.webp)
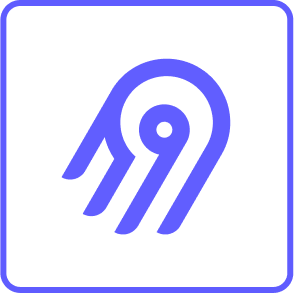

Building your pipeline or Using Airbyte
Airbyte is the only open solution empowering data teams to meet all their growing custom business demands in the new AI era.
- Inconsistent and inaccurate data
- Laborious and expensive
- Brittle and inflexible
- Reliable and accurate
- Extensible and scalable for all your needs
- Deployed and governed your way
Start syncing with Airbyte in 3 easy steps within 10 minutes



Take a virtual tour
Demo video of Airbyte Cloud
Demo video of AI Connector Builder
What sets Airbyte Apart
Modern GenAI Workflows
Move Large Volumes, Fast
An Extensible Open-Source Standard
Full Control & Security
Fully Featured & Integrated
Enterprise Support with SLAs
What our users say
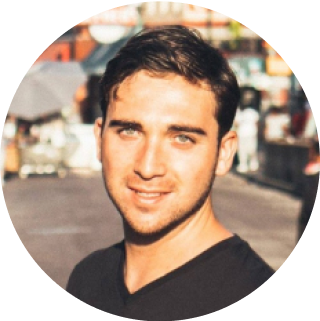
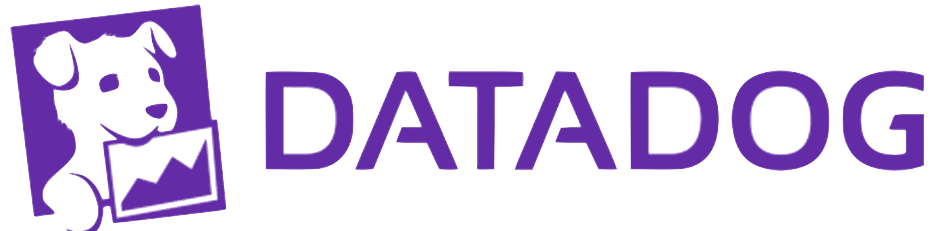
"The intake layer of Datadog’s self-serve analytics platform is largely built on Airbyte.Airbyte’s ease of use and extensibility allowed any team in the company to push their data into the platform - without assistance from the data team!"
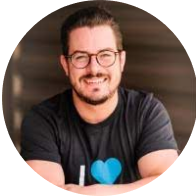
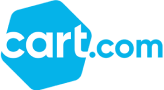
“Airbyte helped us accelerate our progress by years, compared to our competitors. We don’t need to worry about connectors and focus on creating value for our users instead of building infrastructure. That’s priceless. The time and energy saved allows us to disrupt and grow faster.”
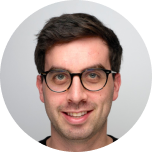

“We chose Airbyte for its ease of use, its pricing scalability and its absence of vendor lock-in. Having a lean team makes them our top criteria. The value of being able to scale and execute at a high level by maximizing resources is immense”
FAQs
What is ETL?
ETL, an acronym for Extract, Transform, Load, is a vital data integration process. It involves extracting data from diverse sources, transforming it into a usable format, and loading it into a database, data warehouse or data lake. This process enables meaningful data analysis, enhancing business intelligence.
CSV (Comma Separated Values) file is a tool used to store and exchange data in a simple and structured format. It is a plain text file that contains data separated by commas, where each line represents a record and each field is separated by a comma. CSV files are widely used in data analysis, data migration, and data exchange between different software applications. The CSV file format is easy to read and write, making it a popular choice for storing and exchanging data. It can be opened and edited using any text editor or spreadsheet software, such as Microsoft Excel or Google Sheets. CSV files can also be imported and exported from databases, making it a convenient tool for data management. CSV files are commonly used for storing large amounts of data, such as customer information, product catalogs, financial data, and scientific data. They are also used for data analysis and visualization, as they can be easily imported into statistical software and other data analysis tools. Overall, the CSV file is a simple and versatile tool that is widely used for storing, exchanging, and analyzing data.
What is ELT?
ELT, standing for Extract, Load, Transform, is a modern take on the traditional ETL data integration process. In ELT, data is first extracted from various sources, loaded directly into a data warehouse, and then transformed. This approach enhances data processing speed, analytical flexibility and autonomy.
Difference between ETL and ELT?
ETL and ELT are critical data integration strategies with key differences. ETL (Extract, Transform, Load) transforms data before loading, ideal for structured data. In contrast, ELT (Extract, Load, Transform) loads data before transformation, perfect for processing large, diverse data sets in modern data warehouses. ELT is becoming the new standard as it offers a lot more flexibility and autonomy to data analysts.
CSV (Comma Separated Values) file is a tool used to store and exchange data in a simple and structured format. It is a plain text file that contains data separated by commas, where each line represents a record and each field is separated by a comma. CSV files are widely used in data analysis, data migration, and data exchange between different software applications. The CSV file format is easy to read and write, making it a popular choice for storing and exchanging data. It can be opened and edited using any text editor or spreadsheet software, such as Microsoft Excel or Google Sheets. CSV files can also be imported and exported from databases, making it a convenient tool for data management. CSV files are commonly used for storing large amounts of data, such as customer information, product catalogs, financial data, and scientific data. They are also used for data analysis and visualization, as they can be easily imported into statistical software and other data analysis tools. Overall, the CSV file is a simple and versatile tool that is widely used for storing, exchanging, and analyzing data.
BigQuery is a cloud-based data warehousing and analytics platform that allows users to store, manage, and analyze large amounts of data in real-time. It is a fully managed service that eliminates the need for users to manage their own infrastructure, and it offers a range of features such as SQL querying, machine learning, and data visualization. BigQuery is designed to handle petabyte-scale datasets and can be used for a variety of use cases, including business intelligence, data exploration, and predictive analytics. It is a powerful tool for organizations looking to gain insights from their data and make data-driven decisions.
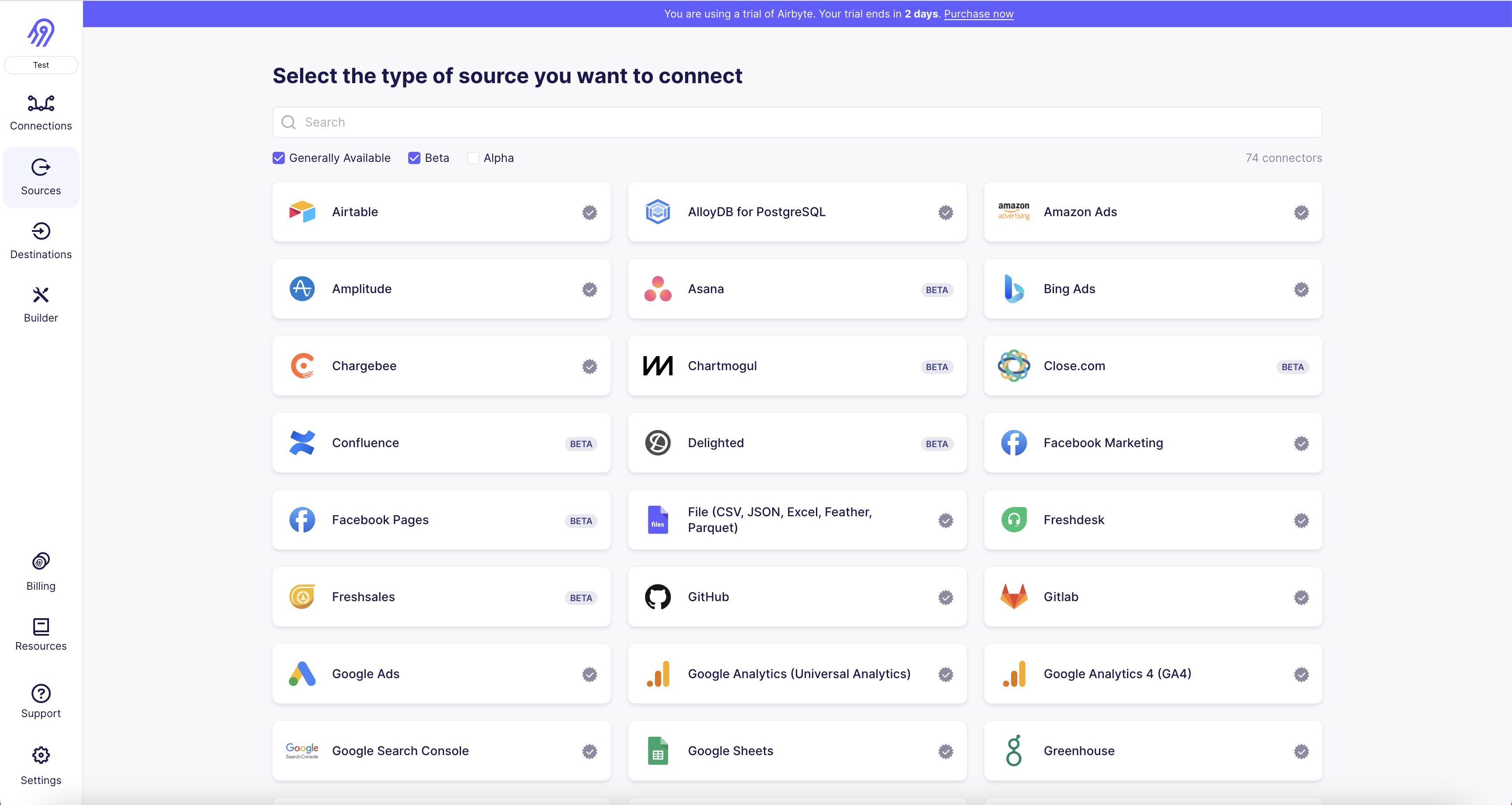
1. Open the Airbyte platform and navigate to the "Destinations" tab on the left-hand side of the screen.
2. Click on the "CSV File" destination connector.
3. Click on the "Create new connection" button.
4. Enter a name for your connection and select the workspace you want to use.
5. Enter the path where you want to save your CSV file.
6. Choose the delimiter you want to use for your CSV file.
7. Select the encoding you want to use for your CSV file.
8. Choose whether you want to append data to an existing file or create a new file each time the connector runs.
9. Enter any additional configuration settings you want to use for your CSV file.
10. Click on the "Test" button to ensure that your connection is working properly.
11. If the test is successful, click on the "Create" button to save your connection.
12. Your CSV File destination connector is now connected and ready to use.

1. First, you need to have a Google Cloud Platform account and a project with BigQuery enabled.
2. Go to the Google Cloud Console and create a new service account with the necessary permissions to access your BigQuery data.
3. Download the JSON key file for the service account and keep it safe.
4. Open Airbyte and go to the Sources page.
5. Click on the "Create a new source" button and select "BigQuery" from the list of available sources.
6. Enter a name for your source and click on "Next".
7. In the "Connection Configuration" section, enter the following information:
- Project ID: the ID of your Google Cloud Platform project
- JSON Key: copy and paste the contents of the JSON key file you downloaded earlier
- Dataset: the name of the dataset you want to connect to
8. Click on "Test Connection" to make sure everything is working correctly.
9. If the test is successful, click on "Create Source" to save your configuration.
10. You can now use your BigQuery source connector to extract data from your dataset and load it into Airbyte for further processing.

With Airbyte, creating data pipelines take minutes, and the data integration possibilities are endless. Airbyte supports the largest catalog of API tools, databases, and files, among other sources. Airbyte's connectors are open-source, so you can add any custom objects to the connector, or even build a new connector from scratch without any local dev environment or any data engineer within 10 minutes with the no-code connector builder.
We look forward to seeing you make use of it! We invite you to join the conversation on our community Slack Channel, or sign up for our newsletter. You should also check out other Airbyte tutorials, and Airbyte’s content hub!
What should you do next?
Hope you enjoyed the reading. Here are the 3 ways we can help you in your data journey:
Efficiently managing and analyzing large datasets is crucial for a business. Google BigQuery, a powerful cloud-based data warehousing solution, offers a seamless way to handle massive amounts of data. This article will guide you through various methods of loading CSV into BigQuery, enabling you to harness the full potential of your data for analysis and decision-making.
What is BigQuery?

Developed by Google, BigQuery is a fully managed and serverless enterprise-ready data warehouse and analytics platform. With BigQuery, you can store, analyze, and gain valuable insights from your data. This makes it a versatile tool for data-driven organizations.
By moving data from CSV to BigQuery, you can streamline your data management and analysis processes. The other advantages include:
- BigQuery is a highly scalable and distributed data warehouse. This makes it suitable to handle enormous amounts of data.
- The serverless architecture of BigQuery ensures that you don’t need to worry about managing infrastructure. This allows you to completely focus on data analysis.
- BigQuery supports standard SQL Queries. If your team is familiar with SQL, you can quickly use it for complex queries and reporting.
- Bigquery seamlessly integrates with other Google Cloud services. This includes Cloud Storage, Dataflow, and Data Studio. This enables you to create an ecosystem that simplifies data management.
How do you prepare a CSV File for BigQuery import?
Structure
- Use a consistent delimiter (comma, tab, or pipe).
- Include a header row with column names.
- Ensure each row has the same number of fields.
Data types
- Format dates as YYYY-MM-DD.
- Format timestamps as YYYY-MM-DD HH:MM:SS[.SSSSSS].
- Use UTF-8 encoding for the file.
Best practices
- Remove any byte order mark (BOM) characters.
- Keep file size under 4GB if using gzip compression.
- Consider splitting very large files for parallel loading.
- Use a consistent representation for null values (e.g., empty string or "NULL").
{{COMPONENT_CTA}}
Methods to Move Data From CSV to Biguery
- Method 1: Connecting CSV to Bigquery using Airbyte.
- Method 2: Connecting CSV to Bigquery manually.
- Method 3: Using the bq Command-Line Tool
Method 1: Connecting CSV to Bigquery using Airbyte
Why use Airbyte to Upload CSV Data to BigQuery?
Some key features of Airbyte include:
- Airbyte provides an intuitive interface for importing data, allowing you to interactively configure load options and monitor the process. Get started with Airbyte for free.
- It offers a growing library of 350+ extensive source and destination connectors.
- Based on configuration settings, Airbyte can automatically discover and infer the source schema changes.
- Using Airbyte, you can establish a Change Data Capture (CDC) pipeline based on logs without the requirement to parse logs manually.
- Airbyte allows users to apply transformations using a web-based interface, making it accessible to non-technical users.
- It supports incremental data synchronization, enabling efficient data replication between various systems.
- Being open-source, Airbyte has an active community of developers who contribute to its development and offer support through forums.
Replicate Data from CSV to BigQuery Using Airbyte
Airbyte is an open-source data integration platform. It is designed to facilitate the collection and movement of data from various sources to different destinations. Its destinations include popular databases, data warehouses, cloud services, APIs, and more.
Apart from a wide range of connectors, what makes Airbyte notable is its support for real-time data synchronization. This feature allows you to quickly make decisions based on the most up-to-date data.
Prerequisites
- A CSV file hosted on AWS S3, GCS, HTTPS, or an SFTP server.
- GCP project in Google Cloud Console.
You would need a BigQuery dataset in your GCP project to load CSV file data. To create a dataset and a table within a dataset, follow the steps mentioned below:
- Log into Google Cloud Console > Create a project > Open BigQuery.
- In the BigQuery, select the project where you want to create the dataset.
- Click on the Create dataset button and provide a unique Dataset ID, Dataset location, description, and set access control for the dataset.
- After configuring the dataset details, click Create Dataset to create a new dataset.
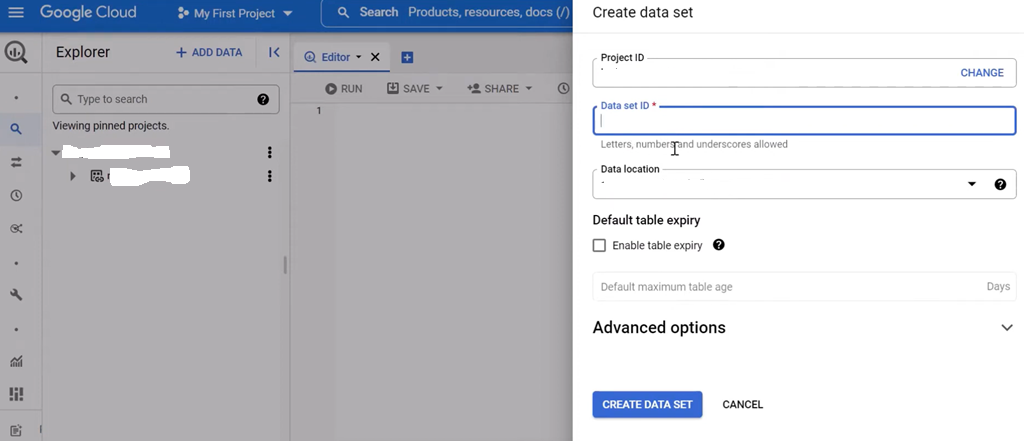
- Open the dataset that you created and click on the Create Table button to create a new table where you’ll be storing CSV file data.
Step 1: Configure a CSV Source in Airbyte
- Sign up for Airbyte Cloud or deploy Airbyte OSS. After successful login, select Sources present on the Airbyte dashboard.
- In the Search bar, type CSV and select the File (CSV, JSON, Excel, Feather, Parquet) connector.
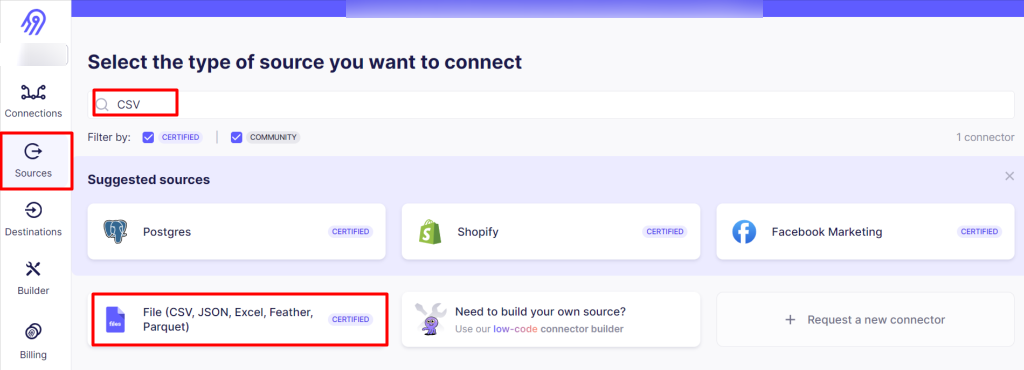
- In the Create a source section:
- Enter a Source name of your choice.
- In the Dataset Name field, enter the name of the target table where you want to replicate the CSV file.
- Select the File Format as CSV from the drop-down.
- Choose the Storage Provider from the drop-down and configure the provider-specific fields.
- Enter the URL path of the CSV file to be replicated in the URL field.

- Once you’ve filled in all the configuration fields, click on Set up source.
