Gutendex is a simple, self-hosted web API for serving book catalog information from Project Gutenberg, an online library of free ebooks.Gutendex. JSON web API for Project Gutenberg ebook metadata.Gutenberg can be a useful source of literature, but its large size makes it difficult to access and analyse it on a large scale. Gutendex downloads these files, stores their data in a database, and publishes the data in a simpler format. Gutendex uses Django to download catalog data and serve it in a simple JSON REST API.
Convex is a platform that provides a suite of tools for building and deploying machine learning models. It offers a user-friendly interface for data scientists and developers to create and train models, as well as a scalable infrastructure for deploying them in production. Convex also includes features such as automated model tuning, version control, and collaboration tools to streamline the machine learning workflow. The platform is designed to be flexible and customizable, allowing users to integrate their own libraries and frameworks. Overall, Convex aims to simplify the process of building and deploying machine learning models, making it accessible to a wider range of users.
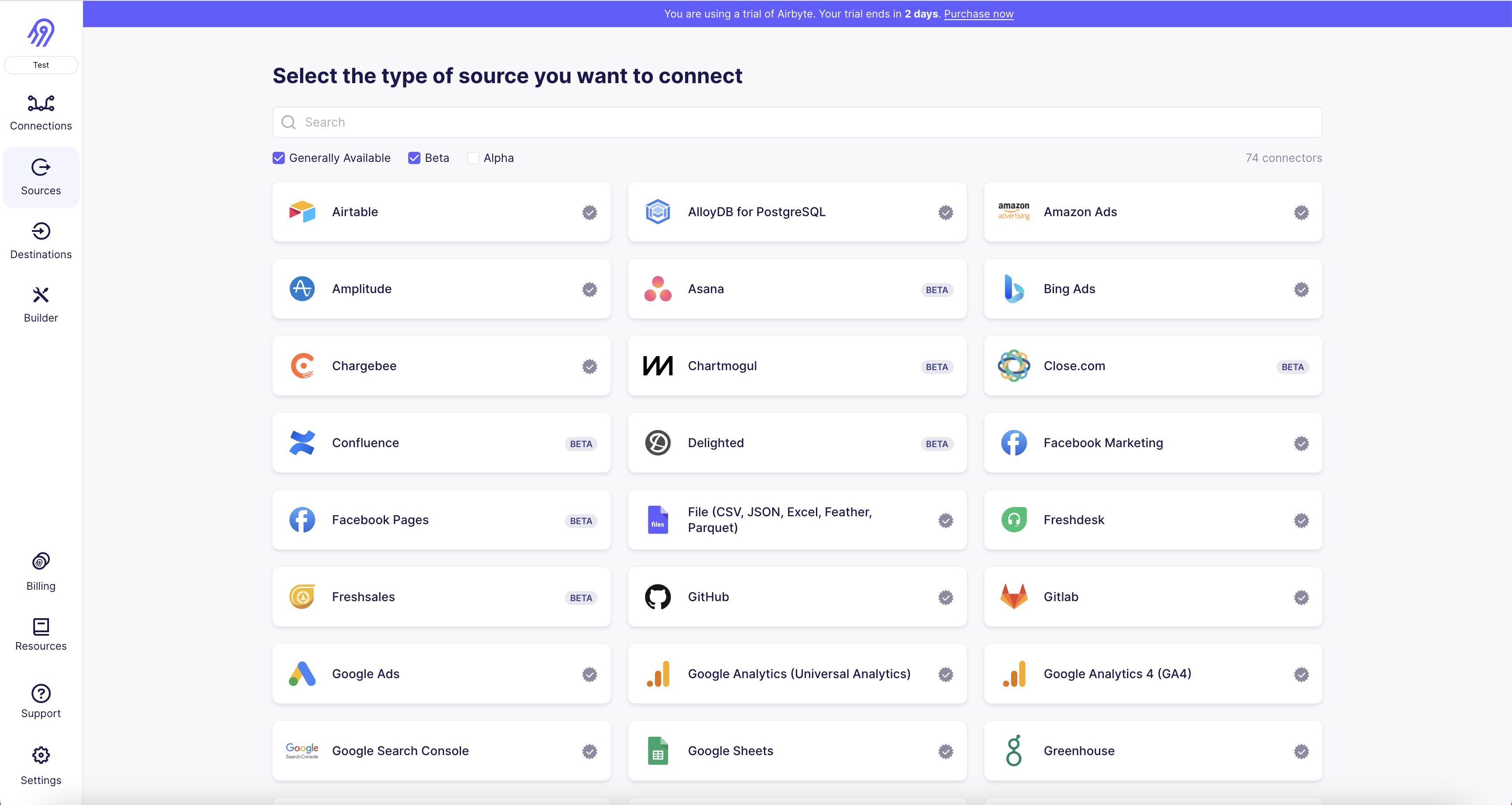
1. First, navigate to the Gutendex source connector page on Airbyte.com.
2. Click on the "Create a new connection" button.
3. Enter a name for your connection and click "Next".
4. In the "Configure your Gutendex connection" section, enter your Gutendex API key and click "Test connection" to ensure that the credentials are correct.
5. Once the connection is successful, select the tables you want to replicate and click "Next".
6. In the "Configure replication frequency" section, select how often you want the data to be replicated and click "Next".
7. In the "Configure destination" section, select the destination where you want to replicate the data and click "Create connection".
8. Your Gutendex source connector is now connected and ready to replicate data to your chosen destination.

1. First, navigate to the Airbyte website and log in to your account.
2. Once you are logged in, click on the "Destinations" tab on the left-hand side of the screen.
3. From there, click on the "Add Destination" button in the top right corner of the screen.
4. In the search bar, type "Convex" and select the Convex destination connector from the list of options.
5. Next, you will need to enter your Convex API key. This can be found in your Convex account settings.
6. Once you have entered your API key, click on the "Test" button to ensure that the connection is working properly.
7. If the test is successful, click on the "Save" button to save your settings.
8. You can now use the Convex destination connector to transfer data from Airbyte to your Convex account.

With Airbyte, creating data pipelines take minutes, and the data integration possibilities are endless. Airbyte supports the largest catalog of API tools, databases, and files, among other sources. Airbyte's connectors are open-source, so you can add any custom objects to the connector, or even build a new connector from scratch without any local dev environment or any data engineer within 10 minutes with the no-code connector builder.
We look forward to seeing you make use of it! We invite you to join the conversation on our community Slack Channel, or sign up for our newsletter. You should also check out other Airbyte tutorials, and Airbyte’s content hub!
What should you do next?
Hope you enjoyed the reading. Here are the 3 ways we can help you in your data journey:
What should you do next?
Hope you enjoyed the reading. Here are the 3 ways we can help you in your data journey:
Ready to get started?
Frequently Asked Questions
Gutendex's API provides access to a vast collection of data related to books and literature. The following are the categories of data that can be accessed through the API:
1. Book metadata: This includes information about the book such as title, author, publisher, publication date, language, and genre.
2. Book content: The API provides access to the full text of the book, which can be used for text analysis and natural language processing.
3. Book covers: The API also provides access to book covers, which can be used for visual analysis and identification.
4. Book reviews: The API provides access to book reviews and ratings, which can be used for sentiment analysis and recommendation systems.
5. Book availability: The API provides information about the availability of the book in different formats such as e-book, audiobook, and print.
6. Book sales data: The API provides access to sales data for books, which can be used for market analysis and forecasting.
Overall, Gutendex's API provides a comprehensive set of data related to books and literature, which can be used for a wide range of applications in the publishing industry, academia, and beyond.