What Is Data Quality Management: Framework & Best Practices
Many firms across industries store large amounts of data in various sources, such as CRMs, internal databases, and marketing platforms. This data plays a crucial role in performing advanced analytics and deriving insights. However, most data is often stored in raw, unrefined format. To realize the full potential of your datasets, you need to employ data quality management (DQM) techniques. DQM provides a systematic framework that ensures your organizational data is accurate, consistent, and reliable. It is a critical discipline that helps you achieve operational efficiency and strong data governance.
This article provides a comprehensive guide on data quality management and explains how to implement a DQM framework with best practices. You will also explore some popular trends in data quality management that can help you mitigate risks associated with regulatory compliance.
What Is Data Quality?
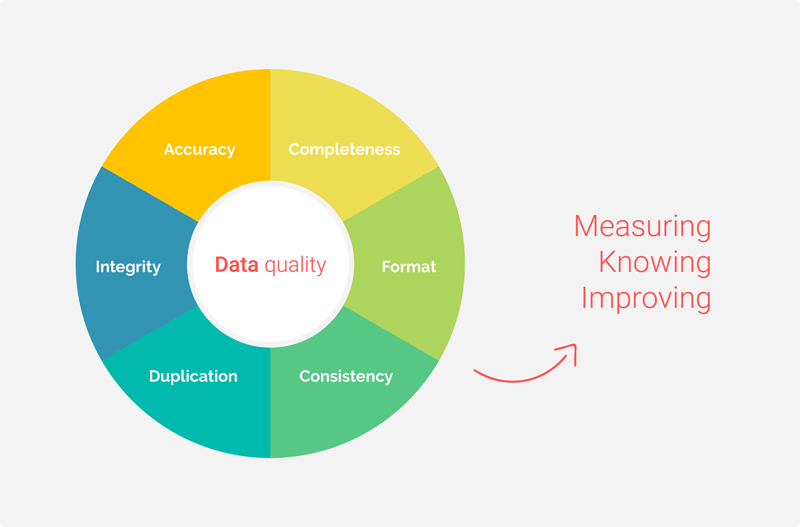
Data quality measures how well your data aligns with your organization’s predefined standards across four key dimensions—validity, completeness, consistency, and accuracy. By adhering to these requirements, you can ensure the trustworthiness and reliability of the data utilized for analysis, reporting, and decision-making.
Closely monitoring these parameters allows you to identify problems that compromise the quality of your data. Addressing them can reduce the time spent fixing errors and reworking, leading to improved employee productivity, customer service, and successful business initiatives.
What Is Data Quality Management?
Data Quality Management involves making data more accurate, consistent, and dependable throughout its lifecycle. It is a robust framework that helps you regularly profile data sources, confirm the data's validation, and run various processes to remove data quality issues.
Every firm has unique data sources, data volumes, and business goals. These factors influence specific key performance indicators (KPIs) for performing data quality management effectiveness.
Features of Data Quality Management
Implementing robust data quality management processes can streamline your organization’s workflows and processes. Here are the main features of efficient DQM:
- Data Cleansing: Data cleansing can correct duplicate records, unusual data representations, and unknown data types. It also ensures that the data standards guidelines are followed while storing and processing datasets.
- Data Profiling: Data profiling helps you validate data through standard statistical methods and identify relationships between data points. This process enables you to find, comprehend, and highlight discrepancies in your data.
- Validating Rules: Validating business rules ensures data aligns with your company’s standards, preventing errors and fostering better decisions. This process, along with clear data definitions, reduces risks and empowers choices based on reliable information.
Why Do You Need Data Quality Management?
When organizations collect large volumes of data and leave it unattended, it leads to unregulated and poorly managed data. This situation is termed a data crisis, where making sense of the data becomes difficult.
Data crises lead to wasted resources and increased maintenance and storage costs. In such a situation, extracting actionable insights from the data and optimizing your business operations is challenging.
Data quality management plays a crucial role in mitigating the data crisis and preventing it from happening in the first place. By implementing DQM techniques, you can transform your data into a valuable asset for informed decision-making. This assists you in identifying data inaccuracies and determining whether the information in your system is precise enough to accomplish your organization’s goals.
How to Implement a Data Quality Management Framework?
A data quality framework is an essential component to consider when developing a data quality plan. It helps you adhere to the predefined requirements by employing various data quality techniques. By following the DQM framework mentioned below, you can prevent problems with data quality in your datasets:
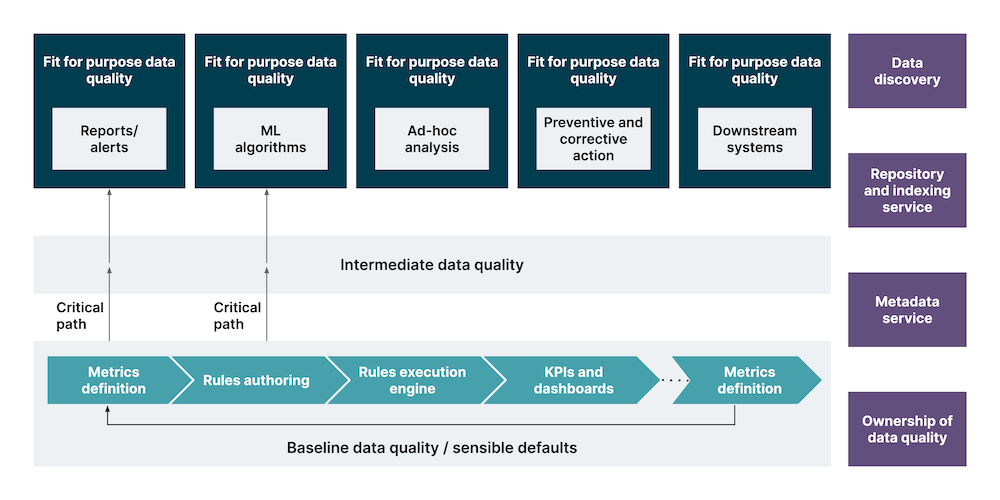
1. Define Your Data Quality Dimensions
The first step involves defining your data quality dimensions. This implies establishing criteria for measuring and ensuring standard data quality requirements across the organization. These dimensions typically include accuracy, completeness, consistency, timeliness, validity, and uniqueness.
2. Establish Data Quality Rules and Guidelines
Data analysts, architects, scientists, senior management teams, and other stakeholders should collaborate to draft rules and regulations to maintain data quality across the organization. This step also involves identifying necessary data cleaning techniques, technologies, and tools that can fit into your existing data infrastructure and budget.
3. Implement Data Cleansing and Enrichment
By implementing cleansing methods, you can remove invalid data from your sources to produce a consistent and useful view of your data. It also involves enriching your data by adding relevant information from reliable sources. This adds context to your data and makes it more comprehensive and insightful.
4. Implement Data Governance and Stewardship
Establishing data governance refers to allocating roles and responsibilities, guidelines, and procedures for effective data utilization and robust security. It entails having version controls for transparency during data modifications, providing rights to data roles, and implementing data recovery measures in case of any disruption.
5. Continuous Monitoring and Improvement
Data quality management is an ongoing process. A sustainable strategy necessitates continuous monitoring of KPIs and target indicators and reporting discrepancies, such as unauthorized access or deviation from the set values. You can also use sophisticated data profiling tools to assess data and produce detailed performance reports.
Best Practices for Data Quality Management
Implementing data quality management best practices can significantly enhance data integrity, ensuring it meets high standards. This maximizes the value your data brings to the organization. Here are some best practices to consider:

Define Your Data Standards Clearly
Implement procedures for identifying and eliminating biases from your data sources. Establish data collection, storage, and processing standards by clearly defining the requirements for data entry, naming conventions, and data formats. This helps you maintain consistency within your datasets.
Automate Processes
Set up automated tests such as pattern matching to avoid mistakes and inconsistencies in data types, date ranges, and unique constraints. Regularly carry out data profiling tasks to gain further understanding of the quality of the data.
Regularize Data Cleaning
Plan on routinely reviewing and cleaning the datasets to find and fix problems, including biases, outliers, duplication, and missing data. This will help preserve your data’s relevance and accuracy over time.
Utilize Data Quality Tools
Specialized data quality tools automate data cleaning, validation, and monitoring processes. This significantly reduces the time and effort required to maintain your data’s quality, accuracy, and consistency.
Encourage a Data-Centric Culture
Provide all employees with thorough training on the importance of high-quality data and clear guidelines for maintaining it. Foster an environment where everyone is accountable for data integrity and understands its impact on the bottom line.
Monitor and Audit Data Quality
Conduct audits and consistently track data quality indicators to evaluate the data quality. This makes it easier to spot new problems quickly and evaluate how well your data quality tactics work.
Enhancing Data Quality Management with Airbyte
Maintaining high data quality standards is crucial for effectively implementing the DQM process. However, integrating data from multiple sources can be complex, leading to inconsistencies and inaccuracies. A streamlined data integration process can help you consolidate all your raw data, transform it, and load it into a central repository. It provides a unified view of your data, making implementing DQM techniques easier. Airbyte is one such tool that facilitates swift data integration.

Airbyte is an ELT data integration platform that helps you integrate your data with its comprehensive library of over 350 pre-built connectors. This array of connectors ensures seamless data movement from diverse sources, promoting consistent and accurate data flow into your desired destination systems. It also provides you the flexibility to create custom connectors using the Connector Development Kit based on your requirements.
