Healthcare Data Integration: Benefits and Best Practices
In the healthcare sector, data forms a crucial foundation of effective patient care, facilitating informed decision-making and improved outcomes. However, patient information is often dispersed across various systems, leading to fragmented health records that could result in misinterpretations or missed diagnoses.
Therefore, to overcome this challenge, you must integrate data from disparate sources to create a holistic view of the patient’s health profile. Research indicates that the global healthcare data integration market was USD 1.34 billion in 2023 and is anticipated to reach USD 4.51 billion by 2032.
In this article, you’ll understand the significance of healthcare data integration, the potential challenges, and best practices to overcome them.
What is Data Integration in Healthcare?
Healthcare data integration is the process of consolidating information from multiple sources, like electronic health records (EHRs) and wearable devices, into a single, cohesive dataset. By centralizing this data, you can gain a comprehensive view of a patient’s medical history. This greatly simplifies workflows and empowers you to make timely decisions, leading to better patient care.
The importance of data integration in healthcare is underscored by the explosive growth of big data in the healthcare industry. According to research, the big data in the healthcare market size was valued at USD 85.91 billion in 2024 and is expected to grow by USD 182.95 billion in 2032.

This surge highlights the increasing volume, variety, and velocity of data being generated from various sources. Big data integration helps you harness this wealth of information, enabling you to identify trends, improve operational efficiencies, and enhance clinical outcomes.
5 Ways to Integrate Data in Healthcare
Let’s explore the various approaches you can use to perform healthcare data integration:
Extract Transform Load (ETL)
ETL involves three sequential steps. First, you should extract relevant data from sources such as lab systems and billing databases. Then, you can transform the data to a usable format, e.g., standardize patient names and medical codes. Finally, you must load the transformed data into a data warehouse or repository for analysis. This approach is particularly beneficial for generating comprehensive reports and insights that can help improve patient care and operational efficiency.
Extract Load Transform (ELT)
ELT differs from ETL primarily in the order of operations. Data is extracted from multiple sources like clinical trials, patient feedback systems, and wearable devices and then immediately loaded into a centralized storage system. Here, data is transformed as needed for specific tasks. This method empowers you to leverage advanced analytics and machine learning techniques on large volumes of unprocessed data.
Data Streaming
Data streaming facilitates real-time integration of healthcare data, which is critical for applications like remote patient monitoring and emergency response systems. This approach allows for the immediate capture and analysis of patient data as it is generated, such as vital signs from monitoring devices or updates from EHRs. This enhances patient care by enabling timely interventions and improving overall responsiveness within healthcare systems.
Application Integration
Application integration involves connecting disparate healthcare applications to facilitate data exchange and interoperability. This could be achieved through APIs (Application Programming Interfaces), which allow diverse software systems to communicate effectively. For instance, you can integrate EHR with a pharmacy management application to ensure that prescriptions are automatically updated and accessible across care teams.
Data Virtualization
Data virtualization lets you access and query data from multiple sources without physically moving it into a central repository. This approach creates a virtual data layer that enables you to integrate information on demand, providing real-time insights while reducing storage costs. With data virtualization, you can create reports that combine information from electronic health records (EHRs), clinical trials, and public health databases without duplicating sensitive data.
Healthcare Data Integration Use Cases
Here are some prominent use cases of healthcare data integration:
Patient-360 View
The patient-360 view refers to a unified view of patient data that enables you to gain a complete, holistic understanding of a patient. This data may include EHR records, patient’s medical history, insurance claims data, lab results, demographic data, and imaging systems. With this single source of truth, you can identify patterns in patient behavior, devise better diagnoses, and deliver personalized care.
Drug Discovery
Developing a new drug is a complex process that requires extensive time, research, and financial resources. Healthcare data integration facilitates access to relevant information from different datasets, which can be used to advance research in drug development. Further, the integration of data from clinical trials and scientific publications provides a more holistic view of drug efficacy and safety.
Crisis Response
Integrated healthcare data is vital for effective crisis response. During times of crisis, such as a pandemic or natural disaster, this data helps you make informed crisis-response decisions. This ensures optimal care for patients and safety for frontline workers, which makes it easier for you to navigate crises with agility and efficiency.
Population Health Management
Data integration lets you analyze big datasets to spot trends and patterns in certain groups of people. This information can then be used to identify risk factors and develop targeted interventions such as vaccination campaigns or chronic disease management programs. This proactive approach improves public health outcomes.
Data Integration Challenges in Healthcare
Below are some of the key challenges you may encounter in healthcare service data integration:
Lack of Standardization
Healthcare data often comes from various sources, such as imaging systems and clinical notes, which can have different formats, code, and terminologies. Lack of standardization can lead to inconsistencies and errors in data, making it difficult to analyze information effectively. Consequently, patients may not receive adequate care due to inaccurate data, which can negatively affect their health outcomes.
Complexity of Legacy Systems
Many healthcare providers still rely on outdated legacy systems that are incompatible with modern technologies. These systems often require significant resources to integrate with new solutions, creating barriers to effective data sharing. The complexity of these legacy systems can lead to inefficiencies in workflows, increased operational costs, and difficulties in accessing patient information.
Data Security Concerns
Data security is a major concern in healthcare, especially as cyber threats continue to rise. In 2024, 67% of surveyed healthcare organizations experienced ransomware attacks, highlighting the vulnerabilities associated with patient data. Therefore, protecting sensitive information is crucial not only for compliance with regulations but also for maintaining patient trust. Breaches can lead to several adverse consequences, including financial losses and reputational damage.
Data Quality Issues
Inaccurate or incomplete data can arise from inconsistent data entry practices or discrepancies between different systems. Relying on such data can lead to misinformed clinical decisions and hinder effective patient care. For example, if a patient's medical history is not accurately reflected due to poor data quality, it could result in inappropriate treatments or medication errors.
Best Practices for Healthcare Data Integration
Here are some best practices that you should consider to perform clinical data integration:
Identify and Document Data Sources
Start by identifying all potential data sources across your healthcare ecosystem, such as EHRs, laboratory test results, insurance systems, and patient portals. Document where critical data resides, how it flows, and any dependencies involved. Mapping out your data sources helps create a clear integration framework.
Design Scalable Integration Solutions
As your healthcare organization expands, scalability becomes essential. To future-proof your integration strategy, choose integration solutions that can easily adapt to new systems and data sources. Look for platforms that offer flexible storage options so you can handle increasing volumes of data without compromising performance.
Implement Industry Standards
Use standardized frameworks like Fast Healthcare Interoperability Resources (FHIR) to exchange data among diverse healthcare systems. These standards act as a common language that enables you to share patient data effectively regardless of how you store or represent data, thereby enhancing interoperability.
Ensure Data Quality
To maintain high-quality data, implement data validation and cleansing processes. Regularly assess the accuracy, completeness, and consistency of your data to detect and resolve inconsistencies, redundancies, or inaccuracies. Further, with healthcare workflow automation, you can reduce errors, enhance clinical decision-making, and deliver better patient care.
Enhance Data Security Protocols
Due to the sensitive nature of healthcare information, it’s crucial to implement robust security measures. You can enforce encryption and multi-factor authentication and conduct regular security audits to identify vulnerabilities. This will safeguard patient data potential breaches and ensure compliance with regulatory frameworks like HIPAA, thereby maintaining patient trust.
Establish Data Governance
Implement a comprehensive data governance framework that encompasses processes and policies for managing data availability, usability, integrity, and security. Clearly define data ownership, access rights, and stewardship responsibilities to foster accountability. This structured approach will create a transparent overview of all data management processes and enhance overall data integrity.
Streamline Healthcare Data Integration Using Airbyte
Manually consolidating data from multiple healthcare sources, such as patient management systems and various other clinical and administrative databases, would be error-prone and time-consuming.
To automate this process, you can leverage Airbyte, a robust data integration and replication tool. It enables you to transfer data from diverse sources to a destination of your choice using 550+ pre-built connectors.
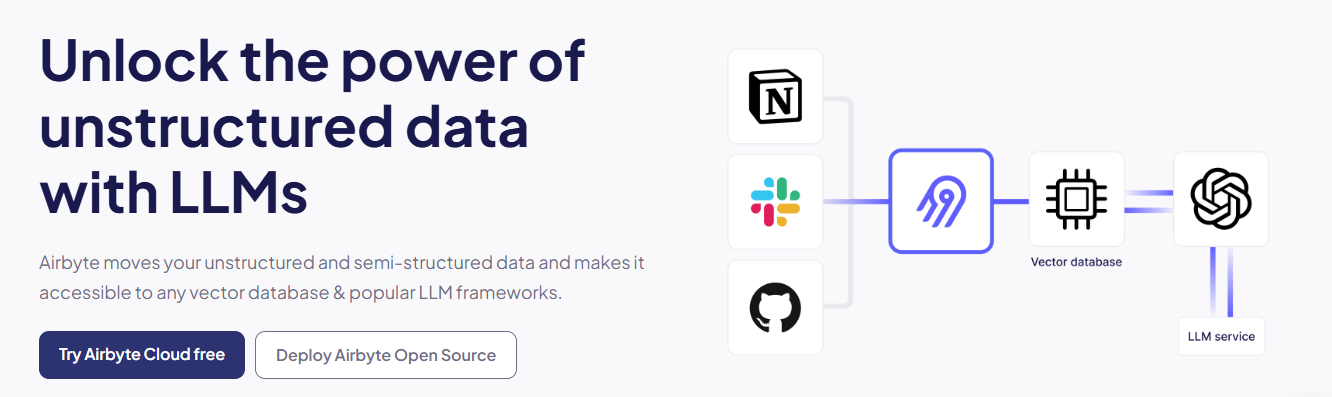
Key Features of Airbyte
Flexible Pipeline Development: Airbyte offers various options for building data pipelines, making them accessible to everyone. These include an intuitive UI, powerful APIs, a Terraform Provider, and PyAirbyte. You can choose the one that best aligns with your requirements.
Custom Connectors: If you can’t find a particular connector, you can utilize Airbyte’s intuitive Connector Builder or Connector Developer Kit (CDK) to build customized connectors. The Connector Builder’s AI-assist functionality scans through your provided API documentation and auto-fills the required fields, drastically reducing setup time.
GenAI Workflows: Airbyte empowers you to simplify AI workflows by loading unstructured data into vector destination stores, such as Pinecone, Chroma, and Weaviate. These vector stores facilitate efficient similarity searches and relevance-based retrieval, which is helpful for data analysis.
Change Data Capture: With Airbyte, you can identify changes made in the source dataset and replicate them in the destination. This enables you to keep track of your data, thus ensuring data integrity and consistency across all your healthcare systems.
Data Pipeline Orchestration: You can integrate Airbyte with popular data orchestrators, such as Dagster, Apache Airflow, Prefect, and Kestra, to optimize your data workflows.
Automatic Detection of Dropped Records: Airbyte enhances data sync reliability by actively monitoring records at the source, platform, and destination levels. It compares record counts across these stages to identify discrepancies caused by issues like serialization and sends notifications for corrective action.
Schema Change Management: You can configure Airbyte to automatically detect schema changes at the source and propagate them to the destination. This functionality maintains data consistency between the source and target systems.
Self-Managed Enterprise: Airbyte offers an Enterprise version that enables you to manage large-scale data effectively. It includes features like multi-tenancy, role-based access control (RBAC), personally identifiable information (PII) masking, and enterprise source connectors.
Enhanced Security: To safeguard your healthcare data from external threats, Airbyte offers various security measures such as authentication mechanisms, encryption, access controls, and audit logging. Further, it adheres to industry-specific regulations, such as HIPAA, GDPR, SOC 2, and ISO 27001, to ensure data privacy and security.
Wrapping Up
This article highlighted the critical role of healthcare data integration in providing high-quality patient care. Further, you've understood various use cases that demonstrate the transformative power of data integration in healthcare environments. While there are some challenges, such as the lack of data standardization, you can use the best practices discussed above to create a more efficient, patient-focused system.