

.png)
Building your pipeline or Using Airbyte
Airbyte is the only open source solution empowering data teams to meet all their growing custom business demands in the new AI era.
- Inconsistent and inaccurate data
- Laborious and expensive
- Brittle and inflexible
- Reliable and accurate
- Extensible and scalable for all your needs
- Deployed and governed your way
Start syncing with Airbyte in 3 easy steps within 10 minutes



Take a virtual tour
Demo video of Airbyte Cloud
Demo video of AI Connector Builder
What sets Airbyte Apart
Modern GenAI Workflows
Move Large Volumes, Fast
An Extensible Open-Source Standard
Full Control & Security
Fully Featured & Integrated
Enterprise Support with SLAs
What our users say

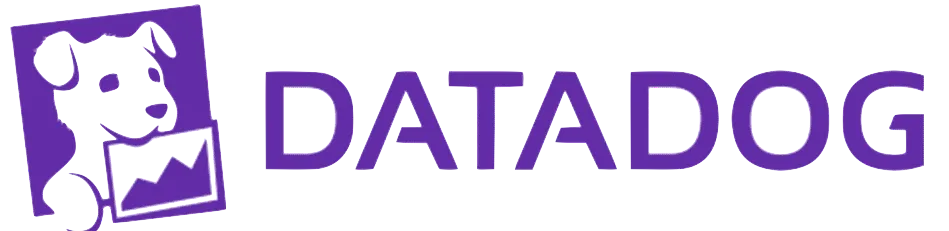
"The intake layer of Datadog’s self-serve analytics platform is largely built on Airbyte.Airbyte’s ease of use and extensibility allowed any team in the company to push their data into the platform - without assistance from the data team!"


“Airbyte helped us accelerate our progress by years, compared to our competitors. We don’t need to worry about connectors and focus on creating value for our users instead of building infrastructure. That’s priceless. The time and energy saved allows us to disrupt and grow faster.”
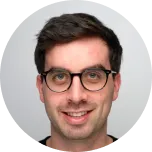

“We chose Airbyte for its ease of use, its pricing scalability and its absence of vendor lock-in. Having a lean team makes them our top criteria. The value of being able to scale and execute at a high level by maximizing resources is immense”
Prerequisites
- You need an active Sentry account with access to the project you want to export data from.
- You should have an API key or an authentication token to access Sentry’s API.
- Basic knowledge of Python programming and working with APIs is required.
- Log in to your Sentry account.
- Navigate to Settings > Developer Settings.
- Under Internal Integrations, click on New Internal Integration.
- Fill in the details, set the necessary permissions, and create the integration.
- Copy the generated token; you’ll need it to authenticate API requests.
- Go to Sentry’s API documentation and familiarize yourself with the endpoints you need to use.
- For instance, to retrieve a list of events, you might use the GET /api/0/projects/{organization_slug}/{project_slug}/events/ endpoint.
- Create a new Python file, e.g., sentry_to_csv.py.
- Import the necessary modules:
import requests
import csv
import json
- Define the Sentry API endpoint and your credentials:
sentry_api_url = "https://sentry.io/api/0/projects/{organization_slug}/{project_slug}/events/"
auth_token = "YOUR_AUTH_TOKEN"
headers = {
"Authorization": f"Bearer {auth_token}"
}
- Write a function to retrieve the data:
def get_sentry_data(url):
response = requests.get(url, headers=headers)
if response.status_code == 200:
return response.json()
else:
raise Exception(f"Error fetching data: {response.status_code}")
- Decide on the fields you want to export to CSV.
- Write a function to parse the JSON data and write it to a CSV file:
def write_to_csv(data, filename):
with open(filename, mode='w', newline='', encoding='utf-8') as file:
writer = csv.writer(file)
# Write the header
writer.writerow(['Event ID', 'Issue ID', 'Timestamp', 'Level', 'Message'])
# Write the data rows
for event in data:
writer.writerow([
event['eventID'],
event['groupID'],
event['dateCreated'],
event['level'],
event.get('message', 'No message provided')
])
- Call the functions in the appropriate order:
if __name__ == "__main__":
sentry_data = get_sentry_data(sentry_api_url)
write_to_csv(sentry_data, 'sentry_events.csv')
- Run your Python script:
python sentry_to_csv.py
Check the generated sentry_events.csv file to ensure that the data has been exported correctly.
Additional Considerations
- Sentry’s API may have rate limits; handle these in your script.
- For large datasets, you may need to implement pagination in your API requests.
- Make sure to handle any potential exceptions or errors in your script.
- If you need to export more complex data structures, you may need to flatten the JSON before writing it to CSV.
- Always ensure that you comply with Sentry’s terms of service and privacy policies when handling data.
FAQs
What is ETL?
ETL, an acronym for Extract, Transform, Load, is a vital data integration process. It involves extracting data from diverse sources, transforming it into a usable format, and loading it into a database, data warehouse or data lake. This process enables meaningful data analysis, enhancing business intelligence.
Sentry is a cloud-based error monitoring platform that helps developers identify and fix issues in their applications. It provides real-time alerts and detailed error reports, allowing developers to quickly diagnose and resolve issues before they impact users. Sentry supports a wide range of programming languages and frameworks, and integrates with popular development tools like GitHub, Jira, and Slack. With features like release tracking, performance monitoring, and customizable dashboards, Sentry helps teams improve the quality and reliability of their software. Overall, Sentry is a powerful tool for any development team looking to streamline their error monitoring and debugging processes.
Sentry's API provides access to a wide range of data related to application performance monitoring and error tracking. The following are the categories of data that can be accessed through Sentry's API:
1. Events: This includes information about errors, crashes, and other events that occur within an application.
2. Issues: This includes details about specific issues that have been identified within an application, including the number of occurrences, the severity of the issue, and any associated metadata.
3. Projects: This includes information about the projects being monitored by Sentry, including project settings, integrations, and other configuration details.
4. Users: This includes information about the users who are interacting with an application, including their IP addresses, browser information, and other relevant data.
5. Releases: This includes information about the releases of an application, including version numbers, release dates, and associated metadata.
6. Performance: This includes data related to the performance of an application, including response times, error rates, and other metrics.
Overall, Sentry's API provides a comprehensive set of data that can be used to monitor and optimize the performance of an application, as well as to identify and resolve errors and other issues.
What is ELT?
ELT, standing for Extract, Load, Transform, is a modern take on the traditional ETL data integration process. In ELT, data is first extracted from various sources, loaded directly into a data warehouse, and then transformed. This approach enhances data processing speed, analytical flexibility and autonomy.
Difference between ETL and ELT?
ETL and ELT are critical data integration strategies with key differences. ETL (Extract, Transform, Load) transforms data before loading, ideal for structured data. In contrast, ELT (Extract, Load, Transform) loads data before transformation, perfect for processing large, diverse data sets in modern data warehouses. ELT is becoming the new standard as it offers a lot more flexibility and autonomy to data analysts.
What should you do next?
Hope you enjoyed the reading. Here are the 3 ways we can help you in your data journey: