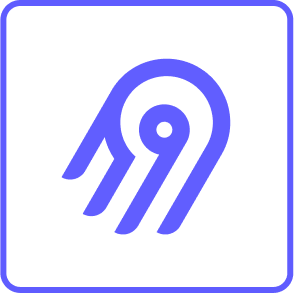

.webp)
Building your pipeline or Using Airbyte
Airbyte is the only open source solution empowering data teams to meet all their growing custom business demands in the new AI era.
- Inconsistent and inaccurate data
- Laborious and expensive
- Brittle and inflexible
- Reliable and accurate
- Extensible and scalable for all your needs
- Deployed and governed your way
Start syncing with Airbyte in 3 easy steps within 10 minutes



Take a virtual tour
Demo video of Airbyte Cloud
Demo video of AI Connector Builder
What sets Airbyte Apart
Modern GenAI Workflows
Move Large Volumes, Fast
An Extensible Open-Source Standard
Full Control & Security
Fully Featured & Integrated
Enterprise Support with SLAs
What our users say
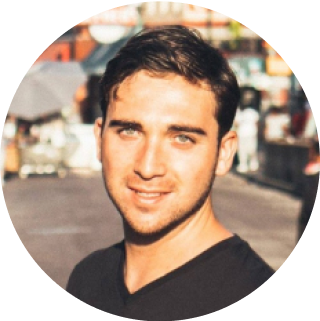
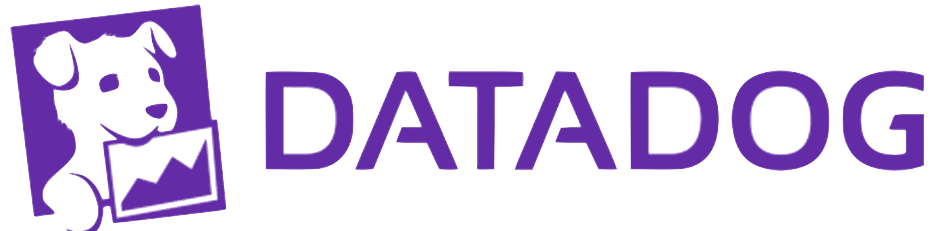
"The intake layer of Datadog’s self-serve analytics platform is largely built on Airbyte.Airbyte’s ease of use and extensibility allowed any team in the company to push their data into the platform - without assistance from the data team!"
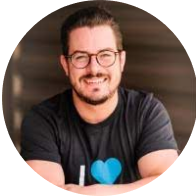
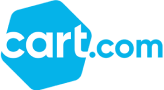
“Airbyte helped us accelerate our progress by years, compared to our competitors. We don’t need to worry about connectors and focus on creating value for our users instead of building infrastructure. That’s priceless. The time and energy saved allows us to disrupt and grow faster.”
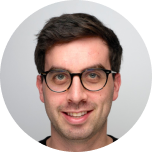

“We chose Airbyte for its ease of use, its pricing scalability and its absence of vendor lock-in. Having a lean team makes them our top criteria. The value of being able to scale and execute at a high level by maximizing resources is immense”
FAQs
What is ETL?
ETL, an acronym for Extract, Transform, Load, is a vital data integration process. It involves extracting data from diverse sources, transforming it into a usable format, and loading it into a database, data warehouse or data lake. This process enables meaningful data analysis, enhancing business intelligence.
Slack is an enterprise software platform that facilitates global communication between all sizes of businesses and teams. Slack enables collaborative work to be more efficient and more productive, making it possible for businesses to connect with immediacy from half a world apart. It allows teams to work together in concert, almost as if they were in the same room. Slack transforms the process of communication, bringing it into the 21st century with powerful style.
Slack's API provides access to a wide range of data, including:
1. Conversations: This includes information about channels, direct messages, and group messages.
2. Users: This includes information about individual users, such as their name, email address, and profile picture.
3. Files: This includes information about files uploaded to Slack, such as their name, size, and type.
4. Apps: This includes information about the apps installed in Slack, such as their name, description, and permissions.
5. Messages: This includes information about individual messages, such as their text, timestamp, and author.
6. Events: This includes information about events that occur in Slack, such as when a user joins or leaves a channel.
7. Workflows: This includes information about workflows created in Slack, such as their name, description, and status.
8. Analytics: This includes information about how users are interacting with Slack, such as the number of messages sent and received, and the most active channels.
What is ELT?
ELT, standing for Extract, Load, Transform, is a modern take on the traditional ETL data integration process. In ELT, data is first extracted from various sources, loaded directly into a data warehouse, and then transformed. This approach enhances data processing speed, analytical flexibility and autonomy.
Difference between ETL and ELT?
ETL and ELT are critical data integration strategies with key differences. ETL (Extract, Transform, Load) transforms data before loading, ideal for structured data. In contrast, ELT (Extract, Load, Transform) loads data before transformation, perfect for processing large, diverse data sets in modern data warehouses. ELT is becoming the new standard as it offers a lot more flexibility and autonomy to data analysts.
What should you do next?
Hope you enjoyed the reading. Here are the 3 ways we can help you in your data journey:
Slack is an enterprise software platform that facilitates global communication between all sizes of businesses and teams. Slack enables collaborative work to be more efficient and more productive, making it possible for businesses to connect with immediacy from half a world apart. It allows teams to work together in concert, almost as if they were in the same room. Slack transforms the process of communication, bringing it into the 21st century with powerful style.
CSV (Comma Separated Values) file is a tool used to store and exchange data in a simple and structured format. It is a plain text file that contains data separated by commas, where each line represents a record and each field is separated by a comma. CSV files are widely used in data analysis, data migration, and data exchange between different software applications. The CSV file format is easy to read and write, making it a popular choice for storing and exchanging data. It can be opened and edited using any text editor or spreadsheet software, such as Microsoft Excel or Google Sheets. CSV files can also be imported and exported from databases, making it a convenient tool for data management. CSV files are commonly used for storing large amounts of data, such as customer information, product catalogs, financial data, and scientific data. They are also used for data analysis and visualization, as they can be easily imported into statistical software and other data analysis tools. Overall, the CSV file is a simple and versatile tool that is widely used for storing, exchanging, and analyzing data.
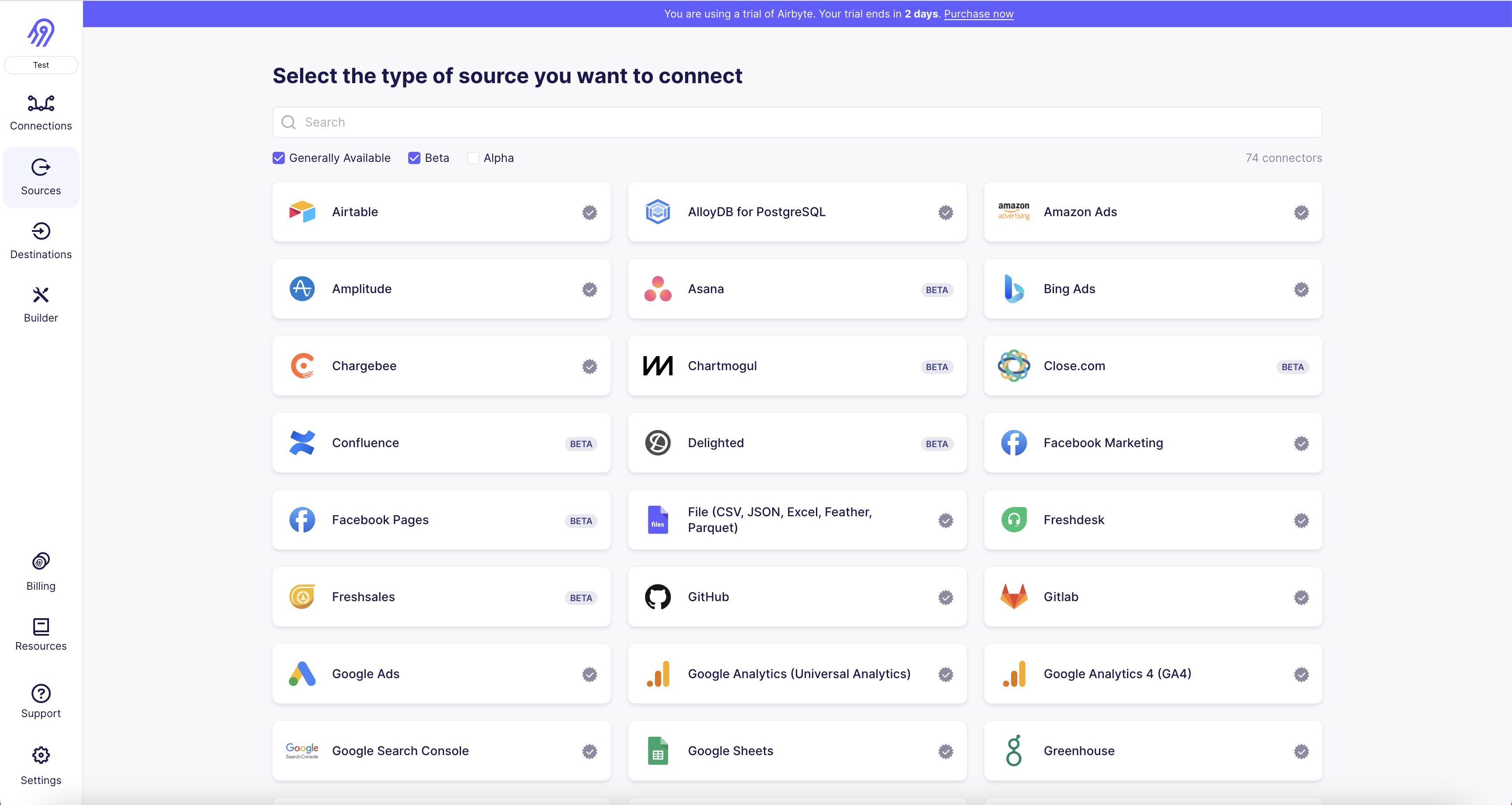
1. First, navigate to the Slack source connector page on Airbyte.com.
2. Click on the "Add Source" button to begin the process of adding your Slack credentials.
3. In the "Connection Configuration" section, enter a name for your Slack connection.
4. Next, enter your Slack workspace's API token in the "API Token" field. You can generate an API token by following the instructions in the Airbyte documentation.
5. In the "Channels" field, enter the names of the Slack channels you want to sync data from. You can enter multiple channels by separating them with commas.
6. If you want to filter the data that is synced from Slack, you can enter a date range in the "Start Date" and "End Date" fields.
7. Once you have entered all the necessary information, click on the "Test" button to ensure that your credentials are valid and that Airbyte can connect to your Slack workspace.
8. If the test is successful, click on the "Save & Continue" button to save your Slack connection.
9. You can now use your Slack source connector to sync data from your Slack workspace to your destination of choice.

1. Open the Airbyte platform and navigate to the "Destinations" tab on the left-hand side of the screen.
2. Click on the "CSV File" destination connector.
3. Click on the "Create new connection" button.
4. Enter a name for your connection and select the workspace you want to use.
5. Enter the path where you want to save your CSV file.
6. Choose the delimiter you want to use for your CSV file.
7. Select the encoding you want to use for your CSV file.
8. Choose whether you want to append data to an existing file or create a new file each time the connector runs.
9. Enter any additional configuration settings you want to use for your CSV file.
10. Click on the "Test" button to ensure that your connection is working properly.
11. If the test is successful, click on the "Create" button to save your connection.
12. Your CSV File destination connector is now connected and ready to use.

With Airbyte, creating data pipelines take minutes, and the data integration possibilities are endless. Airbyte supports the largest catalog of API tools, databases, and files, among other sources. Airbyte's connectors are open-source, so you can add any custom objects to the connector, or even build a new connector from scratch without any local dev environment or any data engineer within 10 minutes with the no-code connector builder.
We look forward to seeing you make use of it! We invite you to join the conversation on our community Slack Channel, or sign up for our newsletter. You should also check out other Airbyte tutorials, and Airbyte’s content hub!
What should you do next?
Hope you enjoyed the reading. Here are the 3 ways we can help you in your data journey:
What should you do next?
Hope you enjoyed the reading. Here are the 3 ways we can help you in your data journey:
Ready to get started?
Frequently Asked Questions
Slack's API provides access to a wide range of data, including:
1. Conversations: This includes information about channels, direct messages, and group messages.
2. Users: This includes information about individual users, such as their name, email address, and profile picture.
3. Files: This includes information about files uploaded to Slack, such as their name, size, and type.
4. Apps: This includes information about the apps installed in Slack, such as their name, description, and permissions.
5. Messages: This includes information about individual messages, such as their text, timestamp, and author.
6. Events: This includes information about events that occur in Slack, such as when a user joins or leaves a channel.
7. Workflows: This includes information about workflows created in Slack, such as their name, description, and status.
8. Analytics: This includes information about how users are interacting with Slack, such as the number of messages sent and received, and the most active channels.