
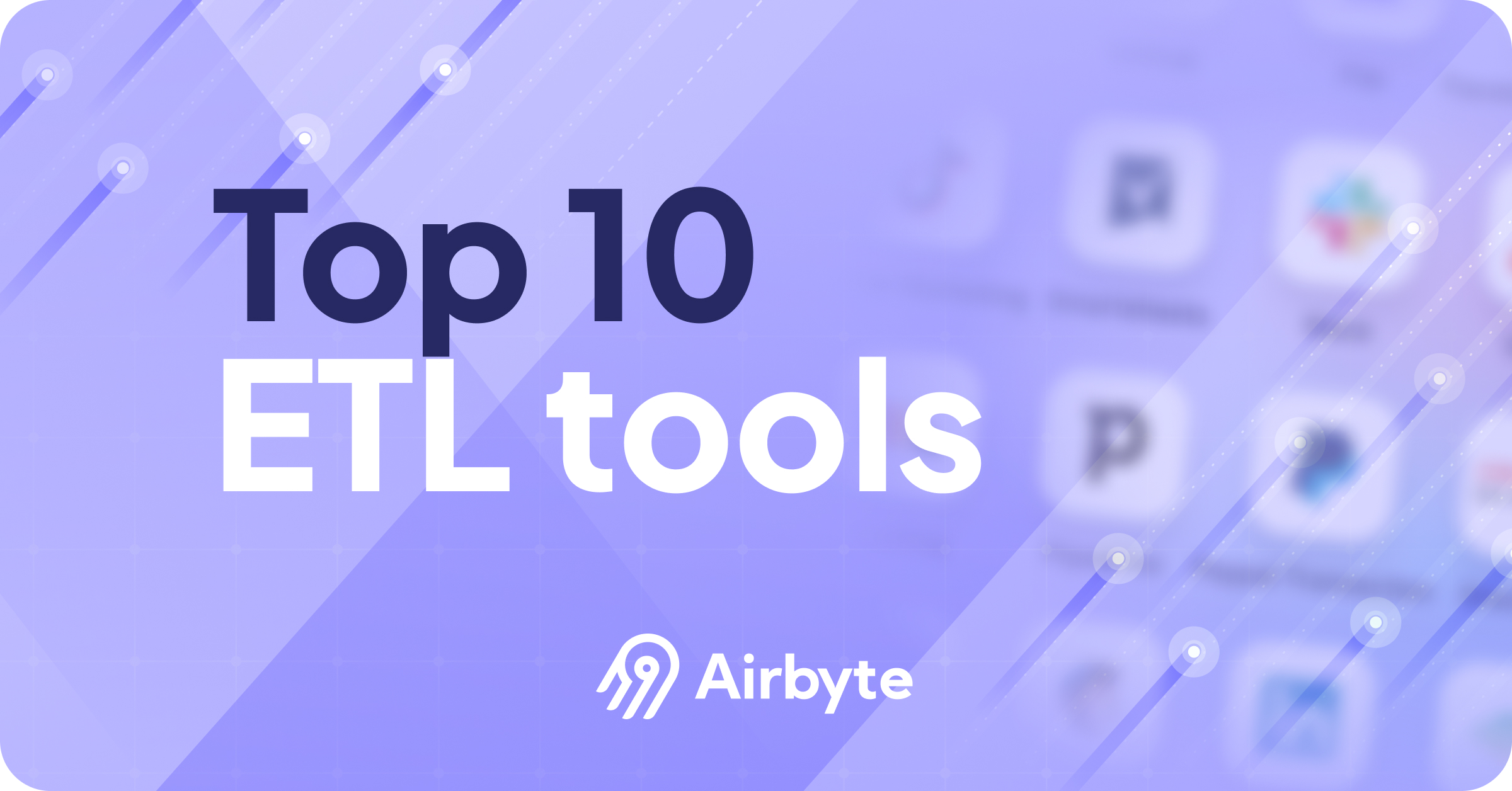
In the world of cloud data warehouses, Snowflake occupies one of the top-ranking positions. While the platform is known for its swift query processing and strict data security measures, it is difficult to extract insights from large datasets. To comprehensively analyze your data, you can integrate your Snowflake datasets with a reporting tool. With such tools, you can comprehend complex data easily. This article will introduce you to the top three Snowflake reporting tools in detail.
Snowflake Overview
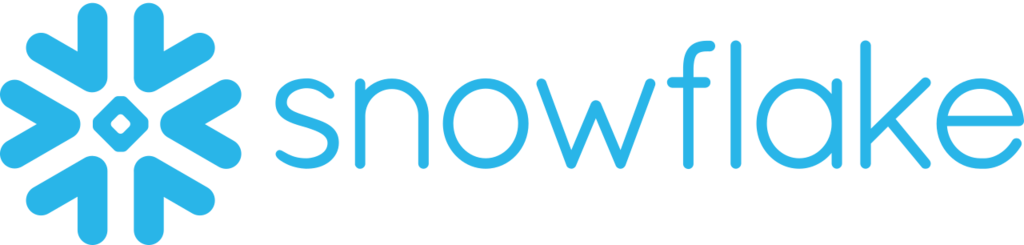
Snowflake is an advanced data platform that provides separate data storage and computation facilities. It offers efficient data processing and analytics solutions that surpass traditional offerings in terms of speed, efficiency, and flexibility. Snowflake is built entirely on cloud infrastructure, and its components operate within public cloud environments.
Snowflake accounts can be deployed on three cloud platforms: AWS, Google Cloud Platform (GCP), and Microsoft Azure. Each platform hosts one or more regions where Snowflake accounts can be provisioned, ensuring scalability and accessibility across diverse cloud environments.
Snowflake’s unique architecture enables quick query processing. There are virtual compute instances that handle computational tasks, while a dedicated storage layer stores your databases. Snowflake leverages massively parallel processing (MPP) compute clusters to swiftly execute your queries.
Why should you Move Snowflake Data into Reporting Tools?
Reporting tools are business intelligence applications that help you present and visualize data in an easily understandable format. These tools offer features such as generating tables, graphs, charts, and other visualizations, enabling you to analyze large Snowflake databases quickly.
Graphical depiction of data is easier to consume and disseminate. Through visualizing, you can identify patterns and trends within the data. It helps you understand the impact of previous strategies, allowing you to make informed decisions quickly.
Automation plays a critical role in reporting tools, streamlining processes that would otherwise be manual. You can generate and share reports in just a few minutes, allowing your team to focus on more strategic tasks. It is especially useful for team members who do not have much technical expertise and find it difficult to analyze data in Snowflake.
Top 3 Snowflake Reporting Tools
Once you securely migrate your dataset into Snowflake, you need to invest in a good BI reporting tool. It is an important transition as the tool helps you coherently visualize the data. You can present the data in visually appealing charts, graphs, and reports to different stakeholders. Take a look at the best Snowflake reporting tools that you can use:
Power BI
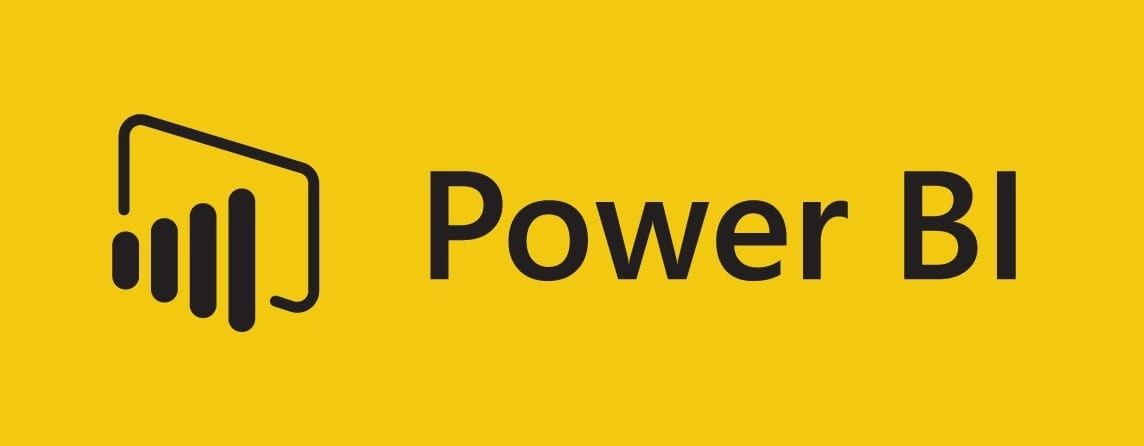
Power BI is one of the leading business intelligence tools that offers you services for data analysis, visualization, and reporting. Its flexibility and extensibility empower you to integrate analytics seamlessly into your workflows and applications and derive useful insights from your datasets.
Power BI encompasses several interconnected elements, including Power BI Desktop, Power BI service, and Power BI Mobile app for Windows, iOS, and Android devices. Collectively, these components enable you to create, share, and consume business insights for different needs and scenarios.
Key Features:
- Report Builder: The tool allows you to create paginated reports, which are formatted to fit well on a page. Paginated reports are often used for operational reports or printing forms such as invoices or transcripts. You can share these reports through the Power BI service.
- APIs: You can leverage Power BI APIs to interact with the platform’s elements programmatically. This includes pushing data into semantic models, embedding dashboards and reports into custom applications, and creating custom visualizations.
Connecting to Snowflake: In Power BI, the connector for Snowflake is slightly different from other connectors. It is primarily due to Snowflake’s capability for Microsoft Enterprise ID, which offers Single Sign-On (SSO) functionality. You can seamlessly authenticate and access data in Snowflake at any time through your Microsoft Enterprise credentials.
Pricing: Although there is a Free plan to get started, Power BI has two paid plans. The Power BI Pro begins at $10 per month for a user, and the Power BI Premium plan is priced at $20 per month per user. There are three monthly plans under Microsoft Fabric, depending on the SKUs you use.
Tableau
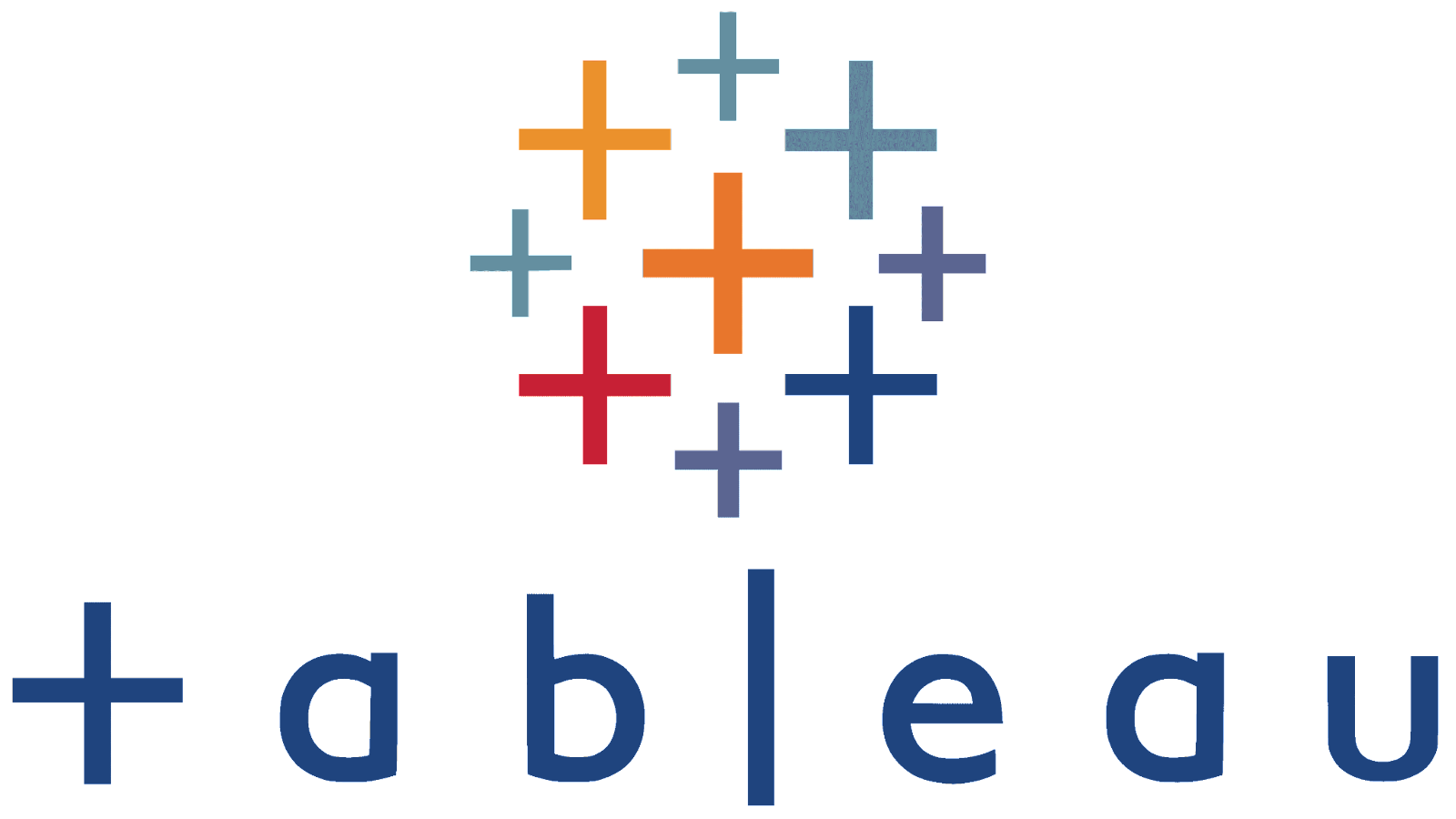
Founded in 2003 and acquired by Salesforce in 2019, Tableau is a well-known visual analytics platform and business intelligence tool. The reporting tool offers various features and functionalities to enhance data analytics and visualization, helping you derive the maximum potential from your datasets.
Key Features:
- Tableau Catalog: This feature automatically catalogs all your data assets and sources into a centralized list, providing metadata in context for efficient data discovery. Tableau Catalog helps you understand the data lineage and integrates data dictionaries, impact analysis, and data quality warnings into other applications of the platform.
- Geospatial Visualization: Through geospatial visualization, you can showcase data in relation to its physical location. You can plot data on maps to gain granular insights into your enterprise’s data across multiple locations. To conduct further analysis, you may also use the geo hierarchies feature. Additionally, Tableau enables the creation of custom territories via Tableau groups, providing a comprehensive view of the entire data.
Connecting with Snowflake: Navigate to the Connect menu of Tableau and select Snowflake from the list of connectors. Choose from one of the authentication methods: Username and Password, OAuth, or Okta Username and Password.
For Okta, remember to enter the URL for the Okta server. You can use Single Sign-On (SSO) for OAuth if Snowflake supports it.
Pricing: Tableau has three pricing plans: Creator, Explorer, and Viewer. If you want to deploy any of them or set up an Enterprise account, you need to contact their sales team.
Qlik Sense

Qlik Sense is a comprehensive data analytics solution designed to address complex data challenges effectively. You can combine, load, and visualize huge volumes of data with its easy drag-and-drop interface. This Snowflake reporting tool has intuitive features for searching, selecting, drilling down, and zooming out datasets to derive insights.
Key Features:
- Qlik AutoML: Qlik AutoML is one of the notable features that helps you create machine learning models and predictions with full explanations in a user-friendly, code-free environment. Qlik Sense allows you to interactively explore advanced analytics from both Qlik AutoML and leading data science platforms and analytics apps. You can also conduct real-time calculations and receive instant answers.
- Natural Language Processing (NLP) Capabilities: Qlik Sense simplifies interaction with data through its robust NLP capabilities. The platform’s Insight Advisor leverages NLP to auto-generate relevant and impactful analyses and insights based on your queries. Furthermore, conversational analytics in Qlik Sense help enhance overall user experience and decision-making.
Connecting with Snowflake: To establish a connection to a Snowflake database in Qlik Sense, you must have the server, database name, and access credentials. Once your Snowflake connection is established, you can select data from different tables and load it into the reporting tool. Qlik Sense offers different authentication methods, including Username and Password (default setting), OAuth (available in Qlik Sense SaaS), and key pairing. You can further authenticate your connection through the Add data dialog or Data load editor.
Pricing: Qlik Sense offers you a free trial of 30 days. It starts billing at $20 per month for a single user. You can contact their sales team to learn more about pricing plans for the enterprise-level setup.
How to Improve the Performance of Your Snowflake Reporting Tools?
The business intelligence tools you utilize for Snowflake reporting have a multitude of features. However, before you analyze the data in the reporting tool, it is important to ensure that your datasets in Snowflake are well-consolidated and up-to-date. To do so, you can turn to data integration and replication platforms like Airbyte.
Airbyte allows you to extract and ingest data from multiple sources. This feature is handy when you have large datasets spread across several locations and database storage systems. Once you have configured the source, you can find the dedicated Snowflake connector from Airbyte’s extensive 350+ pre-built connectors library.
In case you cannot find a desired connector for configuring your data source, you can always create a custom one. Airbyte provides you with a Connector Development Kit (CDK) that guides you on how to build a custom connector in under 30 minutes. Thus, you can expedite the data consolidation process to migrate your data.
Creating a data pipeline with Snowflake as your destination does not require you to write a single line of code. The connection can be established in a few minutes. You can be assured of data security and integrity with Airbyte, as the platform cannot store or view data in the pipeline.
Another notable feature of Airbyte is its Change Data Capture (CDC) capabilities. If your datasets are modified at source, you can set up a sync cycle to capture and integrate your data changes. Once your Snowflake data is loaded and refreshed, you can transform it using various features of the platform. Hence, when all your datasets stay updated and secured in Snowflake, you can perform more accurate data analysis and create better dashboards on the reporting tool.
The Final Word
Migrating your dataset to Snowflake is not enough; you must adopt a robust reporting tool that helps you visualize your data. With these tools, you save time, effort, and resources in creating and presenting reports to different team members. To take the automation process a notch higher, you can establish a data pipeline with Airbyte. It will ensure all your datasets are securely loaded into Snowflake, as your reporting tool may not have several different connectors for sourcing the data. Sign up for free on Airbyte to get started!
What should you do next?
Hope you enjoyed the reading. Here are the 3 ways we can help you in your data journey:
Frequently Asked Questions
What is ETL?
ETL, an acronym for Extract, Transform, Load, is a vital data integration process. It involves extracting data from diverse sources, transforming it into a usable format, and loading it into a database, data warehouse or data lake. This process enables meaningful data analysis, enhancing business intelligence.
This can be done by building a data pipeline manually, usually a Python script (you can leverage a tool as Apache Airflow for this). This process can take more than a full week of development. Or it can be done in minutes on Airbyte in three easy steps: set it up as a source, choose a destination among 50 available off the shelf, and define which data you want to transfer and how frequently.
The most prominent ETL tools to extract data include: Airbyte, Fivetran, StitchData, Matillion, and Talend Data Integration. These ETL and ELT tools help in extracting data from various sources (APIs, databases, and more), transforming it efficiently, and loading it into a database, data warehouse or data lake, enhancing data management capabilities.
What is ELT?
ELT, standing for Extract, Load, Transform, is a modern take on the traditional ETL data integration process. In ELT, data is first extracted from various sources, loaded directly into a data warehouse, and then transformed. This approach enhances data processing speed, analytical flexibility and autonomy.
Difference between ETL and ELT?
ETL and ELT are critical data integration strategies with key differences. ETL (Extract, Transform, Load) transforms data before loading, ideal for structured data. In contrast, ELT (Extract, Load, Transform) loads data before transformation, perfect for processing large, diverse data sets in modern data warehouses. ELT is becoming the new standard as it offers a lot more flexibility and autonomy to data analysts.