ETL processes have been reliable but face challenges as data environments evolve. Hardcoded pipelines often break with schema changes, and batch processing misses real-time insights. AI ETL tools address these issues by adapting to schema drifts, spotting anomalies, and suggesting data transformations automatically, ensuring fewer sync failures and faster insights.
AI ETL tools transform business operations by efficiently managing data flows vital for competitiveness. They feature pre-built connectors and user-friendly interfaces, making data management more accessible. With increasing data volumes, integrating AI into your data stack is crucial for maintaining data quality, integrity, and security.
Incorporating AI into ETL processes enhances data workflows, allowing seamless integration from diverse sources. These tools empower business users to maintain data pipelines without relying heavily on developers, enabling data teams to extract valuable insights from massive datasets while ensuring data accuracy and protection.
What Are AI ETL Tools?
AI ETL tools are advanced data integration platforms leveraging AI and machine learning to enhance ETL (Extract, Transform, Load) processes. Unlike traditional ETL tools, which depend on static logic and manual intervention, AI ETL tools continuously adapt to changes in data environments. They simplify pipeline maintenance and dynamically adjust data workflows to align with evolving business needs.
These tools automatically infer schemas, detect anomalies, optimize job execution, and recommend data transformations. They empower users to efficiently extract raw data from multiple data sources, transform it into a usable format, and load it into a target system. AI ETL tools also learn from errors or performance bottlenecks to fine-tune future runs. Data engineers play a crucial role in performing ETL development, managing data pipelines, and ensuring effective communication with AI services.
Designed to be user-friendly for business users, AI ETL tools allow management of data workflows without heavy reliance on developers, making complex data integration tasks accessible. They emphasize extracting data from diverse sources, vital for streamlining data integration processes and ensuring robust data governance.
For enterprise teams handling high-volume, fast-changing data, AI ETL tools reduce repetitive tasks, minimize failures, and ensure data is delivered with accuracy and integrity. Whether syncing structured warehouse data or integrating third-party APIs, AI ETL tools enhance data accuracy and integrity.
Why Traditional ETL Falls Short for Modern Data Teams
Legacy ETL tools were designed for a bygone era. In the past, data sources were limited, pipelines were mostly static, and updates occurred on predictable schedules. Today's data landscape is dynamic, with data being messy, real-time, and constantly evolving. Traditional tools struggle to meet the demands of modern data processing and the complexities involved in maintaining robust data pipelines. These tools often fall short in automating the ETL process and adapting to contemporary data environments.
Hardcoded logic becomes fragile when schemas change. Manual mapping introduces bottlenecks. Often, failures go unnoticed until a dashboard goes blank or an executive questions missing metrics.
Efficient loading processes are a crucial component of comprehensive solutions that address data extraction, transformation, and integration tasks. These processes are vital for ensuring data protection and maintaining data integrity across the entire workflow.
For data engineers, this means dealing with reactive workflows and endless patching. For IT managers, it raises concerns about compliance and auditability. Data governance is essential for ensuring compliance and robust monitoring capabilities, which traditional tools often lack. For BI teams, it delays the insights they need to make informed decisions.
Modern data teams require tools that can adapt. AI ETL tools go beyond task automation. They anticipate changes, monitor performance, and reduce failure points—strengthening pipelines, not just speeding them up. These advanced data integration tools enhance the ability to extract data efficiently, ensuring that data accuracy and protection are prioritized in every step of the ETL process.
What to Look for in AI ETL Tools
Understanding Your Team's Needs
Selecting the right AI ETL tool starts with a deep understanding of your team’s workflow and the specific demands of your data stack. This strategic decision is crucial for enhancing your organization’s data capabilities and ensuring adaptability for future growth. It’s not just about listing features; it’s about choosing those that effectively streamline data pipelines and enhance data integration processes.
Key Features and Adaptability in Data Pipelines
An ideal AI ETL tool should automatically detect schema changes and adjust without requiring manual intervention, ensuring the smooth operation of complex data pipelines. Understanding architectural patterns in ETL pipeline implementation can enhance efficiency and scalability. This allows non-developers to manage ETL processes and delineate individual services, fostering better agility and fault isolation within entire data workflows.
Transformation intelligence is vital; many platforms use machine learning to suggest or automate data cleaning, enrichment, and mapping, thus reducing repetitive tasks and enhancing data processing efficiency.
Observability and Data Security
Observability is a critical feature. A robust AI ETL tool should alert you to potential issues before they disrupt dashboards or models, offering context to resolve problems quickly. Data security is paramount, with features like GDPR and HIPAA compliance, and field-level encryption to protect sensitive data throughout the data processing lifecycle.
Governance and Flexibility in Data Integration
For IT teams, governance features such as access controls, encryption, and audit logs are essential. Engineers require flexibility and API support to manage complex data pipelines, while BI teams need tools that provide clean data quickly. Monitoring workflows is another crucial feature, enabling users to manage and visualize complex data integrations and dependencies effectively.
Making Smart Choices for Data Processing
Ultimately, smart pipelines are the result of smart choices. By selecting an AI ETL tool that aligns with your team’s specific needs and data workflows, you lay the foundation for efficient, reliable, and secure data integration and processing.
Top AI ETL Tools for Modern Data Teams
1. Airbyte
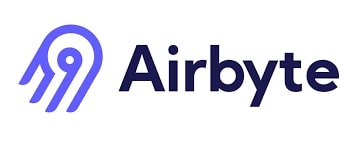
Airbyte combines open-source flexibility with AI-driven automation, efficiently managing data movement between multiple systems and destinations. It supports 400+ data connectors, auto-generates new ones, and adapts to schema changes without breaking pipelines. With support for dbt transformations and both cloud and self-managed deployment, it’s a strong choice for teams seeking scalable, intelligent ETL workflows with full control.
Key features:
- AI-powered schema detection and adaptation
- Extensive library of pre-built and custom data connectors
- Open-source and cloud deployment options
- Seamless integration with dbt for complex data transformations
2. Fivetran
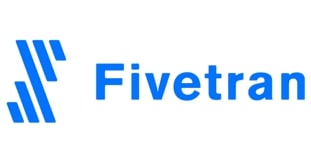
Fivetran optimizes data integration with AI-driven syncs and automatic schema updates, ensuring efficient extraction from varied sources. Fully managed with high reliability, it's ideal for BI teams seeking clean data with minimal effort. Fivetran connects seamlessly to CRM systems and other sources, prioritizing ease of use for streamlined ETL processes.
Key features:
- Automatic schema updates for seamless data integration
- High reliability and minimal maintenance
- Pre-built connectors for diverse data sources
- Optimized for CRM systems and other business applications
3. Hevo Data

Hevo uses machine learning to automate error detection, manage schema drift, and streamline data syncing in real time. With its no-code, drag and drop interface and 150+ connectors, it’s designed for non-technical users and analytics teams that need reliable pipelines without engineering resources or complex setup. Hevo's no code tools simplify the use and functionality of ETL software, saving time and streamlining workflows.
Key features:
- No-code platform with drag-and-drop interface
- Real-time data syncing with machine learning enhancements
- Extensive connector library for diverse data sources
- Designed for non-technical users and analytics teams
4. Informatica

Informatica’s CLAIRE engine, equipped with advanced tools, powers intelligent metadata scanning, compliance automation, and smart transformation suggestions. It’s built for large, complex environments where security, governance, and scalability are non-negotiable, with robust capabilities in tracking data lineage to ensure data integrity and transparency. A strong fit for enterprise IT managers handling sensitive or regulated data flows.
Key features:
- Intelligent metadata management with CLAIRE engine
- Comprehensive data governance and security features
- Smart transformation suggestions for data workflows
- Scalable solutions for large enterprise environments
5. Talend
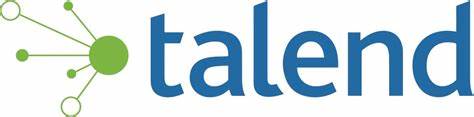
Talend applies machine learning to detect anomalies, assess data trust, and recommend transformation logic. It supports both cloud and hybrid environments, integrating data to facilitate the creation and management of data pipelines for teams handling data across multiple platforms with pre-built data connectors. Governance features and its Trust Score system make it popular among compliance-focused organizations.
Key features:
- Machine learning for anomaly detection and data trust assessment
- Cloud and hybrid environment support
- Pre-built data connectors for seamless integration
- Governance features with Trust Score system
6. Matillion
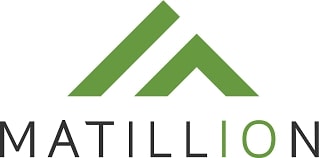
Matillion enhances cloud-based ETL with AI-guided transformation flows to manage complex transformations and transform data at scale. It integrates natively with Snowflake, Redshift, and BigQuery, making it a great choice for data teams already operating within modern cloud data warehouse ecosystems.
Key features:
- AI-guided transformation flows for complex data processing
- Native integration with leading cloud data warehouses
- Scalable solutions for large data volumes
- User-friendly interface for managing data workflows
7. Keboola
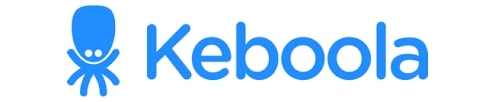
Keboola provides a low-code ETL platform with AI recommendations and collaboration features, ideal for scaling mid-sized teams. It supports diverse data sources, including XML files, ensuring seamless workflows and transformations. Keboola handles both structured and unstructured data, offering solutions for data flow to cloud platforms with pre-built and custom connectors.
Key features:
- Low-code platform with AI recommendations
- Support for structured and unstructured data
- Seamless integration with cloud platforms
- Collaboration features for mid-sized teams
8. IBM DataStage

IBM’s DataStage is a powerful AI-driven data integration tool, featuring a graphical interface that simplifies the creation of data integration solutions. Ideal for high-compliance sectors like finance and healthcare due to its strong security and data quality features, it excels in data movement and management, offering robust capabilities compared to Oracle Data Integrator.
Key features:
- Graphical interface for simplified data integration
- Strong security and data quality features
- Ideal for high-compliance sectors
- Robust data movement and management capabilities
9. AWS Glue

AWS Glue leverages machine learning to infer schemas, generate ETL code, and optimize job execution. As a serverless solution within the AWS ecosystem, it offers managed services that can be seamlessly integrated with existing data on AWS platforms, providing scalability, automation, and minimal infrastructure management for engineering teams. Its efficient loading processes ensure seamless data integration, while its focus on managing streaming data maintains data accuracy and consistency during ETL processes.
Key features:
- Machine learning for schema inference and ETL code generation
- Serverless solution with seamless AWS integration
- Scalable and automated data management
- Efficient loading processes for streaming data
10. Azure Data Factory
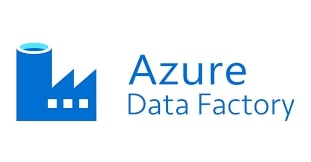
Azure Data Factory brings low-code data integration with a user-friendly interface and graphical tools to Microsoft’s ecosystem, with ML-powered data flow suggestions and performance tuning. It’s ideal for teams already using Azure services that want intelligent orchestration without heavy development overhead.
Key features:
- Low-code platform with user-friendly interface
- ML-powered data flow suggestions
- Seamless integration with Azure services
- Intelligent orchestration for efficient data workflows
11. Google Cloud Dataflow

Google Cloud Dataflow supports real-time and batch processing with embedded ML support during complex data transformation steps, effectively managing large volumes of data. Built on Apache Beam, it’s perfect for predictive analytics use cases and event-driven architectures that require intelligent scaling and advanced data modeling.
Key features:
- Real-time and batch processing support
- Embedded ML support for complex transformations
- Built on Apache Beam for scalability
- Ideal for predictive analytics and event-driven architectures
Which AI ETL Tool Fits Your Team?
Identify Your Team's Needs
Choosing the right AI ETL tool involves more than just evaluating your data stack. Evaluating the top ETL tools using specific criteria such as usability, scalability, and data security is crucial to making an informed decision. It requires a thorough understanding of your team’s size, workflow, goals, and the specific data pipeline processes necessary to manage and integrate your data effectively. Additionally, understanding the cost structure of different AI ETL tools is crucial to making an informed decision. Effective data management is essential for handling increasing data volumes and ensuring data integrity across all stages of the ETL process.
For Fast-Moving Analytics Teams
If you’re part of a fast-moving analytics team with limited engineering support, tools like Hevo Data or Fivetran are ideal. They deliver clean, real-time data with minimal setup, prioritizing automation and allowing teams to move quickly without writing code. These tools enable data analysts to create data pipelines efficiently, facilitating seamless data integration and processing.
For Enterprise IT Managers
For enterprise IT managers in regulated industries, platforms such as Informatica and IBM DataStage are recommended. These tools offer deep governance features, including compliance automation, secure deployments, and audit trails, making them suitable for environments where trust, security, and control are paramount. They are adept at managing streaming data and ensuring that data workflows remain compliant and transparent.
For Data Engineers Seeking Customization
Data engineers who desire full customization and open architecture may prefer Airbyte or Keboola. These platforms allow for flexible deployment and integration with transformation frameworks like dbt, providing the control needed to tailor solutions to specific requirements. They excel in creating complex data pipelines and handling increasing data volumes with precision.
Cloud Ecosystem-Specific Solutions
If your team operates within a specific cloud ecosystem, choosing a native solution is beneficial. Opt for AWS Glue for Amazon-first stacks, Azure Data Factory for Microsoft-heavy environments, and Google Cloud Dataflow for real-time ML pipelines inside the Google Cloud Platform. These solutions are optimized for handling streaming data and ensuring seamless data management across various cloud services.
The Future of AI in ETL Workflows
The integration of AI into ETL processes is set to revolutionize the data landscape.
Extract, Transform, Load (ETL) processes are crucial in defining the future of AI-enhanced ETL workflows. Soon, data pipelines will not only react to changes but will also anticipate them. We are on the brink of experiencing self-healing ETL workflows that autonomously identify issues, determine root causes, and implement solutions without human intervention.
Managing complex data pipelines will become increasingly vital as AI ETL workflows progress, requiring high adaptability and oversight. AI ETL tools are evolving to handle data transformations seamlessly, integrating and transforming data from diverse sources with minimal coding effort. This evolution signifies more than just a productivity boost—it marks a fundamental shift in the operational dynamics of data teams.
Semantic mapping is also gaining momentum. Instead of manually aligning fields across systems, AI can now understand context and match data based on meaning, not just structure. This accelerates integration and reduces errors across various systems.
Expect automated optimization to become more advanced as well. Future AI ETL tools will predict optimal sync windows, dynamically scale resources, and prioritize high-impact pipelines based on usage patterns—all seamlessly in the background.
For modern data teams, adopting AI-powered tools isn't merely about efficiency. It's about constructing an infrastructure that learns and improves with each iteration.
Streamline Intelligence with Airbyte
The way we move data is changing. Rigid pipelines and manual ETL workflows are being replaced by intelligent systems that adapt, optimize, and scale with your business.
Airbyte brings that intelligence to the forefront. With AI-assisted connector generation, automated schema handling, and integration with modern transformation tools like dbt, Airbyte helps teams build faster, break less, and maintain full control. Additionally, Airbyte allows developers to leverage their coding skills for customized workflows.
Whether you’re syncing thousands of records daily or managing sensitive pipelines across multiple environments, Airbyte gives you the flexibility to choose how and where you run your workflows — open source, cloud, or enterprise-grade.