Salesforce is a cloud-based customer relationship management (CRM) platform providing business solutions software on a subscription basis. Salesforce is a huge force in the ecommerce world, helping businesses with marketing, commerce, service and sales, and enabling enterprises’ IT teams to collaborate easily from anywhere. Salesforces is the force behind many industries, offering healthcare, automotive, finance, media, communications, and manufacturing multichannel support. Its services are wide-ranging, with access to customer, partner, and developer communities as well as an app exchange marketplace.
Microsoft SQL Server is a relational database management (RDBMS) built by Microsoft. As a database server, its primary function is to store and retrieve data upon the request of other software applications, either from the same computer or a different computer across a network—including the internet. To serve the needs of different audiences and workload sizes, Microsoft offers multiple editions (at least 12) of its Microsoft SQL Server.
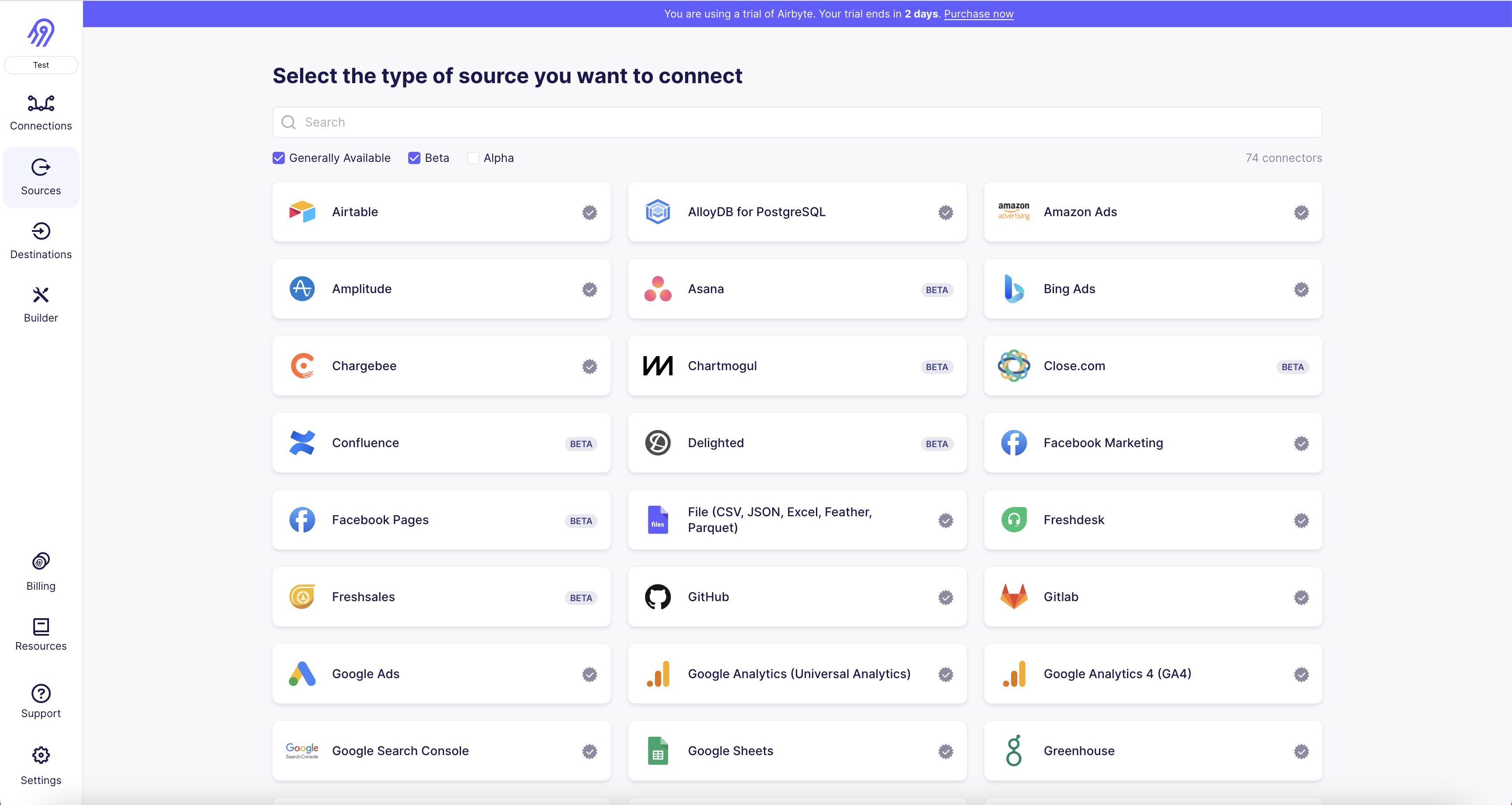
1. Open the Airbyte platform and navigate to the "Sources" tab on the left-hand side of the screen.
2. Click on the "Salesforce" source connector and select "Create new connection."
3. Enter a name for your connection and click "Next."
4. Enter your Salesforce credentials, including your username, password, and security token.
5. Click "Test connection" to ensure that your credentials are correct and that Airbyte can connect to your Salesforce account.
6. Once the connection is successful, select the objects you want to replicate from Salesforce.
7. Choose the replication frequency and any other settings you want to apply to your connection.
8. Click "Create connection" to save your settings and start replicating data from Salesforce to Airbyte.
9. You can monitor the progress of your replication in the "Connections" tab and view the data in the "Dashboard" tab.

1. Open the Airbyte platform and navigate to the "Destinations" tab on the left-hand side of the screen.
2. Scroll down until you find the "MSSQL - SQL Server" connector and click on it.
3. Click on the "Create new destination" button.
4. Fill in the required information, including the destination name, host, port, database name, username, and password.
5. Click on the "Test connection" button to ensure that the connection is successful.
6. Once the connection is successful, click on the "Save" button to save the destination.
7. Navigate to the "Sources" tab on the left-hand side of the screen and select the source that you want to connect to the MSSQL - SQL Server destination.
8. Click on the "Create new connection" button.
9. Select the MSSQL - SQL Server destination that you just created from the drop-down menu.
10. Fill in the required information for the source, including the source name, host, port, database name, username, and password.
11. Click on the "Test connection" button to ensure that the connection is successful.
12. Once the connection is successful, click on the "Save" button to save the connection.13. You can now start syncing data from your source to your MSSQL - SQL Server destination.

With Airbyte, creating data pipelines take minutes, and the data integration possibilities are endless. Airbyte supports the largest catalog of API tools, databases, and files, among other sources. Airbyte's connectors are open-source, so you can add any custom objects to the connector, or even build a new connector from scratch without any local dev environment or any data engineer within 10 minutes with the no-code connector builder.
We look forward to seeing you make use of it! We invite you to join the conversation on our community Slack Channel, or sign up for our newsletter. You should also check out other Airbyte tutorials, and Airbyte’s content hub!
What should you do next?
Hope you enjoyed the reading. Here are the 3 ways we can help you in your data journey:
TL;DR
This can be done by building a data pipeline manually, usually a Python script (you can leverage a tool as Apache Airflow for this). This process can take more than a full week of development. Or it can be done in minutes on Airbyte in three easy steps:
- set up Salesforce as a source connector (using Auth, or usually an API key)
- set up MS SQL Server as a destination connector
- define which data you want to transfer and how frequently
You can choose to self-host the pipeline using Airbyte Open Source or have it managed for you with Airbyte Cloud.
This tutorial’s purpose is to show you how.
What is Salesforce
Salesforce is a cloud-based customer relationship management (CRM) platform providing business solutions software on a subscription basis. Salesforce is a huge force in the ecommerce world, helping businesses with marketing, commerce, service and sales, and enabling enterprises’ IT teams to collaborate easily from anywhere. Salesforces is the force behind many industries, offering healthcare, automotive, finance, media, communications, and manufacturing multichannel support. Its services are wide-ranging, with access to customer, partner, and developer communities as well as an app exchange marketplace.
What is MS SQL Server
Microsoft SQL Server is a relational database management (RDBMS) built by Microsoft. As a database server, its primary function is to store and retrieve data upon the request of other software applications, either from the same computer or a different computer across a network—including the internet. To serve the needs of different audiences and workload sizes, Microsoft offers multiple editions (at least 12) of its Microsoft SQL Server.
{{COMPONENT_CTA}}
Prerequisites
- A Salesforce account to transfer your customer data automatically from.
- A MS SQL Server account.
- An active Airbyte Cloud account, or you can also choose to use Airbyte Open Source locally. You can follow the instructions to set up Airbyte on your system using docker-compose.
Airbyte is an open-source data integration platform that consolidates and streamlines the process of extracting and loading data from multiple data sources to data warehouses. It offers pre-built connectors, including Salesforce and MS SQL Server, for seamless data migration.
When using Airbyte to move data from Salesforce to MS SQL Server, it extracts data from Salesforce using the source connector, converts it into a format MS SQL Server can ingest using the provided schema, and then loads it into MS SQL Server via the destination connector. This allows businesses to leverage their Salesforce data for advanced analytics and insights within MS SQL Server, simplifying the ETL process and saving significant time and resources.
Methods to Move Data From Salesforce to mssql server
- Method 1: Connecting Salesforce to mssql server using Airbyte.
- Method 2: Connecting Salesforce to mssql server manually.
Method 1: Connecting Salesforce to mssql server using Airbyte
Step 1: Set up Salesforce as a source connector
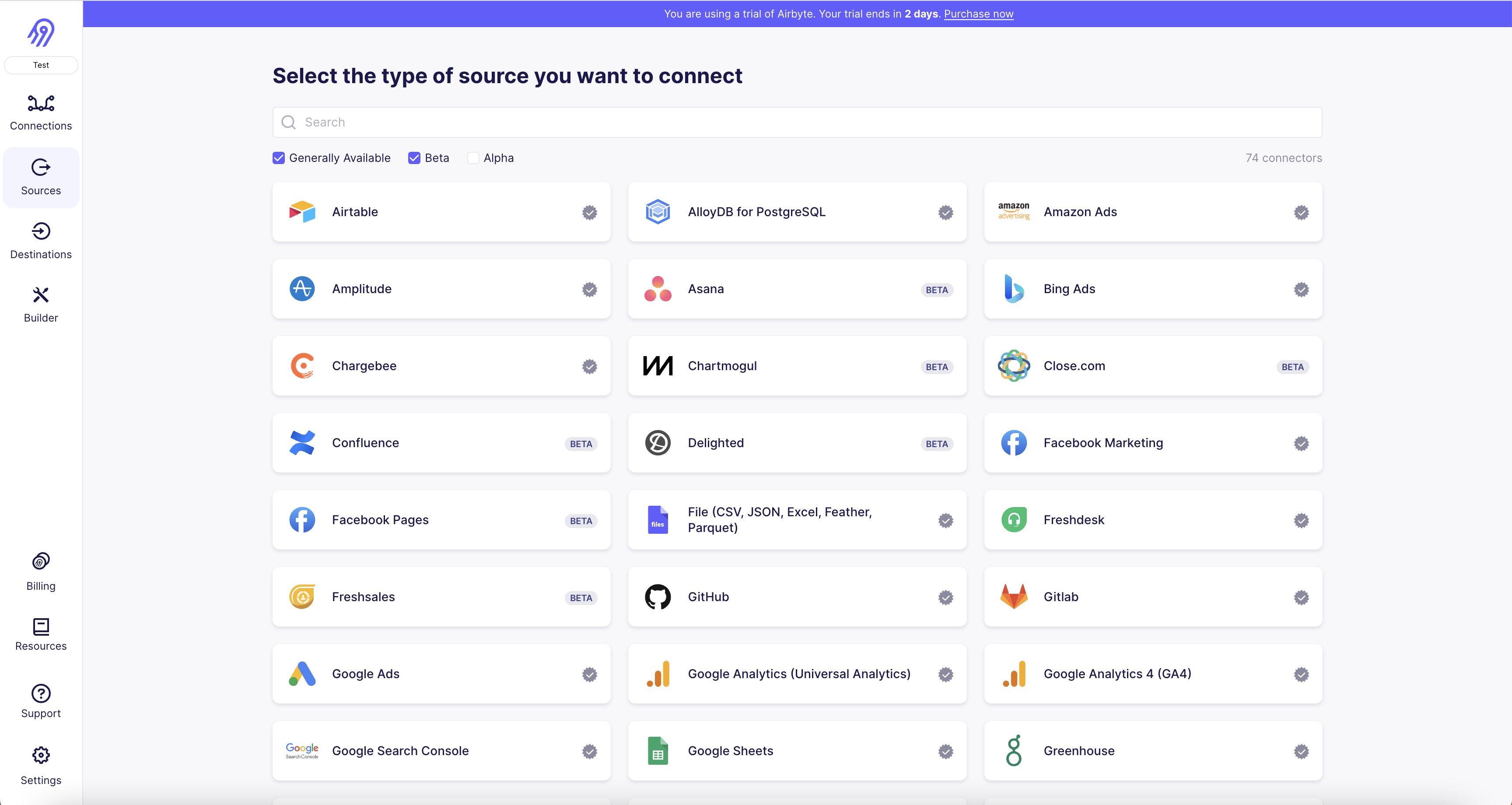
1. Open the Airbyte platform and navigate to the "Sources" tab on the left-hand side of the screen.
2. Click on the "Salesforce" source connector and select "Create new connection."
3. Enter a name for your connection and click "Next."
4. Enter your Salesforce credentials, including your username, password, and security token.
5. Click "Test connection" to ensure that your credentials are correct and that Airbyte can connect to your Salesforce account.
6. Once the connection is successful, select the objects you want to replicate from Salesforce.
7. Choose the replication frequency and any other settings you want to apply to your connection.
8. Click "Create connection" to save your settings and start replicating data from Salesforce to Airbyte.
9. You can monitor the progress of your replication in the "Connections" tab and view the data in the "Dashboard" tab.
Step 2: Set up MS SQL Server as a destination connector

1. Open the Airbyte platform and navigate to the "Destinations" tab on the left-hand side of the screen.
2. Scroll down until you find the "MSSQL - SQL Server" connector and click on it.
3. Click on the "Create new destination" button.
4. Fill in the required information, including the destination name, host, port, database name, username, and password.
5. Click on the "Test connection" button to ensure that the connection is successful.
6. Once the connection is successful, click on the "Save" button to save the destination.
7. Navigate to the "Sources" tab on the left-hand side of the screen and select the source that you want to connect to the MSSQL - SQL Server destination.
8. Click on the "Create new connection" button.
9. Select the MSSQL - SQL Server destination that you just created from the drop-down menu.
10. Fill in the required information for the source, including the source name, host, port, database name, username, and password.
11. Click on the "Test connection" button to ensure that the connection is successful.
12. Once the connection is successful, click on the "Save" button to save the connection.13. You can now start syncing data from your source to your MSSQL - SQL Server destination.
Step 3: Set up a connection to sync your Salesforce data to MS SQL Server

Once you've successfully connected Salesforce as a data source and MS SQL Server as a destination in Airbyte, you can set up a data pipeline between them with the following steps:
- Create a new connection: On the Airbyte dashboard, navigate to the 'Connections' tab and click the '+ New Connection' button.
- Choose your source: Select Salesforce from the dropdown list of your configured sources.
- Select your destination: Choose MS SQL Server from the dropdown list of your configured destinations.
- Configure your sync: Define the frequency of your data syncs based on your business needs. Airbyte allows both manual and automatic scheduling for your data refreshes.
- Select the data to sync: Choose the specific Salesforce objects you want to import data from towards MS SQL Server. You can sync all data or select specific tables and fields.
- Select the sync mode for your streams: Choose between full refreshes or incremental syncs (with deduplication if you want), and this for all streams or at the stream level. Incremental is only available for streams that have a primary cursor.
- Test your connection: Click the 'Test Connection' button to make sure that your setup works. If the connection test is successful, save your configuration.
- Start the sync: If the test passes, click 'Set Up Connection'. Airbyte will start moving data from Salesforce to MS SQL Server according to your settings.
Remember, Airbyte keeps your data in sync at the frequency you determine, ensuring your MS SQL Server data warehouse is always up-to-date with your Salesforce data.
Method 2: Connecting Salesforce to mssql server manually
Moving data from Salesforce to MS SQL Server without third-party connectors or integrations involves several steps. You'll need to use Salesforce's data export capabilities and SQL Server's data import tools. Below is a step-by-step guide to accomplishing this task:
Step 1: Plan the Data Migration
1. Identify the Data to Migrate: Determine which objects and fields from Salesforce you need to move to SQL Server.
2. Schema Mapping: Map the Salesforce data types to the corresponding SQL Server data types.
3. Prepare the SQL Server Database: Create tables in your SQL Server database that will receive the data from Salesforce.
Step 2: Export Data from Salesforce
1. Login to Salesforce: Log in to your Salesforce account with the necessary permissions to access the data.
2. Data Export Service: Navigate to Setup, enter "Data Export" in the Quick Find box, and then select "Data Export."
3. Schedule Export: Choose to either export immediately or schedule an export. Salesforce allows you to manually export data once every 48 hours or schedule an automatic export every week or month.
4. Select Data: Choose the objects and fields you want to export.
5. Export Data: Confirm the export. Salesforce will prepare a series of CSV files containing your data.
6. Download Exported Files: Once the export is ready, download the CSV files to your local machine.
Step 3: Prepare the Data for Import
1. Inspect the CSV Files: Check the CSV files for any inconsistencies or data that may need transformation before importing into SQL Server.
2. Clean the Data: If necessary, clean the data using a spreadsheet program or a scripting language like Python.
3. Format Dates and Times: Ensure that date and time formats match the SQL Server requirements.
Step 4: Import Data into SQL Server
1. Open SQL Server Management Studio (SSMS): Open SSMS and connect to your SQL Server instance.
2. Create a New Database: If you haven't already, create a new database to hold the Salesforce data.
3. Use the Import Wizard:
- Right-click on the database where you want to import the data.
- Select "Tasks" > "Import Data..." to open the SQL Server Import and Export Wizard.
4. Choose a Data Source:
- For the data source, select "Flat File Source" for the CSV files.
- Browse and select the CSV file you want to import.
- Configure the flat file source properties to match the CSV file format, such as text qualifier, column delimiter, and so on.
5. Choose a Destination:
- For the destination, select "SQL Server Native Client" and connect to the target database.
6. Map the Columns:
- Map the source columns in the CSV file to the target columns in the SQL Server database tables.
- Make sure the data types are compatible.
7. Run the Package:
- Review the mappings and other settings.
- Execute the package to start the import process.
- Monitor the process for any errors or warnings that may occur.
Step 5: Verify the Data Migration
1. Check the Data: Once the import is complete, run queries against the tables in SQL Server to ensure the data was imported correctly.
2. Validate Record Counts: Compare the record counts in Salesforce with the counts in SQL Server to ensure completeness.
3. Perform Data Quality Checks: Look for any discrepancies in the data and correct them if necessary.
Step 6: Automate the Process (Optional)
If this is a recurring task, consider automating the process using SQL Server Integration Services (SSIS) or PowerShell scripts to handle the data extraction, transformation, and loading (ETL) process.
Step 7: Clean Up
1. Archive the CSV Files: Store the CSV files in a secure location for backup purposes or delete them if they are no longer needed.
2. Review Security: Ensure that the data in SQL Server has the appropriate security measures, such as access controls and encryption, if necessary.
Notes:
- Always back up your data before starting the migration process.
- Be aware of the limitations of Salesforce data exports, such as the frequency of exports and the volume of data.
- Make sure you comply with any data governance policies and regulations when handling sensitive information.
- Test the migration process with a small subset of data before doing a full migration.
By following these steps, you should be able to move data from Salesforce to MS SQL Server without using third-party connectors or integrations. Remember that this process can be complex and time-consuming, so it's important to plan and test thoroughly.
Use Cases to transfer your Salesforce data to MS SQL Server
Integrating data from Salesforce to MS SQL Server provides several benefits. Here are a few use cases:
- Advanced Analytics: MS SQL Server’s powerful data processing capabilities enable you to perform complex queries and data analysis on your Salesforce data, extracting insights that wouldn't be possible within Salesforce alone.
- Data Consolidation: If you're using multiple other sources along with Salesforce, syncing to MS SQL Server allows you to centralize your data for a holistic view of your operations, and to set up a change data capture process so you never have any discrepancies in your data again.
- Historical Data Analysis: Salesforce has limits on historical data. Syncing data to MS SQL Server allows for long-term data retention and analysis of historical trends over time.
- Data Security and Compliance: MS SQL Server provides robust data security features. Syncing Salesforce data to MS SQL Server ensures your data is secured and allows for advanced data governance and compliance management.
- Scalability: MS SQL Server can handle large volumes of data without affecting performance, providing an ideal solution for growing businesses with expanding Salesforce data.
- Data Science and Machine Learning: By having Salesforce data in MS SQL Server, you can apply machine learning models to your data for predictive analytics, customer segmentation, and more.
- Reporting and Visualization: While Salesforce provides reporting tools, data visualization tools like Tableau, PowerBI, Looker (Google Data Studio) can connect to MS SQL Server, providing more advanced business intelligence options. If you have a Salesforce table that needs to be converted to a MS SQL Server table, Airbyte can do that automatically.
Wrapping Up
To summarize, this tutorial has shown you how to:
- Configure a Salesforce account as an Airbyte data source connector.
- Configure MS SQL Server as a data destination connector.
- Create an Airbyte data pipeline that will automatically be moving data directly from Salesforce to MS SQL Server after you set a schedule
With Airbyte, creating data pipelines take minutes, and the data integration possibilities are endless. Airbyte supports the largest catalog of API tools, databases, and files, among other sources. Airbyte's connectors are open-source, so you can add any custom objects to the connector, or even build a new connector from scratch without any local dev environment or any data engineer within 10 minutes with the no-code connector builder.
We look forward to seeing you make use of it! We invite you to join the conversation on our community Slack Channel, or sign up for our newsletter. You should also check out other Airbyte tutorials, and Airbyte’s content hub!
What should you do next?
Hope you enjoyed the reading. Here are the 3 ways we can help you in your data journey:
Ready to get started?
Frequently Asked Questions
Salesforce's API provides access to a wide range of data types, including:
1. Accounts: Information about customer accounts, including contact details, billing information, and purchase history.
2. Leads: Data on potential customers, including contact information, lead source, and lead status.
3. Opportunities: Information on potential sales deals, including deal size, stage, and probability of closing.
4. Contacts: Details on individual contacts associated with customer accounts, including contact information and activity history.
5. Cases: Information on customer service cases, including case details, status, and resolution.
6. Products: Data on products and services offered by the company, including pricing, availability, and product descriptions.
7. Campaigns: Information on marketing campaigns, including campaign details, status, and results.
8. Reports and Dashboards: Access to pre-built and custom reports and dashboards that provide insights into sales, marketing, and customer service performance.
9. Custom Objects: Ability to access and manipulate custom objects created by the organization to store specific types of data.
Overall, Salesforce's API provides access to a comprehensive set of data types that enable organizations to manage and analyze their customer relationships, sales processes, and marketing campaigns.