Airbyte's unique features that can help enhance your DQM process:
- Complex Transformations: Airbyte allows you to integrate with platforms like dbt, enabling you to perform more complex transformations within your existing workflows. You can also leverage its SQL capabilities to create custom transformations for your pipelines.
- User-Friendly: Airbyte’s user-intuitive interface allows you to get started without technical expertise. This ensures that various stakeholders within your organization can effectively monitor the data.
- Scalability and Flexibility: You can scale Airbyte to meet your organization's growing needs and business requirements. This adaptability is critical to handling increasing data volumes and changing workloads, a vital capability to avoid data crises. Based on your current infrastructure and budget, you can deploy Airbyte as a cloud or self-managed service.
- Schema Change Management: You can configure Airbyte’s settings to detect and propagate schema changes. Airbyte automatically reflects the changes in source data schemas into your target system. This eliminates the need for manual schema definition and mapping, saving valuable time and reducing errors in your DQM process.
To learn more about Airbyte, you can explore Airbyte’s official documentation.
Emerging Trends in Data Quality Management
The rise of new technology and data sources continuously alters the data management processes. Emerging trends are reshaping how you can confirm the accuracy and reliability of your organizational data. Understanding and adopting the trends mentioned below can give you a competitive edge.
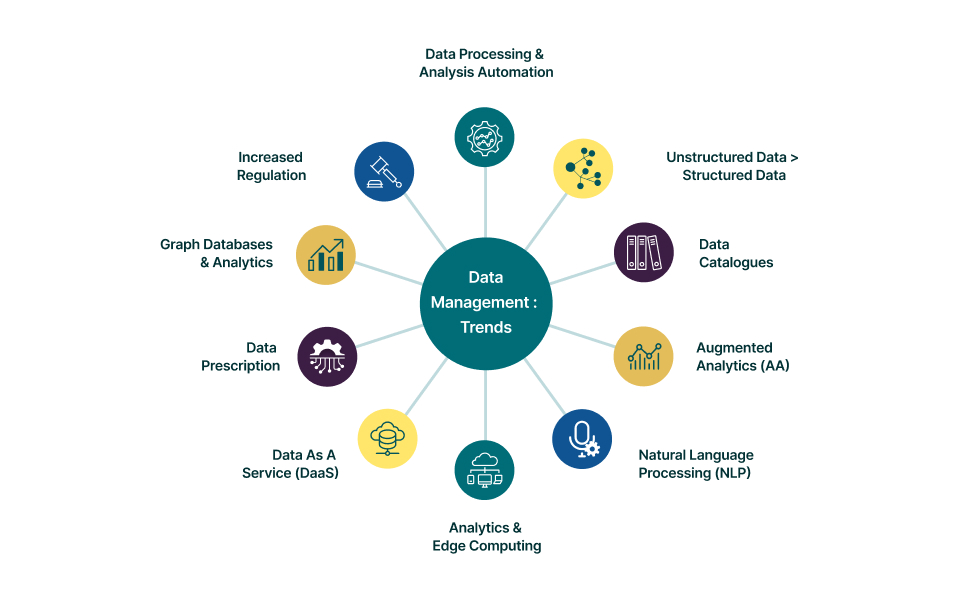
- Machine Learning (ML) and Artificial Intelligence (AI): AI and ML technologies are revolutionizing data quality by automating activities like data cleaning, anomaly detection, and data profiling. This eases your burden, allowing you to focus on high-level strategic tasks.
- Democratization of Data: Accessing high-quality data empowers you to interact with data independently and draw valuable insights. This promotes data literacy and collaboration across departments.
- Cloud-Based Data Quality Management: Cloud-based data management systems are becoming increasingly common due to their ease of use, versatility, and scalability. They also offer a flexible and cost-effective solution.
- Focus on Data Lineage: Data lineage allows you to track and analyze your data from its source to the destination. This transparency promotes data trust, helps understand how the data has transformed over time, and ensures proper data utilization across teams.
- DaaS (Data Quality as a Service): Cloud-based Data-as-a-Service (DaaS) solutions provide access to DQM tools and services over the Internet. It can come in handy if there’s a lack of the necessary resources and expertise to develop your on-premise systems.
Summing It Up
A robust data quality management framework includes a range of procedures and industry standards intended to preserve data consistency, accuracy, and integrity across time. By implementing a structured framework, you can ensure your data is precise, comprehensive, and timely. This also helps you profile your data and recognize its potential.
Remember that DQM is a continuous process. Regularly monitoring your data and adjusting your strategy will help your company get the most out of its information assets. DQM is a necessary investment that will help your organization in the long run.
FAQs
What are the key components of a DQM framework?
A robust DQM framework includes data governance, profiling, cleansing, monitoring, validation, and lineage tracking. These components work together to maintain and improve data quality.
What is data governance?
Data governance involves establishing policies, standards, and procedures for managing data within your organization. It defines roles and responsibilities, sets data quality standards, and ensures compliance with regulatory requirements.