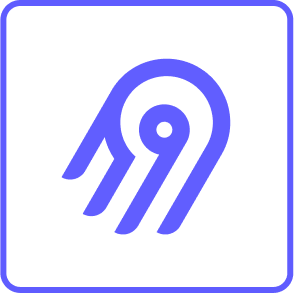

Building your pipeline or Using Airbyte
Airbyte is the only open solution empowering data teams to meet all their growing custom business demands in the new AI era.
- Inconsistent and inaccurate data
- Laborious and expensive
- Brittle and inflexible
- Reliable and accurate
- Extensible and scalable for all your needs
- Deployed and governed your way
Start syncing with Airbyte in 3 easy steps within 10 minutes



Take a virtual tour
Demo video of Airbyte Cloud
Demo video of AI Connector Builder
What sets Airbyte Apart
Modern GenAI Workflows
Move Large Volumes, Fast
An Extensible Open-Source Standard
Full Control & Security
Fully Featured & Integrated
Enterprise Support with SLAs
What our users say
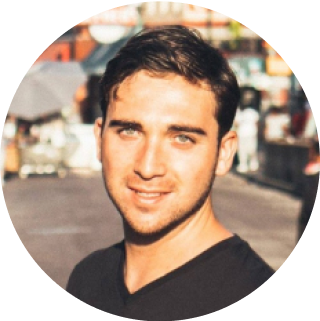
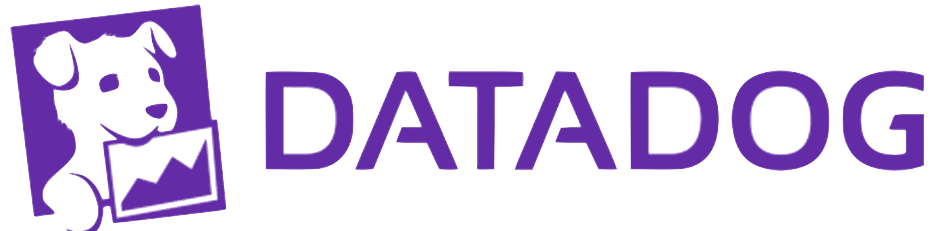
"The intake layer of Datadog’s self-serve analytics platform is largely built on Airbyte.Airbyte’s ease of use and extensibility allowed any team in the company to push their data into the platform - without assistance from the data team!"
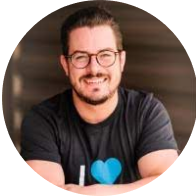
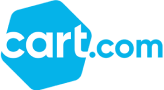
“Airbyte helped us accelerate our progress by years, compared to our competitors. We don’t need to worry about connectors and focus on creating value for our users instead of building infrastructure. That’s priceless. The time and energy saved allows us to disrupt and grow faster.”
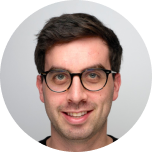

“We chose Airbyte for its ease of use, its pricing scalability and its absence of vendor lock-in. Having a lean team makes them our top criteria. The value of being able to scale and execute at a high level by maximizing resources is immense”
FAQs
What is ETL?
ETL, an acronym for Extract, Transform, Load, is a vital data integration process. It involves extracting data from diverse sources, transforming it into a usable format, and loading it into a database, data warehouse or data lake. This process enables meaningful data analysis, enhancing business intelligence.
Amazon DynamoDB is a fully managed proprietary NoSQL database service that supports key–value and document data structures and is offered by Amazon.com as part of the Amazon Web Services portfolio. DynamoDB exposes a similar data model to and derives its name from Dynamo, but has a different underlying implementation.
What is ELT?
ELT, standing for Extract, Load, Transform, is a modern take on the traditional ETL data integration process. In ELT, data is first extracted from various sources, loaded directly into a data warehouse, and then transformed. This approach enhances data processing speed, analytical flexibility and autonomy.
Difference between ETL and ELT?
ETL and ELT are critical data integration strategies with key differences. ETL (Extract, Transform, Load) transforms data before loading, ideal for structured data. In contrast, ELT (Extract, Load, Transform) loads data before transformation, perfect for processing large, diverse data sets in modern data warehouses. ELT is becoming the new standard as it offers a lot more flexibility and autonomy to data analysts.
Amazon DynamoDB is a fully managed proprietary NoSQL database service that supports key–value and document data structures and is offered by Amazon.com as part of the Amazon Web Services portfolio. DynamoDB exposes a similar data model to and derives its name from Dynamo, but has a different underlying implementation.
Databricks is an American enterprise software company founded by the creators of Apache Spark. Databricks combines data warehouses and data lakes into a lakehouse architecture.
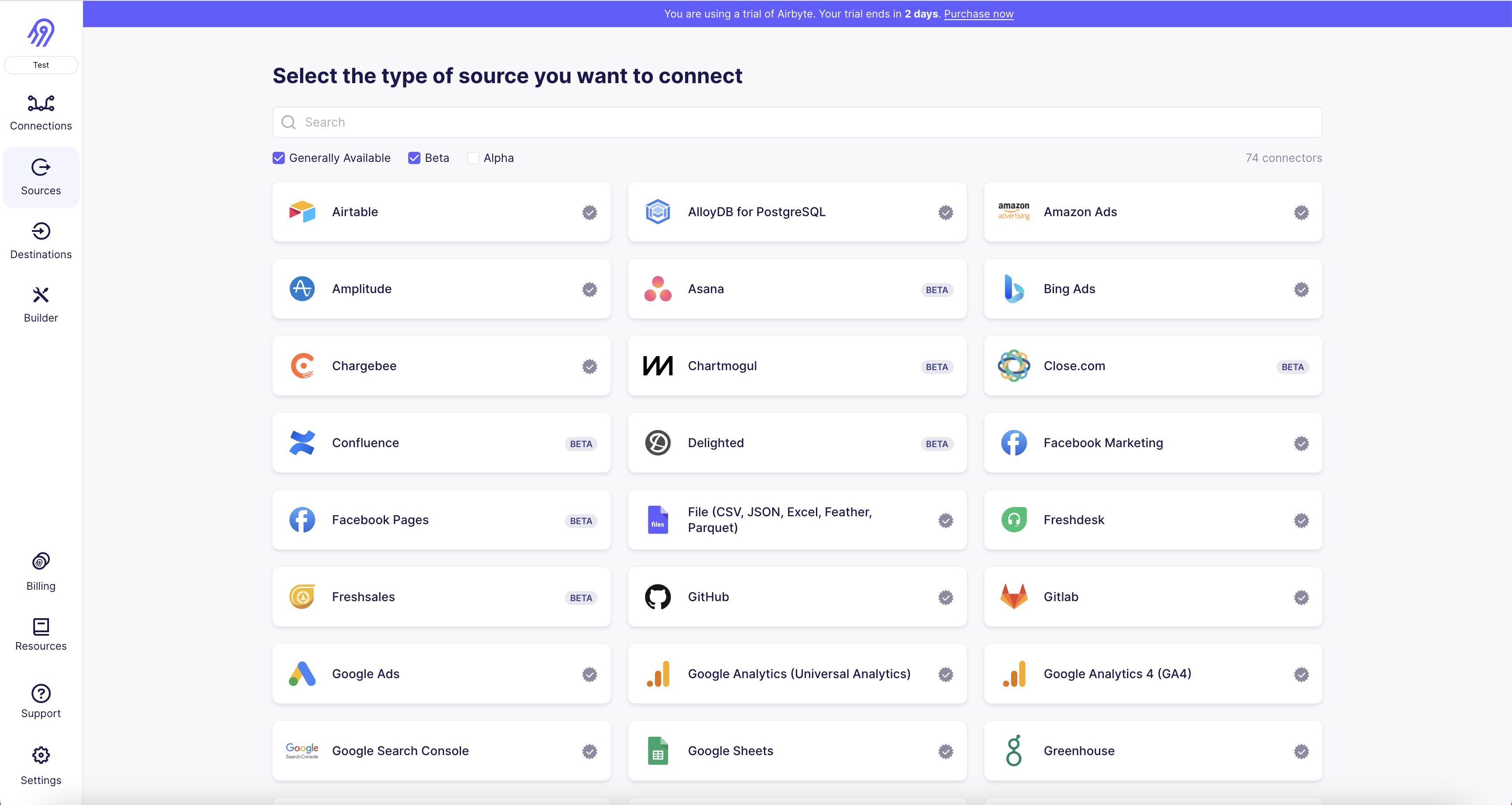

1. First, navigate to the Airbyte website and log in to your account.
2. Once you are logged in, click on the "Destinations" tab on the left-hand side of the screen.
3. Scroll down until you find the "Databricks Lakehouse" connector and click on it.
4. You will be prompted to enter your Databricks Lakehouse credentials, including your account name, personal access token, and workspace ID.
5. Once you have entered your credentials, click on the "Test" button to ensure that the connection is successful.
6. If the test is successful, click on the "Save" button to save your Databricks Lakehouse destination connector settings.
7. You can now use the Databricks Lakehouse connector to transfer data from your source connectors to your Databricks Lakehouse destination.
8. To set up a data transfer, navigate to the "Sources" tab and select the source connector that you want to use.
9. Follow the prompts to enter your source connector credentials and configure your data transfer settings.
10. Once you have configured your source connector, select the Databricks Lakehouse connector as your destination and follow the prompts to configure your data transfer settings.
11. Click on the "Run" button to initiate the data transfer.

With Airbyte, creating data pipelines take minutes, and the data integration possibilities are endless. Airbyte supports the largest catalog of API tools, databases, and files, among other sources. Airbyte's connectors are open-source, so you can add any custom objects to the connector, or even build a new connector from scratch without any local dev environment or any data engineer within 10 minutes with the no-code connector builder.
We look forward to seeing you make use of it! We invite you to join the conversation on our community Slack Channel, or sign up for our newsletter. You should also check out other Airbyte tutorials, and Airbyte’s content hub!
What should you do next?
Hope you enjoyed the reading. Here are the 3 ways we can help you in your data journey:
What should you do next?
Hope you enjoyed the reading. Here are the 3 ways we can help you in your data journey:
Ready to get started?
Frequently Asked Questions
What should you do next?
Hope you enjoyed the reading. Here are the 3 ways we can help you in your data journey: