What is Data Fabric: Architecture, Examples, & Purpose
New technologies like Artificial Intelligence and the Internet of Things (IoT) have significantly increased the volume of big data generated and consumed by organizations. However, this data remains scattered across siloed systems and cloud environments, complicating data management and governance. This leads to security threats, data inaccessibility, and inefficient decision-making.
Many organizations are turning to data fabric solutions to address these issues. These solutions help simplify and optimize data integration, offering a unified and comprehensive view of their information assets. In this article, you will delve deeper into the concept of data fabric, its architecture, use cases, and more.
What Is Data Fabric?
Data fabric is an innovative, network-based architectural framework that facilitates end-to-end data integration of your organization’s data pipelines, whether on-premises, in cloud, or hybrid environments. By utilizing advanced technologies such as artificial intelligence (AI), machine learning (ML), and advanced automation, data fabric ensures that data is consistently available, accurate, and secure across all your platforms.
Advantages of Data Fabric
According to Gartner, implementing data fabric can significantly streamline various aspects of data management in your organization. It can reduce the time required for integration design by 30%, deployment time by 30%, and maintenance efforts by 70%.
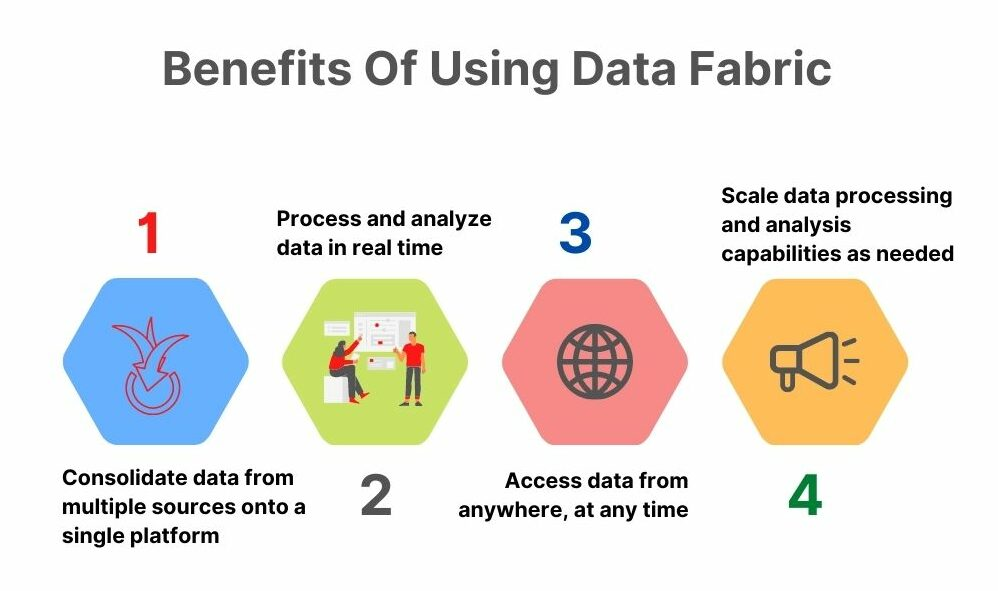
Here are some ways your organization can benefit from adopting data fabric solutions:
- Democratizing Data Access: Data fabric breaks down data silos, making trusted data readily available to the teams across your organization. This fosters self-service data discovery and analytics.
- Enhanced Data Quality and Governance: By leveraging active metadata, the data fabric improves data quality, consistency, and compliance with regulatory requirements. It helps your organization maintain control over its data assets.
- Real-Time Insights through Automation: Data fabric automates data engineering tasks and streamlines integration, enabling you to gain insights and make data-driven decisions based on real-time information.
- Scalability for Any Environment: Automated workflows ensure your data pipelines can handle any data volume, regardless of the environment. Thus, you can quickly adapt and scale your data operations.
What Is the Purpose of Data Fabric?
Data fabric is important because it helps you deal with data fragmentation by ensuring seamless connectivity and promoting a holistic data environment. This capability is crucial for accelerating your digital transformation initiatives, as it provides a robust foundation for innovation, product development, and enhanced customer experiences. The unified data view offered by the data fabric also helps you easily achieve stakeholder buy-in.
Data fabric facilitates real-time data processing, which is essential for applications such as fraud detection and operational monitoring. It also ensures your business’s continuity when disruptions occur by maintaining data integrity and availability, supporting data recovery, and facilitating future planning. These factors collectively explain the purpose of data fabric solutions in your organization.
5 Key Pillars of the Data Fabric Architecture
A data fabric architecture provides a unified approach to managing data across diverse environments. It goes beyond simple data integration to offer your data teams a dynamic, self-service platform. Here’s a breakdown of the key pillars that make up a data fabric architecture:
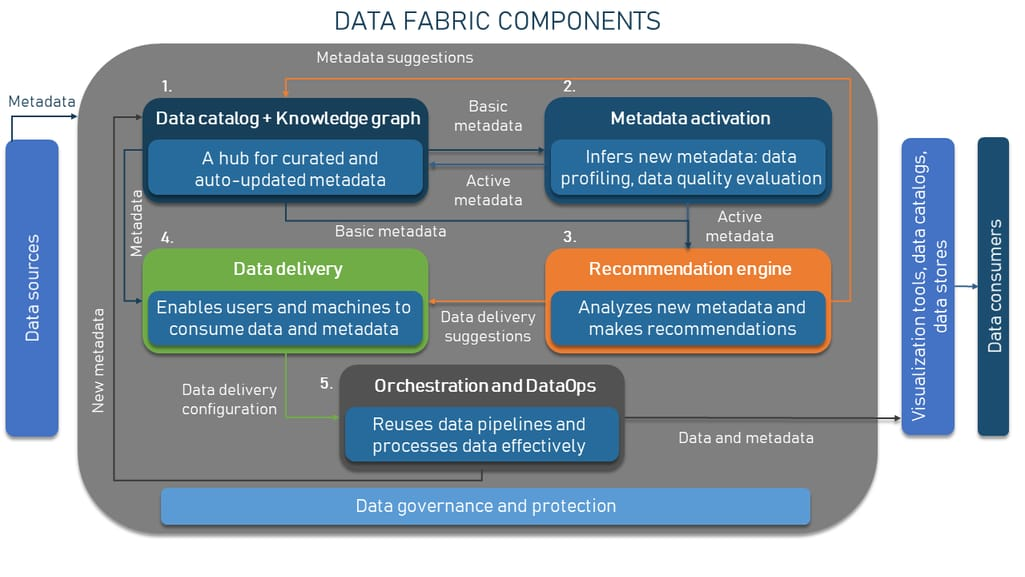
Augmented Data Catalog and Lineage
This pillar focuses on creating a detailed catalog and providing intelligent search functionalities that go beyond listing data sources. It leverages AI and automatically discovers, classifies, and tags data assets, making them easier to find and understand.
Your data teams can easily find relevant data and understand its origin, flow, and transformations throughout its lifecycle. This transparency builds trust among teams and promotes active collaboration.
Enriched Knowledge Graph with Semantics
The data fabric not only captures technical metadata but also business context and relationships between data elements. This enriched metadata becomes the foundation for data governance and insights. Data knowledge graphs help visualize these connections and relationships between data entities and provide a holistic view of your organization’s data.
Metadata Activation and Intelligent Recommendation Engine
Here, AI and machine learning algorithms are employed to analyze metadata and provide actionable recommendations. It automates various data management tasks, such as suggesting relevant datasets, improving data quality and discoverability, and facilitating more efficient data integration processes. You can also utilize ML algorithms to analyze your data usage patterns and recommend optimal strategies for your organization.
Streamlined Data Preparation and Delivery
Having a streamlined data preparation and delivery system in place ensures efficient data movement and transformation to meet your diverse needs. You can utilize multi-latency data pipelines designed to accommodate different latency requirements and enable real-time analytics or batch-processing workflows. This optimizes resource utilization.
Additionally, you can mask and desensitize data to comply with regulations and ensure data privacy. This pillar guarantees the timely delivery of reliable, accurate, and protected data.
Workflow Orchestration and Data Ops Optimization
Applying the DataOps principles can help you automate and orchestrate your entire data fabric ecosystem. This ensures automated data pipelines and streamlines data movement and transformation.
It also incorporates continuous integration and continuous delivery (CI/CD) for data, where you regularly monitor, test, and deploy data pipelines to ensure reliable data delivery. Additionally, with real-time monitoring, you can gain insights into data quality, pipeline health, and performance and identify any potential issues.
By implementing these key pillars, you can establish a robust data fabric that simplifies data management and enables your data teams to explore the opportunities your data assets present for future growth and success.
How to Implement a Data Fabric?
The foundation of a successful data fabric implementation starts by clearly understanding your business requirements and goals. You can implement a data fabric solution at your organization by following the steps outlined below:
- Interact with key stakeholders from various departments, such as marketing, sales, and operations, to understand their data needs, performance indicators (KPIs), and current data challenges.
- Take a detailed note of your existing data infrastructure, including data sources, databases, data warehouses, data lakes, and other existing ETL/ELT processes.
- Establish robust data governance to ensure clear guidelines for data ownership, access controls, and security protocols. This will help improve data quality, integrity, and regulatory compliance.
- Build a cross-functional team with experts in data management, architecture, engineering, and business analysis. This team will oversee the implementation and maintenance of the data fabric.
- Start with a pilot project focusing on a specific business need. This allows you to test your approach, identify potential challenges, and refine your strategy before full-scale deployment.
- A well-planned data fabric solution will yield good results when your data teams can leverage it efficiently. Conduct training sessions to help your employees understand data fabric and utilize its self-service capabilities.
- Working with a data fabric is an ongoing process. You should practice data observability and track its performance, identify unusual patterns, anomalies, or areas for optimization, and scale it as your data needs evolve.
Use Cases of Data Fabric
Data fabric is changing how businesses across industries handle and utilize their ever-growing data. Here are some data fabric examples that help in data governance and management:
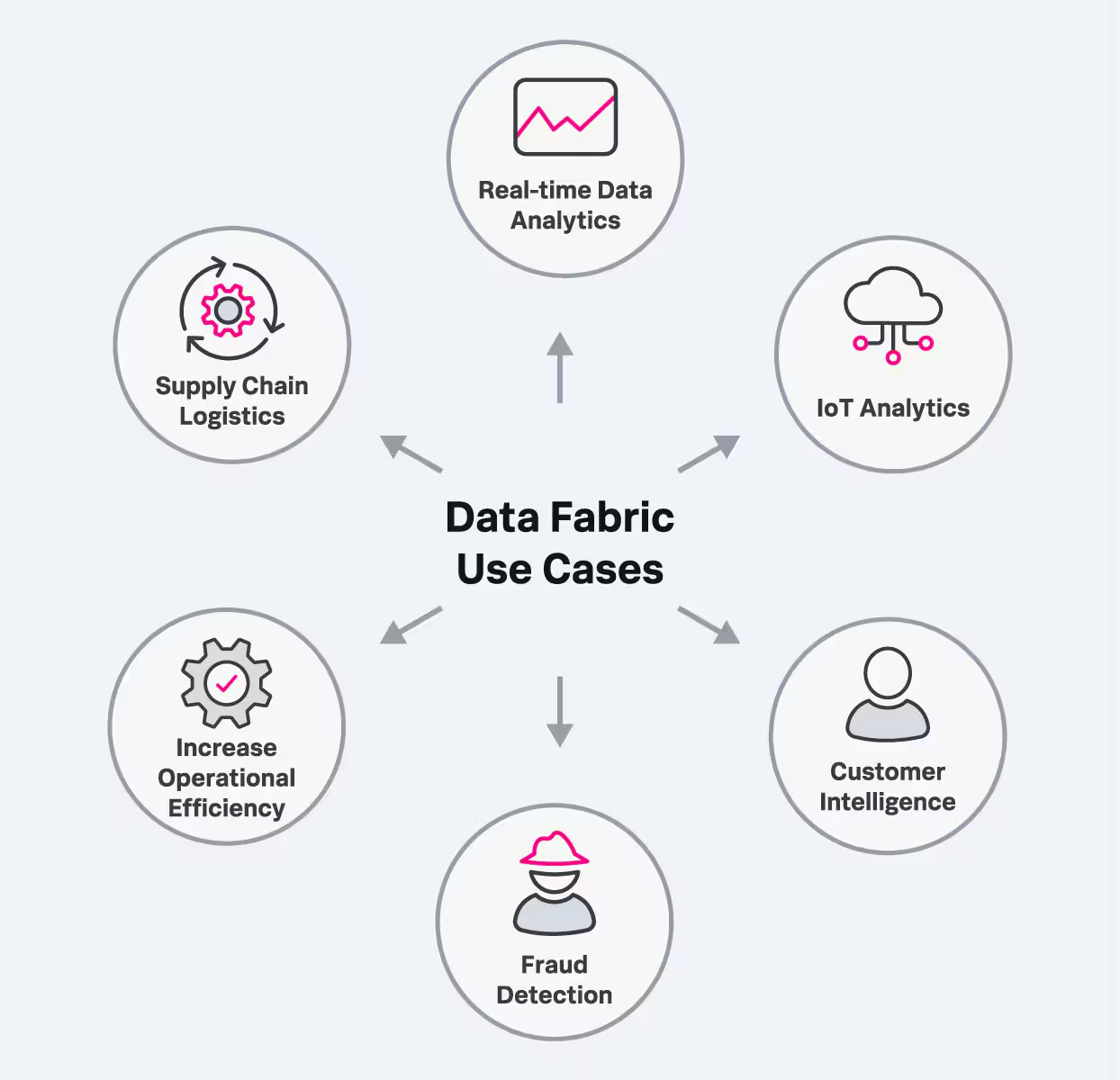
- Manufacturing Sector: A manufacturing company deals with complex supply chains involving multiple suppliers, factories, and distributors. Data fabric can help connect data from production lines, inventory management systems, and logistics providers. This real-time visibility assists in optimizing production schedules, predicting potential bottlenecks, and ensuring efficient delivery of goods.
- Financial Sector: Financial institutions are constantly on the lookout for fraudulent activities. Data fabric can play a crucial role here by integrating data from various sources, such as transactions, customer profiles, and security threat intelligence. This holistic view of the data is useful in detecting suspicious patterns in real-time and ensuring customer safety.
- Retail Sector: A large retail chain owner can implement data fabric and get real-time views of inventory levels, customer preferences, and sales trends. Analyzing this data provides a 360-degree customer view and helps optimize inventory and draft personalized marketing campaigns, boosting the company’s sales.
Popular Data Fabric Vendors
There are many vendors present in the market that provide robust solutions to support the data fabric architecture. Here is a list of the top three vendors:
SAP Data Intelligence Cloud

SAP is a comprehensive data management solution that supports data fabric implementation. It enables you to connect, discover, enrich, and orchestrate data assets across diverse data sources. This empowers you to gain valuable insights from big data.
Some benefits of SAP Data Intelligence Cloud include integrating any type of data, reusing any data processing engine, and democratizing of information.
IBM Cloud Pak for Data
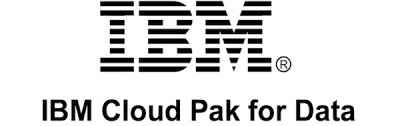
IBM Cloud Pak for Data allows you to build a data fabric that bridges siloed data across hybrid cloud environments. Its benefits include automating data integration, metadata management, and AI governance. This provides your organization with cost-effective, streamlined data management with reduced complexity.
Informatica

Informatica’s Intelligent Data Fabric leverages AI and ML to automate your data pipelines while ensuring their quality. This platform facilitates integrating complex data, optimizing analytics, data migrations, and Master Data management (MDM). Additionally, Informatica scales seamlessly to handle high-volume, complex data requirements.
Streamline Data Integration in the Data Fabric Flow with Airbyte
Seamless data integration within a data fabric is crucial for breaking down silos and enabling unified data view and access. Airbyte simplifies this process by offering a library of over 350 pre-built connectors. This allows you to efficiently move data from various sources to your target systems, including data warehouses, lakes, and datasets.
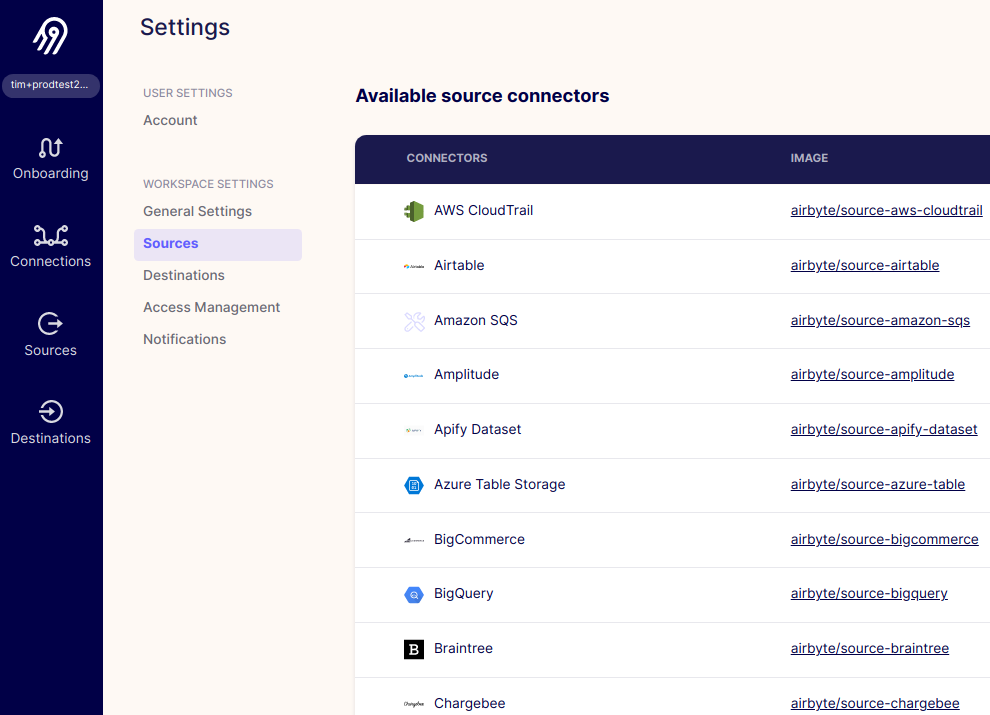
Airbyte also offers a Connector Development Kit to create custom connectors based on your specific needs. It is an open-source, no-code platform with an ELT (extract, load, transfer) approach. Airbyte allows you to seamlessly integrate with platforms like dbt (Data Build Tool) and perform complex data transformations within your existing workflows.
Airbyte’s intuitive, user-friendly interface enables even non-tech teams to build and mange data pipelines without any programming experience. It optimizes efficiency by supporting incremental data synchronization, which allows you to transfer only new or changed data since the last successful sync. This helps in minimizing unnecessary data movement.
With Airbyte, you can streamline your data flow with ease and leverage the full potential of your data fabric architecture. To learn more about the tool, refer to Airbyte’s official documentation.
Closing Thoughts
The data fabric approach offers a compelling solution for your organization’s data management issues. It breaks down silos, ensures data quality, and equips your teams to explore potential ways of utilizing your organizational data. While data fabric implementation requires detailed planning and ongoing maintenance, the benefits of a unified, accessible, and secure data environment are undeniable.
This article provides an overview of data fabric architecture and its implementation steps. The use cases explain how data fabric can offer an optimal solution for several businesses. This fosters a data-driven culture that fuels innovation and business growth.
FAQs
What is the difference between data fabric and data mesh?
Data fabric and data mesh handle complex data but take different approaches. Data fabric creates a central layer for data access, while data mesh decentralizes ownership and empowers individual teams from various departments to manage their data.
Why use data fabric for a data warehouse over ADF + Azure SQL?
Data fabric is better for big data analytics as it empowers you to handle unstructured and real-time streaming data. On the other hand, Azure Data Factory (ADF) and Azure SQL are better for traditional business intelligence (BI) and structured data. Data fabric also offers a more scalable architecture for future growth.
How does a data fabric allow a data-driven culture in an organization?
Data fabric provides a unified view of all your data. This simplifies data governance and lets everyone access trusted information for making informed business decisions, fostering collaboration and a data-driven culture across the organization.