- Now, Airbyte will start testing your connection. If all the fields are entered correctly, you’ll receive a connection successful message.
- For a comprehensive understanding of each field, you can refer to the Airbyte File source connector.
Step 2: Configure a BigQuery Destination in Airbyte
- To set BigQuery as your destination in Airbyte, go back to the dashboard and select Destinations.
- Search BigQuery connector in the Search bar and select the connector.
- Enter the name for the BigQuery connector in the Destination name field. In the Connection section, enter your Google Cloud Project ID, Dataset location, Default Dataset ID, Location Method, Service Account Key JSON, GCS Bucket Name, and GCS Bucket Path.

- Click on the Set up Destination button, and Airbyte will initiate to test your destination connection.
- If you find any of the configuration fields unclear, you can refer to Airbyte’s BigQuery setup guide for clarification.
Step 3: Create an Airbyte Connection
- Now go to the dashboard and select Connections from the left-side pane to establish a connection between CSV and BigQuery. Click on Create a new Connection.
- Next, choose the CSV File source you’ve recently created and repeat the same process for the BigQuery destination.
- Provide the new Connection Name and set the Replication frequency. You can change the frequency depending on your requirements.
- On the same page, you can select the Sync mode for your source files. If you aren’t sure about which replication or sync mode to choose, refer to the replication modes in Airbyte. The image below illustrates the configuration steps within Airbyte.

Step 4: Review Data in BigQuery
Once you start the first sync, your data from CSV will be replicated to BigQuery. After a successful sync, you should be able to see your CSV file data migrated in the BigQuery tables.
This approach provides a user-friendly way to ingest and work with CSV data in BigQuery in real time.
Method 2: Connecting CSV to Bigquery Manually.
Moving data from a CSV file to Google BigQuery can be done using Google Cloud Platform (GCP) tools without relying on third-party connectors. Here's a detailed step-by-step guide to accomplish this:
Step 1: Set up Google Cloud Platform Account
If you haven't already, sign up for a Google Cloud Platform account. You will need to provide billing information to activate your account.
Step 2: Create a New Project
1. Go to the GCP Console (https://console.cloud.google.com/).
2. Click on the project dropdown at the top of the page.
3. Click on "New Project".
4. Enter a project name and select a billing account.
5. Click "Create".
Step 3: Enable BigQuery API
1. Navigate to the "API & Services" dashboard.
2. Click "Enable APIs and Services".
3. Search for "BigQuery API" and enable it.
Step 4: Prepare Your CSV File
Ensure your CSV file is properly formatted and clean. The first row should contain column headers, and the data types should be consistent in each column.
Step 5: Upload Your CSV to Google Cloud Storage
1. Go to the GCP Console and navigate to "Storage" in the left-hand menu.
2. Click "Create bucket", enter a name for your bucket, and follow the prompts to create your bucket.
3. Once the bucket is created, click on its name to open it.
4. Click "Upload files" and select your CSV file to upload it to the bucket.
Step 6: Create a Dataset in BigQuery
1. Go to the BigQuery console.
2. In the left-hand menu, click on your project name.
3. Click "Create Dataset" on the right side.
4. Enter a Dataset ID and choose a data location.
5. Click "Create dataset".
Step 7: Create a Table in BigQuery
1. With the dataset selected, click "Create Table".
2. In the "Create table from" dropdown, select "Google Cloud Storage".
3. In the "Select file" field, browse or enter the path to your CSV file in the bucket.
4. Choose the file format as "CSV".
5. Enter a Table name.
6. Under "Schema", you can either select "Auto-detect" to let BigQuery infer the schema from the CSV header or manually define the schema.
7. Configure other settings if necessary (e.g., partitioning).
8. Click "Create table".
Step 8: Verify Data Import
After the table creation process is complete, you should verify that the data has been imported correctly:
1. In the BigQuery console, navigate to your new table.
2. Click on "Preview" to see a sample of the data.
3. Optionally, run a few queries to ensure the data looks as expected.
Notes:
1. Make sure your CSV file is not too large, as there are limits on how much data you can import to BigQuery at once. If the file is too large, consider splitting it into smaller chunks.
2. Ensure that the CSV file is encoded in UTF-8 if it contains special characters.
3. If you're automating this process, consider using Google Cloud SDK (gcloud command-line tool) or the BigQuery API in your preferred programming language.
4. Watch out for any errors during the import process, and refer to the BigQuery documentation for troubleshooting tips.
Method 3: Using the bq Command-Line Tool
The bq command-line tool is a powerful utility for interacting with BigQuery from your terminal. Here’s how to use it to load CSV data:
Install and Set Up
- Install the Google Cloud SDK containing the bq tool.
- Authenticate using: gcloud auth login
- Set your project: gcloud config set project YOUR_PROJECT_ID
Prepare Your Data
- Ensure your CSV file is ready and accessible.
- If using Cloud Storage, upload your file: gsutil cp your_data.csv gs://your-bucket/
Create a Schema File (Optional)
[
{"name": "column1", "type": "STRING"},
{"name": "column2", "type": "INTEGER"},
{"name": "column3", "type": "DATE"}
]
Run the Load Command
bq load \
--source_format=CSV \
--skip_leading_rows=1 \
--autodetect \
dataset_name.table_name \
gs://your-bucket/your_data.csv \
schema.json
Key options:
- --source_format=CSV: Specifies the file type.
- --skip_leading_rows=1: Skips the header row.
- --autodetect: Automatically detects the schema (or you can provide a schema file).
Monitor the Job
The command will display job progress. Once complete, verify using:
bq show dataset_name.table_name
This method is excellent for scripting and automation, especially in data pipeline scenarios.
Method 4: Using the Python Client Library
The Python client library offers a programmatic approach to loading data, ideal for integrating BigQuery operations into your Python applications.
Set Up Your Environment
- Install the library: pip install google-cloud-bigquery
- Set up authentication (typically using a service account key or application default credentials)
Write Your Python Script
from google.cloud import bigquery
import os
# Set up the client
os.environ["GOOGLE_APPLICATION_CREDENTIALS"] = "path/to/your/service-account-key.json"
client = bigquery.Client()
# Configure the loading job
job_config = bigquery.LoadJobConfig(
schema=[
bigquery.SchemaField("name", "STRING"),
bigquery.SchemaField("age", "INTEGER"),
bigquery.SchemaField("date", "DATE"),
],
skip_leading_rows=1,
source_format=bigquery.SourceFormat.CSV,
)
# Specify the source and destination
table_id = "your-project.dataset_name.table_name"
file_path = "gs://your-bucket/your_data.csv"
# Start the load job
load_job = client.load_table_from_uri(
file_path, table_id, job_config=job_config
)
# Wait for the job to complete
load_job.result()
# Get the result
destination_table = client.get_table(table_id)
print(f"Loaded {destination_table.num_rows} rows.")
Run Your Script
Execute your Python script. It will initiate the load job and wait for completion.
Error Handling and Verification
- Add try/except blocks to handle potential errors.
- Use client.get_table() to verify the loaded data.
Wrapping up
Moving data from a CSV file to a robust warehouse like Google BigQuery can streamline your decision-making journey, facilitating data analysis and informed choices.
This article taught you how to implement an ELT pipeline from CSV to BigQuery quickly. This streamlined approach simplifies the process of capturing and loading CSV data to BigQuery.
You can quickly participate in discussions on Airbyte’s community Slack channel, where you can engage with a thriving community of data professionals, share your insights, and collectively contribute to the success of diverse projects.
What should you do next?
Hope you enjoyed the reading. Here are the 3 ways we can help you in your data journey:
Ready to get started?
Frequently Asked Questions
What should you do next?
Hope you enjoyed the reading. Here are the 3 ways we can help you in your data journey: