Data Integrity vs Data Quality: What is the Difference?
The terms data integrity and data quality are frequently used interchangeably, leading to confusion regarding their distinct meanings and implications. Understanding the fundamental differences between these concepts is crucial if you strive to use your data effectively. Data integrity refers to data's accuracy, consistency, and reliability throughout its lifecycle, ensuring that it remains unaltered and trustworthy. On the other hand, data quality pertains to the fitness of data, encompassing factors such as precision, completeness, and relevance. While both concepts are essential for maintaining the veracity and usability of data, they address distinct aspects of data management and require tailored strategies for implementation.
This article aims to highlight the difference between data integrity and data quality, outlining their respective roles and implications in data management and decision-making processes.
Data Integrity Overview

Data integrity is a fundamental aspect of data management, focusing on maintaining the accuracy and consistency of data. It involves implementing steps to ensure that data remains unchanged and reliable throughout its lifecycle, from creation to storage and usage. This includes implementing validation checks, encryption methods, and access controls to prevent errors, corruption, or unauthorized alterations to the data.
Key characteristics of data integrity:
- Maintaining consistency across various data instances is a core aspect of data integrity. You can rely on consistent datasets to make informed decisions and conduct reliable analyses by ensuring coherence and uniformity in data representation.
- Robust security measures like encryption, access controls, and authentication protocols are integral to data integrity. These efforts help protect your data from unauthorized access, tampering, or corruption, thus ensuring the integrity and confidentiality of sensitive information.
Data Quality Overview

Data quality is essential for ensuring data is fit for its intended purpose. It encompasses various aspects such as precision, completeness, and relevance. Data of high quality is reliable and can be trusted to make informed decisions and derive meaningful insights.
Key characteristics of data quality:
- Data quality ensures that all necessary data is present and available, without any missing or incomplete information, to support accurate analysis and decision-making. This involves assessing data completeness against predefined criteria and addressing gaps or deficiencies to ensure comprehensive datasets.
- By emphasizing the importance of data relevance through data quality, you can ensure that the information collected and maintained is pertinent to the planned purpose or use case. By prioritizing relevant data, you can avoid unnecessary clutter and focus on extracting meaningful insights.
- Data quality maintains consistency across different datasets and sources, ensuring uniformity and coherence in data interpretation and analysis. This involves reconciling inconsistencies and discrepancies between datasets to ensure compatibility and reliability in data-driven decision-making processes.
- Timeliness is critical to data quality, ensuring that data is up-to-date and reflects the most current information available. By regularly updating and refreshing datasets, you can mitigate the risk of relying on outdated information, thus maintaining the relevance and precision of their data assets.
- Data quality focuses on the usability of data, ensuring that it is structured, formatted, and organized in a way that facilitates easy access, analysis, and interpretation. This means making data easy to access and understand so stakeholders can use it effectively to benefit the business.
Differentiating Both Concepts: Data Integrity vs Data Quality
With a solid understanding of the overarching concepts of data integrity and data quality, let's now explore the key differences between these two critical aspects of data management. While both are essential for ensuring the reliability and usability of data, they address distinct dimensions and require unique approaches for implementation and maintenance.
The main difference between Data Integrity and Data Quality is that Data Integrity ensures the accuracy, consistency, and reliability of data throughout its lifecycle, while Data Quality focuses on the overall fitness of data for its intended use, including completeness, accuracy, and timeliness.
Data Quality vs Data Integrity: Objective
- The primary objective of data integrity is to safeguard the security and trustworthiness of data by preventing unauthorized modifications, intentional manipulation, or accidental degradation.
- Data quality aims to optimize the usability and usefulness of data, ensuring it is fit for analysis, decision-making, and operational use within the organization.
Data Quality vs Data Integrity: Implementation
- Data integrity is often implemented through security measures such as encryption, access controls, and verification mechanisms to maintain the integrity of data.
