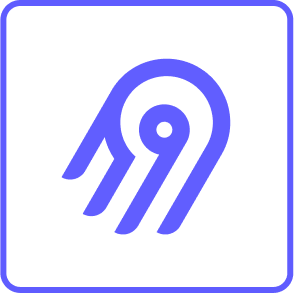

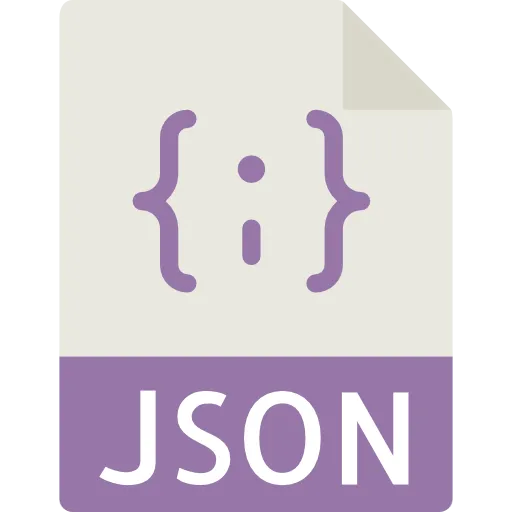
Building your pipeline or Using Airbyte
Airbyte is the only open solution empowering data teams to meet all their growing custom business demands in the new AI era.
- Inconsistent and inaccurate data
- Laborious and expensive
- Brittle and inflexible
- Reliable and accurate
- Extensible and scalable for all your needs
- Deployed and governed your way
Start syncing with Airbyte in 3 easy steps within 10 minutes



Take a virtual tour
Demo video of Airbyte Cloud
Demo video of AI Connector Builder
What sets Airbyte Apart
Modern GenAI Workflows
Move Large Volumes, Fast
An Extensible Open-Source Standard
Full Control & Security
Fully Featured & Integrated
Enterprise Support with SLAs
What our users say
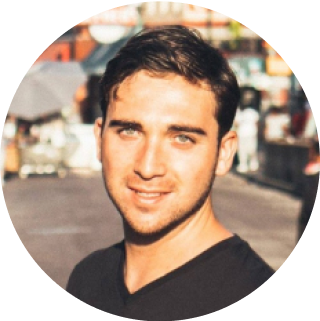
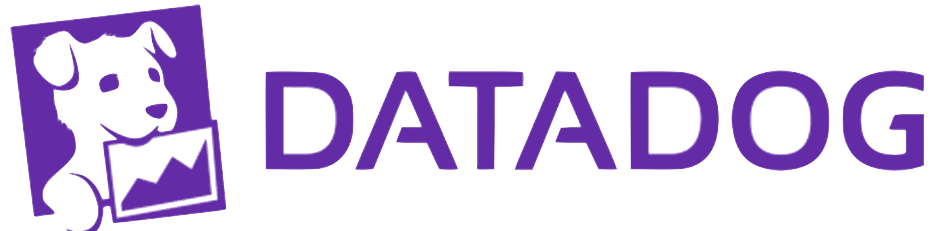
"The intake layer of Datadog’s self-serve analytics platform is largely built on Airbyte.Airbyte’s ease of use and extensibility allowed any team in the company to push their data into the platform - without assistance from the data team!"
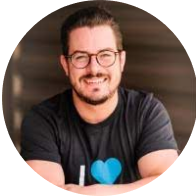
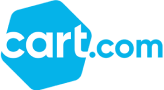
“Airbyte helped us accelerate our progress by years, compared to our competitors. We don’t need to worry about connectors and focus on creating value for our users instead of building infrastructure. That’s priceless. The time and energy saved allows us to disrupt and grow faster.”
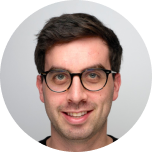

“We chose Airbyte for its ease of use, its pricing scalability and its absence of vendor lock-in. Having a lean team makes them our top criteria. The value of being able to scale and execute at a high level by maximizing resources is immense”
FAQs
What is ETL?
ETL, an acronym for Extract, Transform, Load, is a vital data integration process. It involves extracting data from diverse sources, transforming it into a usable format, and loading it into a database, data warehouse or data lake. This process enables meaningful data analysis, enhancing business intelligence.
Microsoft Teams is a collaborative chat-based workspace designed to enable collaborative teamwork across the Microsoft Office apps (Excel, PowerPoint, OneNote, SharePoint, Word, etc.). Workers can shift between applications within the suite without exiting the platform. Teams can chat through private or standard channels to share insights and ideas on projects in real time. Microsoft Teams streamlines the work process and brings teams together to complete projects more productively.
Microsoft Teams API provides access to a wide range of data that can be used to enhance the functionality of the platform. The following are the categories of data that can be accessed through the API:
1. Teams and Channels: Information about the teams and channels in which the user is a member, including their names, descriptions, and membership details.
2. Messages and Conversations: Access to messages and conversations within a channel, including the content of the messages, the sender and recipient details, and the time and date of the messages.
3. Files and Documents: Access to files and documents shared within a channel, including their names, sizes, and types.
4. Meetings and Calls: Information about scheduled meetings and calls, including the time, date, and participants.
5. Users and Groups: Information about users and groups within the organization, including their names, email addresses, and roles.
6. Apps and Bots: Access to third-party apps and bots integrated with Microsoft Teams, including their names, descriptions, and functionality.
7. Settings and Configuration: Access to the settings and configuration options for Microsoft Teams, including user preferences, notification settings, and security settings.
Overall, the Microsoft Teams API provides a comprehensive set of data that can be used to build custom applications and integrations that enhance the functionality of the platform.
What is ELT?
ELT, standing for Extract, Load, Transform, is a modern take on the traditional ETL data integration process. In ELT, data is first extracted from various sources, loaded directly into a data warehouse, and then transformed. This approach enhances data processing speed, analytical flexibility and autonomy.
Difference between ETL and ELT?
ETL and ELT are critical data integration strategies with key differences. ETL (Extract, Transform, Load) transforms data before loading, ideal for structured data. In contrast, ELT (Extract, Load, Transform) loads data before transformation, perfect for processing large, diverse data sets in modern data warehouses. ELT is becoming the new standard as it offers a lot more flexibility and autonomy to data analysts.
Microsoft Teams is a collaborative chat-based workspace designed to enable collaborative teamwork across the Microsoft Office apps (Excel, PowerPoint, OneNote, SharePoint, Word, etc.). Workers can shift between applications within the suite without exiting the platform. Teams can chat through private or standard channels to share insights and ideas on projects in real time. Microsoft Teams streamlines the work process and brings teams together to complete projects more productively.
JSON File is a tool that is used to store and exchange data in a structured format. JSON stands for JavaScript Object Notation, and it is a lightweight data interchange format that is easy for humans to read and write, and easy for machines to parse and generate. JSON files are commonly used in web applications to transfer data between the server and the client, and they are also used in many other programming languages and platforms. JSON files consist of key-value pairs, where each key is a string and each value can be a string, number, boolean, array, or another JSON object. The syntax of JSON is similar to that of JavaScript, but it is a separate language that can be used independently of JavaScript. JSON File is a tool that allows users to create, edit, and view JSON files. It provides a user-friendly interface for working with JSON data, and it can be used by developers, data analysts, and anyone else who needs to work with structured data. With JSON File, users can easily create and modify JSON files, and they can also validate the syntax of their JSON data to ensure that it is well-formed and error-free.
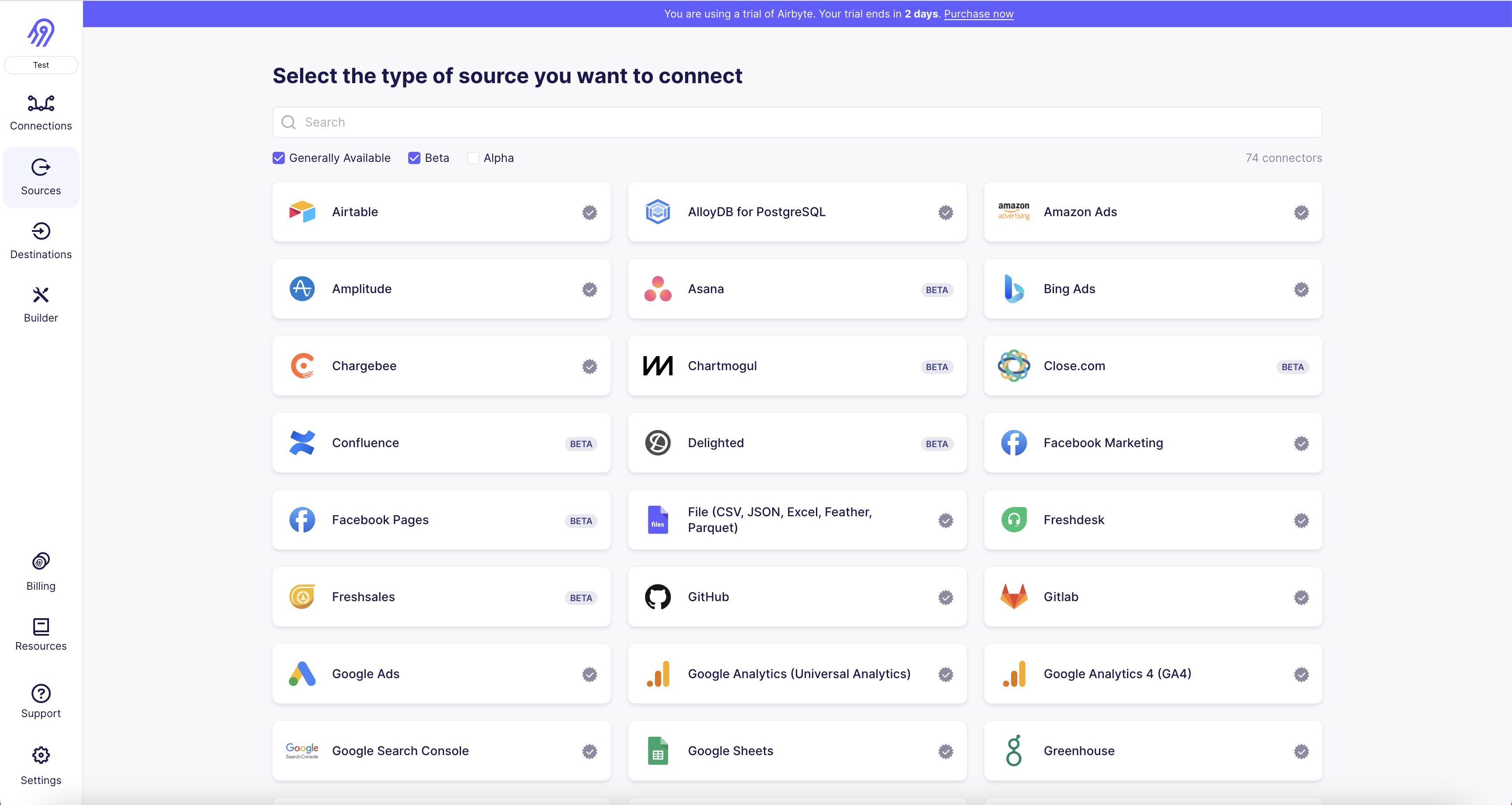
1. First, navigate to the Microsoft Teams source connector page on Airbyte.com.
2. Click on the "Add Source" button to begin the process of adding your Microsoft Teams credentials.
3. In the "Connection Configuration" section, enter a name for your connection and provide your Microsoft Teams credentials, including your email address and password.
4. Click on the "Test" button to ensure that your credentials are correct and that Airbyte can connect to your Microsoft Teams account.
5. Once the test is successful, click on the "Save & Continue" button to proceed to the next step.
6. In the "Schema Selection" section, select the specific data that you want to replicate from your Microsoft Teams account.
7. You can choose to replicate data from channels, messages, users, and more.
8. Once you have selected the data that you want to replicate, click on the "Save & Continue" button to proceed to the final step.
9. In the "Destination Configuration" section, select the destination where you want to send your replicated data.
10. You can choose from a variety of destinations, including databases, data warehouses, and cloud storage services.
11. Once you have selected your destination, click on the "Save & Test" button to ensure that your replication is successful.
12. Congratulations! You have successfully connected your Microsoft Teams source connector on Airbyte.com.

1. Open the Airbyte platform and navigate to the "Destinations" tab on the left-hand side of the screen.
2. Scroll down until you find the "JSON File" destination connector and click on it.
3. Click on the "Create new connection" button.
4. Enter a name for your connection and click on the "Next" button.
5. Fill in the required fields for your JSON File destination, such as the file path and format.
6. Test the connection by clicking on the "Test" button.
7. If the test is successful, click on the "Save & Sync" button to save your connection and start syncing data to your JSON File destination.
8. You can also schedule your syncs by clicking on the "Schedule" button and selecting the frequency and time for your syncs.
9. To view your synced data, navigate to the file path you specified in your JSON File destination and open the file in a text editor or JSON viewer.

With Airbyte, creating data pipelines take minutes, and the data integration possibilities are endless. Airbyte supports the largest catalog of API tools, databases, and files, among other sources. Airbyte's connectors are open-source, so you can add any custom objects to the connector, or even build a new connector from scratch without any local dev environment or any data engineer within 10 minutes with the no-code connector builder.
We look forward to seeing you make use of it! We invite you to join the conversation on our community Slack Channel, or sign up for our newsletter. You should also check out other Airbyte tutorials, and Airbyte’s content hub!
What should you do next?
Hope you enjoyed the reading. Here are the 3 ways we can help you in your data journey:
What should you do next?
Hope you enjoyed the reading. Here are the 3 ways we can help you in your data journey:
Ready to get started?
Frequently Asked Questions
Microsoft Teams API provides access to a wide range of data that can be used to enhance the functionality of the platform. The following are the categories of data that can be accessed through the API:
1. Teams and Channels: Information about the teams and channels in which the user is a member, including their names, descriptions, and membership details.
2. Messages and Conversations: Access to messages and conversations within a channel, including the content of the messages, the sender and recipient details, and the time and date of the messages.
3. Files and Documents: Access to files and documents shared within a channel, including their names, sizes, and types.
4. Meetings and Calls: Information about scheduled meetings and calls, including the time, date, and participants.
5. Users and Groups: Information about users and groups within the organization, including their names, email addresses, and roles.
6. Apps and Bots: Access to third-party apps and bots integrated with Microsoft Teams, including their names, descriptions, and functionality.
7. Settings and Configuration: Access to the settings and configuration options for Microsoft Teams, including user preferences, notification settings, and security settings.
Overall, the Microsoft Teams API provides a comprehensive set of data that can be used to build custom applications and integrations that enhance the functionality of the platform.
What should you do next?
Hope you enjoyed the reading. Here are the 3 ways we can help you in your data journey: