Data Accuracy in 2025: What It Is & How to Ensure
Data accuracy is essential for increasing your organization’s productivity and achieving success.
Incorrect data can affect your organization's ability to make critical decisions or handle operations efficiently. It can even cause financial losses and damage the trust of your stakeholders.
High data accuracy indicates that your organizational dataset values closely align with the actual attribute value of real-world objects. This is essential for conducting reliable analysis and maintaining integrity in data-driven applications.
This article highlights how to measure and validate accuracy to maintain integrity in your organizational data assets.
What is Data Accuracy?
Data accuracy measures how precisely your data reflects real-world scenarios. It is a subset of data quality and integrity, measuring the level of correctness in information collected, utilized, and stored.
Consider a scenario where your IT team built a new navigation app. During testing, you search for a highly recommended restaurant. The app guides you to where it thinks the restaurant is, but it turns out to be incorrect. This misguided information shows the importance of accurate data for applications or websites with a large user base.
What Is the Importance of Data Accuracy For Business?
Data accuracy is essential for sales, accounting, marketing, and other departments of your organization. Here are some key reasons why data accuracy is important for your business:
- Data accuracy enables better decision-making on new products, hiring people, and setting pricing strategies.
- With accurate data, you can drive maximum value from AI models and algorithms.
- Precise data enables you to target the right customers with ads or the best sales strategy for more profits.
- Accurate data enhances customer or stakeholder satisfaction, which upholds and strengthens your business reputation.
- Data accuracy can help improve the confidence level of your organization’s team members in using data to achieve business outcomes.
- You can quickly troubleshoot the root cause of an issue when it occurs, minimizing the time and resources needed to detect and fix the errors.
What Are the Examples of Data Inaccuracies?
Let’s examine a few examples of data inaccuracies. Understanding these examples allows you to take appropriate measures to enhance the accuracy of the data.
Incomplete Data
Incomplete data occurs when required fields in datasets are missing. System errors, human errors, and incomplete user registration forms can lead to missing data.
For example, consider a scenario where some entries in the customer dataset are missing email addresses. When sending out promotional emails, these incomplete entries would result in some customers being left out of the marketing campaign.
Duplicated Data
Duplicated data occurs when the same information is repeated across multiple datasets. This can result in increased storage and operational costs. Identifying and rectifying these duplicates can be effort-intensive.
For example, if the same product information is accidentally entered into your organization’s dataset twice, you may misinterpret the proper distribution of data, leading to incorrect analysis.
Outdated Data
Outdated data arises when your database lacks relevant information or is not consistently updated to reflect changes, causing errors in analysis and decision-making processes.
Consider your organization’s customer dataset, which includes phone numbers that have not been updated in years. Attempting to contact the relevant customer for your product promotions using these outdated numbers would result in unsuccessful collaborations and wasted time.
Inaccurate Data Sources
Data sources from unverified social media accounts, online forums, and websites can provide incorrect, misleading, or incomplete information. This can severely impact the quality and reliability of the data used in various business processes.
Consider a scenario where you are conducting online surveys to assess your product efficiency. Some missing responses or biased samples can skew your understanding of customer satisfaction, and drawing conclusions from such surveys can impact your profitability.
What Are the Common Errors That Hinder Data Accuracy?
Human Error
Human errors happen when users make typographical mistakes, omit required fields, or misinterpretations during manual data entry.
For example, you might accidentally enter an incorrect postal code for a delivery package sent to a customer during the marketing campaign. This can result in the package being misdelivered or returned, wasting time and money.
Integration Issues
Varying data structures across sources can result in misalignment or data loss when integrating them. For example, if one dataset is organized hierarchically while another has a flat file structure, merging them may cause them to lose hierarchical relationships. This occurs as the flat files lack the ability to represent these associations between data entities.
In addition, inconsistencies in date formats, units of measurement, or naming conventions compromise accuracy during data integration. Adding low-quality data from one source can cause inaccuracies throughout the integrated dataset.
Lack of Data Validation
Incorrect or incomplete data can go undetected without proper data validation, hindering the data accuracy. This inaccuracy leads to unreliable analysis and misguided decisions.
For example, you are conducting a survey on customer satisfaction with your company’s products. Few customers may leave out required fields if no validation rules exist. Without the essential information, you cannot perform a valid customer satisfaction analysis.
System Error
System errors such as database crashes or hardware failures can result in data loss. If backups are not properly maintained, you cannot restore the lost data, significantly impacting data accuracy. A single software bug in your database system could incorrectly perform calculations or transformations, leading to inaccurate results.
💡Suggested Read: What is Data Matching?
What Is the Impact of Inaccurate Data on Businesses?
Inaccurate data can seriously impact your business. To thrive in an evolving marketplace, every part of your business must rely on accurate data.
According to a Trifacta study, 46% of data scientists spend over ten hours preparing datasets for analysis and AI and ML projects. If your team is dealing with inaccurate data, it can lead to wasted time and resources. This not only delays projects but also compromises regulatory compliance. Poor data quality essentially makes your data-driven projects more expensive and less likely to succeed.
How to Ensure Data Accuracy
Ensuring data accuracy involves continuous strategic planning and consistent effort. Here are a few practices that help in maintaining high accuracy:
Validate Data Upon Ingestion
You must utilize automated software tools to validate the information upon data ingestion. This approach ensures that only accurate and properly formatted data is entered into the system, minimizing errors and maintaining data integrity.
For example, when a field requires a date, the system can automatically reject entries that do not align with the specified date format.
Establish a Data Governance Team
Creating a dedicated governance team can define and enforce data quality standards, ensuring the accuracy of your organizational data assets. This team serves as the central authority for addressing data-related concerns, such as inconsistencies in customer or product information.
Conduct Regular Data Audits
Regular data audits involve reviewing the datasets periodically to identify inaccuracies before escalating. This can help maintain high data integrity, enhance decision-making processes, and prevent risks associated with misleading information.
Use Data Quality Tools
Utilizing data quality tools involves identifying and resolving incorrect, incomplete, or duplicate values within datasets. These tools help maintain large, high-quality datasets, especially when manual verification is not feasible.
Train Your Staff
Adequate training is essential for people involved in data processing. This involves understanding how to maintain accurate data, identify sources of errors, and implement effective quality control measures.
After proper training, your organization can significantly reduce the risk of data inaccuracies and maintain the integrity of the data assets.
How to Measure & Validate Data Accuracy?
Measuring and validating data accuracy can help you maintain the data integrity and use it for reliable analysis and reporting. The following section explains how to measure data accuracy:
Check for Missing Values, Fields, or Records
You must identify and rectify missing values, fields, or records within your datasets. Missing data can result in incomplete analysis or incorrect results.
Utilize effective data cleansing tools to remove the missing data from your database. In addition, you can regularly review your datasets to ensure all required information is available and complete.
Verify That Data Is Consistent
Inconsistencies, like using St. and Street for addresses in your organization’s customer dataset, can cause discrepancies when analyzing customer distribution by location. By verifying data consistency, you can spot fields or records that deviate from expected standards or formats. Establishing a standardized format within your organization can ensure data remains consistent across all datasets.
Identify Duplicate Records
Duplicate records can negatively affect the accuracy of data analysis and decision-making processes. Use data quality tools to detect and reduce the number of duplicate records in your dataset.
Ensure That Data Is Up-to-date
You must regularly check and refresh the data to ensure it represents up-to-date and accurate information. Outdated data can cause incorrect conclusions and decisions.
Compare Data with Trusted Sources
Validate your data by comparing it with trusted and authoritative sources. Cross-referencing with reliable external sources can help understand how to verify the accuracy of data.
For example, if you have a database of geographic locations, consider validating it against a reliable geographical database to ensure high precision in geo-analytics. This practice helps identify inaccuracies and ensures alignment with industry standards.
Verify That Data Conforms to the Established Quality Standard
Establishing Key Performance Indicators (KPIs) related to data quality can help monitor and measure accuracy over time. For example, a KPI could be the percentage of data records validated each month.
By consistently using your organization's data quality standards, you can maintain high-quality data, supporting more reliable analysis and decision-making.
Data Profiling
Data profiling provides an overview of structure, content, and relationships within your data. Using data profiling tools allows you to review and analyze the current state of your data. These tools also let you identify anomalies, outliers, and other data quality issues.
Check for Any Data Corruption or Unauthorized Modifications
Data corruption occurs from system errors, while unauthorized data modifications occur through malware attacks. You must regularly check for signs of data corruption or unauthorized alterations.
Compare with Historical Data
Comparing current data with historical information involves identifying trends, patterns, or anomalies. This comparison can help validate the accuracy of the recent data and highlight any significant deviations that may require investigation.
Data Accuracy vs. Data Integrity
The following table highlights the key differences between data accuracy and integrity.
Data Accuracy in ETL
Data accuracy is essential for projects that involve ETL processes. This process can help you extract, transform, and load data from varied sources into a target system for better analysis and reporting.
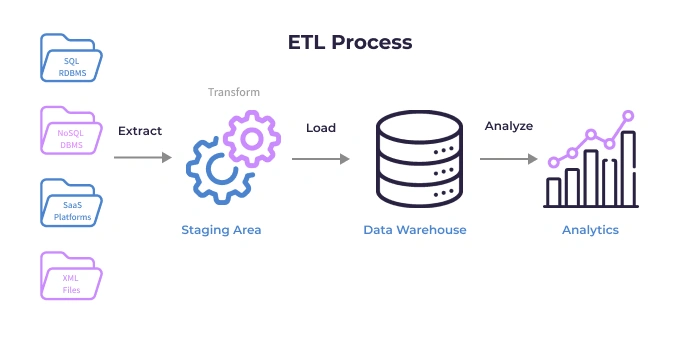
Here are three phases in the ETL process:
- Extract: The extraction phase includes capturing data from multiple sources. Some of the sources can have incorrect or incomplete data, compromising the quality and integrity of your entire dataset. You must use suitable data processing tools to address the inaccuracies.
- Transformation: In the data transformation phase, you need to convert and restructure the extracted data so that it aligns with the destination schema. Any inconsistencies during this process can affect the accuracy of analysis, reporting, and decision-making.
- Loading: In the loading phase, the transformed data is moved into the destination system. You must verify whether the data is loaded without errors and reliable for data analysis.
How Does Airbyte Help in Ensuring Accuracy for Efficient Data Integration?
Instead of manually performing each of the steps in the ETL process, you can use an automated data movement platform like Airbyte. This data integration and replication platform helps you extract data from several sources and load it into a destination of your choice without any data loss. You can even apply custom transformation logic using the Data Build Tool (dbt).
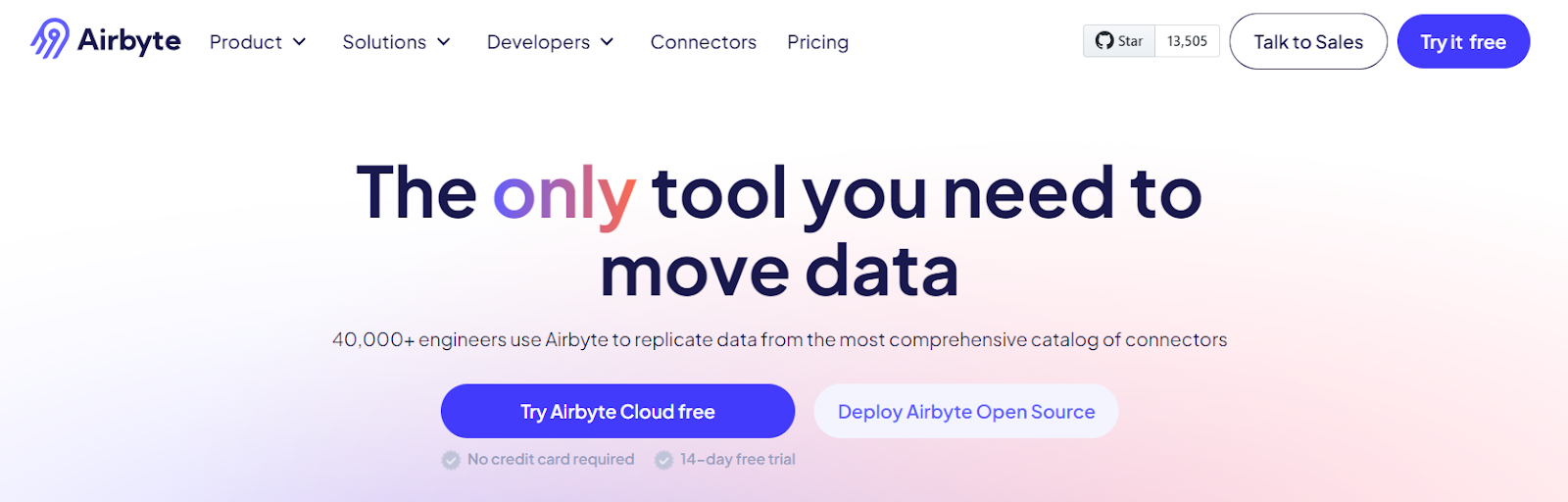
Here are some other unique features of Airbyte:
- Extensive Built-in Connectors: Airbyte allows you to access over 350 pre-built connectors, facilitating integration with numerous data sources.
- Connector Development Kit (CDK): It offers CDK for creating customized connectors tailored to your specific business requirements.
- Change Data Capture (CDC): Airbyte’s CDC approach can help seamlessly capture and synchronize changes from source databases to destination, keeping your target system up-to-date for intelligent decision-making.
- Developer-Friendly Pipelines: PyAirbyte, an open-source Python library offered by Airbyte, allows you to interact with Airbyte connectors and extract data from various sources within your Python workflows.
- Data Security: Airbyte supports SSL, TLS, and HTTPS encryption and SSH tunneling for secure data transfer. Airbyte is also compliant with SOC 2 Type II assessment and ISO 27001 regulatory standards, ensuring safe data management throughout the integration process.
Key Takeaways
Prioritizing data accuracy is crucial for maximizing the value of data and achieving strategic business objectives across diverse sectors. Understanding the examples and common errors that cause data inaccuracies can significantly help improve the accuracy of your organization’s data assets.
A robust data validation process allows your organization to ensure the datasets are consistent, reliable, and fit for the business purpose. Abiding by quality standards mitigates the risks associated with inaccuracies. Investing in data accuracy initiatives enhances better decision-making and strengthens relationships with stakeholders.
Frequently Asked Questions (FAQs)
1. Are there any tools to perform data accuracy checks?
Yes, you can perform data accuracy checks using Monte Carlo and BigEye platforms.
2. What is the most effective way to ensure data accuracy during ETL?
Your organization's thorough data quality testing ensures that the data being processed and transformed during ETL is accurate.
3. What are the few data accuracy testing methods?
Data cleansing, data validation, data profiling, and data auditing are a few testing methods you can follow to ensure accuracy.
💡Suggested Read: