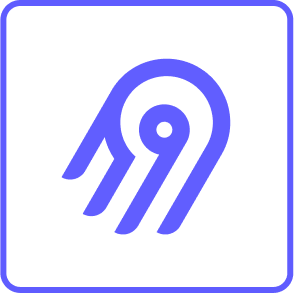

Building your pipeline or Using Airbyte
Airbyte is the only open solution empowering data teams to meet all their growing custom business demands in the new AI era.
- Inconsistent and inaccurate data
- Laborious and expensive
- Brittle and inflexible
- Reliable and accurate
- Extensible and scalable for all your needs
- Deployed and governed your way
Start syncing with Airbyte in 3 easy steps within 10 minutes



Take a virtual tour
Demo video of Airbyte Cloud
Demo video of AI Connector Builder
What sets Airbyte Apart
Modern GenAI Workflows
Move Large Volumes, Fast
An Extensible Open-Source Standard
Full Control & Security
Fully Featured & Integrated
Enterprise Support with SLAs
What our users say
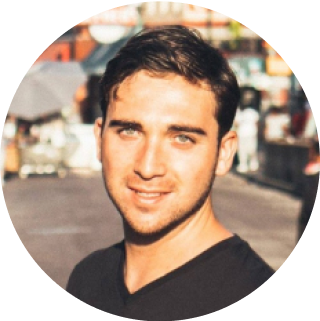
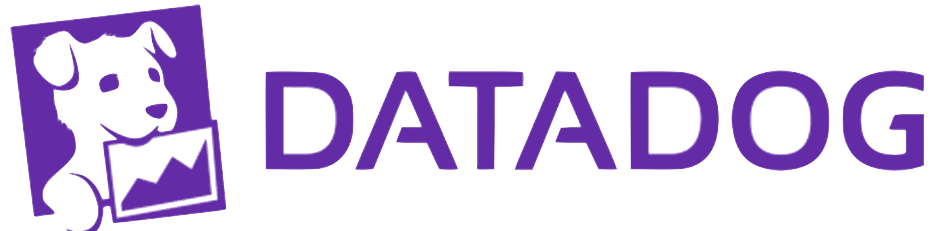
"The intake layer of Datadog’s self-serve analytics platform is largely built on Airbyte.Airbyte’s ease of use and extensibility allowed any team in the company to push their data into the platform - without assistance from the data team!"
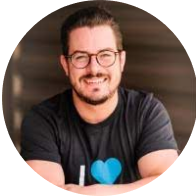
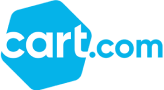
“Airbyte helped us accelerate our progress by years, compared to our competitors. We don’t need to worry about connectors and focus on creating value for our users instead of building infrastructure. That’s priceless. The time and energy saved allows us to disrupt and grow faster.”
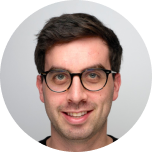

“We chose Airbyte for its ease of use, its pricing scalability and its absence of vendor lock-in. Having a lean team makes them our top criteria. The value of being able to scale and execute at a high level by maximizing resources is immense”
FAQs
What is ETL?
ETL, an acronym for Extract, Transform, Load, is a vital data integration process. It involves extracting data from diverse sources, transforming it into a usable format, and loading it into a database, data warehouse or data lake. This process enables meaningful data analysis, enhancing business intelligence.
MongoDB is a popular open-source NoSQL database that stores data in a flexible, document-based format. It is designed to handle large volumes of unstructured data and is highly scalable, making it a popular choice for modern web applications. MongoDB uses a JSON-like format to store data, which allows for easy integration with web applications and APIs. It also supports dynamic queries, indexing, and aggregation, making it a powerful tool for data analysis. MongoDB is widely used in industries such as finance, healthcare, and e-commerce, and is known for its ease of use and flexibility.
MongoDB gives access to a wide range of data types, including:
1. Documents: MongoDB stores data in the form of documents, which are similar to JSON objects. Each document contains a set of key-value pairs that represent the data.
2. Collections: A collection is a group of related documents that are stored together in MongoDB. Collections can be thought of as tables in a relational database.
3. Indexes: MongoDB supports various types of indexes, including single-field, compound, and geospatial indexes. Indexes are used to improve query performance.
4. GridFS: MongoDB's GridFS is a specification for storing and retrieving large files, such as images and videos, in MongoDB.
