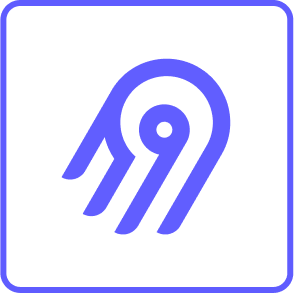

Building your pipeline or Using Airbyte
Airbyte is the only open source solution empowering data teams to meet all their growing custom business demands in the new AI era.
- Inconsistent and inaccurate data
- Laborious and expensive
- Brittle and inflexible
- Reliable and accurate
- Extensible and scalable for all your needs
- Deployed and governed your way
Start syncing with Airbyte in 3 easy steps within 10 minutes



Take a virtual tour
Demo video of Airbyte Cloud
Demo video of AI Connector Builder
What sets Airbyte Apart
Modern GenAI Workflows
Move Large Volumes, Fast
An Extensible Open-Source Standard
Full Control & Security
Fully Featured & Integrated
Enterprise Support with SLAs
What our users say
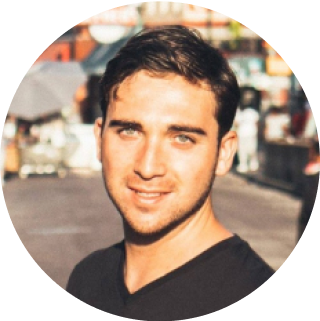
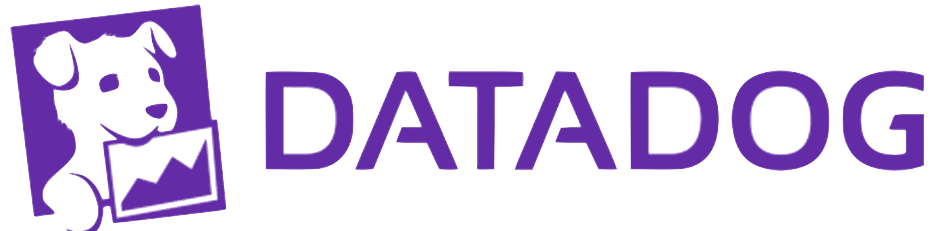
"The intake layer of Datadog’s self-serve analytics platform is largely built on Airbyte.Airbyte’s ease of use and extensibility allowed any team in the company to push their data into the platform - without assistance from the data team!"
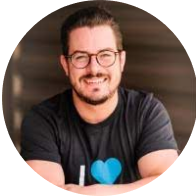
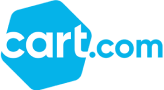
“Airbyte helped us accelerate our progress by years, compared to our competitors. We don’t need to worry about connectors and focus on creating value for our users instead of building infrastructure. That’s priceless. The time and energy saved allows us to disrupt and grow faster.”
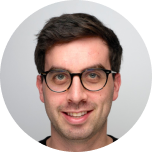

“We chose Airbyte for its ease of use, its pricing scalability and its absence of vendor lock-in. Having a lean team makes them our top criteria. The value of being able to scale and execute at a high level by maximizing resources is immense”
FAQs
What is ETL?
ETL, an acronym for Extract, Transform, Load, is a vital data integration process. It involves extracting data from diverse sources, transforming it into a usable format, and loading it into a database, data warehouse or data lake. This process enables meaningful data analysis, enhancing business intelligence.
Twitter is owned by American company based in San Francisco, California, which permits users to microblog, post videos, and social networking service. Twitter is a popular social networking platform that permits its users to send and read micro-blogs of up to 280-characters well known as “tweets”. Basically, Twitter is needed to be at most 140 characters long, and these messages are generally broadcast to all the users on Twitter. Twitter rolled out a paid verification system and laid off thousands of content moderators for the troubled social media platform.
Twitter's API provides access to a wide range of data, including:
1. Tweets: The API allows access to all public tweets, as well as tweets from specific users or containing specific keywords.
2. User data: This includes information about individual Twitter users, such as their profile information, follower and following counts, and tweet history.
3. Trends: The API provides access to real-time and historical data on trending topics and hashtags.
4. Analytics: Twitter's API also provides access to analytics data, such as engagement rates, impressions, and reach.
5. Lists: The API allows access to Twitter lists, which are curated groups of Twitter users.
6. Direct messages: The API provides access to direct messages sent between Twitter users.
7. Search: The API allows for advanced search queries, including filtering by location, language, and sentiment.
8. Ads: Twitter's API also provides access to advertising data, such as campaign performance metrics and targeting options.
Overall, Twitter's API provides a wealth of data that can be used for a variety of purposes, from social media monitoring to marketing and advertising.
What is ELT?
ELT, standing for Extract, Load, Transform, is a modern take on the traditional ETL data integration process. In ELT, data is first extracted from various sources, loaded directly into a data warehouse, and then transformed. This approach enhances data processing speed, analytical flexibility and autonomy.
Difference between ETL and ELT?
ETL and ELT are critical data integration strategies with key differences. ETL (Extract, Transform, Load) transforms data before loading, ideal for structured data. In contrast, ELT (Extract, Load, Transform) loads data before transformation, perfect for processing large, diverse data sets in modern data warehouses. ELT is becoming the new standard as it offers a lot more flexibility and autonomy to data analysts.
What should you do next?
Hope you enjoyed the reading. Here are the 3 ways we can help you in your data journey:
Twitter is owned by American company based in San Francisco, California, which permits users to microblog, post videos, and social networking service. Twitter is a popular social networking platform that permits its users to send and read micro-blogs of up to 280-characters well known as “tweets”. Basically, Twitter is needed to be at most 140 characters long, and these messages are generally broadcast to all the users on Twitter. Twitter rolled out a paid verification system and laid off thousands of content moderators for the troubled social media platform.
An AWS Data Lake is a centralized repository that allows you to store all your structured and unstructured data at any scale. It is designed to handle massive amounts of data from various sources, such as databases, applications, IoT devices, and more. With AWS Data Lake, you can easily ingest, store, catalog, process, and analyze data using a wide range of AWS services like Amazon S3, Amazon Athena, AWS Glue, and Amazon EMR. This allows you to build data lakes for machine learning, big data analytics, and data warehousing workloads. AWS Data Lake provides a secure, scalable, and cost-effective solution for managing your organization's data.
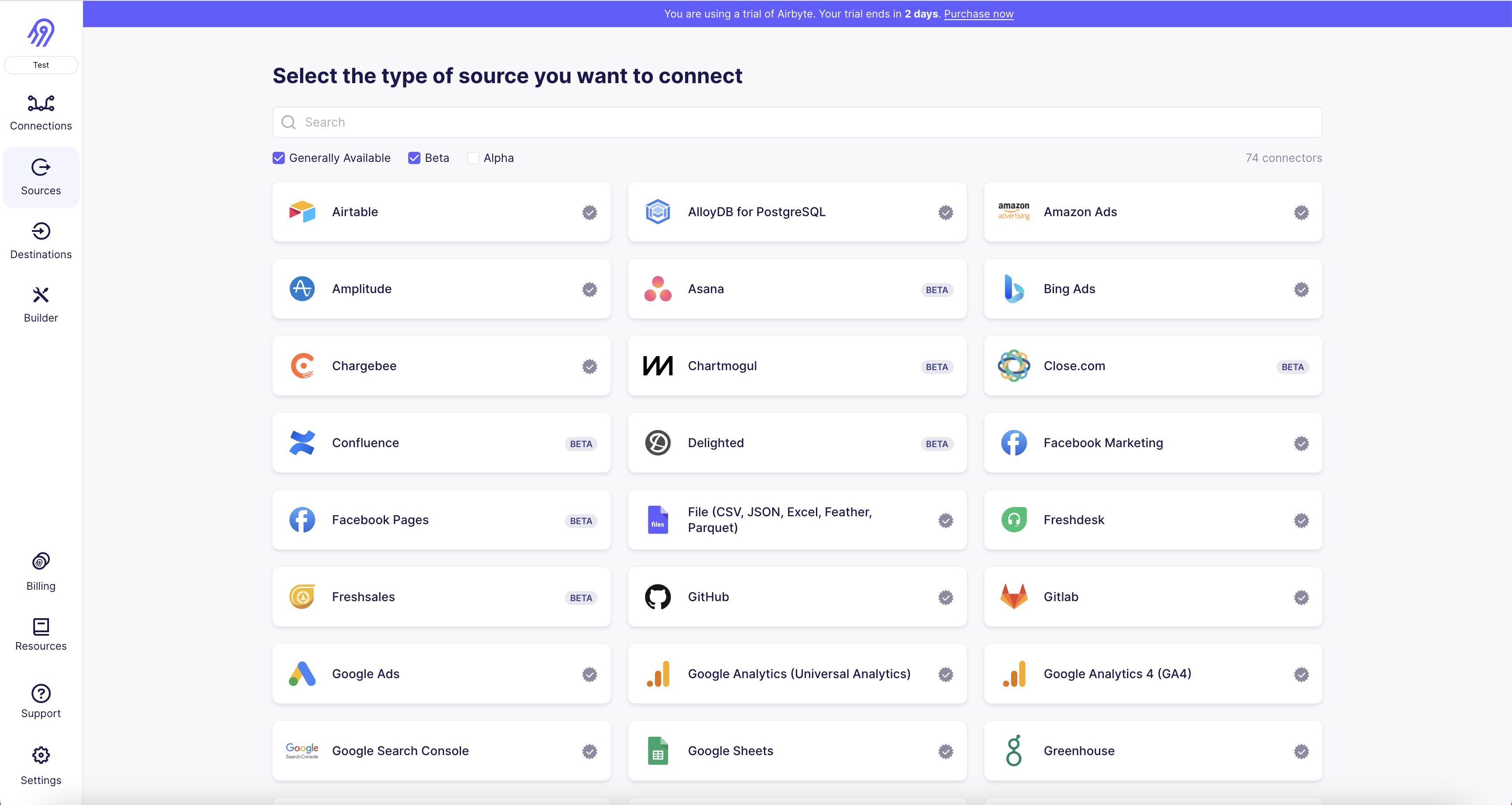
1. Open the Airbyte platform and navigate to the "Sources" tab on the left-hand side of the screen.
2. Click on the "Twitter" source connector and select "Create new connection."
3. Enter a name for your connection and click "Next."
4. Enter your Twitter API credentials, including your Consumer Key, Consumer Secret, Access Token, and Access Token Secret. You can find these credentials by logging into your Twitter Developer account and navigating to the "Keys and Tokens" tab.
5. Once you have entered your credentials, click "Test Connection" to ensure that Airbyte can successfully connect to your Twitter account.
6. If the connection is successful, click "Create" to save your connection.
7. You can now use your Twitter source connector to extract data from your Twitter account. Simply select your connection and choose the data you want to extract, such as tweets, followers, or mentions. You can also set up a schedule to automatically extract data at regular intervals.

1. Log in to your AWS account and navigate to the AWS Management Console.
2. Click on the S3 service and create a new bucket where you will store your data.
3. Create an IAM user with the necessary permissions to access the S3 bucket. Make sure to save the access key and secret key.
4. Open Airbyte and navigate to the Destinations tab.
5. Select the AWS Datalake destination connector and click on "Create new connection".
6. Enter a name for your connection and paste the access key and secret key you saved earlier.
7. Enter the name of the S3 bucket you created in step 2 and select the region where it is located.
8. Choose the format in which you want your data to be stored in the S3 bucket (e.g. CSV, JSON, Parquet).
9. Configure any additional settings, such as compression or encryption, if necessary.
10. Test the connection to make sure it is working properly.
11. Save the connection and start syncing your data to the AWS Datalake.

With Airbyte, creating data pipelines take minutes, and the data integration possibilities are endless. Airbyte supports the largest catalog of API tools, databases, and files, among other sources. Airbyte's connectors are open-source, so you can add any custom objects to the connector, or even build a new connector from scratch without any local dev environment or any data engineer within 10 minutes with the no-code connector builder.
We look forward to seeing you make use of it! We invite you to join the conversation on our community Slack Channel, or sign up for our newsletter. You should also check out other Airbyte tutorials, and Airbyte’s content hub!
What should you do next?
Hope you enjoyed the reading. Here are the 3 ways we can help you in your data journey:
TL;DR
This can be done by building a data pipeline manually, usually a Python script (you can leverage a tool as Apache Airflow for this). This process can take more than a full week of development. Or it can be done in minutes on Airbyte in three easy steps:
- set up Twitter as a source connector (using Auth, or usually an API key)
- set up AWS Datalake as a destination connector
- define which data you want to transfer and how frequently
You can choose to self-host the pipeline using Airbyte Open Source or have it managed for you with Airbyte Cloud.
This tutorial’s purpose is to show you how.
What is Twitter
Twitter is owned by American company based in San Francisco, California, which permits users to microblog, post videos, and social networking service. Twitter is a popular social networking platform that permits its users to send and read micro-blogs of up to 280-characters well known as “tweets”. Basically, Twitter is needed to be at most 140 characters long, and these messages are generally broadcast to all the users on Twitter. Twitter rolled out a paid verification system and laid off thousands of content moderators for the troubled social media platform.
What is AWS Datalake
An AWS Data Lake is a centralized repository that allows you to store all your structured and unstructured data at any scale. It is designed to handle massive amounts of data from various sources, such as databases, applications, IoT devices, and more. With AWS Data Lake, you can easily ingest, store, catalog, process, and analyze data using a wide range of AWS services like Amazon S3, Amazon Athena, AWS Glue, and Amazon EMR. This allows you to build data lakes for machine learning, big data analytics, and data warehousing workloads. AWS Data Lake provides a secure, scalable, and cost-effective solution for managing your organization's data.
{{COMPONENT_CTA}}
Prerequisites
- A Twitter account to transfer your customer data automatically from.
- A AWS Datalake account.
- An active Airbyte Cloud account, or you can also choose to use Airbyte Open Source locally. You can follow the instructions to set up Airbyte on your system using docker-compose.
Airbyte is an open-source data integration platform that consolidates and streamlines the process of extracting and loading data from multiple data sources to data warehouses. It offers pre-built connectors, including Twitter and AWS Datalake, for seamless data migration.
When using Airbyte to move data from Twitter to AWS Datalake, it extracts data from Twitter using the source connector, converts it into a format AWS Datalake can ingest using the provided schema, and then loads it into AWS Datalake via the destination connector. This allows businesses to leverage their Twitter data for advanced analytics and insights within AWS Datalake, simplifying the ETL process and saving significant time and resources.
Methods to Move Data From Twitter to datalake
- Method 1: Connecting Twitter to datalake using Airbyte.
- Method 2: Connecting Twitter to datalake manually.
Method 1: Connecting Twitter to datalake using Airbyte
Step 1: Set up Twitter as a source connector
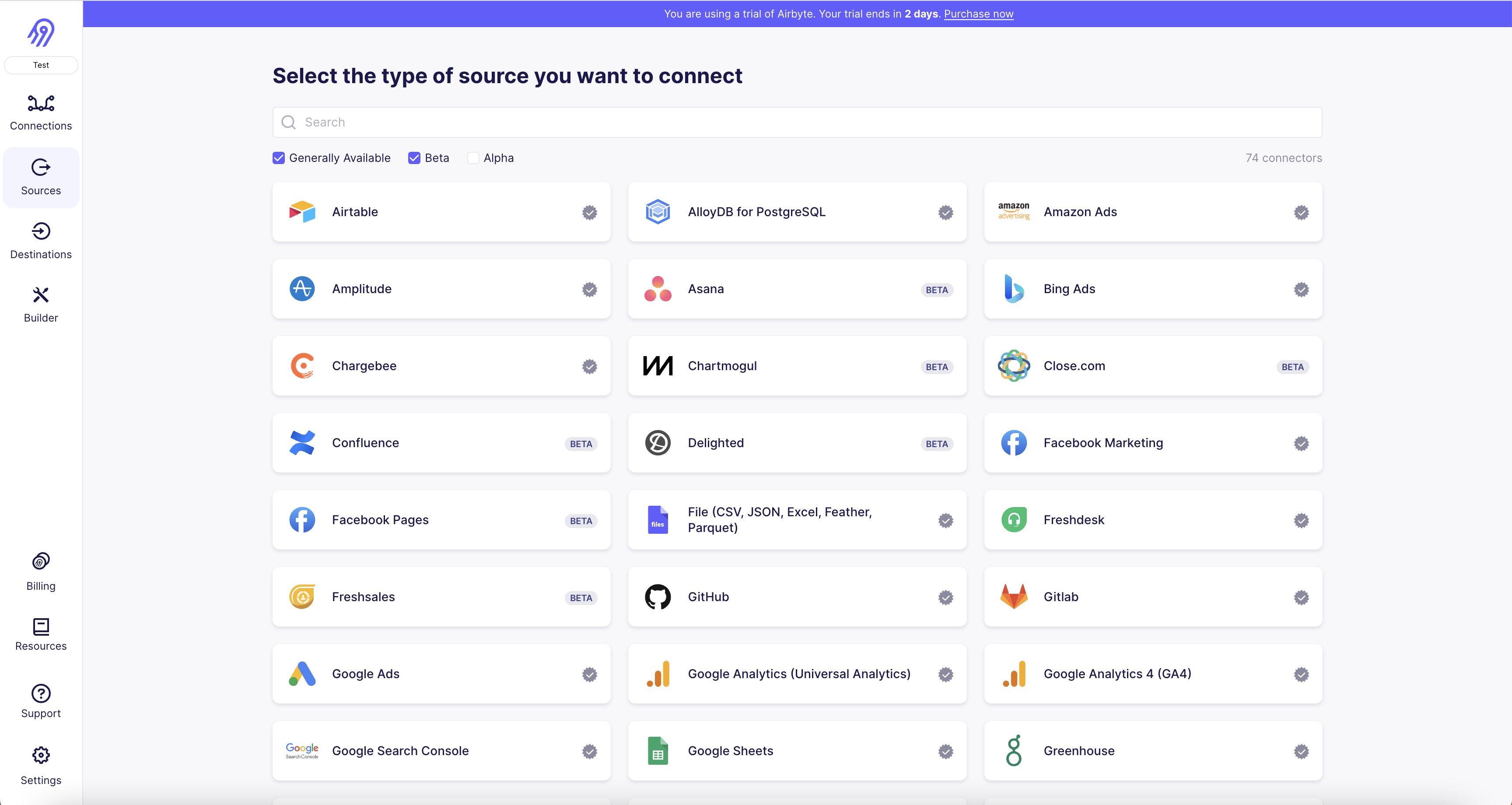
1. Open the Airbyte platform and navigate to the "Sources" tab on the left-hand side of the screen.
2. Click on the "Twitter" source connector and select "Create new connection."
3. Enter a name for your connection and click "Next."
4. Enter your Twitter API credentials, including your Consumer Key, Consumer Secret, Access Token, and Access Token Secret. You can find these credentials by logging into your Twitter Developer account and navigating to the "Keys and Tokens" tab.
5. Once you have entered your credentials, click "Test Connection" to ensure that Airbyte can successfully connect to your Twitter account.
6. If the connection is successful, click "Create" to save your connection.
7. You can now use your Twitter source connector to extract data from your Twitter account. Simply select your connection and choose the data you want to extract, such as tweets, followers, or mentions. You can also set up a schedule to automatically extract data at regular intervals.
Step 2: Set up AWS Datalake as a destination connector

1. Log in to your AWS account and navigate to the AWS Management Console.
2. Click on the S3 service and create a new bucket where you will store your data.
3. Create an IAM user with the necessary permissions to access the S3 bucket. Make sure to save the access key and secret key.
4. Open Airbyte and navigate to the Destinations tab.
5. Select the AWS Datalake destination connector and click on "Create new connection".
6. Enter a name for your connection and paste the access key and secret key you saved earlier.
7. Enter the name of the S3 bucket you created in step 2 and select the region where it is located.
8. Choose the format in which you want your data to be stored in the S3 bucket (e.g. CSV, JSON, Parquet).
9. Configure any additional settings, such as compression or encryption, if necessary.
10. Test the connection to make sure it is working properly.
11. Save the connection and start syncing your data to the AWS Datalake.
Step 3: Set up a connection to sync your Twitter data to AWS Datalake

Once you've successfully connected Twitter as a data source and AWS Datalake as a destination in Airbyte, you can set up a data pipeline between them with the following steps:
- Create a new connection: On the Airbyte dashboard, navigate to the 'Connections' tab and click the '+ New Connection' button.
- Choose your source: Select Twitter from the dropdown list of your configured sources.
- Select your destination: Choose AWS Datalake from the dropdown list of your configured destinations.
- Configure your sync: Define the frequency of your data syncs based on your business needs. Airbyte allows both manual and automatic scheduling for your data refreshes.
- Select the data to sync: Choose the specific Twitter objects you want to import data from towards AWS Datalake. You can sync all data or select specific tables and fields.
- Select the sync mode for your streams: Choose between full refreshes or incremental syncs (with deduplication if you want), and this for all streams or at the stream level. Incremental is only available for streams that have a primary cursor.
- Test your connection: Click the 'Test Connection' button to make sure that your setup works. If the connection test is successful, save your configuration.
- Start the sync: If the test passes, click 'Set Up Connection'. Airbyte will start moving data from Twitter to AWS Datalake according to your settings.
Remember, Airbyte keeps your data in sync at the frequency you determine, ensuring your AWS Datalake data warehouse is always up-to-date with your Twitter data.
Method 2: Connecting Twitter to datalake manually
Moving data from Twitter to an AWS Data Lake without using third-party connectors or integrations involves several steps, including working with the Twitter API, setting up an AWS Data Lake environment, and automating data transfer processes. Below is a detailed guide for developers:
Step 1: Set Up AWS Data Lake Environment
a. Create an AWS Account
- If you don't already have an AWS account, go to https://aws.amazon.com/ and sign up.
b. Set Up S3 Bucket
- Go to the AWS Management Console.
- Navigate to Amazon S3 and create a new bucket where you will store your Twitter data.
- Configure the bucket settings according to your requirements (e.g., versioning, access policies).
c. Set Up AWS Glue (optional)
- Navigate to AWS Glue.
- Create a database that will be used to catalog your Twitter data.
- Set up a crawler to infer schema and populate the AWS Glue Data Catalog.
d. Set Up AWS Lake Formation (optional)
- Go to AWS Lake Formation and register your S3 bucket as a new data lake.
- Define permissions to control access to the data lake resources.
Step 2: Access Twitter Data
a. Apply for a Twitter Developer Account
- Go to https://developer.twitter.com/ and sign up for a developer account.
- Once approved, create a new app and obtain your API keys and access tokens.
b. Use Twitter API
- Familiarize yourself with the Twitter API documentation.
- Choose the appropriate endpoint (e.g., Twitter API v2 or v1.1) for the data you want to collect.
- Write a script using a programming language like Python to access the Twitter API using the keys and tokens obtained earlier.
Step 3: Extract Twitter Data
a. Write Data Extraction Script
- Use the `requests` library in Python to make API calls to Twitter.
- Handle pagination if necessary to retrieve large datasets.
- Parse the JSON response and extract the relevant data fields.
- Save the data in a suitable format (e.g., JSON, CSV) for storage in the S3 bucket.
Step 4: Transfer Data to S3
a. Install AWS CLI or SDK
- Install the AWS Command Line Interface (CLI) or an AWS SDK for your programming language.
b. Configure AWS CLI or SDK
- Run `aws configure` to set up your AWS credentials (Access Key ID and Secret Access Key).
c. Upload Data to S3
- Use the AWS CLI or SDK to upload the extracted Twitter data to your S3 bucket.
- Ensure the data is uploaded in a consistent and query-able format.
Step 5: Automate Data Transfer
a. Schedule the Script
- Use cron jobs (Linux) or Task Scheduler (Windows) to schedule your data extraction script to run at regular intervals.
- Ensure that the script includes steps to upload the new data to the S3 bucket.
b. Monitor Script Execution
- Implement logging in your script to track its execution and any potential errors.
- Optionally, use AWS CloudWatch to monitor the health and performance of your script.
Step 6: Data Management and Analysis
a. Catalog Data with AWS Glue (optional)
- Use the AWS Glue crawler to catalog new data as it arrives in the S3 bucket.
- Use the Data Catalog to query and analyze your data using services like Amazon Athena.
b. Secure Data
- Implement encryption and access control to secure your data in S3.
c. Analyze Data
- Use AWS analytics services like Amazon Athena to run queries directly on your data in S3.
- Connect to Amazon Redshift or EMR for more complex data processing and analysis.
Step 7: Data Maintenance
a. Lifecycle Policies
- Set up lifecycle policies in S3 to archive or delete old data that is no longer needed.
b. Audit and Compliance
- Regularly review access logs and ensure that your data handling practices comply with relevant regulations.
Step 8: Documentation and Best Practices
a. Document the Process
- Create detailed documentation of your setup and scripts for future reference and maintenance.
b. Follow Best Practices
- Regularly update your API keys and access tokens.
- Monitor the Twitter API for changes to endpoints or rate limits.
- Keep your AWS services and tools up to date.
By following these steps, developers can connect Twitter to an AWS Data Lake without third-party connectors or integrations. Remember to handle data securely and comply with Twitter's API usage policies and data protection regulations.
Use Cases to transfer your Twitter data to AWS Datalake
Integrating data from Twitter to AWS Datalake provides several benefits. Here are a few use cases:
- Advanced Analytics: AWS Datalake’s powerful data processing capabilities enable you to perform complex queries and data analysis on your Twitter data, extracting insights that wouldn't be possible within Twitter alone.
- Data Consolidation: If you're using multiple other sources along with Twitter, syncing to AWS Datalake allows you to centralize your data for a holistic view of your operations, and to set up a change data capture process so you never have any discrepancies in your data again.
- Historical Data Analysis: Twitter has limits on historical data. Syncing data to AWS Datalake allows for long-term data retention and analysis of historical trends over time.
- Data Security and Compliance: AWS Datalake provides robust data security features. Syncing Twitter data to AWS Datalake ensures your data is secured and allows for advanced data governance and compliance management.
- Scalability: AWS Datalake can handle large volumes of data without affecting performance, providing an ideal solution for growing businesses with expanding Twitter data.
- Data Science and Machine Learning: By having Twitter data in AWS Datalake, you can apply machine learning models to your data for predictive analytics, customer segmentation, and more.
- Reporting and Visualization: While Twitter provides reporting tools, data visualization tools like Tableau, PowerBI, Looker (Google Data Studio) can connect to AWS Datalake, providing more advanced business intelligence options. If you have a Twitter table that needs to be converted to a AWS Datalake table, Airbyte can do that automatically.
Wrapping Up
To summarize, this tutorial has shown you how to:
- Configure a Twitter account as an Airbyte data source connector.
- Configure AWS Datalake as a data destination connector.
- Create an Airbyte data pipeline that will automatically be moving data directly from Twitter to AWS Datalake after you set a schedule
With Airbyte, creating data pipelines take minutes, and the data integration possibilities are endless. Airbyte supports the largest catalog of API tools, databases, and files, among other sources. Airbyte's connectors are open-source, so you can add any custom objects to the connector, or even build a new connector from scratch without any local dev environment or any data engineer within 10 minutes with the no-code connector builder.
We look forward to seeing you make use of it! We invite you to join the conversation on our community Slack Channel, or sign up for our newsletter. You should also check out other Airbyte tutorials, and Airbyte’s content hub!
What should you do next?
Hope you enjoyed the reading. Here are the 3 ways we can help you in your data journey:
Ready to get started?
Frequently Asked Questions
Twitter's API provides access to a wide range of data, including:
1. Tweets: The API allows access to all public tweets, as well as tweets from specific users or containing specific keywords.
2. User data: This includes information about individual Twitter users, such as their profile information, follower and following counts, and tweet history.
3. Trends: The API provides access to real-time and historical data on trending topics and hashtags.
4. Analytics: Twitter's API also provides access to analytics data, such as engagement rates, impressions, and reach.
5. Lists: The API allows access to Twitter lists, which are curated groups of Twitter users.
6. Direct messages: The API provides access to direct messages sent between Twitter users.
7. Search: The API allows for advanced search queries, including filtering by location, language, and sentiment.
8. Ads: Twitter's API also provides access to advertising data, such as campaign performance metrics and targeting options.
Overall, Twitter's API provides a wealth of data that can be used for a variety of purposes, from social media monitoring to marketing and advertising.