You have collected unstructured data! Now what?
What is unstructured data
Unstructured data is data that does not conform to a data model and that has no easily identifiable structure. Unstructured data cannot be easily queried and is difficult to analyze. Examples of unstructured data could be the contents of an email, contents of a word document, data from social media, photos, videos, survey results, etc.
One example of unstructured data is free-form text that contains interesting information, but that has not been formatted or stored with a well defined schema. You could imagine a scenario where a team has been asked to gather the name, age and phone number of people without specific instructions about how the data should be gathered. This could look as follows:

When data is stored in the above format, it is difficult and computationally expensive to query the data. For example, if a data set contained billions of rows with such data, then finding all the records where the age is 29 would be slow and inefficient.
What is structured data
Structured data refers to data that has been formatted into a well-defined schema. An example would be data that is stored with precisely defined columns in a relational database. Examples of structured fields could be age, name, phone number, etc. Storing data in a structured format allows it to be easily understood and queried with SQL and used by analytics tools.
If your enterprise has gathered large amounts of unstructured data, then it is likely that you will need to transform it into structured data before you can efficiently make use of it. Extracting structured data from unstructured data is often done during the data transformation stage in either an ETL or an ELT process.
In order to efficiently make use of the unstructured data given in the previous example, it could be transformed it into a structured table the looks like the following:
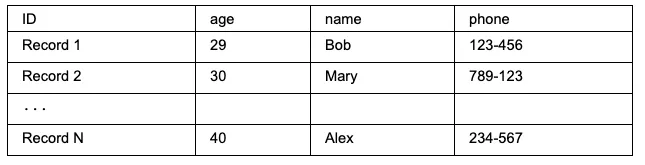
Storing the data in a structured manner makes it much more efficient to query the data. Finding the users where their age is 29 becomes a trivial operation for a database or data warehouse, and could be achieved with the following SQL statement:
ℹ️ Data analysts typically work with structured data stored in relational databases or data warehouses to solve business problems using languages such as SQL, R or Python and visualization tools such as Tableau, Grafana, or Looker Studio.
What is semi-structured data
Semi-structured data is data that lacks a rigid structure and that does not conform directly to a data model, but that has tags, metadata, or elements that describe the data. Examples of semi-structured data are JSON, CSV, or XML files.
Semi-structured data often contains enough information that it can be easily converted into structured data. For example, a text file containing a JSON representation of the same data as presented above could look as follows:

If you are working with a tabular/relational database, then you will likely want to transform the semi-structured data into a table with columns corresponding to the field names, and rows corresponding to each JSON object.
ℹ️ Some NoSQL data stores (eg. MongoDB and Elasticsearch) work directly with JSON objects, and some data stores such as Snowflake support querying semi-structured data.
How can you transform unstructured data into structured data
If you are using an ELT pipeline to load your data into a target system, then you will often want to transform the raw unstructured data into a structured format after it has been stored in the destination. Common methods for transforming data after it has been loaded into the destination include dbt, Spark, or custom code. It is worth noting that Airbyte has excellent support for transforming your data with dbt – however, a detailed discussion on how to transform data is beyond the scope of this article.
On the other hand, if you are using the ETL (legacy) approach for data integration, then data is transformed inside the ETL data pipeline before it is loaded into the destination. In this case, the method for transforming the data would be tool-specific and is also outside the scope of this article.
Conclusion
Collecting data is an important step of your data integration strategy, and this step is well served by Airbyte. Given that up to 80% of the data that is collected by enterprises is unstructured, another commonly required step is the conversion of your data into a structured format that can be efficiently used by SQL and analytics tools.
If you haven’t yet tried out Airbyte to fulfill your data integration needs then you may wish to try Airbyte Cloud for a managed experience, or download Airbyte Open-Source to deploy it in your own infrastructure!
If you've enjoyed reading this article and want more insights, no problem! We've got you covered. Check out our insightful piece on Structured vs Unstructured Data.