
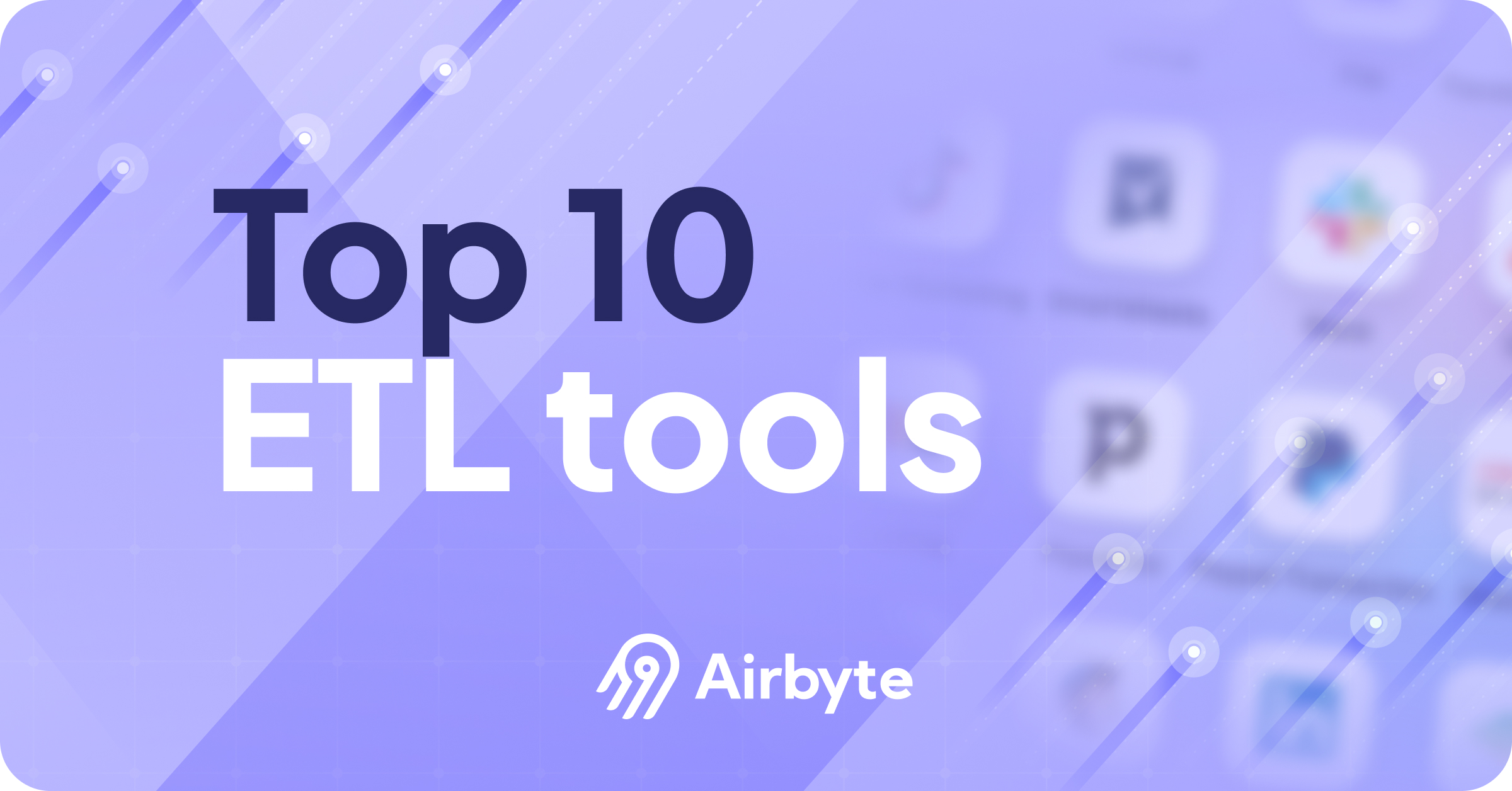
The data around us can be structured like a contact list or unstructured like audio and video files or emails. This data might look unrelated at first glance, but you can gain insights from it when collected and processed properly, which is why data discovery tools are essential. These tools allow you to explore, analyze, and visualize your data to discover hidden patterns and meaningful insights.
This article will help you understand about the data discovery process, its benefits, and the top 5 data discovery tools available today.
What is a Data Discovery Tool?
A data discovery tool is software that enables you to quickly search, access, visualize, and analyze data from different sources to uncover actionable insights. It provides an intuitive interface that allows you to explore data without extensive technical expertise interactively or relying on IT teams. The data discovery tool supports connecting to structured, unstructured, and semi-structured data sources, including cloud applications, databases, data warehouses, flat files, etc.
Benefits of Data Discovery Tool
The benefits of data discovery tools are as follows:
- Self-Service BI: It promotes a self-service business intelligence approach, allowing you to perform data analysis independently without extensive training or technical skills. You can create reports, dashboards, and visualization tailored to your needs.
- Interactive Exploration: The data discovery tool allows you to explore large datasets and visually navigate the data to identify patterns, trends, and outliers. This dynamic exploration enhances the understanding of complex data structures.
- Visual Data Representation: It facilitates the creation of visually compelling charts, graphs, and dashboards. Visualizing complex data insights makes it easier to understand data from disparate sources.
- Data Integration: Many of the data discovery tools often support integrating data from various sources, providing a unified view of the information. This integration allows for a comprehensive analysis of diverse datasets.
Top 10 Data Discovery Tools
Tableau
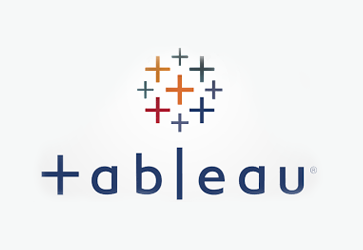
Tableau is a powerful and widely used data visualization and BI platform. It enables you to gain meaningful insights through interactive or shareable dashboards, reports, and charts. The tool has gained immense popularity for its user-friendly interface, robust features, and versatility in handling various data types like numeric, geographic, and many more.
Key capabilities of Tableau include:
- Ad-Hoc Data Exploration: You can explore and analyze real-time data with Tableau without predefined queries. Instant visual feedback can quickly iterate and drill down into specific data points to uncover patterns, trends, and outliers.
- Interactive Dashboards: Tableau’s interactive dashboards allow you to create dynamic and responsive visualizations. You can interact with the data, apply filters, and customize views, providing a more engaging and personalized experience.
- Real-Time Data Analytics: Tableau supports real-time analytics, allowing you to create dashboards that update dynamically as new data becomes available. This capability is crucial for monitoring live data streams and making informed real-time decisions.
- Cross-Database Joins and Data Blending: It enables you to perform cross-database joins, allowing data integration from different sources. Data blending functionality further extends this capability, providing a comprehensive view by combining data from multiple datasets.
Microsoft Power BI

Power BI is a business analytics service provided by Microsoft. It allows you to connect to various data sources to transform data. It is known for its seamless integration with the Microsoft ecosystem and offers a wide range of visualization options for data analysis. Additionally, Power BI offers robust data modeling capabilities and supports collaboration features for sharing insights.
Features of Power BI include:
- Power Query and Power Pivot: Power BI incorporates Power Query for data connectivity and transformation, whereas Power Pivot is for data modeling. These tools enhance data shaping and analysis capabilities, giving you more control over your data.
- Row-Level Security: It incorporates robust security features, such as row-level security, allowing access to data based on user roles. This allows you to assign roles and responsibilities, ensuring that sensitive data is accessed only by authorized users.
- Integration with Microsoft Ecosystem: Power BI integrates effortlessly with other Microsoft tools and services, such as Excel, Azure, and SQL Server. Integrating with different services will help you streamline accessing and analyzing data stored on these platforms.
Looker
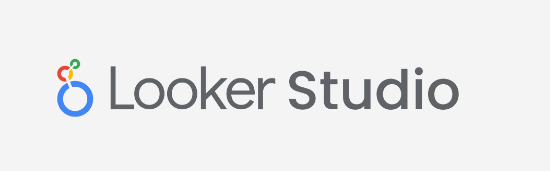
Looker is a business intelligence and data analytics platform that allows you to explore, analyze, and visualize your data. It is designed to help you make informed decisions based on your data by providing a user-friendly interface and powerful data exploration capabilities. Looker uses LookML (Looker Modeling Language) for data modeling. LookML is a powerful and flexible modeling language that allows you to define data structure and relationships in a centralized and reusable way.
Here are some must-known features of Looker:
- Explore in-Database: It promotes the concept of Explore in-Database, where you can closely analyze the data source, minimizing the movement and improving performance. This approach is advantageous for large datasets and distributed data environments.
- Embedded Analytics: Looker offers embedded analytics solutions, allowing you to directly integrate data visualizations and insights into your existing applications or websites. This is particularly valuable if your business wants to provide analytics as a service to its customers.
- Adaptability to Changing Data Sources: When data sources change or evolve in dynamic business environments, LookML will provide a flexible and adaptable solution. With the LookML model, you can update the modified data structures to reflect these changes, maintaining continuity in data analysis.
IBM Cognos Analytics

IBM Cognos Analytics is a business intelligence and analytics platform. It is designed to help you make data-driven decisions by providing capabilities for dashboarding, reporting, data discovery, and advanced analytics. The platform includes advanced analytics capabilities such as predictive modeling, forecasting, and what-if analysis. You can apply statistical techniques to identify patterns and make predictions based on historical data. This is particularly valuable for looking beyond retrospective analysis and making proactive decisions based on predictive models.
Some common features of IBM Cognos Analytics include:
- OLAP (Online Analytical Processing): The IBM Cognos Analytics platform supports OLAP functionality, facilitating you to explore and analyze data in a multidimensional method. OLAP enables you to gain a deeper understanding of data relationships and hierarchies, which enhances analytical capabilities.
- Ad Hoc Query: You can perform ad hoc queries within the platform, exploring data interactively to obtain the necessary information. The intuitive interface also allows you to create ad hoc queries by dragging and dropping elements without extensive technical skills.
- Key Performance Indicators (KPIs): You can define and track KPIs within reports and dashboards. Cognos Analytics allows for the visualization of KPIs, making it easy to analyze performance metrics.
Spotfire
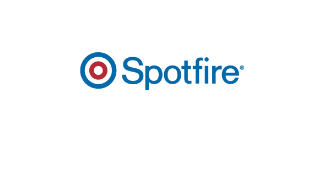
TIBCO Spotfire is a data analytics platform that enables you to merge data in a single analysis and get a holistic view with interactive visualization. Its AI-driven analytics and interactive data maps make exploring, analyzing, and interpreting complex datasets easier.
Here are the key aspects of Spotfire:
- Advanced Analytics: Spotfire integrates advanced analytics capabilities, facilitating the application of statistical and predictive analytics to your data. This includes features for regression analysis, clustering, and other advanced modeling techniques.
- Geospatial Analytics: The platform includes geospatial analytics capabilities, enabling you to visualize and analyze the data points on maps. This is particularly useful for understanding spatial patterns and relationships within the data.
- Integration with R and Python: Spotfire supports integration with popular statistical and programming languages such as R and Python. It allows you to leverage custom scripts and algorithms for advanced analytics within the Spotfire environment.
- Real-time Data Streaming: It supports real-time data streaming, allowing you to analyze and visualize data as it is generated. This is particularly beneficial for monitoring data and making immediate decisions.
Qlik Sense

This robust analytics and data visualization tool enables users to build interactive dashboards and reports. Its self-service features let users make data-driven decisions more quickly, and its associative data model facilitates intuitive data exploration.
Domo
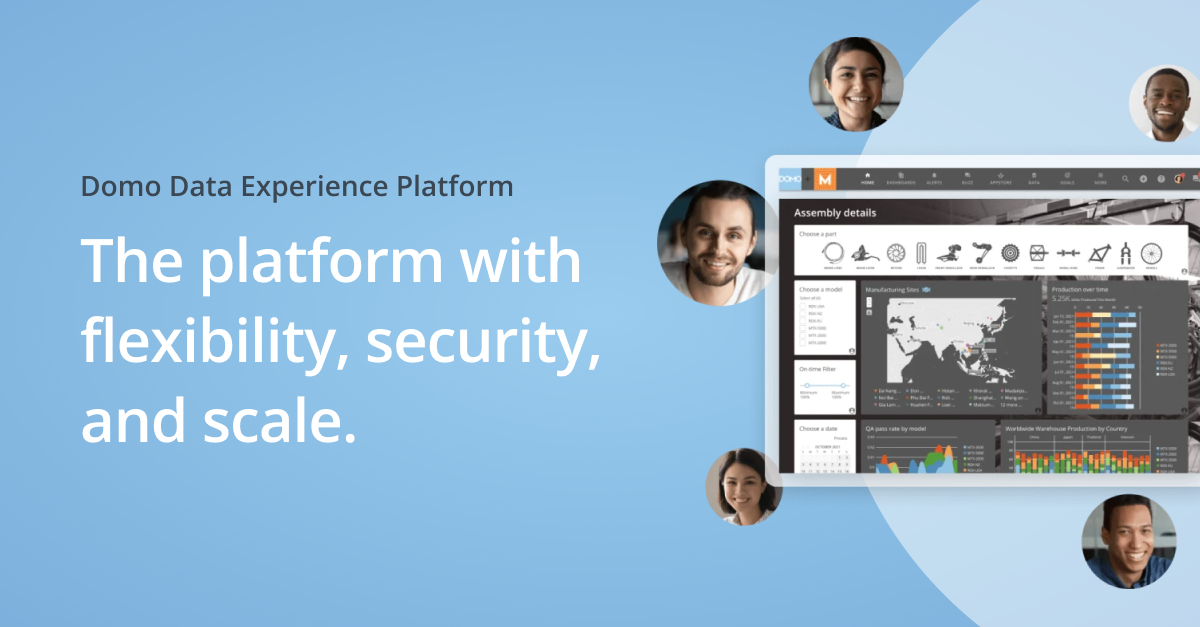
Domo is a cloud-based platform that combines information in real-time from multiple sources. With the help of its collaborative tools, predictive analytics, and configurable dashboards, businesses can increase productivity and foster expansion.
TIBCO Spotfire

This platform for data analytics and visualization is renowned for its interactive visualizations and sophisticated analytics features. It helps users swiftly extract insights from large, complex data sets, giving businesses an advantage over competitors and enabling them to make wise decisions.
SAP Lumira

This self-service data visualization tool enables users to create interactive visualizations and stories from raw data. Lumira's user-friendly interface and sophisticated analytics functionalities facilitate data exploration, trend identification, and insight sharing among team members.
Datapine

Datapine is an excellent business intelligence tool for non-technical users. With its user-friendly drag-and-drop interface, customizable dashboards, and AI-powered insights, data analysis is now accessible to all. With data-driven insights, Datapine assists companies in streamlining decision-making procedures and enhancing data discovery.
Requirements for the best data discovery tool
Ease of Use
The tool should be simple to use and require little technical knowledge to operate. Usability can be improved with simple features like drag-and-drop functionality and pre-made templates.
Data Connectivity
In order to allow users to analyze data from multiple sources in one location, it should support connectivity to a variety of data sources, such as databases, cloud storage, and third-party apps.
Data Preparation
To guarantee data quality and consistency, the data discovery tool should have the ability to integrate, transform, and cleanse data. Features for automated data preparation can make this process more efficient.
Visualization Options
To effectively communicate insights and patterns within the data, it should offer a wide range of visualization options, such as charts, graphs, maps, and dashboards.
Advanced Analytics
To find patterns, correlations, and predictive insights in the data, the data discovery tool should support advanced analytics methods like statistical analysis, machine learning, and predictive modeling.
Security and Governance
The application must comply with industry norms for data security and governance, which include role-based access control, data encryption, and legal compliance with HIPAA and GDPR.
An Efficient Solution for Advanced Analytics - Airbyte
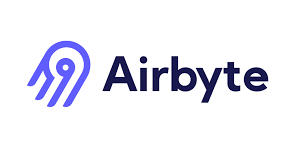
The data discovery tools mentioned above will enable you to explore and analyze data, uncovering valuable insights and trends. However, if your data is located at different locations or stored in disparate systems, you’ll need an integrated platform to effectively manage and access it. This is where Airbyte comes into play.
Airbyte provides a centralized platform for connecting to various data sources. With the help of its 550+ pre-built connectors, you can seamlessly automate the process of collecting, preparing, and moving data to the desired systems without writing a single line of code. This allows you to efficiently streamline the data integration process, ensuring data is easily available and accessible for analysis using data discovery tools.
By leveraging Airbyte alongside data discovery tools, you can optimize your data workflows, uncover deeper insights, and make more informed decisions to drive business success.
Some compelling features of Airbyte include:
- Data Pipeline Flexibility: Airbyte offers versatile options to build and manage data pipelines. You can utilize user-friendly UI, API, Terraform, and PyAirbyte. This flexibility enables you to tailor your data integration processes to meet specific needs and operational requirements.
- GenAI Workflows: You can load unstructured data directly into popular vector databases such as Pinecone, Qdrant, Weaviate, and Milvus. This integration streamlines the process of preparing data for LLMs, enabling efficient context-based retrieval and enhancing the relevance of generated outputs.
- Connector Data Capture (CDC): Airbyte supports CDC, allowing you to capture and replicate only the changes made to the data since the last synchronization. It minimizes the amount of data transferred during updates, enhancing efficiency and reducing processing overhead in data integration flow.
- Schema Change Management: Airbyte automatically manages schema changes by reflecting any modifications made at the source in the destination based on your configured preferences. This functionality minimizes errors and enhances sync resilience, ensuring that your data pipelines remain accurate and up-to-date.
- Connector Development Kit (CDK): The CDK feature provided by Airbyte simplifies the process of creating custom connectors. It streamlines the development of connectors by offering pre-built templates, documentation, and guidelines, enabling you to build your customized connector.
- AI Assistant: Airbyte has recently introduced AI Assistant to simplify the creation of connectors. This tool scans the API documentation you provided and automatically fills in the configuration fields in the Connector Builder, which significantly reduces the development time.
- Uninterrupted Data Syncs: Airbyte employs a checkpointing mechanism to ensure successful data synchronization. This feature enables the platform to resume incremental syncs from the last successful point, minimizing data loss in case of a failure.
- Robust Enterprise Support: The Self-Managed Enterprise edition of Airbyte offers a comprehensive solution for managing and securing large-scale data. It provides additional features like multitenancy, role-based access, PII masking, and dedicated enterprise support with service level agreements (SLAs).
Conclusion
With a user-friendly interface and advanced analytics, these tools empower you to extract valuable insights. By selecting a data discovery tool tailored to your specific requirements, you can quickly uncover hidden patterns, trends, and correlations within your datasets.
FAQs
1. What is the best open-source data discovery tool?
Microsoft Power BI is the best open-source data discovery tool. It has robust data modeling capabilities with strong security features too. Other than that, it is also easily integrated with all other tools of Microsoft helping in streamlining the whole process.
2. What is the difference between a data discovery tool and a data classification tool?
Finding patterns and insights in data is the main goal of a data discovery tool, which gives users the ability to examine, evaluate, and visualize data in order to get important insights. A data classification tool, on the other hand, groups data according to predetermined standards or guidelines, making it easier to organize, manage, and comply with legal requirements for data handling and security.
3. What is the difference between data discovery and data catalog?
Data discovery involves identifying and understanding various data sources, whereas a data catalog is a repository that organizes data assets along with their metadata. Data discovery delivers a real-time, domain-specific insight into data usage and health, while data catalogs provide a more static, centralized inventory of data assets.
What should you do next?
Hope you enjoyed the reading. Here are the 3 ways we can help you in your data journey:
Frequently Asked Questions
What is ETL?
ETL, an acronym for Extract, Transform, Load, is a vital data integration process. It involves extracting data from diverse sources, transforming it into a usable format, and loading it into a database, data warehouse or data lake. This process enables meaningful data analysis, enhancing business intelligence.
This can be done by building a data pipeline manually, usually a Python script (you can leverage a tool as Apache Airflow for this). This process can take more than a full week of development. Or it can be done in minutes on Airbyte in three easy steps: set it up as a source, choose a destination among 50 available off the shelf, and define which data you want to transfer and how frequently.
The most prominent ETL tools to extract data include: Airbyte, Fivetran, StitchData, Matillion, and Talend Data Integration. These ETL and ELT tools help in extracting data from various sources (APIs, databases, and more), transforming it efficiently, and loading it into a database, data warehouse or data lake, enhancing data management capabilities.
What is ELT?
ELT, standing for Extract, Load, Transform, is a modern take on the traditional ETL data integration process. In ELT, data is first extracted from various sources, loaded directly into a data warehouse, and then transformed. This approach enhances data processing speed, analytical flexibility and autonomy.
Difference between ETL and ELT?
ETL and ELT are critical data integration strategies with key differences. ETL (Extract, Transform, Load) transforms data before loading, ideal for structured data. In contrast, ELT (Extract, Load, Transform) loads data before transformation, perfect for processing large, diverse data sets in modern data warehouses. ELT is becoming the new standard as it offers a lot more flexibility and autonomy to data analysts.