Amazon S3 (Simple Storage Service) is a cloud-based object storage service that provides developers and IT teams with secure, durable, and scalable storage for their data. It allows users to store and retrieve any amount of data from anywhere on the web, making it easy to build and scale applications, backup and archive data, and analyze data. S3 is designed to provide high availability and durability, with data automatically replicated across multiple availability zones within a region. It also offers a range of features such as versioning, lifecycle policies, and access control to help users manage their data effectively.
Elasticsearch is a powerful search and analytics engine that is designed to handle large amounts of data in real-time. It is an open-source, distributed, and scalable search engine that is built on top of the Apache Lucene search library. Elasticsearch is used to search, analyze, and visualize data in real-time, making it an ideal tool for businesses and organizations that need to process large amounts of data quickly. Elasticsearch is designed to be highly scalable and can be used to index and search data across multiple servers. It is also highly customizable, allowing users to configure it to meet their specific needs. Elasticsearch is commonly used for log analysis, full-text search, and business analytics. One of the key features of Elasticsearch is its ability to handle unstructured data, such as text, images, and videos. It uses a powerful search algorithm to analyze and index this data, making it easy to search and retrieve information quickly. Elasticsearch also supports a wide range of data formats, including JSON, CSV, and XML, making it easy to integrate with other data sources. Overall, Elasticsearch is a powerful tool that can help businesses and organizations to process and analyze large amounts of data quickly and efficiently.
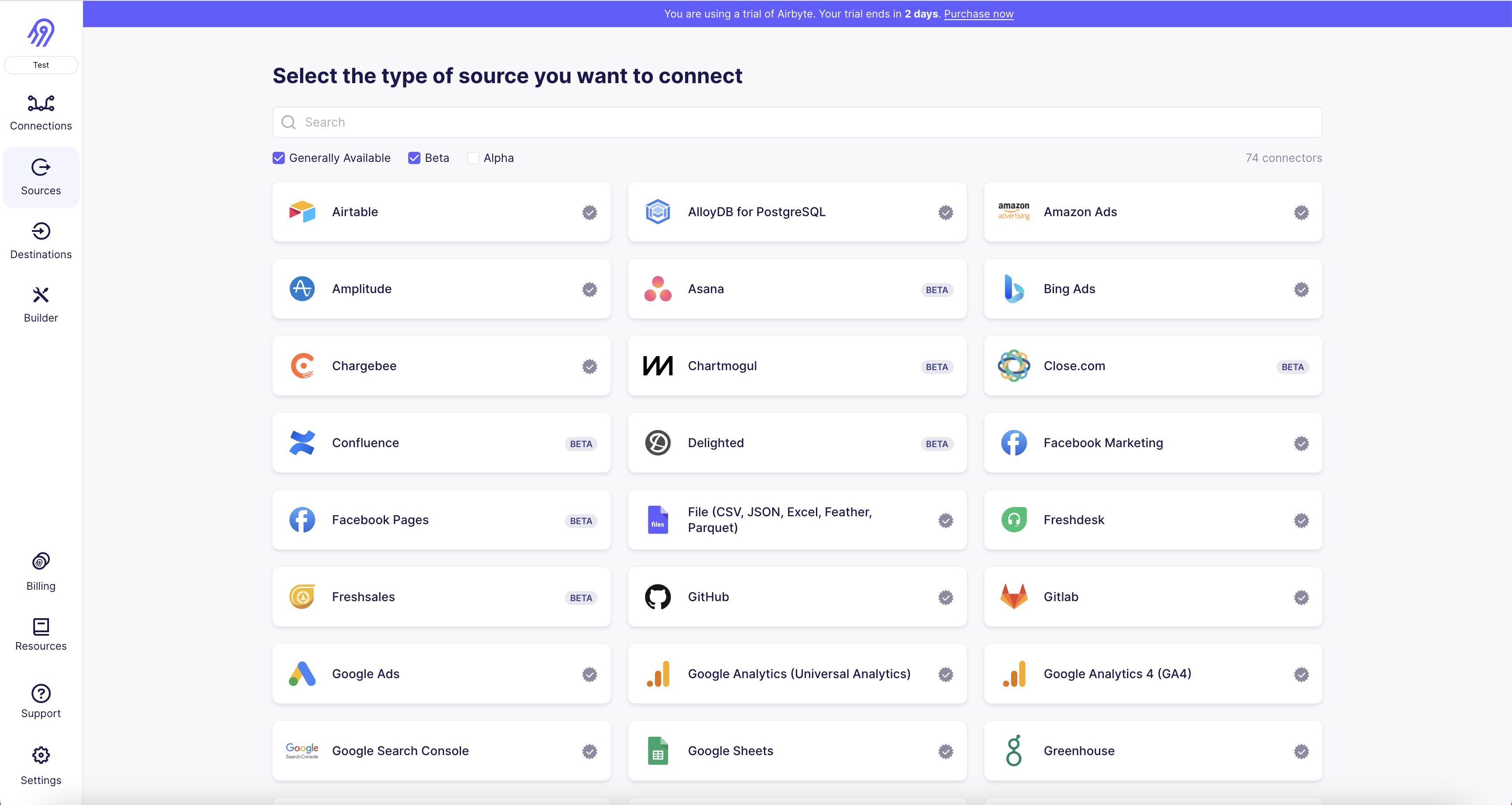
1. Open the Airbyte dashboard and click on "Sources" from the left-hand menu.
2. Click on the "Create Source" button and select "S3" from the list of available connectors.
3. Enter a name for your S3 source and click on "Next".
4. Enter your AWS access key ID and secret access key in the respective fields. You can find these credentials in your AWS account under "Security Credentials".
5. Select the AWS region where your S3 bucket is located from the dropdown menu.
6. Enter the name of your S3 bucket in the "Bucket Name" field.
7. If your S3 bucket is not in the root directory, enter the path to the directory in the "Path Prefix" field.
8. If you want to include only certain files in your data sync, you can enter a file pattern in the "File Pattern" field. For example, "*.csv" will only include CSV files.
9. Click on "Test" to verify your credentials and connection to the S3 bucket.
10. If the test is successful, click on "Create Source" to save your S3 source connector.Once your S3 source connector is set up, you can use it to create a new Airbyte pipeline and sync data from your S3 bucket to your destination of choice.

1. First, navigate to the Airbyte website and log in to your account.
2. Once you are logged in, click on the "Destinations" tab on the left-hand side of the screen.
3. Scroll down until you find the Elasticsearch destination connector and click on it.
4. You will be prompted to enter your Elasticsearch connection details, including the host URL, port number, and any authentication credentials.
5. Once you have entered your connection details, click on the "Test" button to ensure that your connection is working properly.
6. If the test is successful, click on the "Save" button to save your Elasticsearch destination connector settings.
7. You can now use this connector to send data from your Airbyte sources to your Elasticsearch database.
8. To set up a pipeline, navigate to the "Sources" tab and select the source you want to use.
9. Click on the "Create New Connection" button and select your Elasticsearch destination connector from the list.
10. Follow the prompts to map your source data to your Elasticsearch database fields and save your pipeline.

With Airbyte, creating data pipelines take minutes, and the data integration possibilities are endless. Airbyte supports the largest catalog of API tools, databases, and files, among other sources. Airbyte's connectors are open-source, so you can add any custom objects to the connector, or even build a new connector from scratch without any local dev environment or any data engineer within 10 minutes with the no-code connector builder.
We look forward to seeing you make use of it! We invite you to join the conversation on our community Slack Channel, or sign up for our newsletter. You should also check out other Airbyte tutorials, and Airbyte’s content hub!
What should you do next?
Hope you enjoyed the reading. Here are the 3 ways we can help you in your data journey:
What should you do next?
Hope you enjoyed the reading. Here are the 3 ways we can help you in your data journey:
Ready to get started?
Frequently Asked Questions
Amazon S3's API provides access to a wide range of data types, including:
1. Object data: This includes the actual files stored in S3 buckets, such as images, videos, documents, and other types of files.
2. Metadata: S3 stores metadata about each object, including information such as the object's size, creation date, and last modified date.
3. Access control data: S3 provides access control mechanisms to restrict access to objects in a bucket. The API provides access to information about access control policies and permissions.
4. Bucket data: S3 buckets are containers for objects. The API provides access to information about buckets, such as their names, creation dates, and region.
5. Logging data: S3 can log access requests to objects in a bucket. The API provides access to these logs, which can be used for auditing and compliance purposes.
6. Inventory data: S3 can generate inventory reports that provide information about the objects stored in a bucket. The API provides access to these reports.
7. Metrics data: S3 can generate metrics about the usage of a bucket, such as the number of requests and the amount of data transferred. The API provides access to these metrics.