Data has become a crucial part of decision-making, underscoring the importance of high-quality data to make accurate conclusions. However, data quality management is quite challenging. Poor data can cause significant financial losses, as research suggests that organizations incur an average cost of $15 million per year due to bad data.
One key contributor to poor data quality is stale data—outdated, irrelevant information that can skew your analysis. This article will help you explore what stale data is, its impact on your business, and what can be done to avoid it.
What is Stale Data?
Stale data refers to outdated or obsolete information that is no longer accurate or relevant to your current business needs. It relates to data that has not been updated within the required frequency interval for productive use. However, the acceptable interval for data updates can vary based on the specific use case, ranging from days to just a few minutes.
For example, the marketing team may need their ad spend dashboard updated weekly for their regular meetings, during which they make optimization decisions. In contrast, a machine learning algorithm that detects financial fraud requires real-time data updates to analyze transactions, identify patterns, and flag potential fraudulent activities promptly.
Causes of Stale Data
Stale data can be a result of different factors. Let’s explore a few of them:
Infrequent Updates
Data becomes stale if it is not updated frequently enough. This can happen when you do not have regular processes in place to synchronize your data. The lack of timely updates may lead to outdated information, which may hinder decision-making and result in ineffective business strategies.
Lack of Real-time Synchronization
Without real-time synchronization, any changes made to the data in one source may not be immediately reflected in another. For example, you are using a to-do list app on your phone and computer. If the app lacks real-time synchronization, adding a task on one device may not show up immediately on the other. This can cause confusion as you may forget or duplicate tasks.
Human Error
Human errors such as typographical mistakes, incorrect formatting, missing entries, or data duplication may occur while manually inputting data. These inaccuracies accumulate over time, resulting in stale and unreliable information.
Network or System Failures
Issues such as system failures, hardware malfunctions, software glitches, or server downtime can interrupt the flow of data, causing delays in updates. These disruptions can lead to synchronization errors in databases and impede real-time data processing, resulting in inconsistent information.
Lack of Data Governance
Data governance refers to the comprehensive set of policies and processes that ensure data quality and accuracy throughout its lifecycle. However, failure to incorporate a robust data governance framework may lead to outdated information due to a lack of standardized procedures and improper data management.
Examples of stale data
Below are a few examples where stale data can have significant negative implications:
Inventory Management
Outdated inventory data can lead to overstocking or stockouts, impacting profitability and customer satisfaction. For example, if customers visit a website and see a product listed as available, they might place an order, assuming it is in stock.
However, if the inventory data is not updated, the product may be out of stock by the time the order is processed. This results in a poor user experience and potential loss of sales.
Customer Data
Stale customer data may include outdated contact information such as phone numbers or email addresses. This can lead to failed marketing campaigns, ineffective customer engagement, and missed business opportunities.
For instance, if a customer has changed their email address and the company is still using the old one, they will not receive any communications. This means that the customer may not be aware of new products or special offers. As a result, the company loses the chance to nurture the customer relationship and potentially make a sale.
Financial Data
Traders rely on accurate data to buy or sell shares. However, if the data they use does not reflect real-time market conditions, they may end up making poor decisions.
For example, consider investors who act on outdated stock prices. Based on this information, they might buy a stock at a higher price than its actual market value or sell it for less than it's worth. This can contribute to significant financial losses.
Flight Data
Outdated flight information can cause airlines a range of problems, including overbooking, customer dissatisfaction, and operational inefficiencies. For example, if a flight fully booked weeks ago still shows available seats in the airline's scheduling system, it could lead to overbooking and inconvenience for passengers.
This can also make it challenging for the airline to optimize crew schedules and ground operations, leading to increased operational costs.
How to Identify Stale Data?
Here are some approaches that can help you in identifying stale data:
Timestamps
Each data entry is accompanied by a timestamp that records the exact date and time when it was last modified or created. By examining these timestamps, you can determine the age of the data. If a data entry has not been updated for a significant period, it may indicate that the data is no longer accurate or relevant.
Data Comparison
Data comparison involves systematically evaluating the consistency and accuracy of data across different sources. If different datasets provide conflicting information, it clearly indicates potential stale data. For example, if sales figures in a financial system differ from those in a sales management system, it suggests that one of them may not be up-to-date.
💡Suggested Read: What is Data Matching?
Data Freshness Metrics
Data freshness metrics refer to a set of measures used to assess the relevance of your data. These metrics include timestamps, sync frequency, latency, data decay, and more. They help you evaluate how current and reliable your data is, which is crucial for making informed decisions.
Monitoring and Alerts
Setting up monitoring systems allows you to continuously track data updates and capture any deviations from expected update frequencies. Alerts can be configured to notify you instantaneously when data entries surpass predefined thresholds, such as infrequent updates or inconsistencies with other datasets.
Data Profiling
Data profiling is used in data analysis to gain insights about the quality, consistency, and structure of your data. It involves examining the content and characteristics of your data to understand its patterns, anomalies, and potential issues. You can effectively identify outdated data within your dataset by implementing data profiling.
Take a look at the top data profiling tools to enhance the quality of your datasets.
Data Quality Checks
Data quality checks are a systematic process of assessing the accuracy, completeness, consistency, timeliness, and validity of your data. By incorporating these checks into your data management practices, you can identify any outdated or unreliable information, ensuring that your datasets remain trustworthy.

Consequences of Stale Data
Stale data can have a detrimental effect on various aspects of your business operations. Here are a few of them:
Inaccurate Reporting
Reports generated from stale data provide insights that can lead to misguided strategies. For example, using outdated inventory data can lead to incorrect forecasting and procurement decisions, potentially resulting in stockouts or overstock.
Incorrect Business Decisions
A report by Exasol shows that 58% of organizations make decisions based on outdated data. When data is obsolete, the analysis based on that data may not reflect the current state of your business. This can result in flawed insights and misinterpreting trends, leading to incorrect conclusions.
Poor Customer Experience
Stale data in databases can decrease customer satisfaction. For instance, data sources must be regularly updated if you want to send personalized offers based on recent purchase history. Otherwise, you may end up sending irrelevant recommendations that no longer align with your customer's preferences.
Compliance Risks
Your business should comply with regulatory requirements, such as following data protection laws and industry standards for security and privacy. Failure to adhere to these regulations due to stale data can result in legal consequences and financial penalties.
Reputational Damage
Stale data can lead to reputational damage, which can be challenging to recover. If your stakeholders discover that the data being used is outdated, they will lose trust and confidence. This can have long-term consequences, impacting your business success and sustainability.
How to Prevent Stale Data?
Here are some effective ways to prevent stale data:
Real-time Data Integration
Real-time data integration involves the seamless and immediate transfer of data from various sources to target systems in real time. It ensures that data is continuously updated and synchronized across systems, facilitating timely decision-making.
Automated Data Refreshes
Implement automated data refresh routines to ensure that the data remains up-to-date and current. Scheduling regular updates helps maintain the integrity and accuracy of your data by synchronizing it with the latest information from the source systems.
Data Quality Checks and Validation
Enforce data quality checks and validation processes to identify and correct any inaccuracies in the data. This involves checking for missing values, validating data against predefined rules or constraints, and ensuring data integrity. By proactively addressing data quality issues, you can prevent stale or unreliable data from entering your system.
Data Governance and Ownership
Establish robust data governance practices that clearly define data ownership, roles, and responsibilities. Assign dedicated stewards or owners accountable for the data's accuracy and freshness. This framework helps create a structured approach to manage the data lifecycle, enforce data standards, and ensure continuous monitoring and improvement of data quality.
Monitoring and Alerting
Utilize monitoring systems that continuously track the freshness of the data and notify you when it exceeds predefined thresholds. Alerts can be triggered based on specific criteria, such as data age or lack of updates. You can take immediate action to refresh and resolve any underlying issues by receiving timely notifications.
Creating Data-Driven Culture
Inculcate a data-driven culture within your organization, emphasizing the importance of using fresh and reliable data for decision-making. Encourage your staff to prioritize data quality and provide training on data management best practices.
How Airbyte Helps You Prevent Stale Data?
When your business collects and stores data in separate silos, maintaining consistent and up-to-date records becomes significantly challenging. Lack of synchronization and centralized control over data results in delays, inaccuracies, and inconsistencies, ultimately causing the accumulation of stale data.
Therefore you must break down these data silos by implementing integrated data management systems. This approach ensures the timely and accurate flow of information across your entire organization, keeping data fresh and reliable.
Airbyte is one such popular cloud-based data integration platform designed to streamline data movement. It offers a comprehensive catalog of over 350 pre-built connectors that allows you to connect diverse sources to your desired destination. You can easily configure a data pipeline in minutes without extensive coding knowledge.
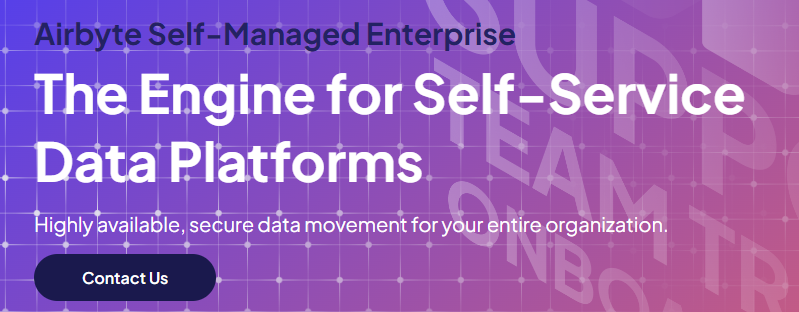
Here are the key features of Airbyte:
Customization of Connectors: Airbyte empowers you with even greater flexibility through its Connector Development Kit (CDK) if you can't find the required connector. With the CDK, you can quickly build custom connectors in less than 30 minutes.
Change Data Capture: Airbyte's CDC capabilities enable you to capture changes made to your source dataset and migrate it to your destination. While setting up the data pipeline, you can set up an incremental sync frequency. This ensures that data flows seamlessly and is continuously updated across different systems, thereby maintaining freshness.
Data Transformations: Airbyte follows the ELT (Extract, Load, Transform) approach, which involves loading data into the destination system prior to transformation. However, it allows you to integrate with dbt (data build tool), enabling you to perform customized data transformations.
Flexibility: It offers multiple development options to ensure ease of use and flexibility for everyone. These options include an intuitive graphical user interface (UI), an API, a Terraform Provider, and PyAirbyte, enabling you to build data pipelines that best align with your needs.
Data Security: It prioritizes the security of your data by adhering to industry-standard practices. Airbyte incorporates encryption methods to safeguard data in transit and at rest. Additionally, it utilizes robust access controls and authentication mechanisms, guaranteeing data access for only authorized users.
Monitoring and Alerting Capabilities: It allows you to integrate with data monitoring platforms like Datadog and OpenTelemetry. This lets you monitor the health and performance of your data pipelines, ensuring timely detection of issues.
Key Takeaways
This article has covered the concept of stale data, its impact on businesses, and various approaches to prevent it. Stale data can result in various issues, such as operational inefficiencies, financial losses, and poor customer experience. Therefore, you should take proactive measures to leverage accurate, reliable data to drive success. By implementing robust data governance, timely syncs, and continuous monitoring, you can mitigate the risks associated with stale data, ensuring your data assets remain valuable and relevant.
💡Suggested Read: