What Is Data Modernization Strategy: Benefits & Examples
Data has become an important asset to most organizations today. Collecting and analyzing data can give you deeper insights into your organization’s operations, maintenance, budget expenditures, and overall performance. Data helps you plan and streamline the execution of your current and upcoming projects, aligning your efforts to reach the outlined business goals and objectives.
However, you can only achieve better results if your data is clean, consistent, accessible, and accurate. This article introduces data modernization as a solution to optimize your data processes and drive business agility, innovation, and growth.
What Is Data Modernization?
Data modernization is a strategic approach to updating and optimizing your organization’s data infrastructure, tools, and practices. This process often implies migrating from legacy systems to modern, cloud-based technologies to overcome data silos, improve data quality, and enhance data security and governance.
Data modernization is vital in transforming large amounts of your organizational data into actionable insights for efficient and informed decision-making. You can also leverage it to meet your ever-evolving needs for data-driven business operations and analytics. Data modernization helps you realize the potential of your data assets to support your organization.
What Is Data-First Modernization?
Data-first modernization is a shift from traditional infrastructure-centric modernization to a data-centric model, where all your business processes and functions revolve around your data. It requires you to decide which data is crucial for your organization, where it resides, and how to make the most of it.
Data-first modernization goes beyond updating technology and cultivates a data-driven culture where employees across all levels are data-literate with skills to manage and utilize data. It also involves implementing robust data protection and governance measures to ensure your data’s quality, security, and compliance.
The data-first modernization approach encourages seamless integration of diverse data sources and automation of data pipelines and workflows using artificial intelligence (AI) and machine learning (ML). This helps you streamline data movement and processing, trace patterns, analyze trends, and make future predictions to stay ahead of the curve.
Why Is Data Modernization Important?
Data is undoubtedly a valuable asset, but without a systematic plan, leveraging your ever-growing data will get challenging and turn it into a liability. Data modernization addresses this issue by providing a scalable, accommodating, and future-proof foundation for your organization’s changing business needs.
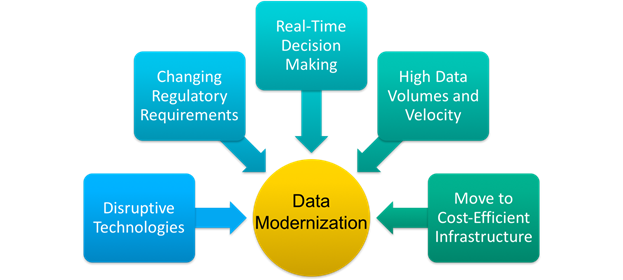
Data modernization eliminates outdated information and democratizes access to reliable and trustworthy data throughout your organization. This allows your staff to explore and analyze relevant data independently, improving the speed and agility of your organization’s operations, product development, and decision-making processes.
Data modernization facilitates real-time insights that help you to react immediately to changing market dynamics and capitalize on high-impact, short-term opportunities. It also enables you to protect your organization’s intellectual property and innovations from cyber threats and data breaches, providing an edge against competitors.
Benefits of Data Modernization
Data modernization can include multiple processes, such as data integration, cleansing, transformation, consolidation, and migration. This comprehensive approach enhances the quality, reliability, and accessibility of your data, providing significant benefits to your organization. Some of them are outlined below:
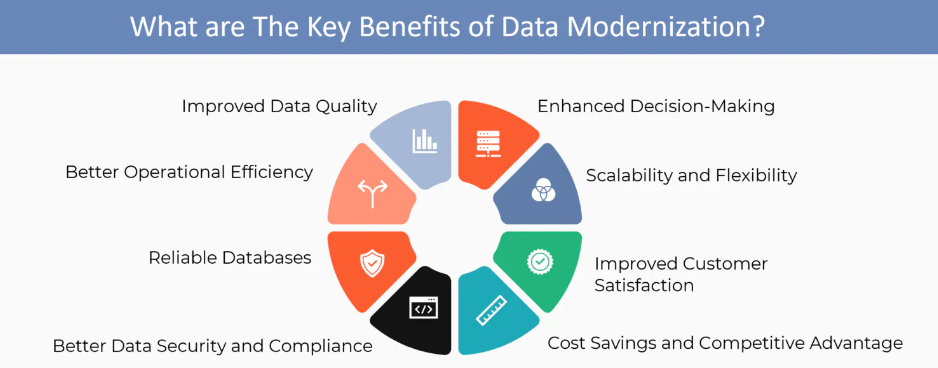
Enhanced Regulatory Compliance
By implementing robust data governance practices, you can easily adhere to evolving data privacy regulations like GDPR and CCPA and ensure consistent data definitions and lineage. You can also utilize various data governance platforms such as Collibra, Ataccama, and IBM to streamline and automate these processes.
These platforms provide built-in security features, including encryption, access controls, audit trails, and intrusion detection to protect sensitive information. This saves you from hefty fines and reputational damage associated with data breaches and non-compliance.
Increased Agility and Scalability
Data modernization enables you to scale your data infrastructure based on your evolving business needs. Cloud-based platforms and modern data architectures provide the flexibility to handle your increasing data volumes without significant investments in physical hardware. This scalability ensures your organization can quickly adapt to changing market trends and demands.
Improved Data Accessibility
Data modernization improves data accessibility by breaking down data silos and creating a unified data environment. With centralized data repositories and user-friendly interfaces, your departments across the organization can access the information whenever needed. This democratization of data empowers employees at all levels to make data-driven decisions, fostering a culture of transparency.
Better Customer Experience
Data modernization can help you identify behavior patterns, gain deeper insights into your customers’ preferences, and tailor your offerings and recommendations accordingly. It also enables you to optimize customer touchpoints, deliver targeted marketing campaigns, and proactively address issues before they escalate, streamlining the overall customer experience.
Enhanced Operational Efficiency
Data modernization can help you automate repetitive tasks and processes. By streamlining and automating your workflows, you can free up resources for more complex strategic initiatives. This optimized resource allocation based on real-time data insights helps achieve proactive decision-making and increases efficiency and productivity across your organization. You can also implement predictive maintenance capabilities to prevent equipment failures and downtime.
Data-driven Collaboration and Experimentation
Many advanced data analytics tools facilitate self-service analytics and data visualization, allowing even non-tech teams to explore data insights and contribute to knowledge sharing. This fosters seamless collaboration among interdisciplinary teams and encourages rapid prototyping and iteration of ideas, accelerating your innovation cycles.
Enhanced Data Governance and Quality
Data modernization helps establish robust data governance frameworks, ensuring data accuracy, consistency, and integrity across the organization. You can maintain high data quality standards with standardized data definitions, automated data quality checks, and comprehensive metadata management. This reduces errors and inconsistencies, leading to more reliable and trustworthy data.
Action-Driven Business Insights
With modernized data systems, you can leverage advanced analytics tools, ML, and AI to gain deeper insights from your data. By integrating diverse data sources and applying sophisticated analytical models, you can predict trends, identify issues before any irreversible damage is caused, and make more informed strategic decisions. This capability transforms raw data into actionable insights, driving sustainable growth.
Stronger Business Continuity and Disaster Recovery
Modern data platforms often include robust disaster recovery and business continuity features. By leveraging cloud-based backups, automated failover systems, and real-time data replication, you can ensure your data remains safe and accessible even during hardware failures or other disruptions. This resilience minimizes downtime and protects critical business operations, providing continuity and reliability.
Improved Cost-Efficiency
With data modernization, you can eliminate the need to maintain outdated, expensive, on-premise hardware and use cloud-based solutions instead. These solutions offer flexibility, scalability, and pay-as-you-go models, reducing the overall IT infrastructure costs. Additionally, improved data quality and automation minimize manual errors and rework, significantly saving computational expenses.
Components of Data Modernization
Data modernization is a comprehensive process that involves updating and enhancing an organization's data infrastructure to leverage modern technologies and practices.
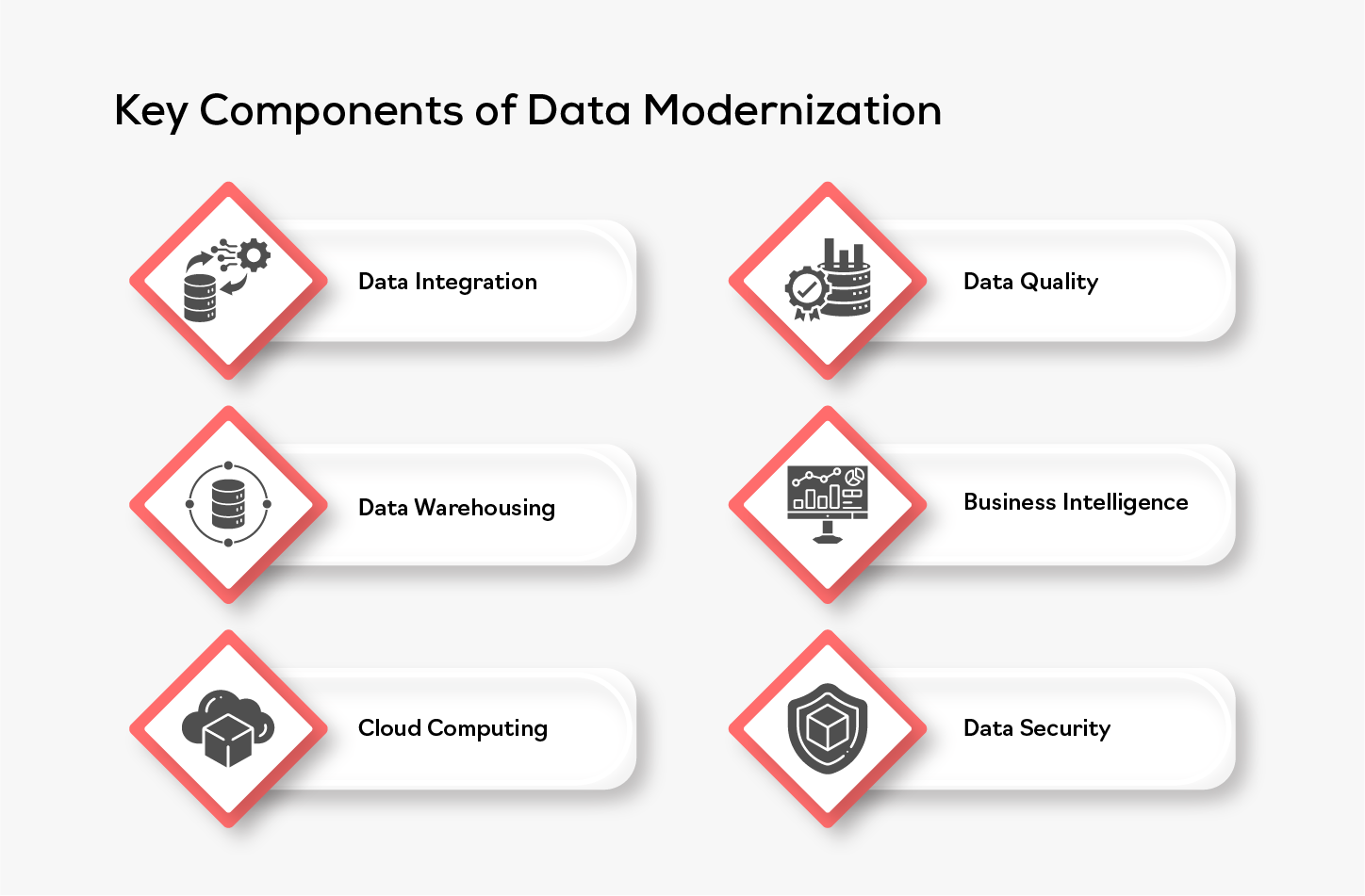
Here are the essential components of data modernization:
Data Integration
Data integration is a crucial component of data modernization. It involves consolidating your data from diverse sources, such as CRMs, ERP systems, APIs, and supply chains, into a unified view for seamless access and analysis.
Effective data integration ensures that your data is accurate, up-to-date, and available across the organization, facilitating better decision-making and operational efficiency. Some popular data integration tools include Airbyte, Fivetran, Pentaho, Qlik, and MuleSoft.
Data Quality
Maintaining high data quality is critical for ensuring data reliability and validity. This component focuses on implementing processes and technologies to clean, validate, and enrich data.
Clean and consistent data is essential for accurate analytics and reporting, reducing the risk of errors and improving overall data trustworthiness. Some data quality tools are OpenRefine, Informatica, Talend Data Fabric, and SAP.
Data Warehousing
Data warehousing involves creating centralized repositories to store and manage the influx of data from various sources. Modern data warehouses can efficiently handle high-volume, high-velocity data and support complex queries and analytics.
These tools are essential to your organization's data architecture, providing a robust data storage and retrieval infrastructure. Some data warehouse solutions you can utilize include Amazon Redshift, Snowflake, and BigQuery.
Cloud Computing
Migrating your datasets to the cloud is pivotal in data modernization. Cloud platforms offer scalable, flexible, and cost-effective solutions for storing and processing data.
The three basic categories of cloud computing services are SaaS, IaaS, and PaaS. These services allow you to scale your data infrastructure, access advanced analytics tools, and improve disaster recovery capabilities.
Business Intelligence and Data Visualization
Business intelligence (BI) and data visualization tools enable you to transform raw data into interactive dashboards and reports that are easy to understand and analyze. These tools even empower your non-technical teams to make data-driven decisions based on their interpretations.
Data Governance
Data governance ensures proper data management within the organization. It involves establishing policies, standards, and procedures to ensure data security, privacy, and compliance.
A robust data governance framework helps you maintain data integrity while protecting against unauthorized access and misuse. Axon, Colliobra, and IBM data governance are some data governance tools you can explore.
By integrating these components of data modernization, your organization can achieve a robust, scalable, and efficient data infrastructure. Data modernization equips you with the tools and capabilities needed to thrive in a competitive business market and stay ahead of the curve.
Stages of Data Modernization
Data modernization is a multi-stage process that helps you to transform your organization’s data infrastructure to improve efficiency, scalability, and security. Here are the key stages of data modernization:
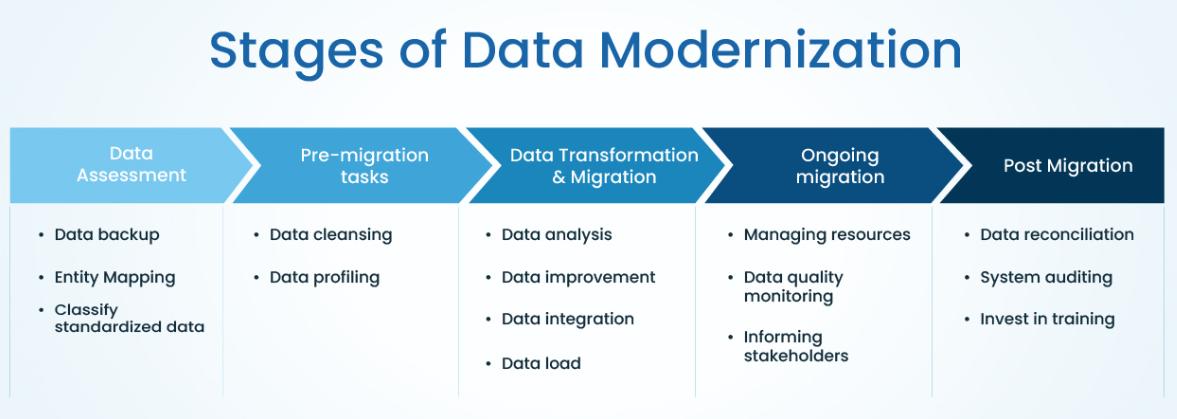
Assessment and Planning
The first step involves creating a data inventory. This includes taking backups to prevent data loss, mapping source entities to identify attributes that can cause issues during migration, and classifying data to establish standards. These steps ensure a clear understanding of your data and potential pitfalls.
Pre-Migration
Before migrating data, you should focus on resolving inconsistencies and preparing your database for migration. Data cleansing helps in removing typos, duplicate entries, and anomalies, while data profiling allows you to analyze data's statistical properties to understand its characteristics. This stage aims to ensure data accuracy and reliability before any transition occurs.
Transformation
Data transformation involves restructuring and manipulating data to fit the target system's requirements. This might include transforming data formats or enriching data with additional attributes. These processes enhance data integrity, making it suitable for advanced analytics and decision-making.
On-Going Migration
This stage focuses on resource management and data quality monitoring. Your data team must manage budgets and technology required for data accuracy to identify and address any issues that might arise. Additionally, keeping stakeholders informed of progress and potential pitfalls can help foster transparency and build trust.
Post-Migration
Post-migration involves validating the migrated data against your original datasets to ensure completeness and accuracy. You can also check whether your new data infrastructure functions as intended by running system audits. Investing in programs that train your employees on new tools and data management practices ensures your organization’s potential to transition successfully into a modernized data environment.
By systematically addressing issues at each stage, your organization can tackle immediate challenges and lay the groundwork for a streamlined and resilient data modernization journey.
Developing a Data Modernization Strategy
Developing a data modernization strategy involves a series of carefully planned steps that must align with your organization’s business goals. Following these steps, you can transform your data infrastructure, improve data quality, and leverage data for better decision-making and competitive advantage.
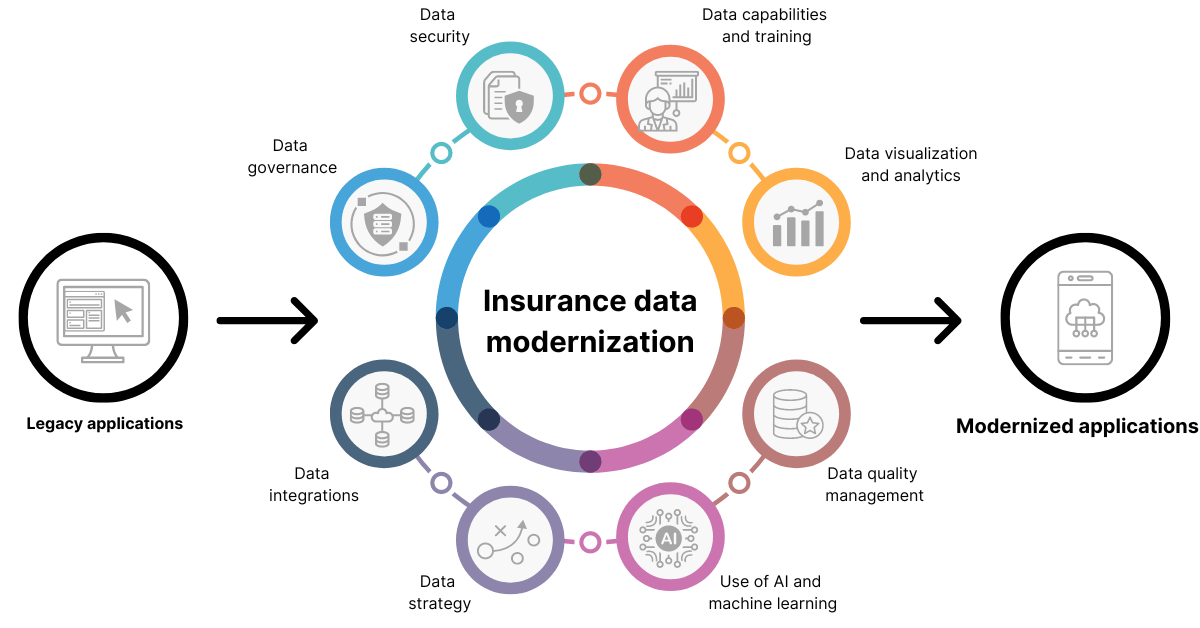
The steps outlining the process of developing a data modernization strategy are mentioned below:
Set Clear Business Objectives
Begin by defining the business objectives that will guide your data modernization efforts. These objectives should revolve around your company's overall strategic goals and address specific pain points and opportunities for growth. Clear objectives provide a roadmap for your modernization journey, ensuring all efforts are targeted and measurable.
Evaluate Current Infrastructure
Conduct a complete assessment of your existing data infrastructure, processes, and capabilities. This includes examining hardware, software, and data management processes, such as data quality, accuracy, governance practices, and regulatory compliance. The assessment helps you identify areas that need improvement. Understanding the pros and cons of your current setup is crucial for planning effective modernization initiatives.
Identify Key Business Drivers
Identify the key business drivers that will influence your data modernization strategy. These drivers include market trends, customer demands, new revenue opportunities, and technological advancements. Recognizing these factors helps prioritize modernization efforts and ensures they support overall business goals.
Develop a Comprehensive Data Strategy
Create a detailed data strategy that outlines how your organization will manage data throughout its lifecycle. This strategy should cover data acquisition, storage, processing, and usage, ensuring efficient and effective data handling. A well-defined data strategy will also establish KPIs to measure success, governance policies, and compliance measures to ensure your data’s integrity.
Prioritize Modernization Initiatives
You can not tackle all modernization projects simultaneously. Prioritize initiatives based on their feasibility, potential impact, and expected return on investment. This approach helps allocate resources efficiently and ensures you address the most critical projects first.
Select Appropriate Technologies
This might involve selecting data integration platforms, data storage solutions, quality management tools, and analytics platforms. Evaluate them depending on their ability to meet your business needs, integrate with existing systems, and scale with future growth. Selecting appropriate technologies ensures that your modernization efforts are built on a solid, flexible foundation.
Implement Data Governance
Establish robust data governance practices to maintain data quality, consistency, and security. Data governance involves outlining policies and standards for data management, assigning roles and responsibilities, and monitoring compliance. Effective governance ensures that data is reliable and trustworthy for decision-making.
Plan Data Integration and Migration
Develop a detailed plan for integrating and migrating data from legacy systems to modern platforms. It involves extracting and transforming data to fit the new infrastructure. This should minimize disruptions to business operations and ensure data integrity. Proper planning helps avoid common pitfalls such as data loss or compatibility issues associated with data migration.
Ensure Data Security and Compliance
Prioritize data security and compliance throughout your modernization efforts. Implement measures to protect data from breaches and cyber-attacks and ensure compliance with relevant HIPAA, PII, and GDPR. Security and compliance are crucial for maintaining customer trust and avoiding legal penalties.
Enhance Data Analytics
Invest in advanced analytics capabilities like ML, AI, and statistical modeling to extract valuable insights from your modernized data systems. Enhanced analytics can help you drive better decision-making, identify emerging business opportunities, and improve operational efficiency.
These steps complete your groundwork for developing a data modernization strategy. However, this is an ongoing process and requires continuous evaluation and adaptation to evolving technologies and business needs.
AI & Data Modernization: How Does the Integration Look?
AI and data modernization are powerful technologies on their own. However, integrating AI into data modernization processes can drastically change how your organization operates, enabling a more agile, efficient, and insightful approach to data management. This integration encourages intelligent automation, which empowers you to streamline repetitive tasks and frees up resources for high-level strategic planning that results in innovation and growth.
Dynamic adaptability is one of the most significant advantages of combining AI and data modernization. With the latest data infrastructures, you can support ML algorithms in enhancing their sensitivity and ability to adjust to continuously changing market dynamics. This capability is crucial for risk management, smart manufacturing, and improving product development based on recent trends and advancements.
AI-powered models, such as computer vision, deep learning, and natural language processing (NLP), combined with data modernization, can greatly help organizations across industries. For example, computer vision applications can improve quality control in the manufacturing sector and assist in diagnostic imaging in healthcare.
Deep learning models can use artificial neural networks with multiple layers to learn complex data patterns and drive predictive and prescriptive analysis. With NLP, you can provide better customer interactions using chatbots and get a general market sentiment about your brand through sentiment analysis.
AI and data modernization can also streamline decision-making processes by providing your organization with data-driven, real-time insights. This translates to improved operational efficiency, reduced costs over time, the ability to respond to market demands swiftly, and having an edge against your competitors.
Examples of Data Modernization
Data modernization is so much more than simply replicating the capabilities of traditional systems in the cloud. It involves leveraging advanced technologies and practices to transform how data is managed and utilized. Some examples of data modernization implementation at your organization can include the following.
Utilizing Big Data Technologies
Adopting big data tools and frameworks allows you to store, process, and analyze high volumes of datasets more efficiently. By leveraging tools like Hadoop and Spark, you can easily handle multiple datasets, extract valuable insights from your data, and act on them quickly. This helps you save on your resources and computational expenses.
Adopting a DaaS Approach
The Data-as-a-Service (DaaS) model offers on-demand access to a centralized data pool. This eliminates the need to maintain separate data stores and promotes seamless sharing between various departments and external partners, fostering collaboration and transparency. DaaS reduces the burden of managing physical infrastructure and provides a cost-effective, scalable solution for your evolving business needs.
Implementing Cloud-Based Storage and Processing
Transitioning to cloud-based storage and processing solutions, such as Microsoft Azure and AWS, provides scalability, flexibility, and adaptability based on your organization’s evolving data needs. It enables you to store large datasets securely, access them from anywhere, and process data at a much faster rate.
Enhancing Data Security and Privacy Measures
Data security and privacy measures help you protect sensitive information from cyber-attacks and ensure compliance with regulations. Implementing robust encryption, access controls, and monitoring systems allows you to avoid breaches, prevent unauthorized data misuse, and identify suspicious activity. Safeguarding your data integrity helps maintain trust with stakeholders and customers.
Utilizing IoT for Data Collection
Leveraging the Internet of Things (IoT) for data collection provides real-time data from connected devices such as home appliances, fitness trackers, and industrial equipment. Monitoring this continuous data stream allows you to optimize operations, gain deeper insights into product usage and customer behavior, and perform predictive maintenance to avoid unwanted machine failures
Transitioning from ETL to Modern Data Integration Tools
Shifting from conventional ETL processes to modern integration tools that utilize the (EL)T approach provides more flexible and automated data pipelines. These tools support multiple data sources and destinations, offer incremental data loading, and near real-time data synchronization, encouraging a more agile integration strategy. This reduces manual efforts and improves data accuracy and scalability
Integrating Advanced Analytics and BI Tools
Advanced analytics empowers you to utilize AI and ML tools to study hidden patterns and emerging trends in your data. BI tools then translate this analysis into clear, actionable dashboards and visualizations, allowing even non-tech teams to contribute to knowledge sharing and practice data-driven decisions. This also helps in stakeholder buy-in, as your proposed plans and strategies have supporting data that everyone understands.
Implementing Edge Computing for Data Processing
Edge computing involves processing data closer to its source, such as local servers, rather than relying solely on centralized data centers. This approach significantly reduces latency, optimizes bandwidth usage, accommodates high-volume, high-velocity data, and provides real-time insights even when the network is compromised.
Developing a Data-Driven Culture
Fostering a data-driven culture within an organization plays an integral role in data modernization. A data-driven culture emphasizes backing your claims or proposals with evidence rather than intuition. This prompts transparency and the opportunity to think critically about how data can lead to innovative solutions and improved business outcomes. Data-driven culture also increases your staff’s efficiency and adaptability.
Investing in Data Literacy and Training Programs
Many organizations have become data-centric in their business processes. This makes knowing how to manage, utilize, and interpret data effortlessly crucial for all your teams. Conducting training programs that equip your employees with skills to use data tools and understand visualizations and dashboards helps them effectively communicate their needs or observations.
How Is Airbyte Making a Difference in Data Modernization?
Most organizations across industries understand the importance of data modernization and have either implemented it, are in the process of implementing it, or are planning to implement it. In 2019, Deloitte surveyed 504 IT firms in the US and recorded its observations in an article by Deloitte Insights. This article showcases a graph (present below) representing the percentage of IT firms adopting data modernization.

According to the survey, over 65% of the respondents have yet to implement complete data modernization. 44% of the firms acknowledge that a lack of understanding of technology is a high hurdle during the implementation process. This is where Airbyte comes into the picture and significantly lowers the barrier to getting started with data modernization, even for those without any technical expertise.
Airbyte is a no-code ELT data integration platform with an intuitive and user-friendly interface. It allows tech and non-tech teams to seamlessly build and manage their organization’s data pipelines. By centralizing your data, Airbyte provides a unified view, enabling you to share your observations and interpretations easily. This further promotes cross-functional collaboration.

Here are some of its features that can ease you into the data modernization process:
- Extensive Library of Connectors: Airbyte offers over 350 pre-built connectors that support various data sources and destinations. This library allows for a quick setup and seamless data integration from multiple platforms within a few minutes. However, Airbyte also provides you the flexibility to build custom connectors using the no-code Connector Development Kit.
- Change Data Capture (CDC) and Incremental Sync: With CDC, you can detect and capture only the incremental changes in your source data, ensuring efficient data pipelines and reduced resource consumption. This is particularly beneficial when dealing with constantly evolving datasets, a common occurrence in modern data architecture.
- Complex Data Transformations: You can easily integrate Airbyte with dbt (Data Build tool) and transform raw data into a suitable format for further analysis and reporting. dbt allows you to leverage its SQL capabilities to create custom transformations.
- Vibrant Community: Airbyte also provides an open-source version that empowers you to start with data modernization without prior commitments. It also fosters a thriving community of over 15k members, offering access to resources and support for any challenges specific to your Aibyte implementations.
- Schema Change Management: Based on your configured settings for detecting and propagating schema changes, Airbyte allows you to automatically reflect the changes in source data schemas into your data warehouse or lake. This eliminates the need for frequent manual schema definition and mapping, saving valuable time and reducing errors in your data modernization process.
- Scalability and Flexibility: Airbyte scales with your organization's needs, whether you are managing small-scale data integrations or complex, large-scale data environments. Based on how Airbyte fits into your existing infrastructure, you can deploy it as a cloud or self-managed service. This adaptability is critical to handle growing data volumes and changing workloads.
You can explore Airbyte’s other features by referring to the official documentation.
Key Takeaways
Data modernization is a strategic approach to revamping your organization's data infrastructure, tools, and practices. It involves migrating from legacy systems to modern, cloud-based technologies to overcome data silos, improve data quality, and enhance data security and governance.
This article also delves into the stages of data modernization, outlining a clear process for assessment and planning, migration, and post-migration validation. Additionally, it provides steps to develop a data modernization strategy aligning with your business objectives. By adopting data modernization and leveraging the right tools, your organization can utilize the full potential of your data, driving sustainable growth.
FAQs
Q. What is the difference between data modernization and data migration?
Data migration involves moving existing data to a new location, while data modernization focuses on improving the quality, structure, and accessibility of your data.
Q. What is data platform modernization?
Data platform modernization refers to upgrading infrastructure and processes to make your data accessible and reliable. It involves adopting technologies and platforms like cloud migration, data lakes, and automation.
Q. What is the goal of data modernization?
Data modernization aims to transform and optimize data for better quality, accessibility, and utilization by using modern technologies and strategies.
Q. What is the difference between digital transformation and modernization?
Digital transformation fundamentally changes a business model, often with new technologies and processes. Contrarily, data modernization focuses on upgrading existing technology and systems for improved efficiency without major overhauls.