI-based data integration enhances operational efficiency in manufacturing, improves patient outcomes in healthcare, streamlines risk management in finance, and optimizes supply chain visibility in logistics. The result? Faster time to value, fewer bottlenecks, and a new layer of intelligence woven into the data stack.
In this roundup, we’ll highlight 10 data integration tools using generative AI in meaningful ways. Whether it’s an open-source framework or an enterprise-grade platform, each offers a glimpse into how AI is evolving the way we move and transform data.
Introduction to Data Management
Data management is the backbone of any modern business, encompassing the processes of collecting, storing, organizing, and maintaining data to ensure its accuracy, security, and accessibility. Effective data management is crucial for making informed decisions, improving operational efficiency, and gaining a competitive edge in the market.
A critical component of data management is ai data integration, which enables organizations to combine data from disparate sources into a unified view. This process involves extracting data from various sources, transforming it into a consistent format, and loading it into a target system or database. By integrating data, organizations can ensure data quality, integrity, and security, while also complying with regulatory requirements.
Data management processes can be significantly enhanced through automation using advanced data integration tools and technologies, such as artificial intelligence (AI) and machine learning (ML). AI-powered data integration can help organizations improve data quality, reduce errors, and increase efficiency, making it an essential aspect of modern data management.
Why AI-Powered Data Integration Is the Next Evolution of the Modern Data Stack
Data integration has always been a cornerstone of the modern data stack. But as the number of data sources continues to grow—and the demand for real-time insights accelerates—manual integration approaches and rigid pipelines just can’t keep up.
AI enhances data integration initiatives by streamlining and optimizing the process, making it more accessible and cost-effective for businesses, especially those with limited resources.
That’s where AI-powered data integration platforms come in.
These next-generation tools use generative AI and machine learning to help teams build, manage, and scale data pipelines with greater speed and less effort. Whether it’s auto-generating connectors, inferring schemas, writing SQL transformations, or identifying pipeline issues before they happen, AI is making data integration more intelligent and developer-friendly.
And for platforms like Airbyte, generative AI isn’t just a layer—it’s reshaping how ai data integration processes are built, deployed, and maintained.
What Are AI-Powered Data Integration Tools?
AI-powered data integration tools are platforms that combine traditional ETL/ELT capabilities with large language models (LLMs) and other forms of artificial intelligence. The result is a smarter, more efficient way to sync, transform, and monitor data across systems.
These tools often support:
- Natural language interfaces for building or editing pipelines
- AI-generated SQL and transformation logic
- Automated schema mapping and inference
- Self-healing or anomaly-detecting workflows
- Connector generation using LLMs—like Airbyte’s AI Connector Builder
- AI-powered data discovery to enhance the efficiency and accuracy of discovering and cataloging data
In short: these platforms reduce the manual work of integration, help scale data operations faster, and empower engineers (and non-engineers) to work with data more easily.
Suggested Read: AI ETL Tools
Why Use an AI-Driven Integration Platform?
Traditional data integration tools have long helped teams automate data movement—but AI takes it a step further by optimizing the entire workflow.
With generative AI embedded into the core product, data teams can:
- Reduce development time by automatically generating connectors and transformation logic
- Accelerate onboarding for new data sources with minimal manual config
- Debug faster using intelligent suggestions and code assistants
- Improve data quality and reliability with anomaly detection and automated validation
- Scale with fewer engineering resources, especially as your data ecosystem grows
- Analyze data from various sources to enhance decision-making
Platforms like Airbyte combine open-source extensibility with these AI-native features, giving teams both flexibility and efficiency.
AI-Powered Data Integration vs. Traditional Tools
The main difference between traditional and AI-powered integration tools lies in who does the heavy lifting.
- Traditional tools rely heavily on manual setup, custom code, and rigid workflows. As data volume and complexity grow, these traditional methods become inadequate, struggling to maintain performance and scalability. AI-powered tools use LLMs and machine learning to infer intent, suggest solutions, and automate repetitive steps.
It’s not just about faster pipelines—it’s about building smarter, more adaptive systems that reduce operational overhead and unlock new capabilities for technical teams. AI data integration further enhances these capabilities by providing more intelligent and efficient ways to manage complex data sets and streamline integration processes.
Types of AI-Powered Data Integration Tools
While the core goals are the same—move and prepare data efficiently—AI-driven platforms tend to offer a mix of traditional functionality enhanced with intelligent features. Choosing the right data integration solution is crucial for efficient data management, especially with increasing data volumes and complexity. Common categories include:
- AI-Augmented ETL/ELT Platforms: Tools that use LLMs for transformation suggestions, SQL generation, or connector creation (e.g., Airbyte, Ascend, Databricks)
- Smart Data Orchestration Tools: Platforms that assist with scheduling, error handling, and optimization using AI
- Natural Language Pipeline Builders: Interfaces that let users define data workflows using plain English
- Real-Time Monitoring and Validation Engines: AI models that flag data quality issues or failed syncs proactively
In practice, many of the best platforms combine several of these elements in a single offering.
What to Look for in an AI-Powered Data Integration Platform
If you’re evaluating platforms that claim to use generative AI or intelligent automation, here are key capabilities to consider:
- Connector Intelligence: Can it help you generate or customize new connectors using AI?
- Schema Flexibility: Does it adapt to changing data structures automatically?
- Transformation Assistance: Can it suggest or auto-generate SQL or logic based on your goals?
- Deployment Options: Is it open-source? Cloud-native? Can you run it in your own environment?
- Monitoring & Observability: Are pipeline issues flagged proactively with suggested fixes?
- Community and Ecosystem: Is there active development, support, and room for extensibility?
- Ease of Use: Does the AI help developers move faster, or just add complexity?
- Data Access: Does the platform address challenges like data silos and improve seamless access to information across the organization through effective data governance and integration of various data sources?
- Advanced Analytics and Insights: Does the platform offer advanced analytics that can help identify patterns, trends, and correlations in data, making it possible to predict future events and make data-driven decisions?
Platforms like Airbyte focus on all of the above, with the added benefit of being open-source and extensible, allowing you to build exactly what you need—without lock-in.
To help you explore what’s out there, here are 10 data integration platforms that are putting generative AI to work in meaningful ways—each with unique capabilities designed to streamline, accelerate, and modernize how you move and manage data.
1. Airbyte
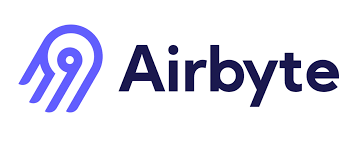
Overview: Airbyte is an open-source data integration platform that helps teams consolidate data from hundreds of sources into data warehouses, lakes, and tools. It offers self-hosted and cloud offerings and is known for its modular design, active community, and developer-first approach.
How it uses Generative AI: Airbyte was among the first platforms to apply generative AI directly to the connector development. Its AI-powered Connector Builder uses large language models to help users generate custom connectors using natural language input. It also integrates with tools like LangChain to support knowledge retrieval use cases and offers a Copilot-like dev experience for writing and debugging connector code.
Additionally, Airbyte ensures seamless data integration by leveraging AI-driven solutions to connect modern and legacy systems effectively, ensuring compatibility across various data formats and protocols.
Key Features:
- AI-powered custom connector generator
- 550+ pre-built connectors and growing via open-source contributions
- Integration with modern data stack tools like dbt, Airflow, and Dagster
- Support for both batch and CDC (change data capture) syncs
Best for: Data and analytics engineers looking for a flexible, open-source integration solution with built-in AI tooling for rapid connector development.
2. Databricks
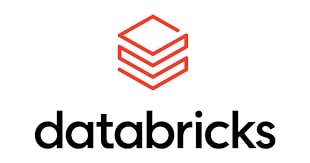
Overview: Databricks is a unified data analytics platform built on a lakehouse architecture that combines the best data warehouses and data lakes. Known for its robust data engineering, machine learning, and real-time analytics capabilities, it’s widely adopted by enterprises managing large-scale data pipelines.
How it uses Generative AI: Databricks integrates generative AI into its platform through tools like Databricks Assistant, an LLM-based coding companion embedded directly in notebooks. It helps users generate SQL queries, debug code, and build pipelines using natural language input faster.
This improves developer productivity and lowers the barrier to entry for less experienced users. Additionally, Databricks enhances business intelligence by leveraging AI-powered tools to consolidate data from diverse sources, streamline decision-making processes, and uncover actionable insights.
Key Features:
- Databricks Assistant for AI-powered SQL and code generation
- Delta Live Tables for declarative data pipeline creation
- Tight integration with MLflow, Unity Catalog, and open-source AI models
- Scalable for batch and streaming workloads
Best for: Teams managing complex, high-volume data workflows who want to blend data engineering, analytics, and ML development in a single platform, with GenAI support to streamline development.
3. Ascend.io
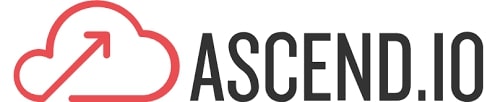
Overview: Ascend.io is a fully managed data pipeline platform designed to automate and orchestrate data workflows with minimal engineering overhead. Built on a declarative framework, it abstracts away much of the manual effort involved in managing pipelines, transformations, and infrastructure.
How it uses Generative AI: Ascend recently introduced the AI Data Assistant, which uses large language models to help users create and manage data transformations through conversational interfaces.
It allows users to write SQL with natural language, troubleshoot issues, and accelerate development cycles with AI-generated recommendations. Additionally, Ascend.io automates data integration tasks, enhancing efficiency and accuracy by streamlining the integration of data from various sources and addressing challenges like data silos.
Key Features:
- Declarative data pipeline orchestration with built-in change detection
- AI-powered SQL generation and transformation logic
- Native integrations with platforms like Snowflake, Databricks, and BigQuery
- Automated lineage tracking and pipeline monitoring
Best for: Data engineers and analysts who want to move fast, reduce manual pipeline work, and utilize GenAI to iterate on data workflows efficiently.
4. Nexla

Overview: Nexla is a no-code/low-code data operations platform for automating data integration and preparation across teams and systems. It offers engineers, analysts, and business users a unified environment to collaborate on real-time data pipelines.
How it uses Generative AI: Nexla uses AI to power its concept of Nexsets—intelligent, schema-aware data objects that abstract complexity for users. These are automatically generated and updated as data flows through systems.
The platform also uses AI to assist with schema mapping, data normalization, and error detection, minimizing manual intervention. Additionally, Nexla leverages AI to create intelligent data mappings, enabling efficient data integration and empowering non-technical users to expedite decision-making.
Key Features:
- Auto-generated Nexsets for seamless schema management
- AI-powered data mapping and transformation
- Role-based interfaces for engineers, analysts, and ops
- Real-time data syncs across apps, databases, and APIs
Best for: Teams that need to scale data operations across business units and want a no-code interface backed by AI-driven automation and monitoring.
5. Keboola
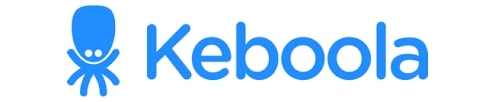
Overview: Keboola is an all-in-one data platform that combines data integration, transformation, orchestration, and governance in a single environment. Designed with modularity, it enables teams to build robust data workflows without heavy infrastructure management.
How it uses Generative AI: Keboola incorporates generative AI to assist users in writing transformation code, managing orchestration tasks, and automating documentation.
The platform also leverages AI to recommend pipeline optimizations, improve data lineage tracking, and reduce manual data engineering effort through predictive configuration and task generation. Additionally, Keboola monitors and optimizes data usage through its AI-powered features, ensuring compliance and efficient data management.
Key Features:
- End-to-end data pipeline management with built-in orchestration
- AI assistance for code generation, task recommendations, and documentation
- Flexible integrations with Snowflake, BigQuery, Redshift, and more
- Governance and auditing features for enterprise-grade compliance
Best for: Data teams that want a full-stack data operations platform with the added benefit of AI-driven acceleration and collaboration across engineering and business users.
6. Mozart Data

Overview: Mozart Data is an all-in-one modern data stack that simplifies data infrastructure for startups and growth-stage teams. It bundles the tools needed for data ingestion, transformation, and orchestration into a streamlined, low-maintenance platform.
How it uses Generative AI: Mozart integrates generative AI features to help users auto-generate SQL queries, optimize transformations, and debug pipelines using natural language prompts.
These capabilities are designed to help less technical users interact with data more confidently while speeding up the analytics workflow for experienced analysts and engineers. Additionally, Mozart Data's AI-powered features identify and extract relevant data from various sources, minimizing human involvement and reducing errors and costs while maintaining efficiency and accuracy in data integration.
Key Features:
- Built-in ELT pipeline management with a no-code UI
- AI-assisted SQL generation and transformation suggestions
- Warehouse integrations with Snowflake and dbt
- Pre-configured modern data stack with minimal setup required
Best for: Startups and lean teams looking to set up a production-ready data stack quickly, with the bonus of AI support to simplify analysis and pipeline maintenance.
7. Estuary

Overview: Estuary is a real-time data integration and streaming platform built on open standards like CDC and Apache Flink. It’s designed to help teams move data continuously and reliably across sources, from databases to SaaS platforms.
How it uses Generative AI: Estuary is in the early stages of embedding generative AI into its development workflows, with efforts focused on schema evolution assistance, stream configuration guidance, and real-time transformation logic generation.
These features aim to reduce the complexity of managing streaming pipelines and adapt to data changes on the fly. Additionally, Estuary enhances data extraction through AI-powered features, improving the efficiency and accuracy of extracting relevant information from diverse data sources, including unstructured formats like text and images.
Key Features:
- Real-time data capture and stream processing
- Early-stage LLM integrations for transformation and schema guidance
- Low-latency syncs with built-in fault tolerance
- Support for both batch and stream-based sources and destinations
Best for: Engineering teams building real-time or event-driven data architectures who want AI assistance layered over a robust streaming backend.
8. Prophecy.io

Overview: Prophecy.io is a low-code data engineering platform that brings visual development to Spark, Airflow, and other enterprise-grade processing engines. It allows teams to build production-grade pipelines quickly while maintaining underlying code visibility and version control.
How it uses Generative AI: Prophecy integrates LLMs to help users write SQL transformations, generate DAGs, and edit code with natural language prompts. These GenAI capabilities are tightly embedded in its low-code builder, giving data teams a more intuitive way to iterate on workflows while preserving complete control over execution. Additionally, Prophecy.io automates data transformation mapping, significantly enhancing the efficiency and accuracy of data integration tasks.
Key Features:
- Visual pipeline builder with underlying Spark and Airflow support
- AI-powered SQL generation, code suggestions, and pipeline scaffolding
- Git-native environment for version control and collaboration
- Seamless handoff between visual and code-based editing
Best for: Enterprises that want to accelerate pipeline development without sacrificing flexibility or code-level transparency.
9. Coalesce.io
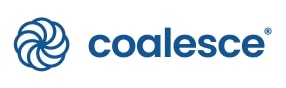
Overview: Coalesce is a data transformation platform that is purpose-built for cloud data warehouses, especially Snowflake. It combines a visual interface with column-level lineage and automation to speed up modeling and transformation workflows.
How it uses Generative AI: Coalesce is integrating generative AI to help users auto-generate SQL, build models from metadata, and recommend transformation logic based on table structures and previous pipeline patterns. By leveraging historical data, Coalesce can identify anomalies, predict data quality issues, and suggest corrections, enhancing the reliability and efficiency of data integration processes. This reduces time spent on boilerplate modeling work and helps teams scale warehouse transformations with less friction.
Key Features:
- Visual transformation UI layered over SQL
- AI-powered model generation and transformation assistance
- Deep Snowflake integration for fast execution
- Column-level lineage and metadata-driven design
Best for: Data teams building and maintaining complex warehouse models who want intelligent automation without abandoning SQL-first workflows.
10. Informatica

Overview: Informatica is a renowned data integration and cloud data management company that has integrated generative AI into its platform to enhance data processing and management capabilities.Informatica
How it uses Generative AI: Informatica's AI engine, CLAIRE, employs machine learning and generative AI to automate data discovery, cataloging, and lineage tracking. This enables users to gain deeper insights and streamline data governance processes.
Key Features:
- AI-driven data cataloging and metadata management
- Automated data quality assessments
- Comprehensive data governance tools
- Cloud-native architecture for scalability
Best for: Enterprises aiming to leverage AI for enhanced data governance, quality, and integration across hybrid and multi-cloud environments.
Implementation and Best Practices
Successfully deploying an AI-powered data integration strategy requires more than just plugging in a tool—it’s about creating a well-orchestrated system that can ingest, map, transform, and analyze data across diverse data sources. Whether you’re working with structured, semi-structured, or unstructured data, a thoughtful implementation approach will help improve data quality, reduce data silos, and ensure seamless integration across platforms.
To get the most out of your data integration initiatives, follow these proven best practices:
- Start with strategic planning: Define business goals and identify the data sources, data sets, and data structures critical to your organization. Map out data usage, desired outcomes, and any legacy systems you must integrate.
- Build a cross-functional data team: Successful integration efforts require collaboration between data engineers, architects, analysts, and business users. These teams help manage data ingestion, design pipelines, and develop intelligent data mappings tailored to your business logic.
- Choose the right data integration solution: Look for AI-based data integration tools that support real-time data processing, natural language processing (NLP), and machine learning capabilities. These technologies help automate data discovery, speed up data mapping, and flag data quality issues like anomalies and inconsistencies.
- Design for flexibility and scale: Ensure your data integration architecture can handle complex data sets, different data formats, and raw data from both on-premise and cloud environments. If you're managing streaming data or real-time customer data, prioritize platforms with low-latency data transformation and data access features.
- Test and validate early: Before going live, run end-to-end testing of your data integration processes—this includes validating data transformation mapping, checking for data security and access controls, and confirming that you can derive accurate business insights from the integrated data.
- Enforce governance and security: Implement robust data management processes that include data governance, compliance, and access control mechanisms. This is especially critical when handling sensitive data like patient or electronic health records.
- Monitor, optimize, and evolve: Post-deployment, continuously monitor your integration processes to identify bottlenecks, poor performance, or poor data quality. Leverage AI systems for anomaly detection and predictive analytics to maintain high performance and support advanced analytics and business intelligence use cases.
- Consider cloud-native platforms: Cloud-based data integration solutions offer built-in scalability and cost-efficiency. They’re ideal for integrating data lakes, performing automated data discovery, and enabling faster data mapping across global teams.
By following these best practices and leveraging AI-driven data integration capabilities, organizations can move beyond traditional methods and build a future-ready foundation for accurate data analysis, improved decision-making, and scalable data management.
Wrapping Up: Build Smarter Pipelines with Airbyte + Generative AI
Generative AI is no longer a novelty—it’s a practical force driving real change in how data teams operate. From reducing the manual effort of connector creation to streamlining transformations and improving pipeline resilience, AI-driven data integration is transforming data integration into a faster, more intelligent process.
If you’re looking to integrate these capabilities into your workflows, Airbyte offers a powerful, open-source platform built with flexibility and extensibility in mind—and now supercharged with generative AI.
With 550+ connectors, an AI-powered Connector Builder, and deep integrations with the modern data stack, Airbyte helps you move faster without giving up control.
Start building smarter, AI-enhanced pipelines today—get started with Airbyte Cloud or explore the open-source project.