Jira is an issue tracking software by Atlassian that assists developers in bug tracking and agile project management. With software support throughout the entire development process, from planning to tracking, to the final release, and reports based on real-time data to improve team performance, Jira is the go-to software development tool for agile teams.
A communication solutions agency, Kafka is a cloud-based / on-prem distributed system offering social media services, public relations, and events. For event streaming, three main functionalities are available: the ability to (1) subscribe to (read) and publish (write) streams of events, (2) store streams of events indefinitely, durably, and reliably, and (3) process streams of events in either real-time or retrospectively. Kafka offers these capabilities in a secure, highly scalable, and elastic manner.
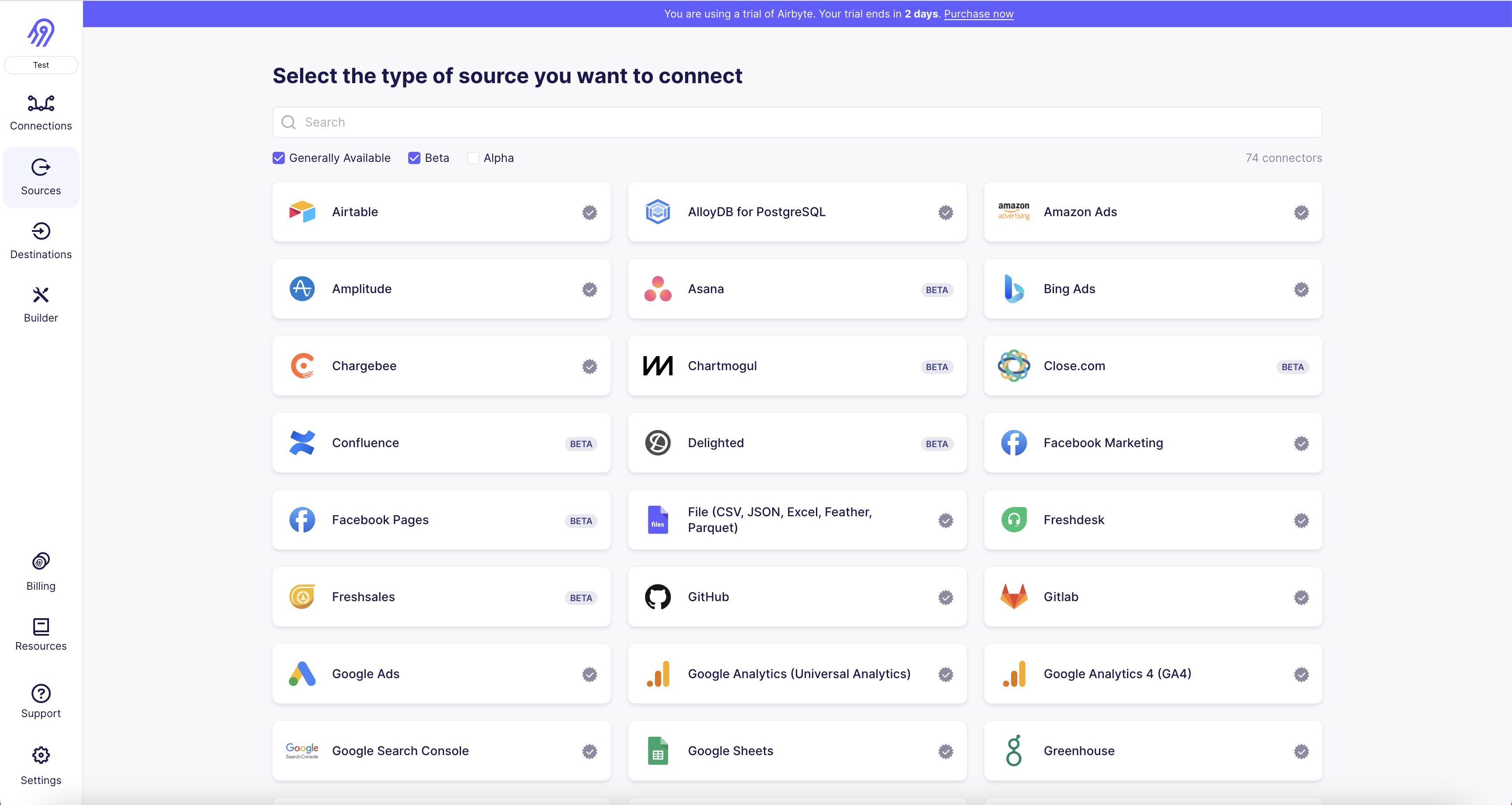
1. First, navigate to the Airbyte dashboard and click on "Sources" on the left-hand side of the screen.
2. Click on the "Add Source" button in the top right corner of the screen.
3. Select "Jira" from the list of available sources.
4. Enter a name for your Jira source connector and click "Next".
5. Enter your Jira credentials, including the Jira URL, email address, and API token.
6. Test the connection to ensure that the credentials are correct and the connection is successful.
7. Select the Jira projects and issue types that you want to replicate in Airbyte.
8. Choose the replication frequency and any other settings that you want to apply to your Jira source connector.
9. Click "Create Source" to save your Jira source connector and begin replicating data from Jira to Airbyte.
It is important to note that the specific steps for connecting your Jira source connector may vary depending on your specific use case and the version of Jira that you are using. For more detailed instructions and troubleshooting tips, refer to the Airbyte documentation or consult with a Jira expert.

1. First, you need to have an Apache Kafka destination connector installed on your system. If you don't have it, you can download it from the Apache Kafka website.
2. Once you have the Apache Kafka destination connector installed, you need to create a new connection in Airbyte. To do this, go to the Connections tab and click on the "New Connection" button. 3. In the "New Connection" window, select "Apache Kafka" as the destination connector and enter the required connection details, such as the Kafka broker URL, topic name, and authentication credentials.
4. After entering the connection details, click on the "Test Connection" button to ensure that the connection is working properly.
5. If the connection test is successful, click on the "Save" button to save the connection.
6. Once the connection is saved, you can create a new pipeline in Airbyte and select the Apache Kafka destination connector as the destination for your data.
7. In the pipeline configuration, select the connection you created in step 3 as the destination connection.
8. Configure the pipeline to map the source data to the appropriate Kafka topic and fields.
9. Once the pipeline is configured, you can run it to start sending data to your Apache Kafka destination.

With Airbyte, creating data pipelines take minutes, and the data integration possibilities are endless. Airbyte supports the largest catalog of API tools, databases, and files, among other sources. Airbyte's connectors are open-source, so you can add any custom objects to the connector, or even build a new connector from scratch without any local dev environment or any data engineer within 10 minutes with the no-code connector builder.
We look forward to seeing you make use of it! We invite you to join the conversation on our community Slack Channel, or sign up for our newsletter. You should also check out other Airbyte tutorials, and Airbyte’s content hub!
What should you do next?
Hope you enjoyed the reading. Here are the 3 ways we can help you in your data journey:
TL;DR
This can be done by building a data pipeline manually, usually a Python script (you can leverage a tool as Apache Airflow for this). This process can take more than a full week of development. Or it can be done in minutes on Airbyte in three easy steps:
- set up Jira as a source connector (using Auth, or usually an API key)
- set up Kafka as a destination connector
- define which data you want to transfer and how frequently
You can choose to self-host the pipeline using Airbyte Open Source or have it managed for you with Airbyte Cloud.
This tutorial’s purpose is to show you how.
What is Jira
Jira is an issue tracking software by Atlassian that assists developers in bug tracking and agile project management. With software support throughout the entire development process, from planning to tracking, to the final release, and reports based on real-time data to improve team performance, Jira is the go-to software development tool for agile teams.
What is Kafka
A communication solutions agency, Kafka is a cloud-based / on-prem distributed system offering social media services, public relations, and events. For event streaming, three main functionalities are available: the ability to (1) subscribe to (read) and publish (write) streams of events, (2) store streams of events indefinitely, durably, and reliably, and (3) process streams of events in either real-time or retrospectively. Kafka offers these capabilities in a secure, highly scalable, and elastic manner.
{{COMPONENT_CTA}}
Prerequisites
- A Jira account to transfer your customer data automatically from.
- A Kafka account.
- An active Airbyte Cloud account, or you can also choose to use Airbyte Open Source locally. You can follow the instructions to set up Airbyte on your system using docker-compose.
Airbyte is an open-source data integration platform that consolidates and streamlines the process of extracting and loading data from multiple data sources to data warehouses. It offers pre-built connectors, including Jira and Kafka, for seamless data migration.
When using Airbyte to move data from Jira to Kafka, it extracts data from Jira using the source connector, converts it into a format Kafka can ingest using the provided schema, and then loads it into Kafka via the destination connector. This allows businesses to leverage their Jira data for advanced analytics and insights within Kafka, simplifying the ETL process and saving significant time and resources.
Methods to Move Data From jira to kafka
- Method 1: Connecting jira to kafka using Airbyte.
- Method 2: Connecting jira to kafka manually.
Method 1: Connecting jira to kafka using Airbyte
Step 1: Set up Jira as a source connector
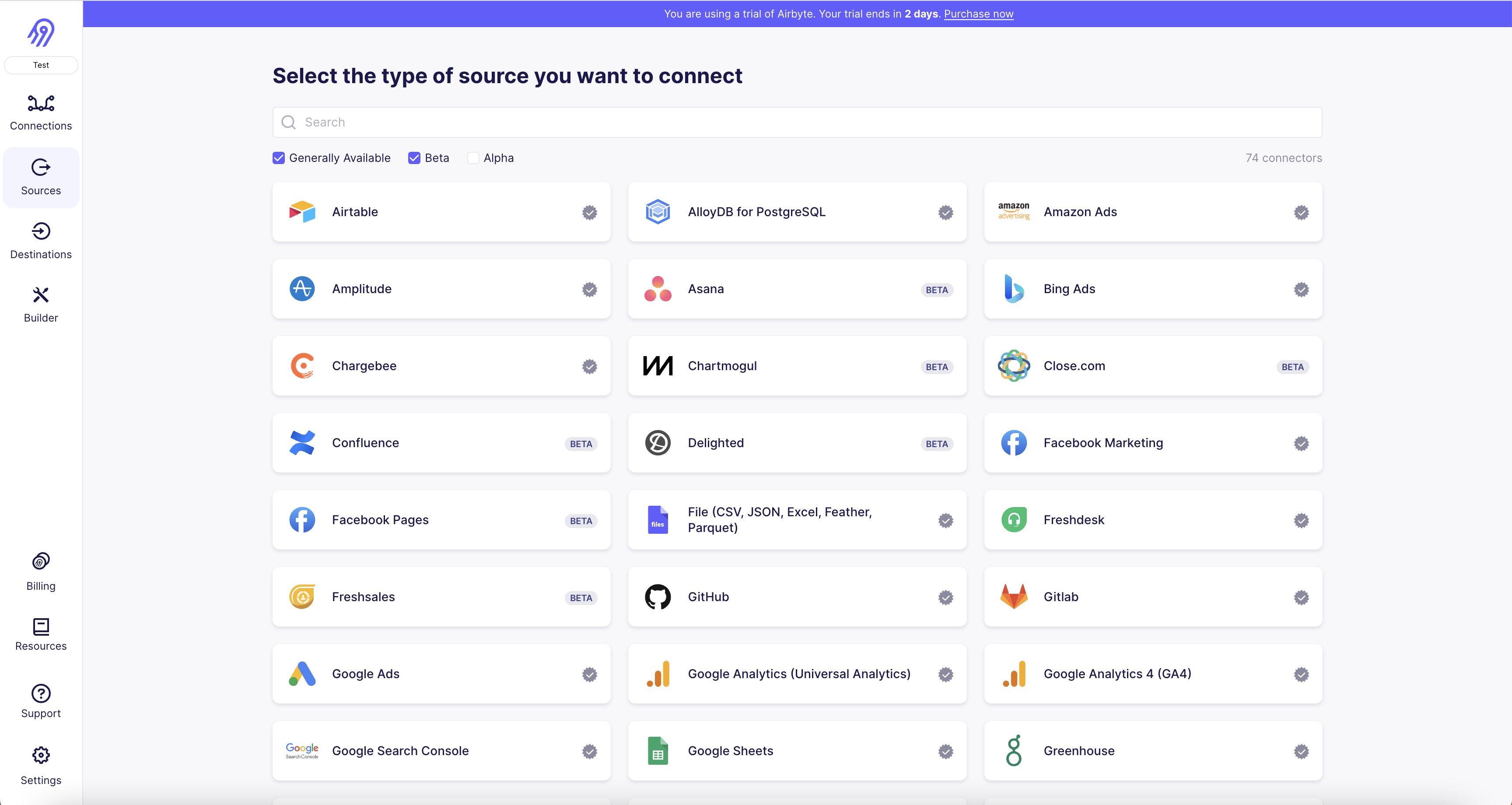
1. First, navigate to the Airbyte dashboard and click on "Sources" on the left-hand side of the screen.
2. Click on the "Add Source" button in the top right corner of the screen.
3. Select "Jira" from the list of available sources.
4. Enter a name for your Jira source connector and click "Next".
5. Enter your Jira credentials, including the Jira URL, email address, and API token.
6. Test the connection to ensure that the credentials are correct and the connection is successful.
7. Select the Jira projects and issue types that you want to replicate in Airbyte.
8. Choose the replication frequency and any other settings that you want to apply to your Jira source connector.
9. Click "Create Source" to save your Jira source connector and begin replicating data from Jira to Airbyte.
It is important to note that the specific steps for connecting your Jira source connector may vary depending on your specific use case and the version of Jira that you are using. For more detailed instructions and troubleshooting tips, refer to the Airbyte documentation or consult with a Jira expert.
Step 2: Set up Kafka as a destination connector

1. First, you need to have an Apache Kafka destination connector installed on your system. If you don't have it, you can download it from the Apache Kafka website.
2. Once you have the Apache Kafka destination connector installed, you need to create a new connection in Airbyte. To do this, go to the Connections tab and click on the "New Connection" button. 3. In the "New Connection" window, select "Apache Kafka" as the destination connector and enter the required connection details, such as the Kafka broker URL, topic name, and authentication credentials.
4. After entering the connection details, click on the "Test Connection" button to ensure that the connection is working properly.
5. If the connection test is successful, click on the "Save" button to save the connection.
6. Once the connection is saved, you can create a new pipeline in Airbyte and select the Apache Kafka destination connector as the destination for your data.
7. In the pipeline configuration, select the connection you created in step 3 as the destination connection.
8. Configure the pipeline to map the source data to the appropriate Kafka topic and fields.
9. Once the pipeline is configured, you can run it to start sending data to your Apache Kafka destination.
Step 3: Set up a connection to sync your Jira data to Kafka

Once you've successfully connected Jira as a data source and Kafka as a destination in Airbyte, you can set up a data pipeline between them with the following steps:
- Create a new connection: On the Airbyte dashboard, navigate to the 'Connections' tab and click the '+ New Connection' button.
- Choose your source: Select Jira from the dropdown list of your configured sources.
- Select your destination: Choose Kafka from the dropdown list of your configured destinations.
- Configure your sync: Define the frequency of your data syncs based on your business needs. Airbyte allows both manual and automatic scheduling for your data refreshes.
- Select the data to sync: Choose the specific Jira objects you want to import data from towards Kafka. You can sync all data or select specific tables and fields.
- Select the sync mode for your streams: Choose between full refreshes or incremental syncs (with deduplication if you want), and this for all streams or at the stream level. Incremental is only available for streams that have a primary cursor.
- Test your connection: Click the 'Test Connection' button to make sure that your setup works. If the connection test is successful, save your configuration.
- Start the sync: If the test passes, click 'Set Up Connection'. Airbyte will start moving data from Jira to Kafka according to your settings.
Remember, Airbyte keeps your data in sync at the frequency you determine, ensuring your Kafka data warehouse is always up-to-date with your Jira data.
Method 2: Connecting jira to kafka manually
Moving data from Jira to Kafka without using third-party connectors or integrations requires a custom development approach. This typically involves using Jira's REST API to extract data and Kafka's Producer API to send data to a Kafka topic. Here is a detailed step-by-step guide to achieve this:
Step 1: Set up Kafka Environment
1. Download Kafka: Go to the official Kafka website and download the latest binary files.
2. Install Kafka: Unpack the downloaded files into your preferred directory.
3. Start Zookeeper: Kafka uses Zookeeper, so you first need to start a Zookeeper server if you don't have one running already.
```
bin/zookeeper-server-start.sh config/zookeeper.properties
```
4. Start Kafka Server: Open another terminal window and start the Kafka server.
```
bin/kafka-server-start.sh config/server.properties
```
5. Create a Kafka Topic: Create a topic where Jira data will be published.
```
bin/kafka-topics.sh --create --topic jira-topic --bootstrap-server localhost:9092 --replication-factor 1 --partitions 1
```
Step 2: Access Jira REST API
1. Jira API Documentation: Familiarize yourself with the Jira REST API documentation to understand how to retrieve the data you need.
2. Authentication: Set up the necessary authentication to access the Jira API. This usually involves creating an API token or using OAuth.
3. Permissions: Ensure the user account used for the API has the right permissions to access the data you want to extract.
Step 3: Develop a Data Extraction Script
1. Choose a Programming Language: Select a programming language you are comfortable with that has good support for HTTP requests and Kafka producer libraries (e.g., Java, Python, Node.js).
2. Set Up Your Development Environment: Make sure you have the necessary SDKs and libraries installed for HTTP requests and Kafka.
3. Write a Script to Call Jira API:
- Use an HTTP client library to make requests to the Jira API.
- Handle pagination if you are dealing with large datasets.
- Parse the API response and extract the necessary data.
- Handle errors and exceptions appropriately.
4. Serialize the Data: Convert the extracted data into a format suitable for Kafka (e.g., JSON, Avro, String).
Step 4: Develop Kafka Producer
1. Kafka Producer API: Use the Kafka Producer API available in your chosen language to send messages to Kafka.
2. Configure Producer: Set up the required Kafka producer configurations (e.g., bootstrap servers, key and value serializers, retries).
3. Send Data to Kafka Topic: Write a function that takes the serialized data and sends it to the Kafka topic created earlier.
4. Error Handling: Implement proper error handling to manage any issues that occur while sending data to Kafka.
5. Logging: Add logging to track the data flow and any issues.
Step 5: Schedule Data Transfer
1. Cron Job: Set up a cron job or a scheduled task to run your script at regular intervals, depending on your data freshness requirements.
2. Continuous Service: Alternatively, develop your script as a long-running service that continuously polls Jira for updates and sends them to Kafka.
Step 6: Testing and Validation
1. Unit Testing: Write unit tests for your code to ensure each component (API calls, data serialization, Kafka producer) works as expected.
2. End-to-End Testing: Test the entire pipeline from Jira to Kafka to ensure data is correctly extracted, transformed, and loaded into Kafka.
3. Monitor Kafka Topic: Use Kafka consumer scripts or tools like Kafka Tool to monitor the topic and validate that data is arriving correctly.
Step 7: Deployment and Monitoring
1. Deploy the Script: Deploy your script or service to a stable environment that has access to both Jira and Kafka.
2. Monitoring: Set up monitoring and alerting to track the health of the data pipeline and quickly detect failures.
3. Logging: Ensure that your script logs important events and errors to facilitate troubleshooting.
Step 8: Documentation and Maintenance
1. Documentation: Document the entire setup, including the purpose of the pipeline, configurations, deployment steps, and any operational procedures.
2. Maintenance Plan: Establish a plan for maintaining the code, including handling API changes, Kafka upgrades, and other potential disruptions.
Please note that this is a high-level guide and actual implementation details may vary based on the specific requirements and constraints of your environment. Always ensure to follow best practices for security, error handling, and data privacy when accessing and transferring sensitive information.
Use Cases to transfer your Jira data to Kafka
Integrating data from Jira to Kafka provides several benefits. Here are a few use cases:
- Advanced Analytics: Kafka’s powerful data processing capabilities enable you to perform complex queries and data analysis on your Jira data, extracting insights that wouldn't be possible within Jira alone.
- Data Consolidation: If you're using multiple other sources along with Jira, syncing to Kafka allows you to centralize your data for a holistic view of your operations, and to set up a change data capture process so you never have any discrepancies in your data again.
- Historical Data Analysis: Jira has limits on historical data. Syncing data to Kafka allows for long-term data retention and analysis of historical trends over time.
- Data Security and Compliance: Kafka provides robust data security features. Syncing Jira data to Kafka ensures your data is secured and allows for advanced data governance and compliance management.
- Scalability: Kafka can handle large volumes of data without affecting performance, providing an ideal solution for growing businesses with expanding Jira data.
- Data Science and Machine Learning: By having Jira data in Kafka, you can apply machine learning models to your data for predictive analytics, customer segmentation, and more.
- Reporting and Visualization: While Jira provides reporting tools, data visualization tools like Tableau, PowerBI, Looker (Google Data Studio) can connect to Kafka, providing more advanced business intelligence options. If you have a Jira table that needs to be converted to a Kafka table, Airbyte can do that automatically.
Wrapping Up
To summarize, this tutorial has shown you how to:
- Configure a Jira account as an Airbyte data source connector.
- Configure Kafka as a data destination connector.
- Create an Airbyte data pipeline that will automatically be moving data directly from Jira to Kafka after you set a schedule
With Airbyte, creating data pipelines take minutes, and the data integration possibilities are endless. Airbyte supports the largest catalog of API tools, databases, and files, among other sources. Airbyte's connectors are open-source, so you can add any custom objects to the connector, or even build a new connector from scratch without any local dev environment or any data engineer within 10 minutes with the no-code connector builder.
We look forward to seeing you make use of it! We invite you to join the conversation on our community Slack Channel, or sign up for our newsletter. You should also check out other Airbyte tutorials, and Airbyte’s content hub!
What should you do next?
Hope you enjoyed the reading. Here are the 3 ways we can help you in your data journey:
Ready to get started?
Frequently Asked Questions
Jira's API provides access to a wide range of data related to project management and issue tracking. The following are the categories of data that can be accessed through Jira's API:
1. Issues: This includes all the information related to the issues such as issue type, status, priority, description, comments, attachments, and more.
2. Projects: This includes information about the projects such as project name, description, project lead, and more.
3. Users: This includes information about the users such as user name, email address, and more.
4. Workflows: This includes information about the workflows such as workflow name, workflow steps, and more.
5. Custom fields: This includes information about the custom fields such as custom field name, type, and more.
6. Dashboards: This includes information about the dashboards such as dashboard name, description, and more.
7. Reports: This includes information about the reports such as report name, description, and more.
8. Agile boards: This includes information about the agile boards such as board name, board type, and more.
Overall, Jira's API provides access to a vast amount of data that can be used to improve project management and issue tracking.