Qdrant Vs Pinecone - Which Vector Database Fits Your AI Needs?
Vector databases are specialized systems that enable you to interact with abstract data representations generated by machine learning models such as deep learning. These representations are called vector embeddings. The embeddings are a compressed version of large data that is used to train AI models to execute tasks like sentiment analysis or speech recognition.
Qdrant and Pinecone are two of the best vector databases. Qdrant comprises features like scalable search and advanced filtering, and Pinecone is known for its high-performance similarity search.
This article will outline Qdrant vs Pinecone differences, along with their unique benefits and use cases.
Brief Overview of Qdrant
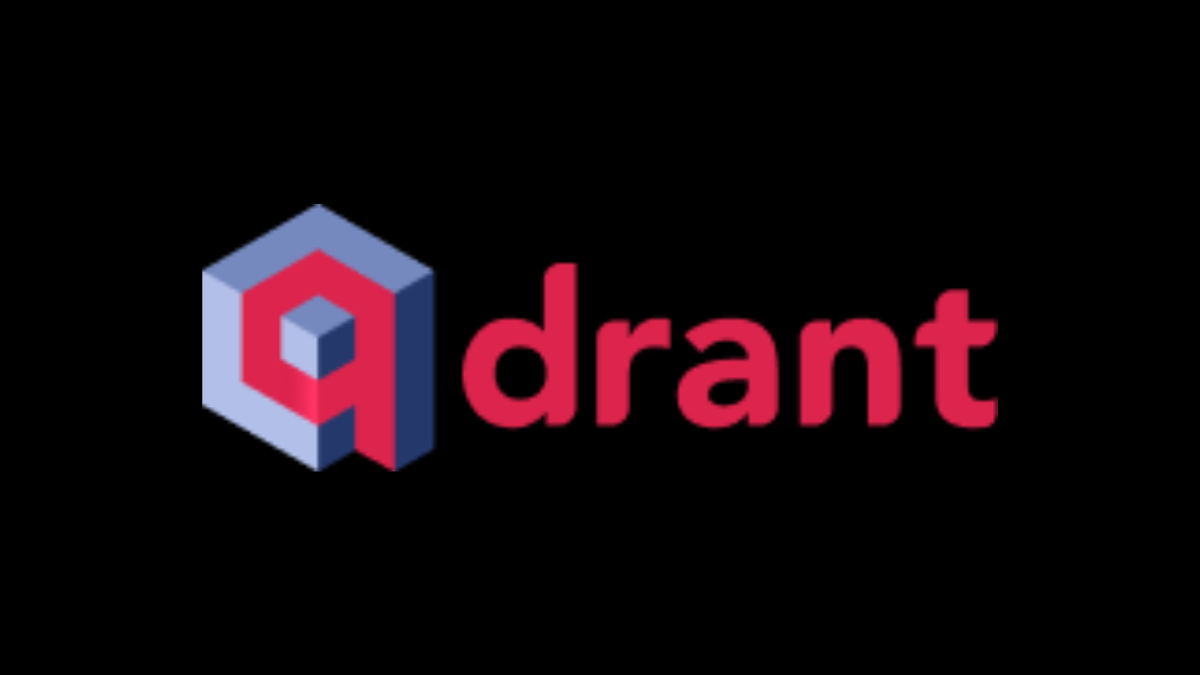
Qdrant is the industry’s first vector database that can be used in a managed hybrid cloud model in addition to its Qdrant Cloud and Docker node models. It specializes in similarity search and offers features like production-ready service that allows you to store, manage, and search data with additional payload. Payload is the additional information attached to your vector representation, which helps to enhance your search and provide relevant information to users.
Key Features and Functionalities
Here are some of the key features and functionalities of Qdrant:
Filtering
Qdrant allows you to set conditions for your search and retrieve operations. Filtering becomes essential when you can not describe the features of your object within the embedding. You can apply the following filtering options:
- Filtering Clauses: This feature allows you to combine conditions using different logical operations, including OR, AND, and NOT.
- Filtering Conditions: You can apply different conditional queries to different values within a payload. For instance, a condition can be applied to the payload to check if the stored value matches the results of the given query.
Hybrid Queries
Hybrid queries enable your database system to retrieve highly relevant results by considering two aspects: the proximity of vector embeddings and predefined filters. You can perform hybrid queries by blending similarity-based searches with conditions like metadata filtering.
Recommendation Searches
Qdrant offers APIs that help you find vectors similar to or different from each other. The results, as well as the information generated, are useful for recommendation systems and data exploration. For instance, Qdrant’s recommend API allows you to apply search based on positive and negative values.
Indexing
Indexing speeds up vector search. Qdrant supports different types of indexing, including vector dex, full-text index, payload index, tenant index, and more. The combination of vector and traditional indexes improves data filtering and retrieval.
Quantization
Quantization is a process of compressing the data while preserving the essence of the original data. Quantization is generally used to accelerate the search process in high-dimensional space. Qdrant offers different quantization methods, including scalar, binary quantization, and product quantization.
Applications of Qdrant
- Retrieval Augmented Generation (RAG): AI models use the RAG technique to generate more relevant outcomes derived from the information from a database system. You can leverage Qdrant’s search and filtering features to amplify retrieval augmented generation by accessing apt vector data values for better results.
- Data Analysis: Using the Qdrant similarity search engine, you can optimize your vectors to quickly identify patterns in complex datasets. This enhances the process of data analysis.
- Recommendation Systems: You can create highly responsive recommendation systems using Qdrant’s recommend API. It offers the flexibility of using multiple vectors in a single query, which increases output relevancy.
Practical Use Case of Qdrant
Anomaly detection is quite difficult as it involves patterns and outliers in the data, indicating possible issues or irregularities. Agrivero.ai utilizes AI-enabled solutions to check the quality of coffee beans and traces of green coffee within those beans for producers and traders. The organization collected and labeled more than 30 thousand coffee bean images with various defects. The data collected is used to train a classifier to evaluate crop quality and highlight problems.
How Qdrant Helps
Agrivero.ai uses Qdrant to find underlying anomalies and improve the quality of coffee. Here’s how this works:
- The coffee bean images are converted into vector embedding using a neural network model and are stored inside the Qdrant database.
- When the new images are collected, the database generates and queries with this new data, comparing it to the old data stored within it. The unusual data points or deviations are flagged based on similarity.
Follow this step-by-step guide to set up the Qdrant Database and optimize your business operations: A beginner’s guide to Qdrant.
Brief Overview of Pinecone

Pinecone is a cloud-native and managed vector database. You can optimize Pinecone to store and query the vector embeddings. It provides you with long-term memory, which is helpful for high-performance AI applications. The database also offers low-latency query results, scaling billions of vectors at a quick speed.
💡Suggested Read: Pinecone Vector Database Features You Can Unleash with Airbyte
Key Features and Functionalities
Here are some of the key features and functionalities of the Pinecone vector database:
Fully Managed
Pinecone offers you a fully managed SaaS service that handles the complexities of infrastructure management while you can focus on tasks such as building applications. This helps optimize resources and performance and increase productivity.
Serverless and Pod Architecture
You can run your vector database in two different settings within Pinecone. The first is serverless architecture, a managed service on the AWS platform that provides automatic resource scaling. Second is pod architecture, which uses preconfigured hardware units to host and execute services. You can run Pinecone pod architecture on Azure, GCP, or AWS.
Hybrid Search
Pinecone supports dense and sparse vector values, which allows you to perform hybrid search on the Pinecone index data. Hybrid search amalgamates semantic and keyword search in a single query to produce more relevant outputs.
Metadata Filtering
With Pinecone’s metadata filtering, you can limit the vector search. The database enables you to attach a metadata key-value pair with the vector point within an index and specify the filter expression during querying. These searches retrieve the exact information that matches with the filter query, improving efficiency.
Pinecone Assistant
Pinecone Assistant service allows you to upload documents, ask questions, and receive responses based on your documents. At the time of upload, your Pinecone Assistant processes the contents within the documents into vector embedding. It queries the vector embeddings as per your input and provides accurate information.
Applications of Pinecone
- Similarity Search: With Pinecone, you can apply similarity searches within your database systems and generate the most accurate results based on the meaning and context of data.
- Natural Language Processing Tasks: Pinecone enhances various NLP tasks, including text classification, sentence similarity, and summarization, using its search capabilities.
- Real-time AI-Powered Applications: The low latency search capabilities of Pinecone make it ideal for developing real-time applications. These systems help you quickly retrieve information and provide timely responses.
Practical Use Case of Pinecone
Chatbot applications have been used for a long time now. These applications are trained on the latest AI models to generate relevant responses to user questions. However, the main challenge that chatbots face is that they can’t retain information for long periods of time. There is a limit to how much context a certain model can support.
Pinecone has no context limits. This vector database provides the chatbot models with a data store that can retain context information for a longer period of time.
How it Works
- The first step is to pull the data from a data warehouse or the storage system and generate vector embeddings.
- The second step is to save these vector embeddings in Pinecone.
- The third step is where the application embed queries using an AI model to create a query vector.
- The embedded query is then used to search through the Pinecone database and provides results using similarity search algorithms.
- The results generated through the search algorithm are attached to the original query in the form of text context. These text prompts are then sent to the GenAI chatbot application for more grounded responses.
Core Feature Comparison: Qdrant vs Pinecone
The main difference between Qdrant and Pinecone is that Qdrant is an open-source vector search engine designed for high-performance similarity searches, while Pinecone is a managed vector database service optimized for scalable, real-time machine learning applications.
Pinecone and Qdrant are versatile vector database solutions, but they differ significantly in their architecture and technical capabilities. Below are the key differences between Pinecone vs Qdrant that you can consider when choosing the database that aligns with your requirements:
Here is a detailed explanation of the key comparative features of Qdrant and Pinecone:
Deployment Model
Qdrant supports multiple deployment options, including a local Docker node, Qdrant Cloud, and Hybrid Cloud. This allows you to deploy your project in an environment that best suits your requirements.
Pinecone, on the other hand, is a fully managed SaaS solution. To use Pinecone, you need to connect your deployment environment to the cloud service it offers, making it less flexible in terms of deployment compared to Qdrant.
Storage Model
Qdrant offers two types of storage models for its vector and payload data. The in-memory storage stores all your vectors and payloads within RAM. This type of storage works quite fast. You can create an on-disk storage model for vector data. It creates virtual address spaces associated with the file on the disk and uses a page cache to access the file’s content. Similarly, the payload can also be stored using in-memory storage, where the data is loaded into RAM and disk, and RockDB is used for persistence. Where RockDB is a key-value pair-storing system.
Comparatively, Pinecone provides in-memory storage that allows you to scale storage without manually configuring storage options. It also provides persistent cloud-based storage for long-term durability, ensuring data safety and recovery.
Performance
Qdrant provides you with different performance profiles for specific use cases. It offers vector and payload indexing, optimizers, low latency queries, and quantization for better performance output.
On the flip side, Pinecone increases the number of replicas to boost the throughput of pod indexes. It automatically scales the resources, deriving high throughput for serverless indexes and partition records for the namespace to decrease latency for a single index.
Hybrid Search
You can implement a hybrid search in Qdrant using Query API. This API enables you to build a search system that combines different search methods, providing flexibility and improving retrieval performance.
In Comparison, Pinecone uses a combination of sparse and dense vector values to perform a hybrid search. This method helps you construct a single query for semantic and keyword, generating more relevant results. While this is easier to implement, it lacks customization like Qdrant.
Security Considerations
Qdrant allows you to tailor security configurations specific to your deployment environment. It supports an API key for client authentication and read-only API to access read-only operations in your instance. In addition, you can generate JSON web tokens and implement TLS encryption for connections. You can also build role-based access control according to your application requirements.
In a similar vein, Pinecone also offers features like role-based access control. It also provides an AWS private link to keep your VPC private from the public internet and API keys for authentication.
Pricing Models
The price to employ Qdrant depends on the type of deployment you choose for your application. It provides you with a free tier, hybrid, and private Qdrant cloud. The free tier Qdrant cloud is sufficient for 1M 768-dimensional vectors without any limitations on collections used. For the Hybrid Cloud and Private Cloud setup, the cost varies depending on the infrastructure on which it is deployed.
In contrast, Pinecone offers two pricing options, which are further categorized into sub-parts. The first is product pricing, where you can select from starter, standard, and enterprise services. In the product model, the starter service is a free tier, and the cost varies for the other two depending on the features you opt for. The second is support pricing, where you can select from free, developer, pro, and enterprise services.
Unique Differentiators: Qdrant vs Pinecone
Here are some unique differentiator features of both databases:
Qdrant Unique Differentiators
- Open-Source: Quadrant is open-source, enabling you to customize and modify your database as needed.
- Multi Vectors per Point: Qdrant authorizes you to assign multiple vector embeddings to a single data point. This makes it ideal for applications that deal with multimodal data, where data such as text and images are combined for analysis.
- Metadata Size: Quadrant doesn’t have an inherent limit on metadata size. You can attach extra information as you wish, and you can also set a limit for the configuration.
Pinecone Unique Differentiators
- Ease of Integration: Pinecone provides easy-to-use APIs and a developer-friendly interface, making it simpler to integrate with AI platforms and machine learning models.
- Automatic Scaling: Pinecone’s fully managed, serverless solution automatically handles the infrastructure, scaling, and maintenance.
- Separate Storage and Compute: Pinecone's cloud-native paradigm now uses Kafka ingestion and Kubernetes orchestrations. This re-architecture separates read and write paths and dissociates storage and compute resources.
Choosing the Right Vector Database: Qdrant vs Pinecone
You need to correctly analyze the factors mentioned in the above section to make a choice between Qdrant and Pinecone. The choice eventually depends on your specific needs.
Why Choose Qdrant?
If you are looking for a vector database that provides flexible deployment options and customizable features, then Qdrant is the ideal choice for you. It is also suitable for projects that require integration with secure infrastructure.
Why Choose Pinecone?
Pinecone vector database is more suitable when you want a fully managed solution with robust security features. Its low operational overhead cost, automatic scaling, and ease of use make it more popular for building and deploying AI and machine learning applications.
Streamline Data Flow for Vector Databases with Airbyte
Vector databases are widely used in GenAI and machine learning models to train and process complex data. Integrating data in and out of vector databases is challenging, as these systems contain vector embeddings that are far more complicated than normal table data. To enhance your GenAI workflow, it's important to adopt tools and practices that streamline the data integration process.

Airbyte is a data integration tool that simplifies data synchronization between different systems and vector databases like Qdrant and Pinecone. Its intuitive interface enables you to set up a data pipeline within minutes and quickly transfer data from a source to your vector database.
Here’s how you can manage data flows with Airbyte:
- Pre-Built Connectors: Airbyte offers a library consisting of 400+ pre-built connectors. You can use these connectors to build a data pipeline and transfer data from any source into your Qdrant or Pinecone database.
- Automatic Chunking and Indexing: With Airbyte’s automatic chucking and indexing, you can transform your raw data into chunks and then convert them into embeddings using pre-built LLM providers. This feature enhances data storage and retrieval within Qdrant, Pinecone, and other vector databases.
- Multi-Sync Modes: The sync mode feature enables you to select how your data will be read from the source and written in your destination. You can combine different sync modes together for better control.
- Flexibility in Deployment: Airbyte provides deployment flexibility. You can deploy it locally or directly utilize Airbyte Cloud.
Conclusion
The choice of the right vector database depends on the database features and your operational goals. Qdrant offers flexible deployment options and customization features, making it ideal for projects that require a mix of on-premises and cloud environments. Conversely, Pinecone is a fully managed vector database offering features like automatic scaling and robust security features ideal for high-performance applications. Your decision should align with the technical and feasible requirements, ensuring that the chosen database supports the long-term goals.