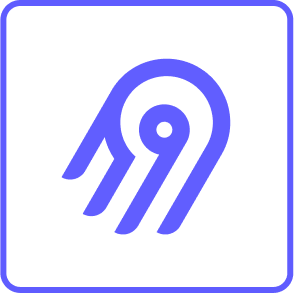

Building your pipeline or Using Airbyte
Airbyte is the only open source solution empowering data teams to meet all their growing custom business demands in the new AI era.
- Inconsistent and inaccurate data
- Laborious and expensive
- Brittle and inflexible
- Reliable and accurate
- Extensible and scalable for all your needs
- Deployed and governed your way
Start syncing with Airbyte in 3 easy steps within 10 minutes



Take a virtual tour
Demo video of Airbyte Cloud
Demo video of AI Connector Builder
What sets Airbyte Apart
Modern GenAI Workflows
Move Large Volumes, Fast
An Extensible Open-Source Standard
Full Control & Security
Fully Featured & Integrated
Enterprise Support with SLAs
What our users say
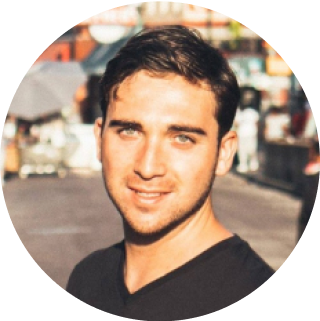
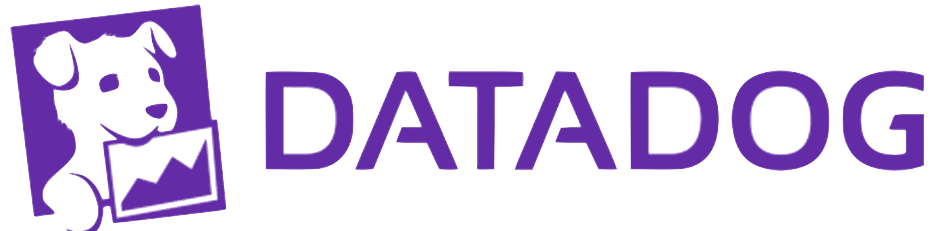
"The intake layer of Datadog’s self-serve analytics platform is largely built on Airbyte.Airbyte’s ease of use and extensibility allowed any team in the company to push their data into the platform - without assistance from the data team!"
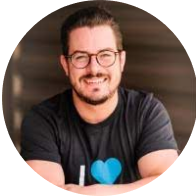
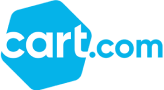
“Airbyte helped us accelerate our progress by years, compared to our competitors. We don’t need to worry about connectors and focus on creating value for our users instead of building infrastructure. That’s priceless. The time and energy saved allows us to disrupt and grow faster.”
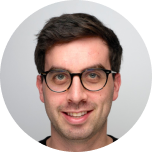

“We chose Airbyte for its ease of use, its pricing scalability and its absence of vendor lock-in. Having a lean team makes them our top criteria. The value of being able to scale and execute at a high level by maximizing resources is immense”
- Prepare PostgreSQL for Export:
- Ensure that you have the necessary permissions to read the data from the PostgreSQL database.
- Choose the tables or data that you want to export.
- Export Data to a CSV File:
- Use the psql command-line tool or another PostgreSQL client to export your data.
Run the following command to export a table to a CSV file:
COPY your_table_name TO '/path_to_export/your_table_name.csv' DELIMITER ',' CSV HEADER;
Replace your_table_name with the name of your table and /path_to_export/your_table_name.csv with the path where you want to save the CSV file.
- Use the psql command-line tool or another PostgreSQL client to export your data.
- Check Data Types:
- Ensure that the data types in your CSV files are compatible with BigQuery data types.
- Make any necessary modifications to the data types or formats.
- Split Large Files:
- If you have very large CSV files, consider splitting them into smaller chunks to make the upload process more manageable.
- Validate CSV Files:
- Check for any issues in the CSV files, such as missing data, incorrect delimiters, or encoding problems.
Step 3: Upload Data to Google Cloud Storage
Create a Google Cloud Storage Bucket:
- Navigate to the Google Cloud Console.
- Go to the Storage section and create a new bucket to store your CSV files.
Upload CSV Files to the Bucket:Use the Google Cloud Console or the gsutil command-line tool to upload your CSV files to the bucket.
Run the following command to upload a file:
gsutil cp /path_to_export/your_table_name.csv gs://your_bucket_name/
Replace /path_to_export/your_table_name.csv with the path to your CSV file and your_bucket_name with the name of your bucket.
- Go to the BigQuery Console:
- Navigate to the BigQuery section in the Google Cloud Console.
- Create a New Dataset:
- Click on "Create dataset" and fill in the necessary information such as dataset ID and location.
- Create a Table in BigQuery:
- In the BigQuery console, select the dataset you created.
- Click on "Create table" and choose the source as Google Cloud Storage.
- Select the CSV file from your bucket.
- Specify the table schema, either by manually entering it or by auto-detecting it.
- Load Data:
- Configure the load job settings, such as the field delimiter, quote character, and whether to allow jagged rows or skip leading rows.
- Click on "Create table" to start the import process.
- After the load job is complete, check the table to ensure that the data has been imported correctly.
- Run a few queries to verify the integrity and correctness of the data.
- If you no longer need the CSV files in Google Cloud Storage, delete them to avoid incurring storage costs.
- Use the gsutil rm command or delete them via the Google Cloud Console.
By following these steps, you can move data from PostgreSQL to Google BigQuery without the need for third-party connectors or integrations. Remember to handle sensitive data with care and ensure that your data transfer complies with any applicable data protection regulations.
FAQs
What is ETL?
ETL, an acronym for Extract, Transform, Load, is a vital data integration process. It involves extracting data from diverse sources, transforming it into a usable format, and loading it into a database, data warehouse or data lake. This process enables meaningful data analysis, enhancing business intelligence.
An object-relational database management system, PostgreSQL is able to handle a wide range of workloads, supports multiple standards, and is cross-platform, running on numerous operating systems including Microsoft Windows, Solaris, Linux, and FreeBSD. It is highly extensible, and supports more than 12 procedural languages, Spatial data support, Gin and GIST Indexes, and more. Many webs, mobile, and analytics applications use PostgreSQL as the primary data warehouse or data store.
PostgreSQL gives access to a wide range of data types, including:
1. Numeric data types: This includes integers, floating-point numbers, and decimal numbers.
2. Character data types: This includes strings, text, and character arrays.
3. Date and time data types: This includes dates, times, and timestamps.
4. Boolean data types: This includes true/false values.
5. Network address data types: This includes IP addresses and MAC addresses.
6. Geometric data types: This includes points, lines, and polygons.
7. Array data types: This includes arrays of any of the above data types.
8. JSON and JSONB data types: This includes JSON objects and arrays.
9. XML data types: This includes XML documents.
10. Composite data types: This includes user-defined data types that can contain multiple fields of different data types.
Overall, PostgreSQL's API provides access to a wide range of data types, making it a versatile and powerful tool for data management and analysis.
What is ELT?
ELT, standing for Extract, Load, Transform, is a modern take on the traditional ETL data integration process. In ELT, data is first extracted from various sources, loaded directly into a data warehouse, and then transformed. This approach enhances data processing speed, analytical flexibility and autonomy.
Difference between ETL and ELT?
ETL and ELT are critical data integration strategies with key differences. ETL (Extract, Transform, Load) transforms data before loading, ideal for structured data. In contrast, ELT (Extract, Load, Transform) loads data before transformation, perfect for processing large, diverse data sets in modern data warehouses. ELT is becoming the new standard as it offers a lot more flexibility and autonomy to data analysts.
What should you do next?
Hope you enjoyed the reading. Here are the 3 ways we can help you in your data journey:
An object-relational database management system, PostgreSQL is able to handle a wide range of workloads, supports multiple standards, and is cross-platform, running on numerous operating systems including Microsoft Windows, Solaris, Linux, and FreeBSD. It is highly extensible, and supports more than 12 procedural languages, Spatial data support, Gin and GIST Indexes, and more. Many webs, mobile, and analytics applications use PostgreSQL as the primary data warehouse or data store.
BigQuery is an enterprise data warehouse that draws on the processing power of Google Cloud Storage to enable fast processing of SQL queries through massive datasets. BigQuery helps businesses select the most appropriate software provider to assemble their data, based on the platforms the business uses. Once a business’ data is acculumated, it is moved into BigQuery. The company controls access to the data, but BigQuery stores and processes it for greater speed and convenience.
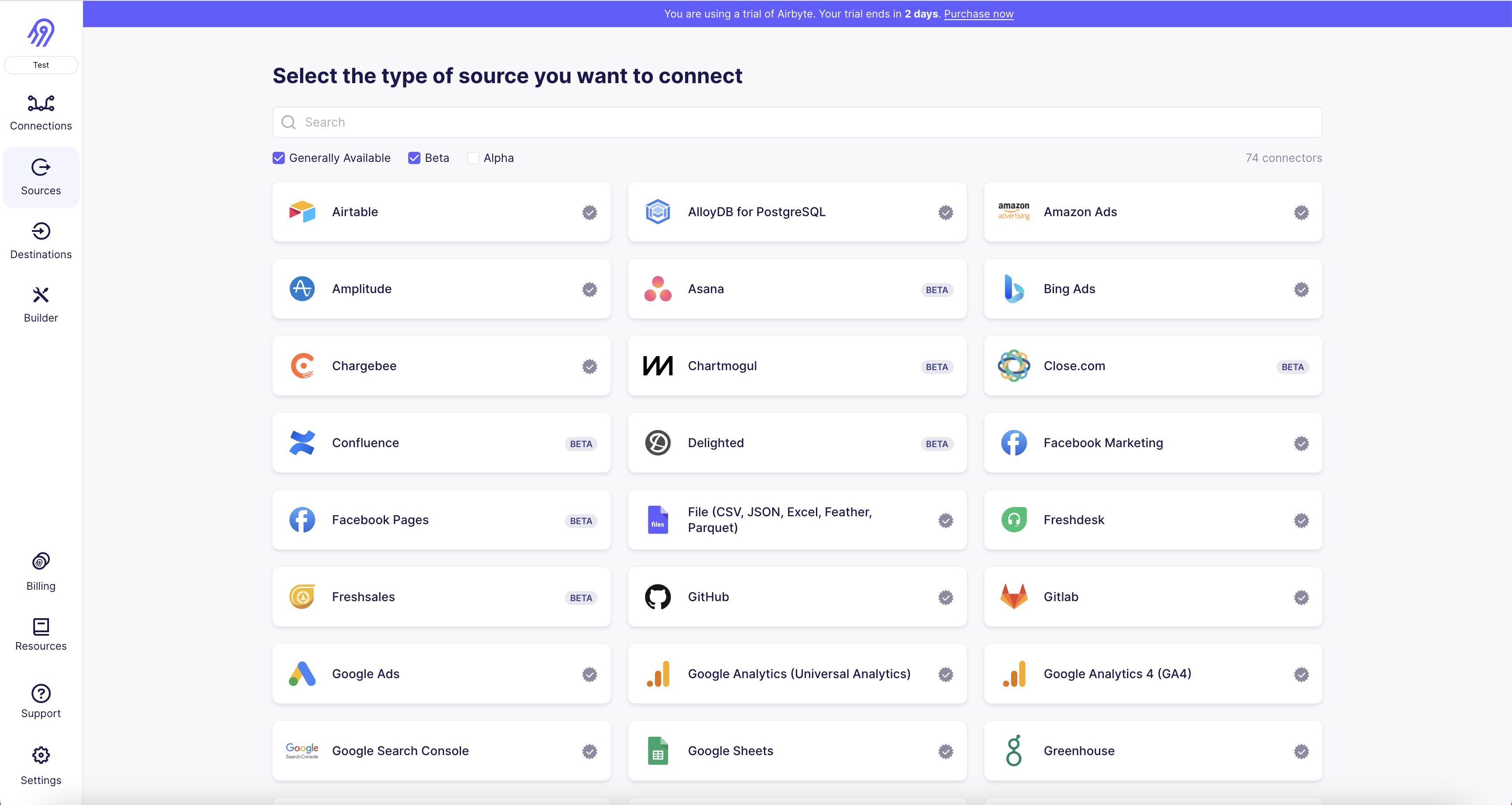
1. Open your PostgreSQL database and create a new user with the necessary permissions to access the data you want to replicate.
2. Obtain the hostname or IP address of your PostgreSQL server and the port number it is listening on.
3. Create a new database in PostgreSQL that will be used to store the replicated data.
4. Obtain the name of the database you just created.
5. In Airbyte, navigate to the PostgreSQL source connector and click on "Create Connection".
6. Enter a name for your connection and fill in the required fields, including the hostname or IP address, port number, database name, username, and password.
7. Test the connection to ensure that Airbyte can successfully connect to your PostgreSQL database.
8. Select the tables or views you want to replicate and configure any necessary settings, such as the replication frequency and the replication method.
9. Save your configuration and start the replication process.
10. Monitor the replication process to ensure that it is running smoothly and troubleshoot any issues that arise.

1. First, navigate to the Airbyte dashboard and select the "Destinations" tab on the left-hand side of the screen.
2. Scroll down until you find the "BigQuery" destination connector and click on it.
3. Click the "Create Destination" button to begin setting up your BigQuery destination.
4. Enter your Google Cloud Platform project ID and service account credentials in the appropriate fields.
5. Next, select the dataset you want to use for your destination and enter the table prefix you want to use.
6. Choose the schema mapping for your data, which will determine how your data is organized in BigQuery.
7. Finally, review your settings and click the "Create Destination" button to complete the setup process.
8. Once your destination is created, you can begin configuring your source connectors to start syncing data to BigQuery.
9. To do this, navigate to the "Sources" tab on the left-hand side of the screen and select the source connector you want to use.
10. Follow the prompts to enter your source credentials and configure your sync settings.
11. When you reach the "Destination" step, select your BigQuery destination from the dropdown menu and choose the dataset and table prefix you want to use.
12. Review your settings and click the "Create Connection" button to start syncing data from your source to your BigQuery destination.

With Airbyte, creating data pipelines take minutes, and the data integration possibilities are endless. Airbyte supports the largest catalog of API tools, databases, and files, among other sources. Airbyte's connectors are open-source, so you can add any custom objects to the connector, or even build a new connector from scratch without any local dev environment or any data engineer within 10 minutes with the no-code connector builder.
We look forward to seeing you make use of it! We invite you to join the conversation on our community Slack Channel, or sign up for our newsletter. You should also check out other Airbyte tutorials, and Airbyte’s content hub!
What should you do next?
Hope you enjoyed the reading. Here are the 3 ways we can help you in your data journey:
The need to sync data from an operational database like Postgres to BigQuery, a data warehouse, is a prevalent challenge that data engineers need to solve. The data team at Airbyte is not the exception.
Airbyte OSS and Airbyte Cloud use Postgres databases to store the state of data replication jobs. Hence, our internal databases contain information about job executions, connectors, workspaces, permissions, credit consumption, and configurations used by our Cloud customers. Since the data in Postgres is precious to the business, we move it to BigQuery to make it available to all teams in the company who are interested in analyzing and visualizing such data.
ℹ️ If you’re using Airbyte OSS, similar data is also available to you. Airbyte stores internal state and metadata in two internal Postgres databases that you can access to gain insights. These databases contain information about: Execution of Airbyte jobs and various runtime metadata. The internal orchestrator used by Airbyte (tasks, workflow data, events, and visibility data). Connectors, sync connections, and various Airbyte configuration objects.
Once the raw data is in BigQuery, we use dbt to transform it and structure it into different layers. From there, the various teams can create dashboards in Metabase. Furthermore, the data from BigQuery is propagated to other systems such as Salesforce and Customer.io using reverse ETL to enrich customer and prospects’ data.
But why do we need to move the data to BigQuery? In short, because using operational databases for analytical purposes is far from ideal!
Postgres is a robust, open-source relational database system with a good reputation for reliability and performance. It implements ACID transactions and is designed to manage a wide range of workloads, from single computers to web applications with many concurrent users.
But, because Postgres is also an online transaction processing (OLTP) database, it’s not optimized for data warehouse use. Data warehouses serve analytical workloads and are typically built on columnar databases – also called online analytical processing (OLAP) databases.
Analytical queries often select a few columns and several rows (sometimes the whole table) and then aggregate those. A columnar database can be 1000x faster for an analytical query than an OLTP database.
BigQuery – Google’s fully managed serverless data warehouse – is one of the most widely used columnar databases. It’s NoOps, meaning there is no infrastructure to operate and can handle data analysis over petabytes.
Now, it’s easy to understand why analyzing data in BigQuery is preferred over Postgres, but how’s that achieved? Replicating a Postgres database to another database or data warehouse is generally tricky. It is not sufficient to export the database using the pg_dump command. You must also handle loading new or updated data by continually checking the tables for fresh updates.
A possible solution is to create an ETL or ELT pipeline and orchestrate it using a platform like Airflow.
The term ELT pipeline, which stands for “extract, load, transform,” refers to a collection of procedures that transport data from one or more sources into a database, such as a data warehouse.
Airflow has built-in transfer and database operators that can be used to sync data from Postgres to BigQuery, such as the PostgresToGCSOperator and the GCSToBigQueryOperator. Building your data pipelines with Airflow is a good starting point; however, it doesn’t come without challenges. That’s why at Airbyte, we use our product, Airbyte Cloud, to sync data from Postgres to BigQuery! With a few configurations, we implemented a Postgres to Bigquery ELT pipeline that incrementally ingests data and provides us with deduplicated and historical data in the destination.
In this tutorial, you will learn how we created an ELT pipeline to sync data from Postgres to BigQuery using Airbyte Cloud. You can follow these steps to create your own.
{{COMPONENT_CTA}}
Prerequisites
- A Postgres database v9.3.x or above
- A GCS account for BigQuery.
- Signing up for Airbyte Cloud or deploying Airbyte OSS.
Methods to Move Data From Postgresql to BigQuery
- Method 1: Connecting Postgresql to BigQuery using Airbyte.
- Method 2: Connecting Postgresql to BigQuery manually.
Method 1: Connecting Postgresql to BigQuery using Airbyte.
Step 1: Configure a Postgres source in Airbyte
Before setting up your Postgres source in Airbyte, check our getting started documentation.
To set up a new Postgres Airbyte source, go to Airbyte's UI, click on sources and add a new source. As the connector type, select Postgres. The subsequent illustrations show how we configured one of the sources at Airbyte. Fill in the following configuration fields with the details of your Postgres database.
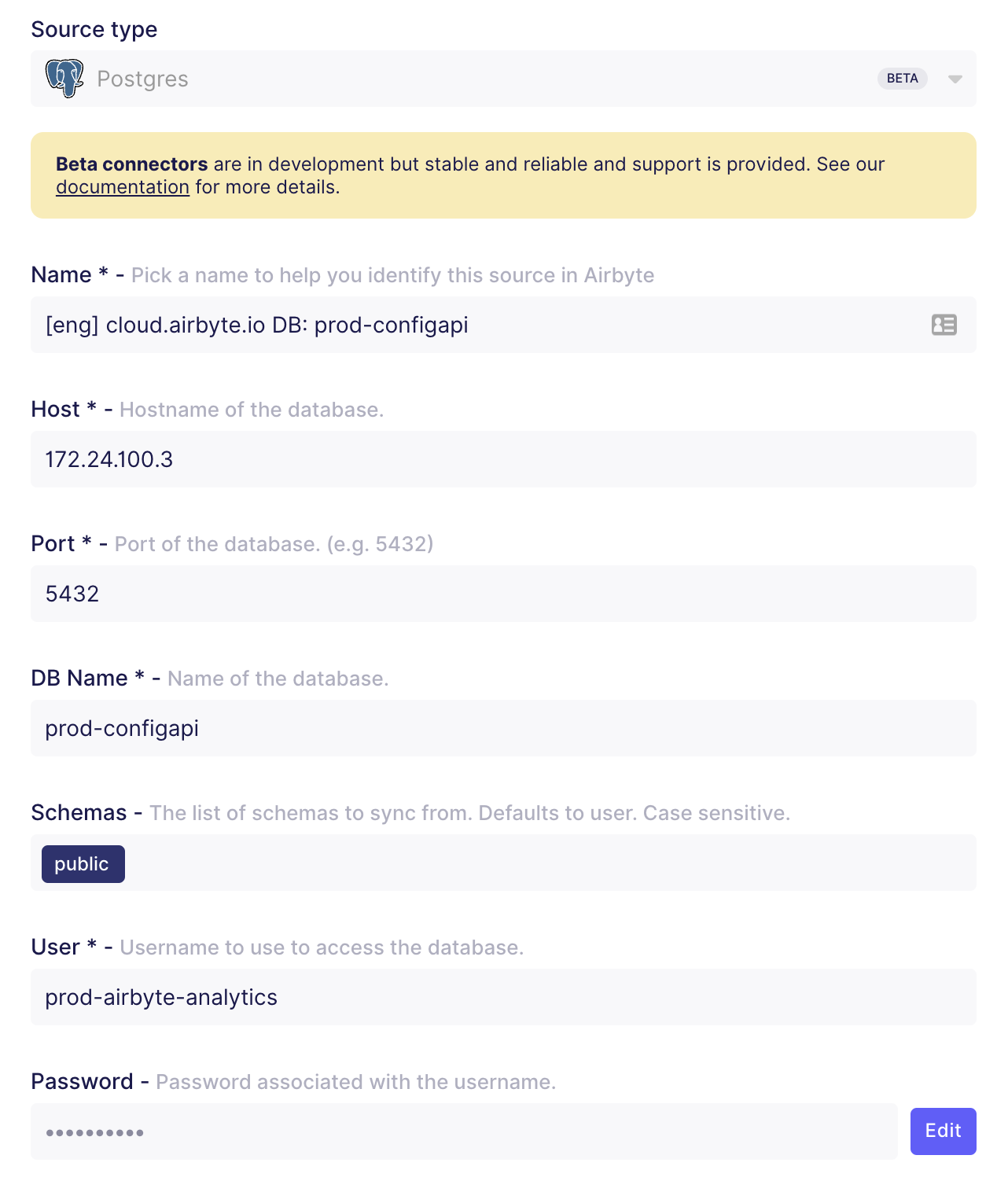
Then click on Set up source, and Airbyte will test the connection. If everything goes well, you should see a successful message.
Step 2: Configure a BigQuery destination in Airbyte
Go to destinations and add a new one. As demonstrated in the following diagram, select BigQuery as the destination type and fill in with the following details.
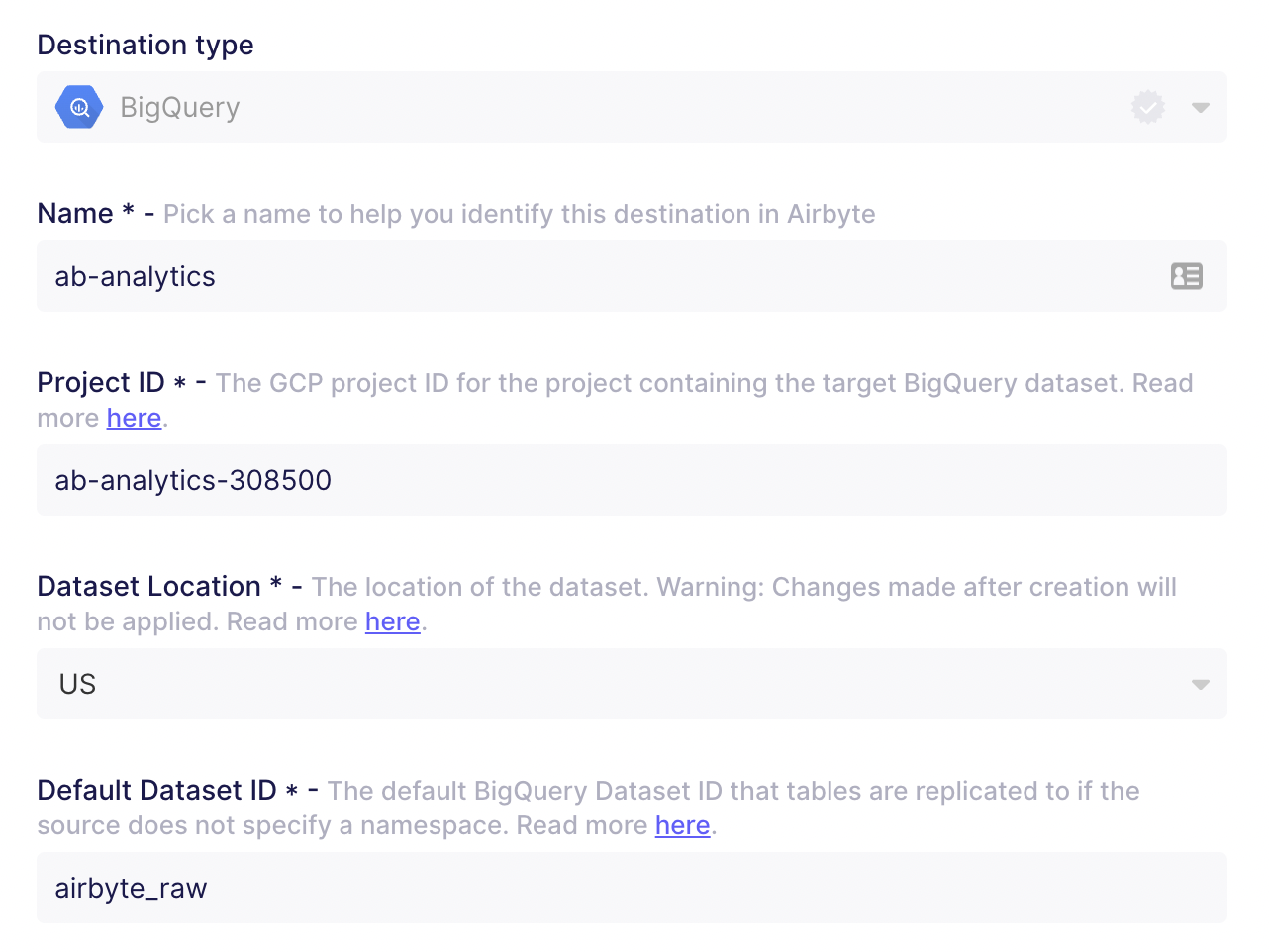
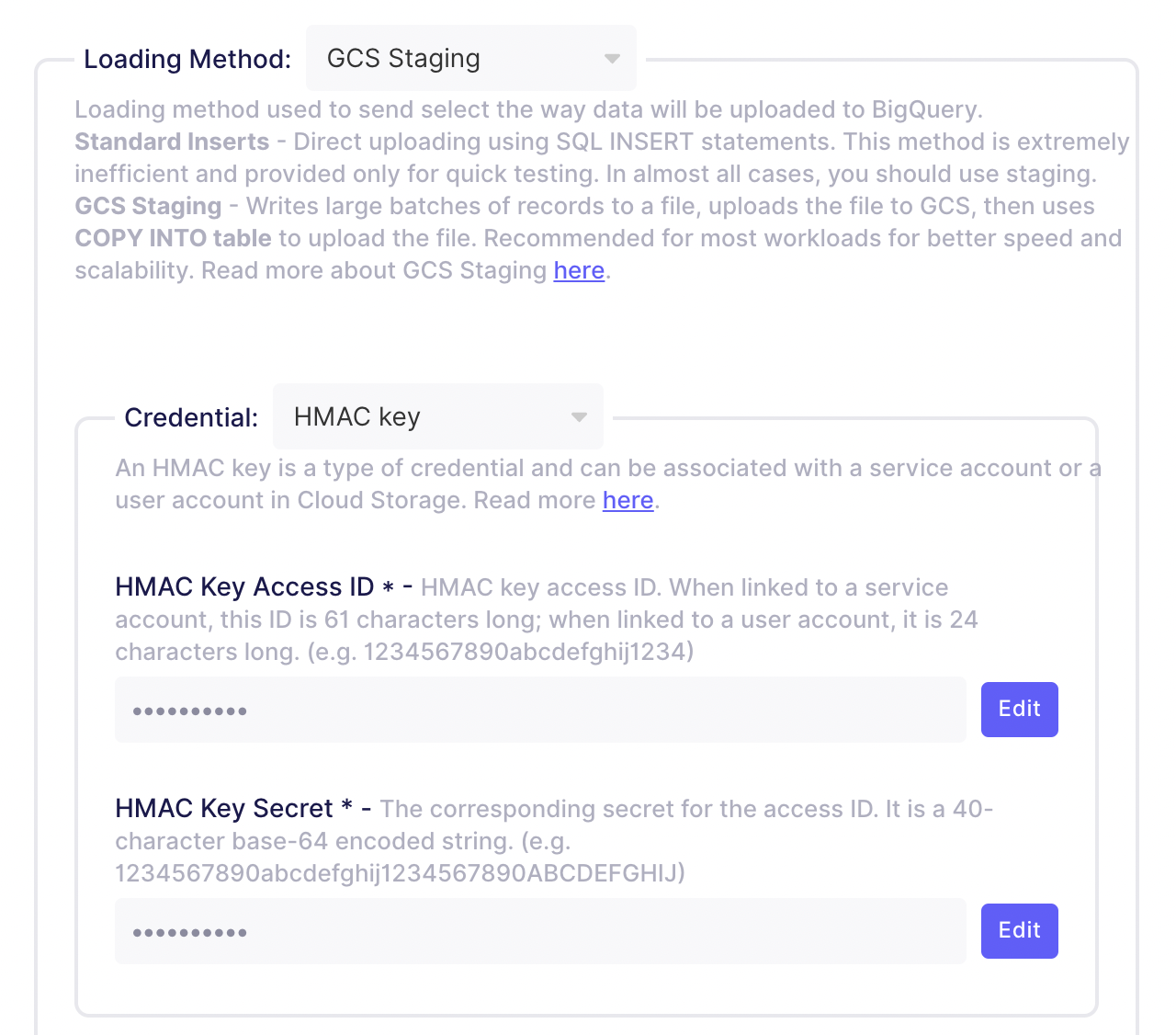

If you’re in doubt regarding the meaning of any of the configuration fields, you can read our BigQuery setup guide. At the same time, you will find hints about each field and links with additional information in the UI.
Then, click on Set up source and let Airbyte test the destination.
Step 3: Create an Airbyte connection
Go to connections and create a new connection. Then, select the existing Postgres source you have just created, and then do the same for the BigQuery destination. The following diagram shows how we set up the connection at Airbyte.
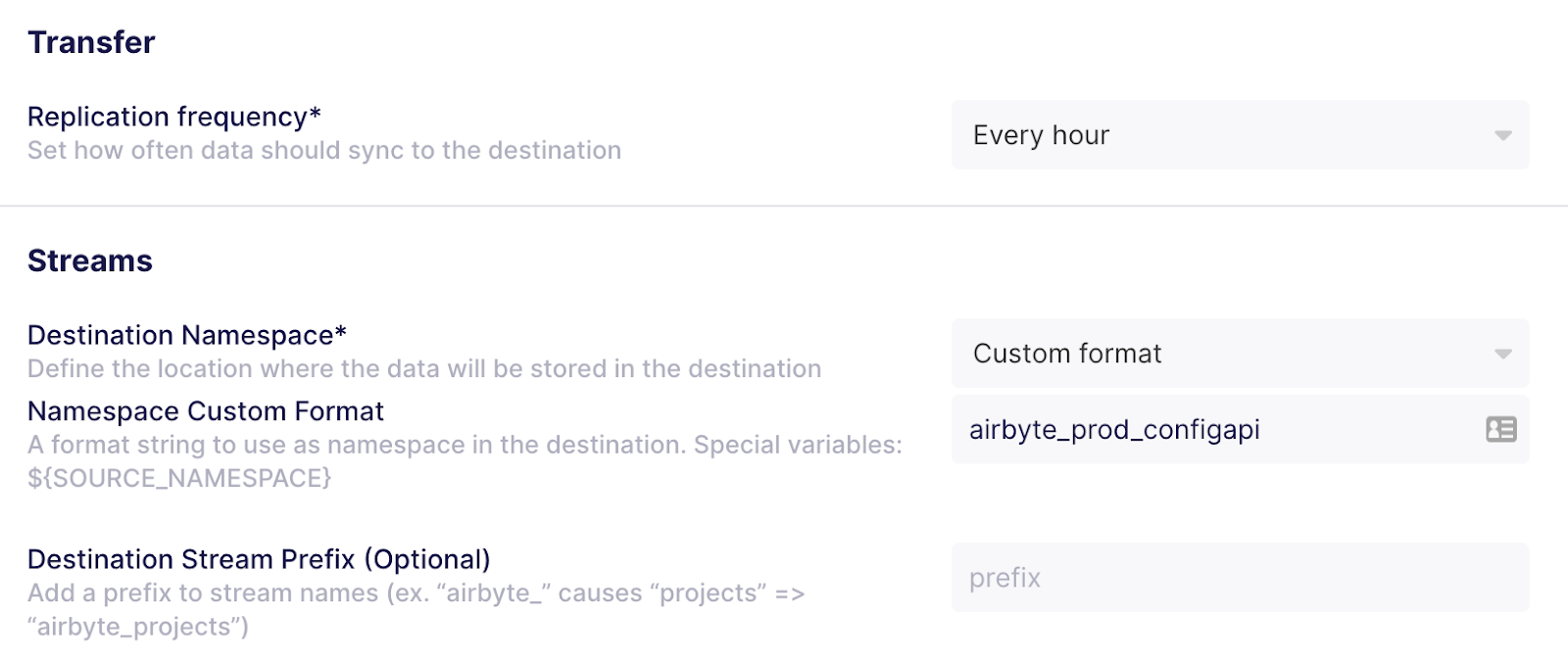
As you can see, we set the replication frequency to every hour. You can change the replication frequency depending on your needs.
At Airbyte, we load data from multiple sources to a single BigQuery project. Because we don’t want data to be held in the default dataset specified in the destination configuration (airbyte_raw), we used the “Custom format” option so the data will be stored in the airbyte_prod_configapi dataset. If you want to know more about namespaces, check our documentation.
Then, it's time to configure the streams. Each stream represents a table that will be created in BigQuery. You can expand each stream to see the fields they contain, their data type, how they will be named in BigQuery, etc. You can select which streams you want to sync using the “Sync” toggle.
Now, you should select a sync mode for each stream. As shown in the image below, we extract data from Postgres in an Incremental way and load it using Deduped + history mode.
We use the Deduped + history mode because we want to model our data as Slowly Changing Dimensions of type 2 (SCD). For example, in our dashboards, we want to know when a connection stopped being active (when someone disabled it or deleted it), but we still need it to count for periods when it was active. It means a connection could be counted for June but not in July if it was disabled for July.
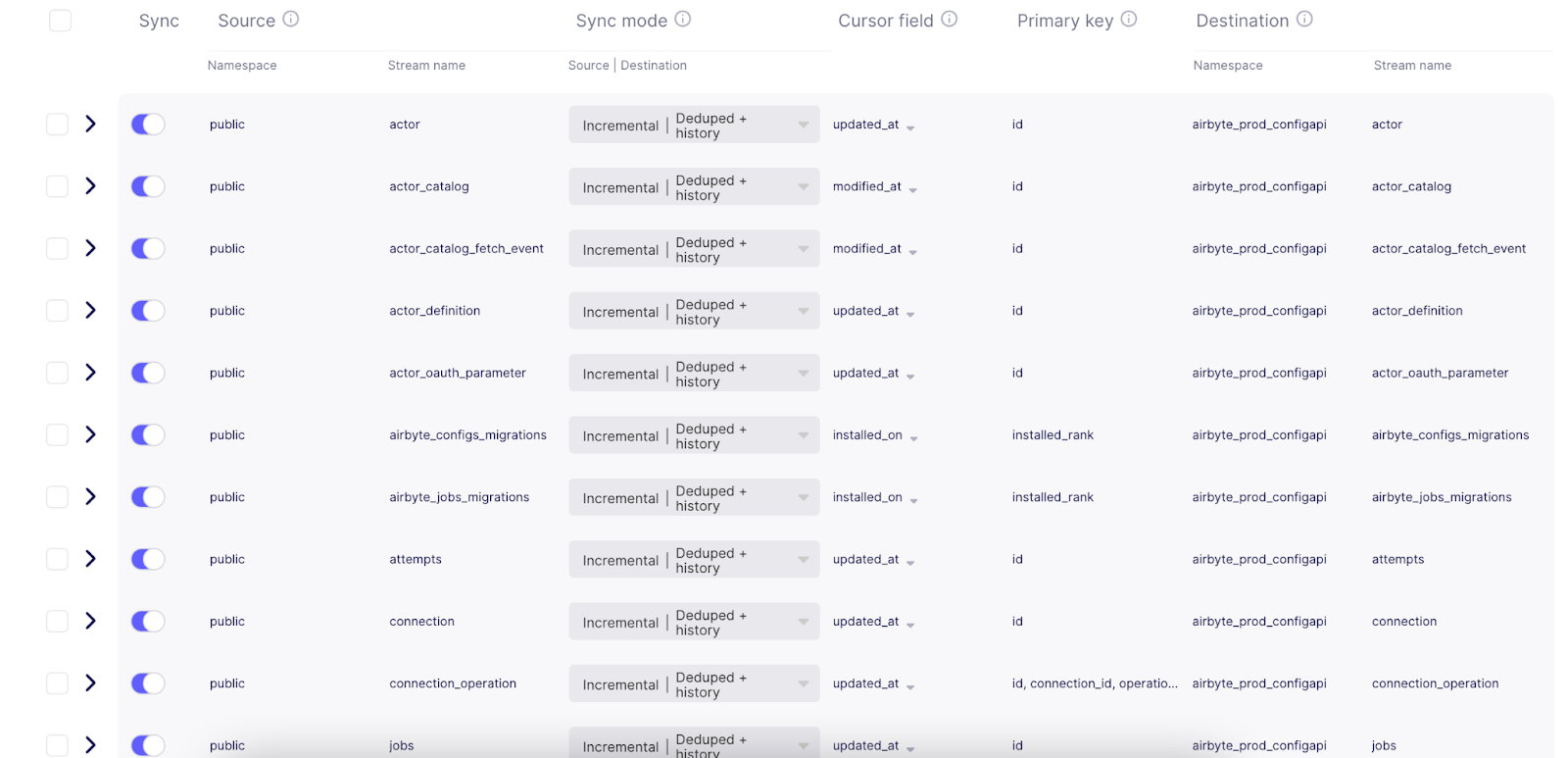
You can learn more about sync modes in our documentation.
On top of replication, Airbyte provides the option to enable or disable an additional transformation step at the end of the sync, called normalization. In this case, the basic normalization maps the JSON object from the Postgres ingestion to a format compatible with BigQuery. Hence, we suggest using the “Normalized tabular data” option.

Once you're ready, save the changes. Then, you can run your first sync by clicking on Sync now. You can check your run logs to verify everything is going well. Just wait for the sync to be completed, and that's it! You've created an ELT pipeline from Postgres to BigQuery where the EL steps are the responsibility of Airbyte. In this case, the T step is a simple data normalization step done by dbt and orchestrated by Airbyte. You can then execute other dbt transformations as we do at Airbyte to consolidate your data before creating dashboards.
Step 4: Review data in BigQuery
After a successful sync, you should be able to see the data in BigQuery. If you go to your dataset, you’ll see several sets of tables were created.
Raw data tables
The tables named _airbyte_raw_* contain the raw JSON data fetched from the source. They only have three columns: _airbyte_ab_id, _airbyte_emitted_at, _airbyte_data. These tables exist because a core tenet of ELT philosophy is that data should be untouched as it moves through the “E” and “L” stages so that the raw data is always accessible.

Normalized tables
If you enabled basic normalization, the tables whose name matches the stream name are your normalized tables. The raw JSON data should have been mapped into columns.
Because we selected the Deduped + history sync mode, there should be no duplicates in these tables. Deduped implies data in the final table is unique per primary key, which is achieved by sorting the data using the cursor field and preserving only the latest de-duplicated data row. This is known as an SCD table of type 1 in dimensional data warehouse lingo.
You will also see some metadata columns added to these tables.

Slowly Changing Dimension (SCD) tables
The tables named *_scd are the historical tables and are only created when you use the Deduped + history sync mode. History denotes creating an extra intermediate table to which data is constantly appended (with duplicates). This is known as an SCD table of type 2.
You will also see some metadata columns added to these tables.

Method 2: Connecting Postgresql to BigQuery manually.
To move data from PostgreSQL to Google BigQuery manually, you can follow these steps:
Step 1: Export Data from PostgreSQL
- Prepare PostgreSQL for Export:
- Ensure that you have the necessary permissions to read the data from the PostgreSQL database.
- Choose the tables or data that you want to export.
- Export Data to a CSV File:
- Use the psql command-line tool or another PostgreSQL client to export your data.
Run the following command to export a table to a CSV file:
COPY your_table_name TO '/path_to_export/your_table_name.csv' DELIMITER ',' CSV HEADER;
Replace your_table_name with the name of your table and /path_to_export/your_table_name.csv with the path where you want to save the CSV file.
- Use the psql command-line tool or another PostgreSQL client to export your data.
Step 2: Prepare the Data for BigQuery
- Check Data Types:
- Ensure that the data types in your CSV files are compatible with BigQuery data types.
- Make any necessary modifications to the data types or formats.
- Split Large Files:
- If you have very large CSV files, consider splitting them into smaller chunks to make the upload process more manageable.
- Validate CSV Files:
- Check for any issues in the CSV files, such as missing data, incorrect delimiters, or encoding problems.
Step 3: Upload Data to Google Cloud Storage
Create a Google Cloud Storage Bucket:
- Navigate to the Google Cloud Console.
- Go to the Storage section and create a new bucket to store your CSV files.
Upload CSV Files to the Bucket:
Use the Google Cloud Console or the gsutil command-line tool to upload your CSV files to the bucket.
Run the following command to upload a file:
gsutil cp /path_to_export/your_table_name.csv gs://your_bucket_name/
Replace /path_to_export/your_table_name.csv with the path to your CSV file and your_bucket_name with the name of your bucket.
Step 4: Create a Dataset in BigQuery
- Go to the BigQuery Console:
- Navigate to the BigQuery section in the Google Cloud Console.
- Create a New Dataset:
- Click on "Create dataset" and fill in the necessary information such as dataset ID and location.
Step 5: Load Data into BigQuery
- Create a Table in BigQuery:
- In the BigQuery console, select the dataset you created.
- Click on "Create table" and choose the source as Google Cloud Storage.
- Select the CSV file from your bucket.
- Specify the table schema, either by manually entering it or by auto-detecting it.
- Load Data:
- Configure the load job settings, such as the field delimiter, quote character, and whether to allow jagged rows or skip leading rows.
- Click on "Create table" to start the import process.
Step 6: Verify the Data
Check the Table in BigQuery:
- After the load job is complete, check the table to ensure that the data has been imported correctly.
- Run a few queries to verify the integrity and correctness of the data.
Step 7: Clean Up
Remove Temporary Files:
- If you no longer need the CSV files in Google Cloud Storage, delete them to avoid incurring storage costs.
- Use the gsutil rm command or delete them via the Google Cloud Console.
By following these steps, you can move data from PostgreSQL to Google BigQuery without the need for third-party connectors or integrations. Remember to handle sensitive data with care and ensure that your data transfer complies with any applicable data protection regulations.
Wrapping up
Taking your operational data to the next level by loading it into a data warehouse can give you significant advantages. At Airbyte, we use our product, Airbyte Cloud, to achieve that, and you can get started for free. This tutorial taught you how we quickly implemented an ELT pipeline from Postgres to BigQuery that incrementally ingests data and provides us with deduplicated and SCD tables.
The next step for us is to enable log-based Change Data Capture (CDC) in the source, which Airbyte supports. Using CDC will allow us to have a more granular data change tracking and is one of the most efficient ways to ingest data.
We invite you to join the conversation at Airbyte’s community Slack Channel to share your ideas with thousands of data practitioners and help make everyone’s project successful. With Airbyte, the integration possibilities are endless, and we can't wait to see what you're going to build!
What should you do next?
Hope you enjoyed the reading. Here are the 3 ways we can help you in your data journey:
Ready to get started?
Frequently Asked Questions
PostgreSQL gives access to a wide range of data types, including:
1. Numeric data types: This includes integers, floating-point numbers, and decimal numbers.
2. Character data types: This includes strings, text, and character arrays.
3. Date and time data types: This includes dates, times, and timestamps.
4. Boolean data types: This includes true/false values.
5. Network address data types: This includes IP addresses and MAC addresses.
6. Geometric data types: This includes points, lines, and polygons.
7. Array data types: This includes arrays of any of the above data types.
8. JSON and JSONB data types: This includes JSON objects and arrays.
9. XML data types: This includes XML documents.
10. Composite data types: This includes user-defined data types that can contain multiple fields of different data types.
Overall, PostgreSQL's API provides access to a wide range of data types, making it a versatile and powerful tool for data management and analysis.