- Data quality requires processes such as data profiling, cleansing, and governance to address errors, inconsistencies, and inaccuracies that may affect the usability and reliability of data.
Data Quality vs Data Integrity: Assessment
- Data integrity is typically assessed through verification mechanisms such as checksums or digital signatures to validate the authenticity and integrity of data.
- Data quality is evaluated based on various criteria, including completeness, accuracy, relevance, consistency, and timeliness, using techniques such as data profiling and quality metrics.
Data Quality vs Data Integrity: Impact
- Data integrity helps you build trust in your data assets, safeguarding against data breaches, fraud, and regulatory non-compliance.
- Maintaining data quality enhances decision-making processes, operational efficiency, and customer satisfaction, leading to improved business performance and competitiveness.
Data Quality vs Data Integrity: Timeframe
- Data integrity focuses on maintaining the accuracy of data over its entire lifecycle, from creation to archival or disposal.
- Data quality may prioritize timeliness, ensuring that data is up-to-date and relevant at the moment of use, which may vary depending on the application's specific requirements.
Data Quality vs Data Integrity: Responsibility
- Ensuring data integrity often falls under the purview of IT with data engineers. Also, with security teams who implement technical standards to protect data from unauthorized actions.
- Data quality is often a shared responsibility across various departments, including analysts, managers, and business users, who collaborate to ensure data meets the organization's standards and requirements.
Data Quality vs Data Integrity: Risk Management
- Data integrity safeguards aim to minimize the risks of deliberate or accidental alterations, distortions, or unauthorized use of sensitive information.
- Data quality efforts also contribute to risk management by reducing the likelihood of making decisions based on inaccurate or incomplete data, thereby mitigating operational, financial, and reputational risks.
Data Quality vs Data Integrity: Regulatory Compliance
- Data integrity measures are often driven by regulatory requirements and industry standards that mandate your organization to protect the integrity and confidentiality of sensitive data or financial records.
- Data quality initiatives may also align with regulatory compliance efforts, as regulations often require your organization to maintain accurate and reliable data for reporting, auditing, and compliance purposes. However, data quality may encompass broader aspects beyond regulatory compliance to ensure data meets your organizational objectives and stakeholder needs.
Best Practices for Maintaining Data Integrity and Data Quality
Ensuring data integrity and quality requires a proactive approach and adherence to best practices throughout the data lifecycle. Here are some key strategies:
Establish Data Governance Frameworks
Implement robust data governance frameworks that define policies, procedures, and responsibilities for managing and protecting data. This includes establishing roles and responsibilities, defining data standards and guidelines, and enforcing compliance with regulatory requirements.
Conduct Regular Audits and Assessments
Conduct regular audits and assessments of data to identify potential issues or discrepancies. This involves implementing data quality metrics, conducting data profiling, and performing validation checks to ensure data meets established standards.
Implement Security Measures
Execute a comprehensive set of security measures to protect data. You can enforce encryption techniques, access controls, and authentication mechanisms, as well as data masking or tokenization practices, to safeguard sensitive information and prevent unauthorized disclosure.
Invest in Quality Data Integration Solutions
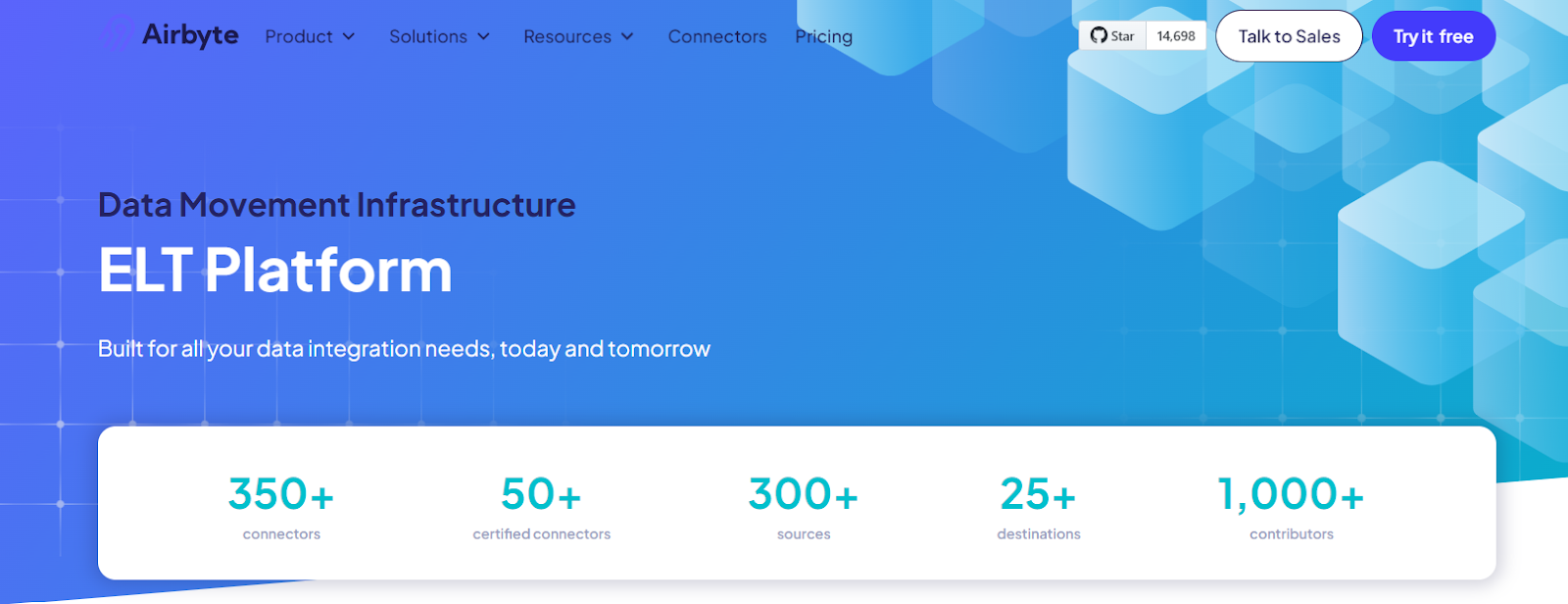
Data integration solutions simplify data integration tasks, reduce manual effort, and ensure data consistency and reliability. One such state-of-the-art solution is Airbyte, which will help you streamline the collection and loading of data from disparate sources into a centralized repository.
With Airbyte's intuitive user interface and 350+ pre-built connectors, you can easily configure and automate data pipelines without the need for extensive coding. This streamlines data integration processes, reduces human error risk and enhances data integrity by ensuring accurate and reliable data transfers.
Some of the unique features of Airbyte include:
- Airbyte provides both open-source and cloud-version deployment options, allowing you to choose the deployment option that best fits your infrastructure, scalability, and management preferences.
- Airbyte offers a centralized platform for connecting to various data sources, such as databases, APIs, file systems, and cloud services. Whether using the self-hosted or cloud version, Airbyte consolidates data ingestion processes and helps you maintain data consistency and reliability across different systems and sources.
- Airbyte provides monitoring and validation features, allowing you to track the performance of your data pipelines and validate data accuracy. This helps ensure that your data integration processes are running smoothly and data being transferred is error-free.
Conclusion
The distinction between data integrity vs data quality is crucial for navigating the complexities of modern data management. Data integrity ensures the accuracy, consistency, and reliability of data, safeguarding against unauthorized alterations and maintaining the trustworthiness of information. On the other hand, data quality focuses on the fitness for the purpose of data, encompassing factors such as completeness, relevance, and timeliness to meet your needs.
While both concepts are integral to effective data management, they require tailored strategies and ongoing efforts to address their unique challenges and implications.