How to Use Retail Data Analytics to Drive Sales & Revenue?
The global retail analytics market was $7.56 billion in 2023 and is expected to reach $31.08 billion by 2032. This rapid expansion demonstrates the increasing importance of data-driven decision-making in the retail sector. Retailers harness vast amounts of data to understand customer behavior, optimize inventory, and enhance marketing strategies, leading to improved performance.
For instance, major retail giants like Walmart leverage weather data to strategically plan inventory, pricing, and advertising campaigns. They analyze weather forecasts and adjust pricing based on expected weather conditions, like offering discounts on sunscreens when there’s wet weather. This helps manage stocks effectively and increases sales.
This blog explores how retail data analytics can boost sales and outlines the best practices to maximize the impact.
What is Retail Data Analytics?
Retail data analytics is the process of analyzing various data points from retail operations to drive better decision-making. It involves collecting and examining data from multiple sources like point-of-sale systems, customer transactions, and market trends to forecast demand, optimize pricing, and personalize marketing campaigns.
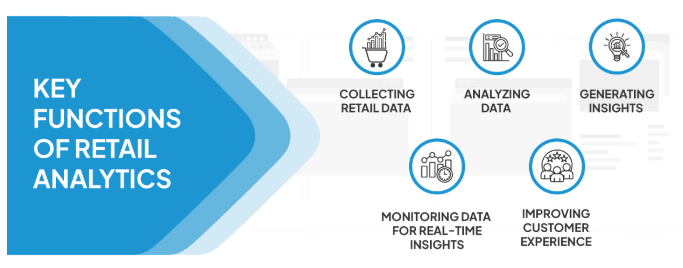
For example, an online retailer could use analytics to suggest items based on past purchases, increasing the likelihood of additional sales. This data-driven approach enhances customer satisfaction and boosts profitability by aligning offerings more closely with consumer requirements.
Benefits Of Data Analytics in the Retail Industry
Here are the key advantages of using data analytics for retail industries:
Streamlined Inventory Management
Data analytics lets you manage your inventory better by forecasting demand and matching stock levels. You can examine historical sales data and seasonal changes to ensure the products are readily available when required. This reduces stockouts and overstock situations, leading to a more agile and responsive supply chain.
Enhanced Customer Experience
By leveraging data analytics, you can gain deeper insights into customers and create personalized shopping experiences for them. For instance, you can analyze purchase history and browsing patterns to recommend products that best fit their interests.
Improved Operational Efficiency
Utilizing data analytics enhances your overall operational efficiency by providing insights into various aspects of your business. You can evaluate supply chain performance, identify bottlenecks, and streamline processes. This helps you allocate resources strategically, which leads to reduced costs and improved service delivery.
Personalized Marketing Strategies
With data analytics, you can refine your marketing efforts by identifying specific customer segments and their unique behaviors. This allows you to craft targeted campaigns that align with the needs of your audience. For example, if you see a particular group of customers who prefer eco-friendly products, you can create targeted campaigns for sustainable products. This drives engagement and increases conversion rates.
Price Optimization
Analyzing factors like competitor pricing, demand sensitivity, and customer buying patterns helps you set prices that maximize your profits. For example, during busy shopping seasons, you can raise prices on products that are in high demand while offering discounts on slower-selling products. This results in increased sales and profit margins.
Types Of Retail Data Analytics
There are four main categories of retail data analytics. Let's understand them in detail:

Descriptive Analytics
Descriptive analytics is the foundational stage of data analysis that provides a clear picture of what has happened in your business. It involves the analysis of historical data to discover patterns and trends. For instance, you might look at sales data from the past year to see which products were top sellers or customer demographics to understand your target market.
Diagnostic Analytics
While descriptive analytics tells you what happened, diagnostic analytics digs deeper to answer the question, Why did it happen? This type of analytics lets you understand patterns, correlations, and root causes behind your business outcomes. For example, if you notice a decline in sales, diagnostic analytics can help you explore potential reasons behind this drop, such as changes in customer preferences, pricing issues, or increased competition. This enables you to address problems proactively and refine your strategies based on data-driven insights.
Predictive Analytics
Predictive analytics empowers you to anticipate future events by identifying patterns in historical and current data. In this approach, techniques like decision theory and optimization algorithms are used to make forecasts and find risks and opportunities. For instance, with predictive analytics, you can observe customers who are more likely to churn and develop strategies to retain them.
Prescriptive Analytics
Prescriptive analytics is an advanced type of analytics that not only predicts outcomes but provides actionable insights to optimize results. Prescriptive models use a combination of complex algorithms and business rules to analyze data and generate recommendations.
For example, you can leverage prescriptive analytics to determine the optimal pricing and inventory levels for specific products based on the predicted seasonal demand fluctuations.
Retail Data You Can Use For Data Analytics
Here are some key categories of retail data that can be utilized for data analytics:
Transaction Data
Transaction data is the detailed records of sales and purchases made by customers. This includes the date and time of the transaction, items purchased, quantities, prices, and payment methods. Analyzing transaction data enables you to identify sales trends and understand customer purchasing behavior.
Customer Data
Customer data is all the information collected about individuals who interact with your retail business. It contains demographic details (age, gender, and location), purchase history, preferences, and feedback from various touch points like online interactions and in-store visits. Effective use of customer data can help you find target segments for promotions and enhance customer loyalty through personalized offers.
Inventory Data
Inventory data gives you insight into stock levels, turnover rates, and product availability. By tracking this, you can ensure popular items are in stock and minimize excess inventory. This prevents stockouts and overstock situations, reduces carrying costs, and optimizes supply chain operations.
Marketing Data
Marketing data includes information about the performance of various marketing campaigns. This data allows you to assess which strategies resonate with your audience. For instance, if a specific social media campaign leads to increased sales among a particular demographic, you can allocate more resources to similar initiatives in the future. This targeted approach maximizes marketing ROI and fosters stronger connections with your customers.
How to Prepare Retail Data For Analytics?
Preparing retail data for analytics involves several critical steps. Let's explore them in detail:
1. Define Your Analytics Goals
The first step is to clearly define your objectives. By setting specific goals, you can determine what data you need to collect and analyze, which will guide the entire data preparation process. For instance, if your objective is to improve marketing strategies, you can focus more on customer purchase history and demographic data.
2. Data Integration
Retail data can come from various channels, including point-of-sale systems, e-commerce platforms, customer relationship management (CRM) systems, and social media. To effectively harness this fragmented data, you should collect and integrate it into a centralized repository for better analysis. Tools like Airbyte simplify this process and help you gain a holistic view of your business operations.
How Airbyte Streamlines Retail Data Integration?
Airbyte is a powerful data movement platform that helps you consolidate retail data from diverse sources into a destination of your choice. With its vast catalog of over 550 pre-built connectors, Airbyte enables you to easily extract data from various retail sources, such as e-commerce platforms and CRM systems like Salesforce and HubSpot. This helps you to gain a comprehensive view of your retail operations for strategic analysis and decision-making.

Key Features of Airbyte:
Flexible Pipeline Development: Airbyte provides various options to build and manage data pipelines. These include an intuitive UI, powerful APIs, a Terraform Provider, and PyAirbyte. You can choose the one that best aligns with your business requirements.
GenAI Workflows: It facilitates integration with popular vector databases, including Qdrant, Milvus, Chroma, Pinecone, and more. This enables you to optimize your AI workflows by loading semi-structured and unstructured data directly to vector store destinations.
Custom Connectors: Airbyte empowers you to build custom connectors within 30 minutes with its easy-to-use Connector Development Kit (CDK). You can also leverage Airbyte’s AI-powered Connector Builder to speed up the development process.
Sync Resilience: Airbyte's Record Change History feature prevents synchronization failures caused by problematic rows like oversized or invalid records. If any record breaks the sync, Airbyte modifies it during transit, logs the changes, and guarantees that the sync completes successfully.
Schema Change Management: With Airbyte, you can automate schema change detection, ensuring your destination reflects updates made in the source, such as new product categories or modified customer fields. This keeps your analytics accurate and up-to-date.
Self-Managed Enterprise: Airbyte provides an Enterprise Edition with advanced features for large-scale organizations. These include role-based access control (RBAC), multitenancy, enterprise source connectors, and personally identifiable information (PII) masking to safeguard sensitive information.
3. Data Processing
Once the relevant data is gathered, the next step is to clean and organize it. This involves removing duplicates, correcting errors, and transforming into a format suitable for analysis. You can also categorize the data by segmenting customer information based on demographics or organizing product data into specific categories for targeted analysis. Proper processing is crucial for eliminating noise in the data that could lead to inaccurate conclusions.
4. Data Enrichment
Data enrichment is the process of adding value to existing datasets with additional information from external sources to provide a more comprehensive view. For example, you can integrate weather data to understand how it impacts sales or add demographic data to better profile your customers. This enriched data facilitates personalized marketing and targeted promotions that resonate more effectively with your users.
5. Data Storage
After processing and enriching your data, you need to store it in a system that supports fast and efficient querying for analytics. Choose a data storage solution based on the scale and complexity of your data. This ensures that your data is accessible and manageable for future analysis.
6. Data Analysis
Once your data is stored and ready, you can analyze it to derive actionable insights. Apply various techniques, such as descriptive analytics and predictive modeling, to understand trends, forecast sales, and tailor marketing strategies accordingly.
Using Retail Data Analytics to Drive Revenue
Below are key areas where retail data analytics can significantly drive revenue.
Market Basket Analysis
Market basket analysis involves examining the purchasing behavior of customers to identify patterns in the items they buy together. By understanding these associations, you can optimize product placement, enhance cross-selling strategies, and create targeted promotions. For example, if customers buy chips with soda, you can put them together or offer a bundle discount to increase sales.
Churn Prediction Analysis
Churn analysis is about finding customers who are likely to stop buying. By looking at customer behavior, transaction history, and feedback, you can pinpoint the factors that cause churn. Then, you can implement proactive retention strategies like offers or loyalty programs to reduce customer churn and maintain long-term profitability.
Demand Forecasting
Demand forecasting uses historical sales data and market trends to predict future product demand. This analysis helps you manage inventory levels better, reducing the risk of overstocking or stockouts. Accurate demand forecasting ensures popular products are available when needed, which drives customer satisfaction and sales. You can also adjust your supply chain operations based on these forecasts to optimize costs.
Customer Sentiment Analysis
By analyzing customer feedback from social media, reviews, and surveys, you can assess overall sentiment—positive, negative, or neutral. This analysis yields valuable insights into customer preferences and pain points that empower you to make strategic decisions. For example, if sentiment analysis reveals negative feedback about a product feature, you can address these concerns through improvements or targeted communication.
Retail Data Analytics Best Practices to Follow
Here are some best practices that will help you leverage retail data analytics effectively:
Have a Clear Data Collection Methods
Establishing clear data collection methods is essential for accurate analytics. Define what data you need and how you will gather it, whether through point-of-sale systems, online transactions, or customer surveys. This ensures all relevant data is captured and you have a holistic view of your customers and business operations.
Clean and Validate the Data
Data cleaning and validation is a critical step in the analytics process, as the raw data often contains duplicates or invalid entries. You must ensure that the data you collect is accurate, complete, and consistent. Implement rigorous data validation checks to spot and rectify errors before analysis. This prevents flawed insights that could misguide strategic decisions.
Make Use of Historical Data
Leverage historical data to identify trends, seasonal patterns, and customer preferences. This information helps you anticipate future demands and optimize inventory or marketing strategies. For example, if you see sales of certain items rise during the holiday season, you can adjust your inventory accordingly so you have enough stock.
Utilize Data Visualization Tools
Data visualization tools aid you in making complex datasets understandable and actionable through dashboards, charts, and graphs. This facilitates you to discover trends, outliers, and opportunities. Effective visualization also enhances communication across teams, fostering a data-driven culture within your organization.
Monitor Key Performance Indicators (KPIs)
Tracking KPIs is key to measuring the success of your retail strategies. Discover the right metrics that align with your business goals, like customer acquisition cost (CAC), sales growth, and customer lifetime value (CLV). Review these regularly to gauge performance and make adjustments for better results.
Conclusion
This article discusses the significant benefits of retail data analytics, such as enhanced customer insights, optimized inventory management, and targeted marketing strategies that can significantly boost revenue. Furthermore, you've explored best practices to implement it effectively. By implementing retail data analytics, you can craft personalized shopping experiences that resonate with customers, resulting in sustained growth and profitability.