5. Aggregation: MongoDB's aggregation framework provides a way to perform complex data analysis operations, such as grouping, filtering, and sorting, on large datasets.
6. Transactions: MongoDB supports multi-document transactions, which allow multiple operations to be performed atomically.
7. Change streams: MongoDB's change streams provide a way to monitor changes to data in real-time, allowing applications to react to changes as they occur.
Overall, MongoDB provides access to a flexible and powerful data model that can handle a wide range of data types and use cases.
What is ELT?
ELT, standing for Extract, Load, Transform, is a modern take on the traditional ETL data integration process. In ELT, data is first extracted from various sources, loaded directly into a data warehouse, and then transformed. This approach enhances data processing speed, analytical flexibility and autonomy.
Difference between ETL and ELT?
ETL and ELT are critical data integration strategies with key differences. ETL (Extract, Transform, Load) transforms data before loading, ideal for structured data. In contrast, ELT (Extract, Load, Transform) loads data before transformation, perfect for processing large, diverse data sets in modern data warehouses. ELT is becoming the new standard as it offers a lot more flexibility and autonomy to data analysts.
MongoDB is a popular open-source NoSQL database that stores data in a flexible, document-based format. It is designed to handle large volumes of unstructured data and is highly scalable, making it a popular choice for modern web applications. MongoDB uses a JSON-like format to store data, which allows for easy integration with web applications and APIs. It also supports dynamic queries, indexing, and aggregation, making it a powerful tool for data analysis. MongoDB is widely used in industries such as finance, healthcare, and e-commerce, and is known for its ease of use and flexibility.
An integrated cloud application and platform service, Oracle offers an array of enterprise information technology solutions. Other company offerings include software-as-a-service (SaaS), platform-as-a-service (PaaS, and infrastructure-as-a-service (IaaS). The Oracle Cloud Infrastructure provides companies the convenience of the public cloud combined with the security and control of on-premises infrastructure. Oracle Cloud Applications help companies streamline their business processes, increase productivity and reduce costs with software applications such as Project Portfolio Management, ERP Financials, Procurement, and more.
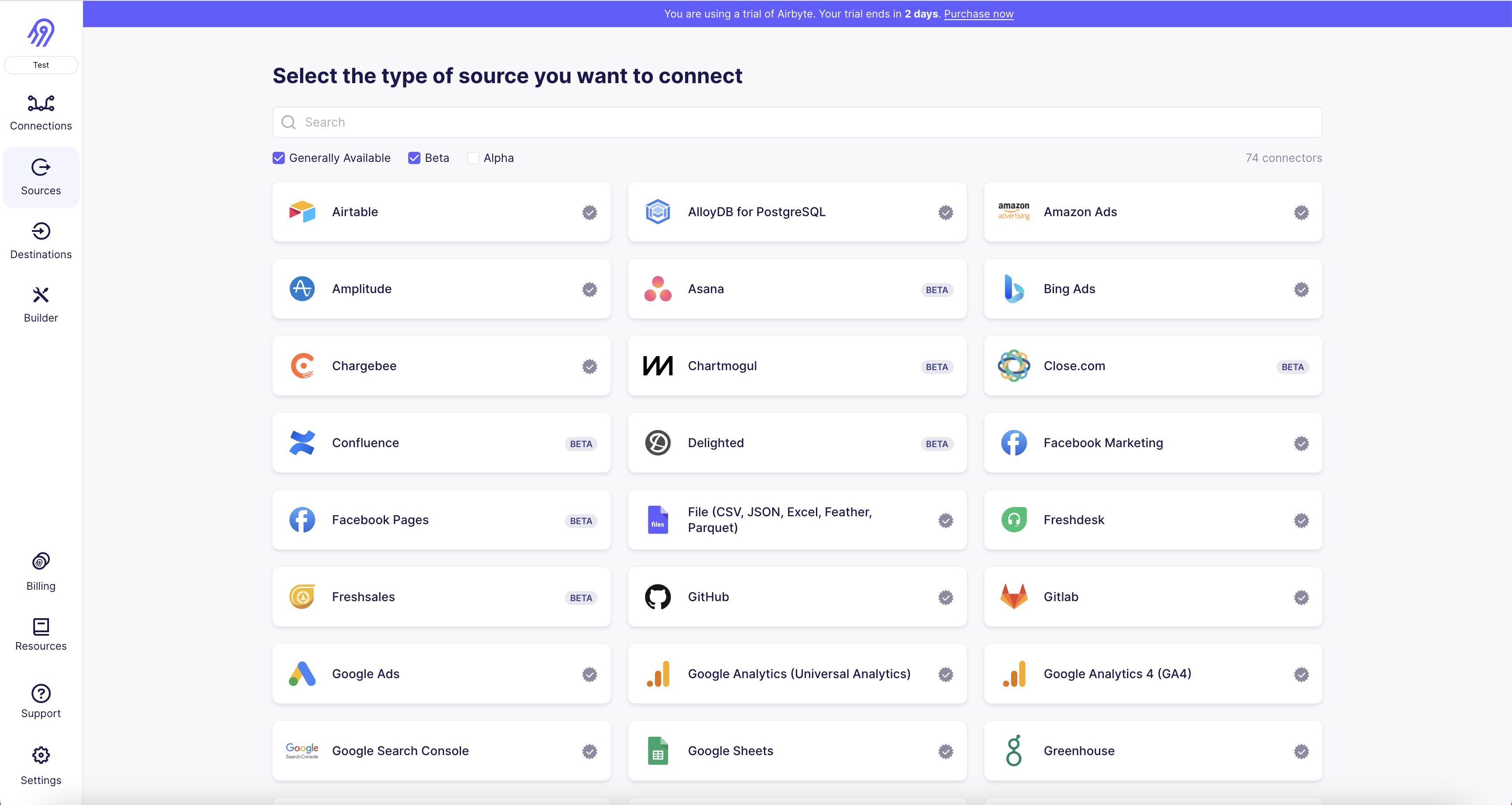
1. First, you need to have a MongoDB instance running and accessible from the internet. You will also need to have the necessary credentials to access the database.
2. In the Airbyte dashboard, click on "Sources" and then click on "New Source."
3. Select "MongoDB" from the list of available sources.
4. In the "Connection Configuration" section, enter the following information:
- Host: The hostname or IP address of your MongoDB instance.
- Port: The port number on which your MongoDB instance is running.
- Username: The username you use to access your MongoDB instance.
- Password: The password you use to access your MongoDB instance.
- Authentication Database: The name of the database where your authentication credentials are stored.
5. Click on "Test Connection" to ensure that Airbyte can connect to your MongoDB instance.
6. If the connection is successful, click on "Save" to save your MongoDB source configuration.
7. You can now create a new pipeline and select your MongoDB source as the input. You can then configure the pipeline to transform and load your data into your desired destination.

1. First, ensure that you have the necessary credentials to access your Oracle DB. This includes the hostname, port number, database name, username, and password.
2. Open the Airbyte platform and navigate to the "Destinations" tab on the left-hand side of the screen.
3. Scroll down until you find the "Oracle DB" destination connector and click on it.
4. Click on the "Create new connection" button to begin setting up your Oracle DB destination.
5. Enter a name for your connection and fill in the required fields with your Oracle DB credentials.
6. Test the connection to ensure that Airbyte can successfully connect to your Oracle DB.
7. Once the connection is successful, you can configure the settings for your Oracle DB destination. This includes selecting the tables you want to sync, setting up any transformations or mappings, and scheduling the sync frequency.
8. Save your settings and start the sync process. Airbyte will begin pulling data from your source and pushing it to your Oracle DB destination.
9. Monitor the sync process to ensure that it is running smoothly and troubleshoot any issues that may arise.
10. Once the sync is complete, you can access your data in your Oracle DB and use it for analysis, reporting, or any other purposes.

With Airbyte, creating data pipelines take minutes, and the data integration possibilities are endless. Airbyte supports the largest catalog of API tools, databases, and files, among other sources. Airbyte's connectors are open-source, so you can add any custom objects to the connector, or even build a new connector from scratch without any local dev environment or any data engineer within 10 minutes with the no-code connector builder.
We look forward to seeing you make use of it! We invite you to join the conversation on our community Slack Channel, or sign up for our newsletter. You should also check out other Airbyte tutorials, and Airbyte’s content hub!
What should you do next?
Hope you enjoyed the reading. Here are the 3 ways we can help you in your data journey:
TL;DR
This can be done by building a data pipeline manually, usually a Python script (you can leverage a tool as Apache Airflow for this). This process can take more than a full week of development. Or it can be done in minutes on Airbyte in three easy steps:
- set up MongoDb as a source connector (using Auth, or usually an API key)
- set up Oracle as a destination connector
- define which data you want to transfer and how frequently
You can choose to self-host the pipeline using Airbyte Open Source or have it managed for you with Airbyte Cloud.
This tutorial’s purpose is to show you how.
What is MongoDb
MongoDB is a popular open-source NoSQL database that stores data in a flexible, document-based format. It is designed to handle large volumes of unstructured data and is highly scalable, making it a popular choice for modern web applications. MongoDB uses a JSON-like format to store data, which allows for easy integration with web applications and APIs. It also supports dynamic queries, indexing, and aggregation, making it a powerful tool for data analysis. MongoDB is widely used in industries such as finance, healthcare, and e-commerce, and is known for its ease of use and flexibility.
What is Oracle
An integrated cloud application and platform service, Oracle offers an array of enterprise information technology solutions. Other company offerings include software-as-a-service (SaaS), platform-as-a-service (PaaS, and infrastructure-as-a-service (IaaS). The Oracle Cloud Infrastructure provides companies the convenience of the public cloud combined with the security and control of on-premises infrastructure. Oracle Cloud Applications help companies streamline their business processes, increase productivity and reduce costs with software applications such as Project Portfolio Management, ERP Financials, Procurement, and more.
{{COMPONENT_CTA}}
Prerequisites
- A MongoDb account to transfer your customer data automatically from.
- A Oracle account.
- An active Airbyte Cloud account, or you can also choose to use Airbyte Open Source locally. You can follow the instructions to set up Airbyte on your system using docker-compose.
Airbyte is an open-source data integration platform that consolidates and streamlines the process of extracting and loading data from multiple data sources to data warehouses. It offers pre-built connectors, including MongoDb and Oracle, for seamless data migration.
When using Airbyte to move data from MongoDb to Oracle, it extracts data from MongoDb using the source connector, converts it into a format Oracle can ingest using the provided schema, and then loads it into Oracle via the destination connector. This allows businesses to leverage their MongoDb data for advanced analytics and insights within Oracle, simplifying the ETL process and saving significant time and resources.
Methods to Move Data From Mongodb to oracle db
- Method 1: Connecting Mongodb to oracle db using Airbyte.
- Method 2: Connecting Mongodb to oracle db manually.
Method 1: Connecting Mongodb to oracle db using Airbyte
Step 1: Set up MongoDb as a source connector
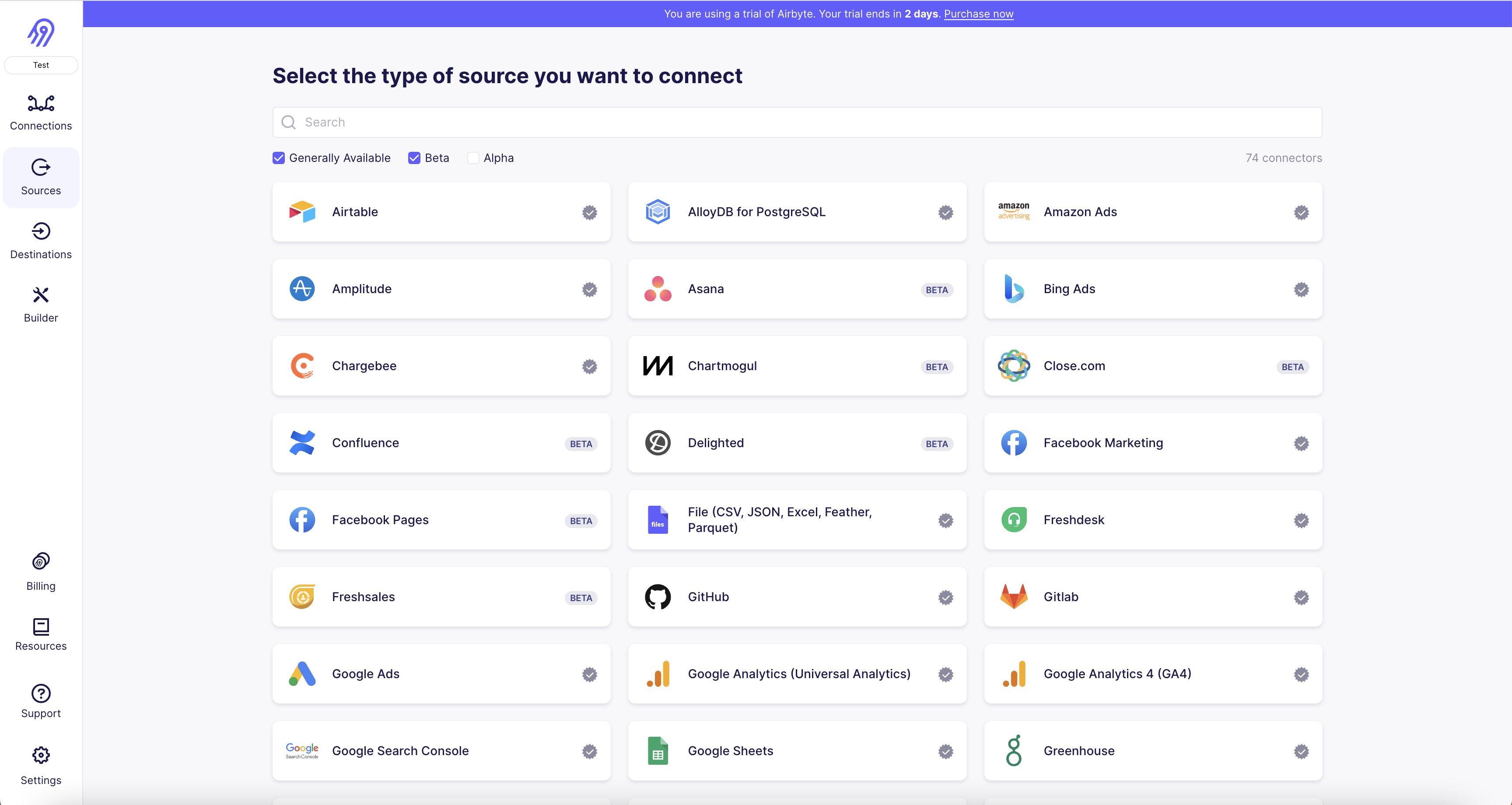
1. First, you need to have a MongoDB instance running and accessible from the internet. You will also need to have the necessary credentials to access the database.
2. In the Airbyte dashboard, click on "Sources" and then click on "New Source."
3. Select "MongoDB" from the list of available sources.
4. In the "Connection Configuration" section, enter the following information:
- Host: The hostname or IP address of your MongoDB instance.
- Port: The port number on which your MongoDB instance is running.
- Username: The username you use to access your MongoDB instance.
- Password: The password you use to access your MongoDB instance.
- Authentication Database: The name of the database where your authentication credentials are stored.
5. Click on "Test Connection" to ensure that Airbyte can connect to your MongoDB instance.
6. If the connection is successful, click on "Save" to save your MongoDB source configuration.
7. You can now create a new pipeline and select your MongoDB source as the input. You can then configure the pipeline to transform and load your data into your desired destination.
Step 2: Set up Oracle as a destination connector

1. First, ensure that you have the necessary credentials to access your Oracle DB. This includes the hostname, port number, database name, username, and password.
2. Open the Airbyte platform and navigate to the "Destinations" tab on the left-hand side of the screen.
3. Scroll down until you find the "Oracle DB" destination connector and click on it.
4. Click on the "Create new connection" button to begin setting up your Oracle DB destination.
5. Enter a name for your connection and fill in the required fields with your Oracle DB credentials.
6. Test the connection to ensure that Airbyte can successfully connect to your Oracle DB.
7. Once the connection is successful, you can configure the settings for your Oracle DB destination. This includes selecting the tables you want to sync, setting up any transformations or mappings, and scheduling the sync frequency.
8. Save your settings and start the sync process. Airbyte will begin pulling data from your source and pushing it to your Oracle DB destination.
9. Monitor the sync process to ensure that it is running smoothly and troubleshoot any issues that may arise.
10. Once the sync is complete, you can access your data in your Oracle DB and use it for analysis, reporting, or any other purposes.
Step 3: Set up a connection to sync your MongoDb data to Oracle

Once you've successfully connected MongoDb as a data source and Oracle as a destination in Airbyte, you can set up a data pipeline between them with the following steps:
- Create a new connection: On the Airbyte dashboard, navigate to the 'Connections' tab and click the '+ New Connection' button.
- Choose your source: Select MongoDb from the dropdown list of your configured sources.
- Select your destination: Choose Oracle from the dropdown list of your configured destinations.
- Configure your sync: Define the frequency of your data syncs based on your business needs. Airbyte allows both manual and automatic scheduling for your data refreshes.
- Select the data to sync: Choose the specific MongoDb objects you want to import data from towards Oracle. You can sync all data or select specific tables and fields.
- Select the sync mode for your streams: Choose between full refreshes or incremental syncs (with deduplication if you want), and this for all streams or at the stream level. Incremental is only available for streams that have a primary cursor.
- Test your connection: Click the 'Test Connection' button to make sure that your setup works. If the connection test is successful, save your configuration.
- Start the sync: If the test passes, click 'Set Up Connection'. Airbyte will start moving data from MongoDb to Oracle according to your settings.
Remember, Airbyte keeps your data in sync at the frequency you determine, ensuring your Oracle data warehouse is always up-to-date with your MongoDb data.
Method 2: Connecting Mongodb to oracle db manually
Moving data from MongoDB to Oracle Database without using third-party connectors or integrations involves exporting the data from MongoDB, transforming it into a format that Oracle can understand, and then importing it into the Oracle Database. Below is a step-by-step guide to achieve this:
Step 1: Export Data from MongoDB
1. Identify the Data to Export: Determine which collections you need to export from MongoDB.
2. Use `mongoexport`: Use the `mongoexport` utility to export the data from MongoDB to a JSON or CSV file. This tool is part of the MongoDB server installation.
For JSON:
```sh
mongoexport --db yourDatabaseName --collection yourCollectionName --out yourData.json
```
For CSV (you'll need to specify the fields):
```sh
mongoexport --db yourDatabaseName --collection yourCollectionName --type=csv --fields field1,field2 --out yourData.csv
```
Step 2: Prepare the Data for Oracle
1. Review Data Types: Check the MongoDB data types and map them to the corresponding Oracle data types.
2. Transform Data: If needed, write a script (in a language like Python, Perl, or even a shell script) to transform the data from the MongoDB export into a format suitable for Oracle. This may include:
- Converting BSON ObjectId to a string.
- Formatting dates to match Oracle's date format.
- Handling nested documents or arrays appropriately.
3. Create an Intermediate File: Save the transformed data into an intermediate CSV or SQL file that can be understood by Oracle.
Step 3: Set Up Oracle Database
1. Create Tables: Create the necessary tables in Oracle Database to hold the data. Make sure the columns match the data types of the transformed data.
```sql
CREATE TABLE your_table_name (
column1 datatype1,
column2 datatype2,
...
);
```
2. Prepare the Environment: Ensure that the Oracle environment is ready to receive the data. This may include setting up user permissions, tablespaces, and other necessary database configurations.
Step 4: Import Data into Oracle
1. Use SQL*Loader or External Tables: Decide whether to use SQL*Loader or Oracle External Tables for the import process.
- SQL*Loader: This utility allows you to load data from external files into tables of an Oracle database.
Create a control file that describes how the data is formatted and how it should be loaded into Oracle:
```sh
LOAD DATA
INFILE 'yourData.csv'
INTO TABLE your_table_name
FIELDS TERMINATED BY ',' OPTIONALLY ENCLOSED BY '"'
(column1, column2, ...)
```
Run SQL*Loader using the control file:
```sh
sqlldr userid=yourOracleUsername/yourOraclePassword@yourOracleDB control=yourControlFile.ctl
```
- External Tables: This feature allows you to query data from a flat file as though it is a regular table.
```sql
CREATE TABLE your_table_name (
column1 datatype1,
column2 datatype2,
...
)
ORGANIZATION EXTERNAL (
TYPE ORACLE_LOADER
DEFAULT DIRECTORY your_directory
ACCESS PARAMETERS (
RECORDS DELIMITED BY NEWLINE
FIELDS TERMINATED BY ',' OPTIONALLY ENCLOSED BY '"'
)
LOCATION ('yourData.csv')
);
```
After creating the external table, you can use `INSERT INTO ... SELECT FROM ...` to move the data into the actual table.
Step 5: Verify the Data Transfer
1. Check the Data: After the import process, verify that the data has been transferred correctly by running some queries on the Oracle tables.
```sql
SELECT * FROM your_table_name;
```
2. Check for Errors: Review any logs or error files generated by SQL*Loader or the External Tables process to ensure that all records have been imported successfully.
Step 6: Cleanup
1. Remove Temporary Files: After a successful import, remove any intermediate or temporary files that were created during the process.
2. Finalize the Database: Add any necessary indexes, constraints, or triggers to the Oracle tables to finalize the setup.
Notes:
- It's important to ensure that the character encoding is consistent between the exported data and the Oracle Database to avoid any issues with special characters.
- Always test the migration process with a subset of data before attempting to migrate the entire dataset.
- Back up your databases before beginning the migration process to prevent data loss in case of any issues.
- Since you are not using third-party connectors or integrations, you'll need to handle data type conversions and complex data structures manually, which can be error-prone and time-consuming. Ensure thorough testing and validation during each step.
By following these steps, you should be able to move data from MongoDB to Oracle Database without using third-party connectors or integrations. Remember that this process can be complex and may require custom scripting depending on the structure and size of your data.
Use Cases to transfer your MongoDb data to Oracle
Integrating data from MongoDb to Oracle provides several benefits. Here are a few use cases:
- Advanced Analytics: Oracle’s powerful data processing capabilities enable you to perform complex queries and data analysis on your MongoDb data, extracting insights that wouldn't be possible within MongoDb alone.
- Data Consolidation: If you're using multiple other sources along with MongoDb, syncing to Oracle allows you to centralize your data for a holistic view of your operations, and to set up a change data capture process so you never have any discrepancies in your data again.
- Historical Data Analysis: MongoDb has limits on historical data. Syncing data to Oracle allows for long-term data retention and analysis of historical trends over time.
- Data Security and Compliance: Oracle provides robust data security features. Syncing MongoDb data to Oracle ensures your data is secured and allows for advanced data governance and compliance management.
- Scalability: Oracle can handle large volumes of data without affecting performance, providing an ideal solution for growing businesses with expanding MongoDb data.
- Data Science and Machine Learning: By having MongoDb data in Oracle, you can apply machine learning models to your data for predictive analytics, customer segmentation, and more.
- Reporting and Visualization: While MongoDb provides reporting tools, data visualization tools like Tableau, PowerBI, Looker (Google Data Studio) can connect to Oracle, providing more advanced business intelligence options. If you have a MongoDb table that needs to be converted to a Oracle table, Airbyte can do that automatically.
Wrapping Up
To summarize, this tutorial has shown you how to:
- Configure a MongoDb account as an Airbyte data source connector.
- Configure Oracle as a data destination connector.
- Create an Airbyte data pipeline that will automatically be moving data directly from MongoDb to Oracle after you set a schedule
With Airbyte, creating data pipelines take minutes, and the data integration possibilities are endless. Airbyte supports the largest catalog of API tools, databases, and files, among other sources. Airbyte's connectors are open-source, so you can add any custom objects to the connector, or even build a new connector from scratch without any local dev environment or any data engineer within 10 minutes with the no-code connector builder.
We look forward to seeing you make use of it! We invite you to join the conversation on our community Slack Channel, or sign up for our newsletter. You should also check out other Airbyte tutorials, and Airbyte’s content hub!
What should you do next?
Hope you enjoyed the reading. Here are the 3 ways we can help you in your data journey:
Ready to get started?
Frequently Asked Questions
MongoDB gives access to a wide range of data types, including:
1. Documents: MongoDB stores data in the form of documents, which are similar to JSON objects. Each document contains a set of key-value pairs that represent the data.
2. Collections: A collection is a group of related documents that are stored together in MongoDB. Collections can be thought of as tables in a relational database.
3. Indexes: MongoDB supports various types of indexes, including single-field, compound, and geospatial indexes. Indexes are used to improve query performance.
4. GridFS: MongoDB's GridFS is a specification for storing and retrieving large files, such as images and videos, in MongoDB.
5. Aggregation: MongoDB's aggregation framework provides a way to perform complex data analysis operations, such as grouping, filtering, and sorting, on large datasets.
6. Transactions: MongoDB supports multi-document transactions, which allow multiple operations to be performed atomically.
7. Change streams: MongoDB's change streams provide a way to monitor changes to data in real-time, allowing applications to react to changes as they occur.
Overall, MongoDB provides access to a flexible and powerful data model that can handle a wide range of data types and use cases.
What should you do next?
Hope you enjoyed the reading. Here are the 3 ways we can help you in your data journey: