What is Data in Statistics & Types Of Data With Examples
Data forms the bedrock of analysis and decision-making in statistics. Knowing data and its various types is essential for conducting meaningful statistical studies.
This article explores data and types of data in statistics. By understanding these concepts, you will be better equipped to interpret and utilize data effectively in your analysis.
What is Data?
Data, in statistics, is a collection of facts, observations, or measurements used for analysis and decision-making. Data can be numerical, such as counts or measurements, or categorical, such as labels or classifications.
In statistics, data serves as the starting point for analysis. It's what you examine, manipulate, and interpret to draw conclusions or make predictions about a particular phenomenon or population.
What is the Role of Data in Statistics?
Data plays an important role in understanding and drawing conclusions. It forms the foundation for analysis, providing the evidence needed to make informed decisions. Without data, your statistical studies lack the real-world information necessary to be meaningful.
Exploration is driven forward by examining and interpreting collected data. Through this process, you uncover patterns, relationships, and trends, aiding in making sense of the world around you. Ultimately, data serves as the guiding light, illuminating the path to understanding complex events.
What are the Types of Data in Statistics?

Data types are crucial in statistics because different types require different statistical methods for analysis. For instance, analyzing continuous data requires fundamentally different techniques from analyzing categorical data. Using the wrong method for a particular data type can lead to erroneous conclusions. Therefore, understanding the types of data you're working with enables you to select the appropriate method of analysis, ensuring accurate and reliable analytics insights.
In statistical analysis, data is broadly categorized into;
- Nominal Data
- Ordinal Data
- Discrete Data
- Continuous Data
Each type has its own characteristics, examples, and applications, which are essential for understanding and interpreting statistical information effectively.
Qualitative Data
Qualitative data, also known as categorical data, consist of categories or labels that represent qualitative characteristics. It simply categorizes individuals or items based on shared attributes.
There are two types of qualitative data:
Nominal Data
Nominal data are categories without any inherent order. Examples include gender (male, female), types of fruits (apple, banana, orange), and city names (New York, London, Paris). Nominal data are typically analyzed using frequency counts and percentages. For example, counting the number of males and females population or the frequency of different types of fruits sold in a specific region.
Ordinal Data
Ordinal data are categories with a natural order or ranking. Examples include survey ratings (poor, fair, good, excellent), educational levels (high school, college, graduate school), and socioeconomic status (low, middle, high). Ordinal data are used for ranking or ordering data, and they can be analyzed using median and mode, as well as non-parametric tests like the Mann-Whitney U test.
Quantitative Data
Quantitative data, also known as numerical data, consists of numbers representing quantities or measurements. Unlike qualitative data, which categorizes individuals or items based on attributes, quantitative data can be measured and expressed numerically, allowing for mathematical operations and statistical data analysis.
There are two types of Quantitative Data:
Discrete Data
Discrete data are distinct, separate values that can be counted. Examples include the number of students in a class, the count of defects in a product, and the number of goals scored in a game. Discrete data are used for counting and tracking occurrences, and they can be analyzed using measures of central tendency such as mean and median, as well as discrete probability distributions like the Poisson distribution.
Continuous Data
Continuous data can take any value within a range. Examples include height, weight, temperature, and time. Continuous data are used for measurements and observations, and they can be analyzed using mean and median, as well as continuous probability distributions like the normal distribution.
💡Suggested Read: What is Data Matching?
Difference Between Qualitative vs Quantitative Data
Quantitative and qualitative data exhibit significant differences. The fundamental distinctions are explored in the table below.
Examples of Qualitative Data
Some examples of qualitative data include:
Documents
Documents are a prevalent form of qualitative data, comprising materials like letters, diaries, blog posts, and digital images. These sources offer valuable insights into various research topics by providing firsthand accounts of individuals' thoughts and experiences. They are precious for understanding historical events, offering unique perspectives. When examining qualitative documents, you can use platforms like Flipsnack to present and share these materials in an interactive, digital format, helping to enhance the interpretation process and extract deeper meaning from the text, considering its potential for multiple interpretations.
Case Studies
Case studies are frequently utilized qualitative research methodolody, involving detailed investigations into specific individuals, groups, or events. They offer insights into complex phenomena, shedding light on human thought processes, behaviors, and influencing factors. While valuable, case studies have limitations due to their reliance on a small sample size, potentially leading to a lack of representativeness and researcher bias.
Photographs
Photographs serve as a valuable form of qualitative data, providing insights into various visual aspects of human life, such as clothing, social interactions, and daily activities. They can also document changes over time, such as urban development or product evolution. Apart from their informational value, photographs can evoke emotions and visually capture human behavior complexities.
Audio Recordings
Audio recordings represent raw and unprocessed qualitative data, offering firsthand accounts of events or experiences. They capture spoken language nuances, emotions, and nonverbal cues, making them valuable for research purposes. Audio recordings are commonly used for interviews, focus groups, and studying naturalistic behaviors, albeit requiring meticulous analysis due to their complexity.
Examples of Quantitative Data
Some examples of quantitative data include:
Age in Years
Age commonly serves as a quantitative variable, often recorded in years. Whether precisely documented or categorized broadly (e.g., infancy, adolescence), age is a vital metric in various contexts. It can be represented continuously in units like days, weeks, or months or dichotomously to differentiate between child and adult age groups. Understanding age distribution facilitates demographic analysis and informs decisions across sectors like education and healthcare.
Height Measurement in Feet or Inches
Gathering quantitative data involves various methods. For instance, if you aim to measure the height of a group of individuals, you could utilize a tape measure, ruler, or yardstick to collect data in feet or inches. Once data is gathered, it can be used to compute the average height of the group and discern patterns or trends. For instance, you might observe correlations such as taller individuals tend to have higher weights or gender disparities in average height. Quantitative data proves invaluable for comprehending human behavior and making informed predictions.
Number of Weeks in a Year
A year comprises 52 weeks, providing a precise and measurable quantity, which exemplifies quantitative data. This type of data is crucial in scientific research because the number of weeks allows for standardized comparisons across studies.
For instance, you can track changes in a population's health over 52 weeks (a year) and compare those findings to studies that measured health changes over 26 weeks (half a year). This consistency in measurement enables the identification of trends and relationships between variables more effectively, leading to insightful analyses.
Revenue in Dollars
Quantitative data, which is numerical and measurable, encompasses metrics like revenue expressed in any form of currency. This data type proves invaluable for assessing various aspects, such as a company's financial performance, products sold on a website and its traffic volume, or product sales quantity. The data is commonly gathered through surveys, experiments, or data analysis, enabling statistical methods to discern trends and correlations.
Distance in Kilometers
Distance measurement stands as another quintessential example of quantitative data, with kilometers being the universally accepted unit for long distances. Kilometers provide a manageable scale for expressing distances without necessitating unwieldy numbers. For instance, kilometers offer a convenient and widely understood metric when measuring the distance from a source to destination.
Since statistical analysis hinges on a unified data set, Airbyte can help you bridge the gap. It effortlessly allows you to gather and centralize information, eliminating the hassle of data collection.
💡Suggested Read: Features of Graph Database in NoSQL
Simplify Statistical Data Analysis with Airbyte
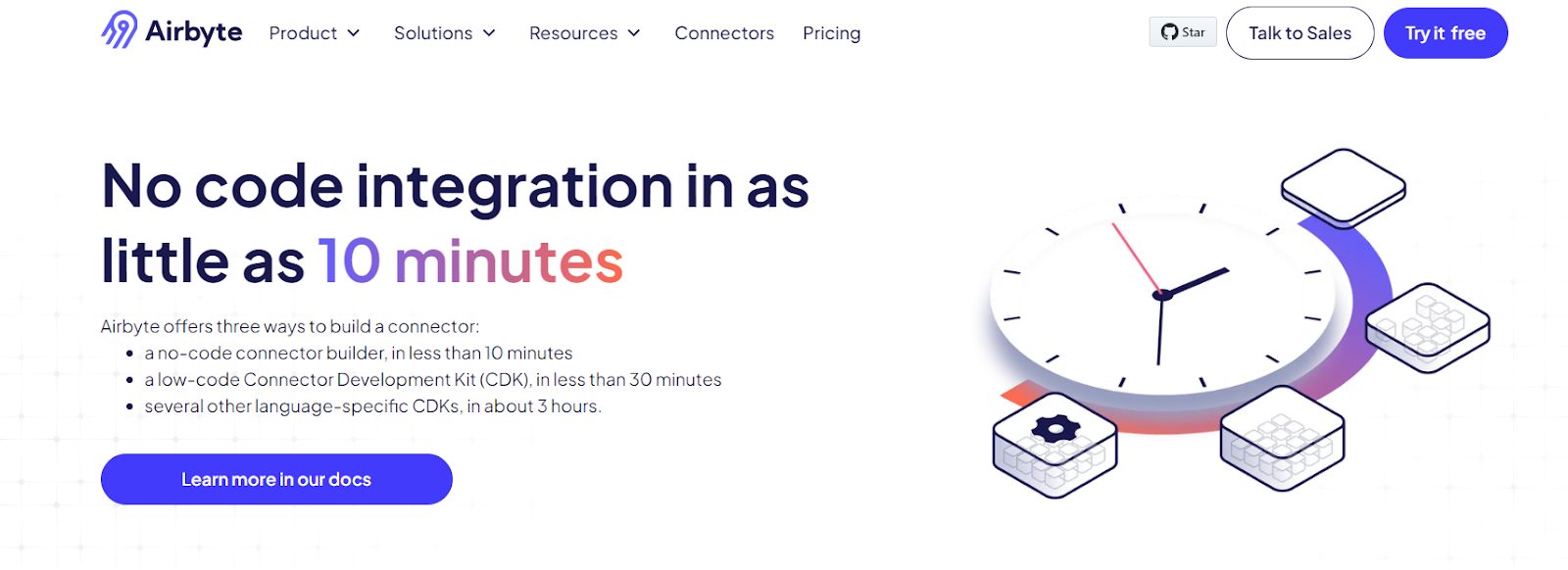
Airbyte, a data integration platform, simplifies the process of integrating and replicating data from various sources. Once centralized, this data empowers statisticians to perform in-depth analysis. By eliminating manual data transfer and ensuring consistent data flow, Airbyte saves valuable time and resources. This allows them to focus on what matters most—extracting meaningful insights from the data.
Here’s what Airbyte offers:
- Connectors: Airbyte has a vast library of pre-built connectors, exceeding 350 sources and applications. This lets you connect to a wide range of data sources effortlessly, eliminating the need for custom development in many cases.
- Open-source and Customizable: Airbyte is an open-source platform providing access to its source code for transparency and customization. You can modify existing connectors or build entirely new ones using their Connector Development Kit (CDK).
- Monitoring and Integrations: Airbyte allows you to seamlessly integrate with monitoring platforms like Datadog, enabling you to keep track of data pipeline health and performance. Additionally, it supports integrations with popular workflow orchestration tools like Airflow, Prefect, and Dagster for streamlined data pipeline management and processing.
- Security Features: Airbyte takes security seriously. It offers features like dedicated secret stores to store sensitive information. The platform also supports OAuth for secure authentication and role-based access control for user management.
- PyAirbyte: PyAirbyte, a Python library, lets you programmatically interact with Airbyte's vast library of pre-built connectors. This allows you to automate data integration tasks and leverage Airbyte's extensive functionality through code.
Conclusion
Data and types of data in statistics are significant as they aid in understanding global phenomena and guiding your decision-making process. Statistics data encompass various data types, each with its use cases. However, by comprehending these data types, you can utilize them effectively to obtain the most accurate insights possible.
💡Suggested Read: