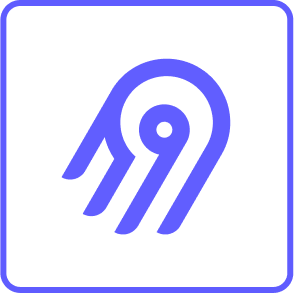

Building your pipeline or Using Airbyte
Airbyte is the only open source solution empowering data teams to meet all their growing custom business demands in the new AI era.
- Inconsistent and inaccurate data
- Laborious and expensive
- Brittle and inflexible
- Reliable and accurate
- Extensible and scalable for all your needs
- Deployed and governed your way
Start syncing with Airbyte in 3 easy steps within 10 minutes



Take a virtual tour
Demo video of Airbyte Cloud
Demo video of AI Connector Builder
What sets Airbyte Apart
Modern GenAI Workflows
Move Large Volumes, Fast
An Extensible Open-Source Standard
Full Control & Security
Fully Featured & Integrated
Enterprise Support with SLAs
What our users say
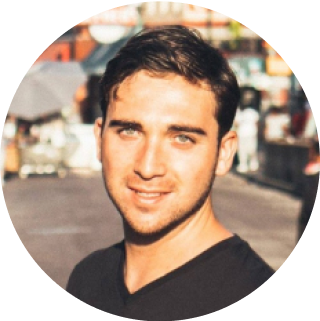
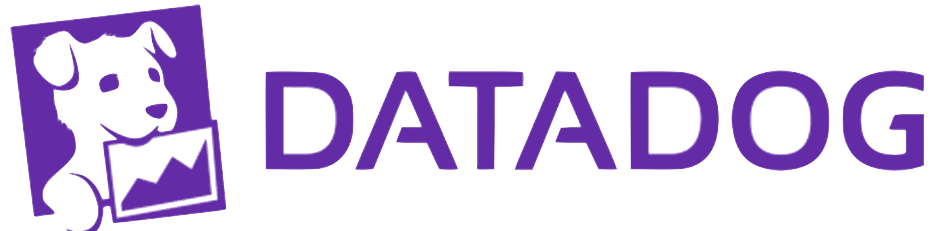
"The intake layer of Datadog’s self-serve analytics platform is largely built on Airbyte.Airbyte’s ease of use and extensibility allowed any team in the company to push their data into the platform - without assistance from the data team!"
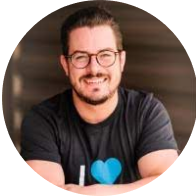
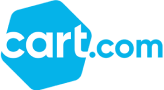
“Airbyte helped us accelerate our progress by years, compared to our competitors. We don’t need to worry about connectors and focus on creating value for our users instead of building infrastructure. That’s priceless. The time and energy saved allows us to disrupt and grow faster.”
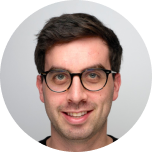

“We chose Airbyte for its ease of use, its pricing scalability and its absence of vendor lock-in. Having a lean team makes them our top criteria. The value of being able to scale and execute at a high level by maximizing resources is immense”
- Log in to Snowflake: Access your Snowflake account using the web interface or a Snowflake client.
- Select the Data: Identify the data you want to move to Google Sheets. You may want to create a specific SELECT query to filter and format your data as needed.
- Export Query Results: Execute the query and export the results. This can be done in several ways:
- Using the Web Interface: Run your query and use the option to download the results directly from the interface in a CSV format.
- Using SnowSQL (CLI Client): If you prefer using the command line, use SnowSQL to run your query and use the COPY INTO <location> command to export the results to a file.
- Open the CSV File: Use a text editor or a spreadsheet program like Microsoft Excel to open the exported CSV file.
- Check the Formatting: Ensure that the data is correctly formatted and that there are no issues with delimiters, text qualifiers, or special characters.
- Save the File: If you made any changes, save the file with the appropriate CSV settings.
- Open Google Sheets: Go to Google Sheets and sign in with your Google account.
- Create a New Spreadsheet: Click on the “+” button or “Blank” to create a new spreadsheet.
- Import the CSV File:
- Go to the File menu > Import.
- Choose the “Upload” tab and drag your CSV file into the space provided or browse to upload it.
- Select the import options you prefer (e.g., replace the current sheet, create a new sheet, etc.).
- Click on the “Import Data” button.
- Check the Imported Data: Once the data is in Google Sheets, check it to ensure that it has been imported correctly.
- Adjust Formatting: Google Sheets may not always preserve the formatting from the CSV, so you may need to adjust text formats, date formats, and column widths.
If you need to perform this operation regularly, you can create a script using Google Apps Script to automate the import process:
- Open Google Apps Script: In Google Sheets, go to Extensions > Apps Script.
- Write a Script: Create a script that fetches the CSV file from a specific location (like Google Drive) and imports it into your Google Sheet.
- Trigger the Script: Set up a time-driven trigger to run your script at regular intervals.
Tips and Considerations
- Always verify that your data does not contain sensitive information before importing it into Google Sheets, as Google Sheets is a cloud service and the data will be stored online.
- If you're dealing with large datasets, be aware that Google Sheets has a limit on the number of cells you can have in a single spreadsheet.
- Remember that manual processes like this can be error-prone and time-consuming. If you find yourself needing to do this frequently, it may be worth investing in a third-party connector like Airbyte.
This guide provides a manual method to move data from Snowflake to Google Sheets without third-party connectors. If you're comfortable with coding, you could also write scripts to partially automate the process, such as using Python with Snowflake's Python connector and Google Sheets API. However, this would require additional steps and setup.
FAQs
What is ETL?
ETL, an acronym for Extract, Transform, Load, is a vital data integration process. It involves extracting data from diverse sources, transforming it into a usable format, and loading it into a database, data warehouse or data lake. This process enables meaningful data analysis, enhancing business intelligence.
Snowflake Data Cloud is a cloud-based data warehousing and analytics platform that allows organizations to store, manage, and analyze large amounts of data in a secure and scalable manner. It provides a single, integrated platform for data storage, processing, and analysis, eliminating the need for multiple tools and systems. Snowflake Data Cloud is built on a unique architecture that separates compute and storage, allowing users to scale up or down as needed without affecting performance. It also offers a range of features such as data sharing, data governance, and machine learning capabilities, making it a comprehensive solution for modern data management and analytics.
Snowflake Data Cloud provides access to a wide range of data types, including:
1. Structured Data: This includes data that is organized in a specific format, such as tables, columns, and rows. Examples of structured data include customer information, financial data, and inventory records.
2. Semi-Structured Data: This type of data is partially organized and may not fit into a traditional relational database structure. Examples of semi-structured data include JSON, XML, and CSV files.
3. Unstructured Data: This includes data that does not have a specific format or organization, such as text documents, images, and videos.
4. Time-Series Data: This type of data is organized based on time stamps and is commonly used in industries such as finance, healthcare, and manufacturing.
5. Geospatial Data: This includes data that is related to geographic locations, such as maps, GPS coordinates, and satellite imagery.
6. Machine Learning Data: This type of data is used to train machine learning models and includes features and labels that are used to predict outcomes.
Overall, Snowflake Data Cloud provides access to a wide range of data types, making it a versatile tool for data analysis and management.
What is ELT?
ELT, standing for Extract, Load, Transform, is a modern take on the traditional ETL data integration process. In ELT, data is first extracted from various sources, loaded directly into a data warehouse, and then transformed. This approach enhances data processing speed, analytical flexibility and autonomy.
Difference between ETL and ELT?
ETL and ELT are critical data integration strategies with key differences. ETL (Extract, Transform, Load) transforms data before loading, ideal for structured data. In contrast, ELT (Extract, Load, Transform) loads data before transformation, perfect for processing large, diverse data sets in modern data warehouses. ELT is becoming the new standard as it offers a lot more flexibility and autonomy to data analysts.
What should you do next?
Hope you enjoyed the reading. Here are the 3 ways we can help you in your data journey:
Snowflake Data Cloud is a cloud-based data warehousing and analytics platform that allows organizations to store, manage, and analyze large amounts of data in a secure and scalable manner. It provides a single, integrated platform for data storage, processing, and analysis, eliminating the need for multiple tools and systems. Snowflake Data Cloud is built on a unique architecture that separates compute and storage, allowing users to scale up or down as needed without affecting performance. It also offers a range of features such as data sharing, data governance, and machine learning capabilities, making it a comprehensive solution for modern data management and analytics.
Google Sheets is a cloud-based spreadsheet tool that allows users to create, edit, and share spreadsheets online. It is a part of the Google Drive suite of productivity tools and is accessible from any device with an internet connection. Google Sheets offers a range of features that make it a powerful tool for data analysis, project management, and collaboration. Users can create and format spreadsheets, add formulas and functions, and create charts and graphs to visualize data. Google Sheets also allows users to collaborate in real-time, making it easy to work on projects with others. Users can share spreadsheets with specific people or make them public, and can control who has access to edit or view the document. Additionally, Google Sheets integrates with other Google tools such as Google Forms, allowing users to collect data and automatically populate it into a spreadsheet. Overall, Google Sheets is a versatile and user-friendly tool that can be used for a variety of tasks, from simple calculations to complex data analysis.
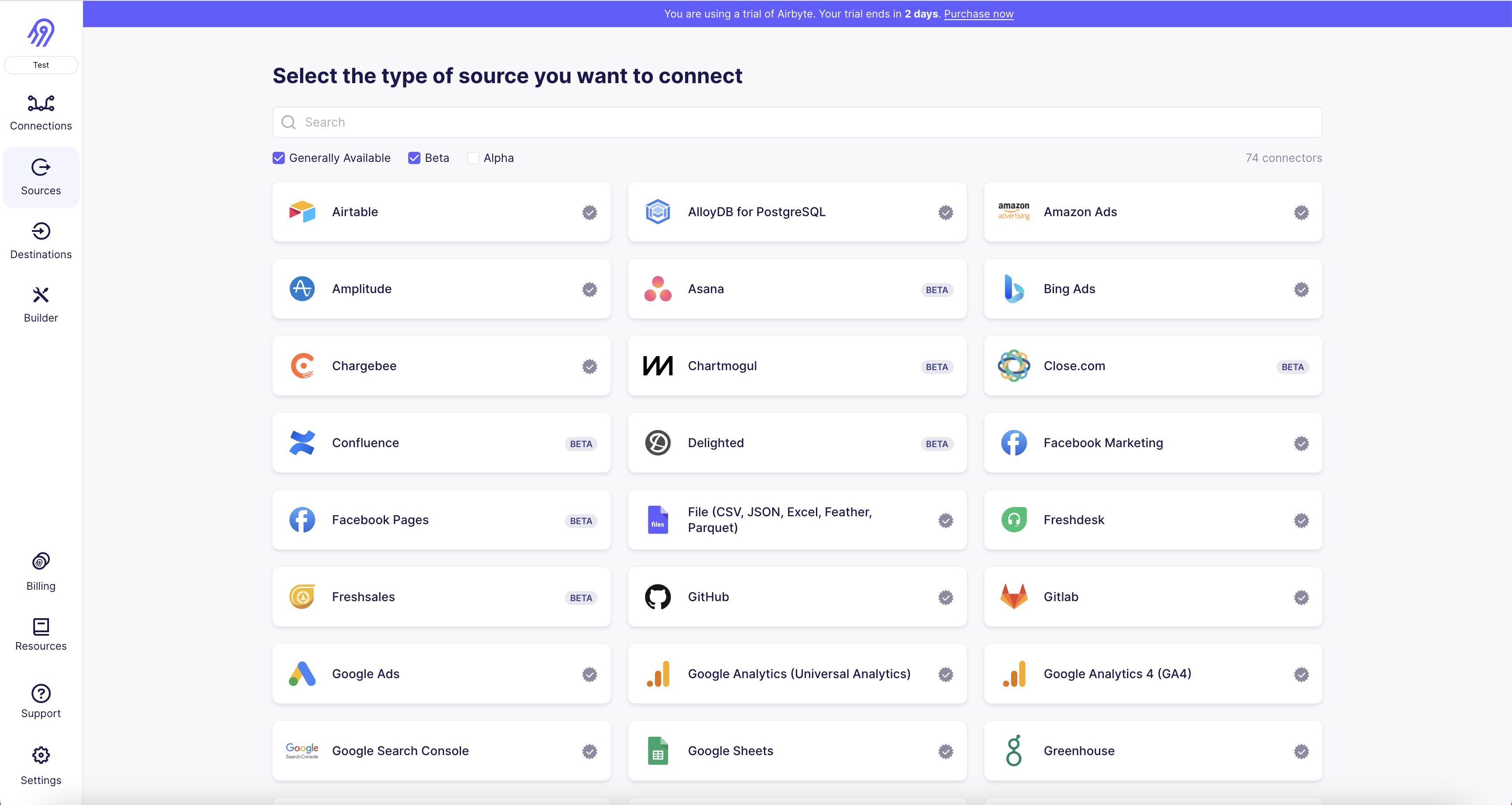
1. First, you need to have a Snowflake Data Cloud account and the necessary credentials to access it.
2. Once you have the credentials, go to the Airbyte dashboard and click on "Sources" on the left-hand side of the screen.
3. Click on the "Create a new source" button and select "Snowflake Data Cloud" from the list of available sources.
4. Enter a name for your Snowflake Data Cloud source and click on "Next".
5. In the "Connection" tab, enter the following information:
- Account name: the name of your Snowflake account
- Username: your Snowflake username
- Password: your Snowflake password
- Warehouse: the name of the warehouse you want to use
- Database: the name of the database you want to use
- Schema: the name of the schema you want to use
6. Click on "Test connection" to make sure that the connection is successful.
7. If the connection is successful, click on "Next" to proceed to the "Configuration" tab.
8. In the "Configuration" tab, select the tables or views that you want to replicate and configure any necessary settings.
9. Click on "Create source" to save your Snowflake Data Cloud source and start replicating data.

1. Go to the Airbyte website and log in to your account.
2. Click on the "Destinations" tab on the left-hand side of the screen.
3. Scroll down until you find the "Google Sheets" destination connector and click on it.
4. Click on the "Create Destination" button.
5. Enter a name for your destination and click on the "Create" button.
6. You will be redirected to the Google Sheets authorization page. Sign in to your Google account if you haven't already.
7. Click on the "Allow" button to grant Airbyte access to your Google Sheets account.
8. You will be redirected back to the Airbyte website. Select the Google Sheets destination you just created from the list of destinations.
9. Enter the name of the spreadsheet you want to use as your destination and select the worksheet you want to use.
10. Click on the "Test" button to make sure the connection is working properly.
11. If the test is successful, click on the "Save" button to save your destination settings.
12. You can now use the Google Sheets destination connector to transfer data from your source to your Google Sheets destination.

With Airbyte, creating data pipelines take minutes, and the data integration possibilities are endless. Airbyte supports the largest catalog of API tools, databases, and files, among other sources. Airbyte's connectors are open-source, so you can add any custom objects to the connector, or even build a new connector from scratch without any local dev environment or any data engineer within 10 minutes with the no-code connector builder.
We look forward to seeing you make use of it! We invite you to join the conversation on our community Slack Channel, or sign up for our newsletter. You should also check out other Airbyte tutorials, and Airbyte’s content hub!
What should you do next?
Hope you enjoyed the reading. Here are the 3 ways we can help you in your data journey:
TL;DR
This can be done by building a data pipeline manually, usually a Python script (you can leverage a tool as Apache Airflow for this). This process can take more than a full week of development. Or it can be done in minutes on Airbyte in three easy steps:
- set up Snowflake as a source connector (using Auth, or usually an API key)
- set up Google Sheets as a destination connector
- define which data you want to transfer and how frequently
You can choose to self-host the pipeline using Airbyte Open Source or have it managed for you with Airbyte Cloud.
This tutorial’s purpose is to show you how.
What is Snowflake
Snowflake Data Cloud is a cloud-based data warehousing and analytics platform that allows organizations to store, manage, and analyze large amounts of data in a secure and scalable manner. It provides a single, integrated platform for data storage, processing, and analysis, eliminating the need for multiple tools and systems. Snowflake Data Cloud is built on a unique architecture that separates compute and storage, allowing users to scale up or down as needed without affecting performance. It also offers a range of features such as data sharing, data governance, and machine learning capabilities, making it a comprehensive solution for modern data management and analytics.
What is Google Sheets
Google Sheets is a cloud-based spreadsheet tool that allows users to create, edit, and share spreadsheets online. It is a part of the Google Drive suite of productivity tools and is accessible from any device with an internet connection. Google Sheets offers a range of features that make it a powerful tool for data analysis, project management, and collaboration. Users can create and format spreadsheets, add formulas and functions, and create charts and graphs to visualize data. Google Sheets also allows users to collaborate in real-time, making it easy to work on projects with others. Users can share spreadsheets with specific people or make them public, and can control who has access to edit or view the document. Additionally, Google Sheets integrates with other Google tools such as Google Forms, allowing users to collect data and automatically populate it into a spreadsheet. Overall, Google Sheets is a versatile and user-friendly tool that can be used for a variety of tasks, from simple calculations to complex data analysis.
{{COMPONENT_CTA}}
Prerequisites
- A Snowflake account to transfer your customer data automatically from.
- A Google Sheets account.
- An active Airbyte Cloud account, or you can also choose to use Airbyte Open Source locally. You can follow the instructions to set up Airbyte on your system using docker-compose.
Airbyte is an open-source data integration platform that consolidates and streamlines the process of extracting and loading data from multiple data sources to data warehouses. It offers pre-built connectors, including Snowflake and Google Sheets, for seamless data migration.
When using Airbyte to move data from Snowflake to Google Sheets, it extracts data from Snowflake using the source connector, converts it into a format Google Sheets can ingest using the provided schema, and then loads it into Google Sheets via the destination connector. This allows businesses to leverage their Snowflake data for advanced analytics and insights within Google Sheets, simplifying the ETL process and saving significant time and resources.
Methods to Move Data From Snowflake to Google sheets
- Method 1: Connecting Snowflake to Google sheets using Airbyte.
- Method 2: Connecting Snowflake to Google sheets manually.
Method 1: Connecting Snowflake to Google sheets using Airbyte.
Airbyte is an open-source data integration platform that consolidates and streamlines the process of extracting and loading data from multiple data sources to data warehouses. It offers pre-built connectors, including Snowflake and Google Sheets, for seamless data migration.
When using Airbyte to move data from Snowflake to Google Sheets, it extracts data from Snowflake using the source connector, converts it into a format Google Sheets can ingest using the provided schema, and then loads it into Google Sheets via the destination connector. This allows businesses to leverage their Snowflake data for advanced analytics and insights within Google Sheets, simplifying the ETL process and saving significant time and resources.
Step 1: Set up Snowflake as a source connector
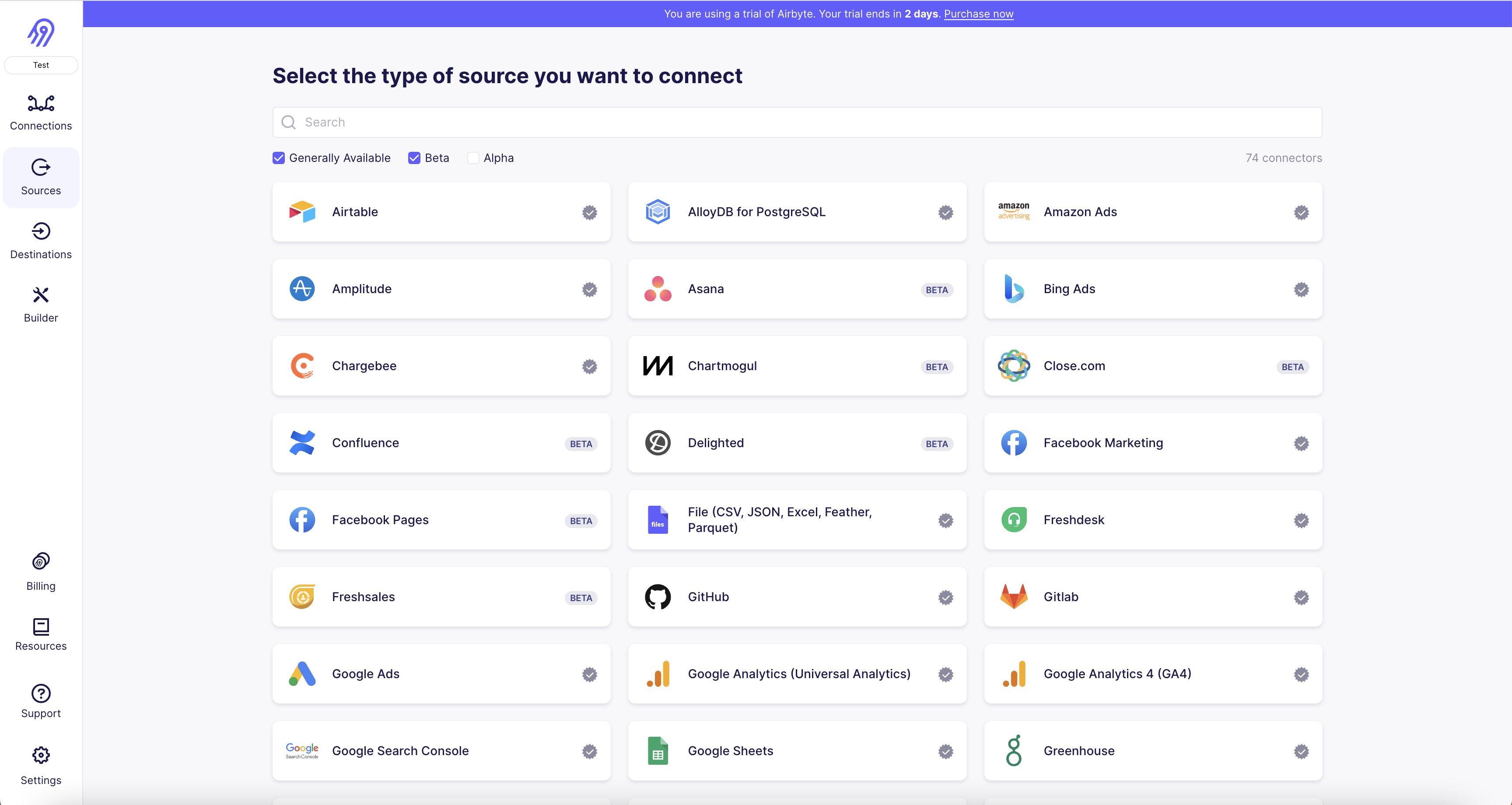
1. First, you need to have a Snowflake Data Cloud account and the necessary credentials to access it.
2. Once you have the credentials, go to the Airbyte dashboard and click on "Sources" on the left-hand side of the screen.
3. Click on the "Create a new source" button and select "Snowflake Data Cloud" from the list of available sources.
4. Enter a name for your Snowflake Data Cloud source and click on "Next".
5. In the "Connection" tab, enter the following information:
- Account name: the name of your Snowflake account
- Username: your Snowflake username
- Password: your Snowflake password
- Warehouse: the name of the warehouse you want to use
- Database: the name of the database you want to use
- Schema: the name of the schema you want to use
6. Click on "Test connection" to make sure that the connection is successful.
7. If the connection is successful, click on "Next" to proceed to the "Configuration" tab.
8. In the "Configuration" tab, select the tables or views that you want to replicate and configure any necessary settings.
9. Click on "Create source" to save your Snowflake Data Cloud source and start replicating data.
Step 2: Set up Google Sheets as a destination connector

1. Go to the Airbyte website and log in to your account.
2. Click on the "Destinations" tab on the left-hand side of the screen.
3. Scroll down until you find the "Google Sheets" destination connector and click on it.
4. Click on the "Create Destination" button.
5. Enter a name for your destination and click on the "Create" button.
6. You will be redirected to the Google Sheets authorization page. Sign in to your Google account if you haven't already.
7. Click on the "Allow" button to grant Airbyte access to your Google Sheets account.
8. You will be redirected back to the Airbyte website. Select the Google Sheets destination you just created from the list of destinations.
9. Enter the name of the spreadsheet you want to use as your destination and select the worksheet you want to use.
10. Click on the "Test" button to make sure the connection is working properly.
11. If the test is successful, click on the "Save" button to save your destination settings.
12. You can now use the Google Sheets destination connector to transfer data from your source to your Google Sheets destination.
Step 3: Set up a connection to sync your Snowflake data to Google Sheets

Once you've successfully connected Snowflake as a data source and Google Sheets as a destination in Airbyte, you can set up a data pipeline between them with the following steps:
- Create a new connection: On the Airbyte dashboard, navigate to the 'Connections' tab and click the '+ New Connection' button.
- Choose your source: Select Snowflake from the dropdown list of your configured sources.
- Select your destination: Choose Google Sheets from the dropdown list of your configured destinations.
- Configure your sync: Define the frequency of your data syncs based on your business needs. Airbyte allows both manual and automatic scheduling for your data refreshes.
- Select the data to sync: Choose the specific Snowflake objects you want to import data from towards Google Sheets. You can sync all data or select specific tables and fields.
- Select the sync mode for your streams: Choose between full refreshes or incremental syncs (with deduplication if you want), and this for all streams or at the stream level. Incremental is only available for streams that have a primary cursor.
- Test your connection: Click the 'Test Connection' button to make sure that your setup works. If the connection test is successful, save your configuration.
- Start the sync: If the test passes, click 'Set Up Connection'. Airbyte will start moving data from Snowflake to Google Sheets according to your settings.
Remember, Airbyte keeps your data in sync at the frequency you determine, ensuring your Google Sheets data warehouse is always up-to-date with your Snowflake data.
Method 2: Connecting Snowflake to Google sheets manually.
Moving data from Snowflake to Google Sheets manually can be a bit more involved since you'll be handling the export and import processes manually.
Step 1: Prepare Your Snowflake Data for Export
- Log in to Snowflake: Access your Snowflake account using the web interface or a Snowflake client.
- Select the Data: Identify the data you want to move to Google Sheets. You may want to create a specific SELECT query to filter and format your data as needed.
- Export Query Results: Execute the query and export the results. This can be done in several ways:
- Using the Web Interface: Run your query and use the option to download the results directly from the interface in a CSV format.
- Using SnowSQL (CLI Client): If you prefer using the command line, use SnowSQL to run your query and use the COPY INTO <location> command to export the results to a file.
Step 2: Format the Data (Optional)
- Open the CSV File: Use a text editor or a spreadsheet program like Microsoft Excel to open the exported CSV file.
- Check the Formatting: Ensure that the data is correctly formatted and that there are no issues with delimiters, text qualifiers, or special characters.
- Save the File: If you made any changes, save the file with the appropriate CSV settings.
Step 3: Import Data into Google Sheets
- Open Google Sheets: Go to Google Sheets and sign in with your Google account.
- Create a New Spreadsheet: Click on the “+” button or “Blank” to create a new spreadsheet.
- Import the CSV File:
- Go to the File menu > Import.
- Choose the “Upload” tab and drag your CSV file into the space provided or browse to upload it.
- Select the import options you prefer (e.g., replace the current sheet, create a new sheet, etc.).
- Click on the “Import Data” button.
Step 4: Verify the Data
- Check the Imported Data: Once the data is in Google Sheets, check it to ensure that it has been imported correctly.
- Adjust Formatting: Google Sheets may not always preserve the formatting from the CSV, so you may need to adjust text formats, date formats, and column widths.
Step 5: Automate the Process (Optional)
If you need to perform this operation regularly, you can create a script using Google Apps Script to automate the import process:
- Open Google Apps Script: In Google Sheets, go to Extensions > Apps Script.
- Write a Script: Create a script that fetches the CSV file from a specific location (like Google Drive) and imports it into your Google Sheet.
- Trigger the Script: Set up a time-driven trigger to run your script at regular intervals.
Tips and Considerations
- Always verify that your data does not contain sensitive information before importing it into Google Sheets, as Google Sheets is a cloud service and the data will be stored online.
- If you're dealing with large datasets, be aware that Google Sheets has a limit on the number of cells you can have in a single spreadsheet.
- Remember that manual processes like this can be error-prone and time-consuming. If you find yourself needing to do this frequently, it may be worth investing in a third-party connector like Airbyte.
This guide provides a manual method to move data from Snowflake to Google Sheets without third-party connectors. If you're comfortable with coding, you could also write scripts to partially automate the process, such as using Python with Snowflake's Python connector and Google Sheets API. However, this would require additional steps and setup.
Use Cases to transfer your Snowflake data to Google Sheets
Integrating data from Snowflake to Google Sheets provides several benefits. Here are a few use cases:
- Advanced Analytics: Google Sheets’s powerful data processing capabilities enable you to perform complex queries and data analysis on your Snowflake data, extracting insights that wouldn't be possible within Snowflake alone.
- Data Consolidation: If you're using multiple other sources along with Snowflake, syncing to Google Sheets allows you to centralize your data for a holistic view of your operations, and to set up a change data capture process so you never have any discrepancies in your data again.
- Historical Data Analysis: Snowflake has limits on historical data. Syncing data to Google Sheets allows for long-term data retention and analysis of historical trends over time.
- Data Security and Compliance: Google Sheets provides robust data security features. Syncing Snowflake data to Google Sheets ensures your data is secured and allows for advanced data governance and compliance management.
- Scalability: Google Sheets can handle large volumes of data without affecting performance, providing an ideal solution for growing businesses with expanding Snowflake data.
- Data Science and Machine Learning: By having Snowflake data in Google Sheets, you can apply machine learning models to your data for predictive analytics, customer segmentation, and more.
- Reporting and Visualization: While Snowflake provides reporting tools, data visualization tools like Tableau, PowerBI, Looker (Google Data Studio) can connect to Google Sheets, providing more advanced business intelligence options. If you have a Snowflake table that needs to be converted to a Google Sheets table, Airbyte can do that automatically.
Wrapping Up
To summarize, this tutorial has shown you how to:
- Configure a Snowflake account as an Airbyte data source connector.
- Configure Google Sheets as a data destination connector.
- Create an Airbyte data pipeline that will automatically be moving data directly from Snowflake to Google Sheets after you set a schedule
With Airbyte, creating data pipelines take minutes, and the data integration possibilities are endless. Airbyte supports the largest catalog of API tools, databases, and files, among other sources. Airbyte's connectors are open-source, so you can add any custom objects to the connector, or even build a new connector from scratch without any local dev environment or any data engineer within 10 minutes with the no-code connector builder.
We look forward to seeing you make use of it! We invite you to join the conversation on our community Slack Channel, or sign up for our newsletter. You should also check out other Airbyte tutorials, and Airbyte’s content hub!
What should you do next?
Hope you enjoyed the reading. Here are the 3 ways we can help you in your data journey:
Ready to get started?
Frequently Asked Questions
Snowflake Data Cloud provides access to a wide range of data types, including:
1. Structured Data: This includes data that is organized in a specific format, such as tables, columns, and rows. Examples of structured data include customer information, financial data, and inventory records.
2. Semi-Structured Data: This type of data is partially organized and may not fit into a traditional relational database structure. Examples of semi-structured data include JSON, XML, and CSV files.
3. Unstructured Data: This includes data that does not have a specific format or organization, such as text documents, images, and videos.
4. Time-Series Data: This type of data is organized based on time stamps and is commonly used in industries such as finance, healthcare, and manufacturing.
5. Geospatial Data: This includes data that is related to geographic locations, such as maps, GPS coordinates, and satellite imagery.
6. Machine Learning Data: This type of data is used to train machine learning models and includes features and labels that are used to predict outcomes.
Overall, Snowflake Data Cloud provides access to a wide range of data types, making it a versatile tool for data analysis and management.