Amplitude is a cross-platform product intelligence solution that helps companies accelerate growth by leveraging customer data to build optimum product experiences. Advertised as the digital optimization system that “helps companies build better products,” it enables companies to make informed decisions by showing how a company’s digital products drive business. Amplitude employs a proprietary Amplitude Behavioral Graph to show customers the impact of various combinations of features and actions on business outcomes.
A cloud data platform, Snowflake Data Cloud provides a warehouse-as-a-service built specifically for the cloud. The Snowflake platform is designed to empower many types of data workloads, and offers secure, immediate, governed access to a comprehensive network of data. Snowflake’s innovative technology goes above the capabilities of the ordinary database, supplying users all the functionality of database storage, query processing, and cloud services in one package.
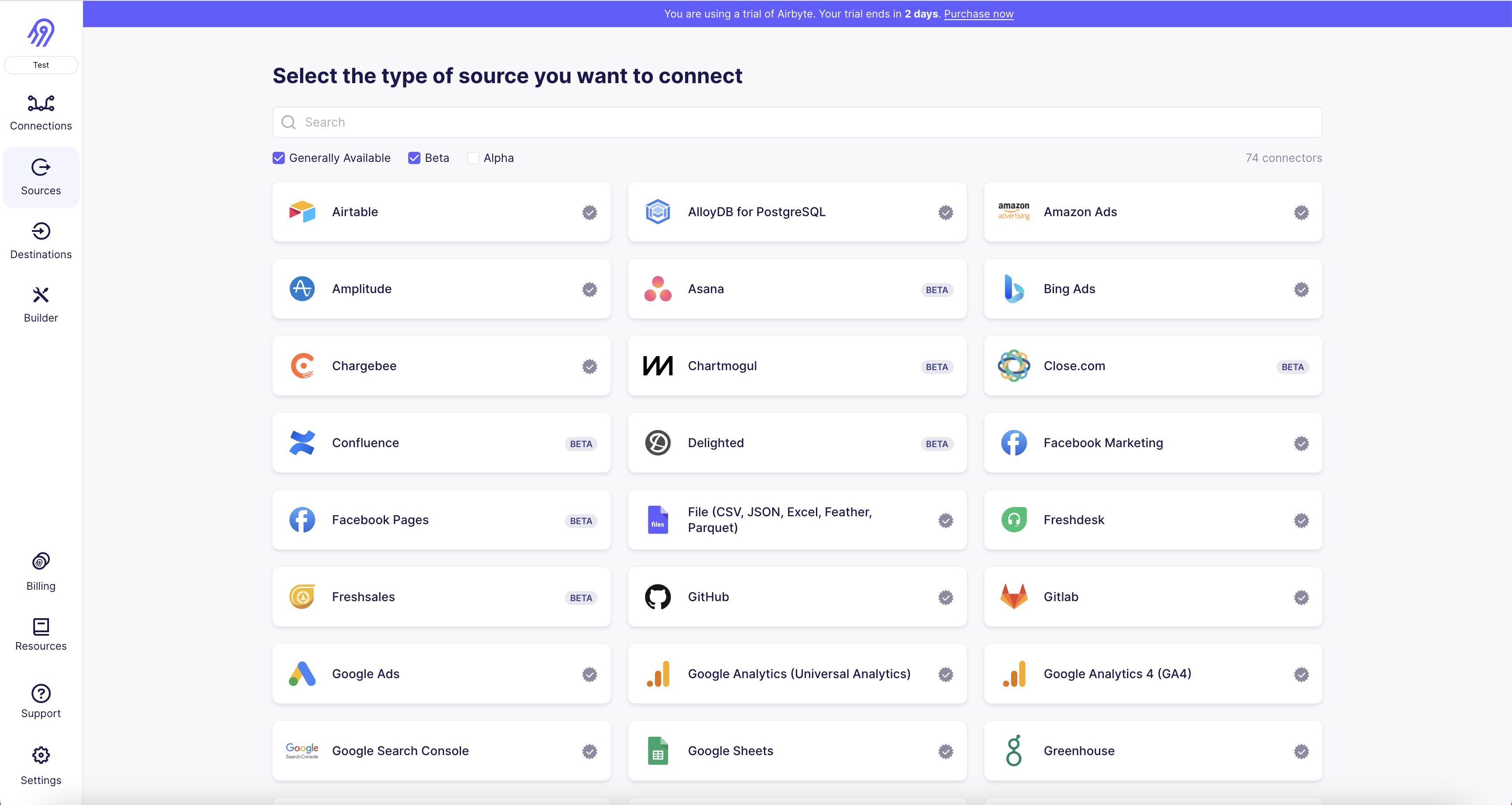
1. First, navigate to the Amplitude source connector page on Airbyte.com.
2. Click on the "Create a new connection" button.
3. Enter a name for your connection and click "Next".
4. Enter your Amplitude API Key and Secret Key in the appropriate fields.
5. Click "Test connection" to ensure that the credentials are correct and the connection is successful.
6. Once the connection is successful, select the data you want to replicate from Amplitude.
7. Choose the replication frequency and the destination where you want to send the data.
8. Click "Create connection" to save your settings and start replicating data from Amplitude to your chosen destination.

1. First, navigate to the Airbyte website and log in to your account.
2. Once you are logged in, click on the "Destinations" tab on the left-hand side of the screen.
3. Scroll down until you find the Snowflake Data Cloud destination connector and click on it.
4. You will be prompted to enter your Snowflake account information, including your account name, username, and password.
5. After entering your account information, click on the "Test" button to ensure that the connection is successful.
6. If the test is successful, click on the "Save" button to save your Snowflake Data Cloud destination connector settings.
7. You can now use the Snowflake Data Cloud destination connector to transfer data from your Airbyte sources to your Snowflake account.
8. To set up a data transfer, navigate to the "Sources" tab on the left-hand side of the screen and select the source you want to transfer data from.
9. Click on the "Create New Connection" button and select the Snowflake Data Cloud destination connector as your destination.
10. Follow the prompts to set up your data transfer, including selecting the tables or data sources you want to transfer and setting up any necessary transformations or mappings.
11. Once you have set up your data transfer, click on the "Run" button to start the transfer process.

With Airbyte, creating data pipelines take minutes, and the data integration possibilities are endless. Airbyte supports the largest catalog of API tools, databases, and files, among other sources. Airbyte's connectors are open-source, so you can add any custom objects to the connector, or even build a new connector from scratch without any local dev environment or any data engineer within 10 minutes with the no-code connector builder.
We look forward to seeing you make use of it! We invite you to join the conversation on our community Slack Channel, or sign up for our newsletter. You should also check out other Airbyte tutorials, and Airbyte’s content hub!
What should you do next?
Hope you enjoyed the reading. Here are the 3 ways we can help you in your data journey:
The key priority of data-driven organizations is seamlessly integrating various data storage systems. Two tools that are leading examples of a source and destination are Amplitude and Snowflake. One is the analytical platform with robust visualization features, and the other is used to store and manage data efficiently on a large scale.
Moving data from Amplitude to Snowflake allows you to perform in-depth analysis and better historical data storage capabilities. It can also enable you to use machine learning techniques for predictive analytics.
This article will discuss the straightforward method to move data from Amplitude to Snowflake.
Amplitude Overview
Amplitude is a unified platform that offers analytics, data integration, and management services. However, it is uniquely designed as an event-based analytics tool. It helps you track user behavior based on their real-time product interaction and identifies trends. Today, Amplitude serves more than 2,000 companies globally. Some organizations that use Amplitude are PayPal, Microsoft, Capital One, and Shopify.
Key features of Amplitude include:
- Interactive Dashboards: Amplitude offers highly customizable and interactive dashboards that allow you to visualize data in a way that best fits your analytical requirements. These dashboards can be customized to emphasize important indicators and trends to help you better understand complex data and make data-driven decisions.
- A/B Testing: The A/B testing feature of Amplitude enables you to test different versions of the product, user interface, and user experience. With this, you can determine which one performs better based on metrics such as click-through rates, retention rates, and more.
- Cohort Analysis: The cohort analysis of Amplitude gives you the ability to group users based on shared characteristics or behaviors and monitor their actions over time. You can understand user retention, lifetime value, and success of particular product changes using this analysis.
Snowflake Overview
Snowflake is a widely known software company that offers many tools for different data management services in one platform. Of all the different services, including data lake, data analytics, and data engineering, Snowflake is mostly known for its data warehousing services. Its cloud-based data warehousing platform is built of shared architecture, allowing you to store and analyze huge datasets in near real-time. For handling data with a focus on adaptability, Snowflake also offers an SQL-based interface for querying structured and semi-structured data.
Key features of Snowflake include:
- Cloud Provider Agnostic: Snowflake is available on three major cloud providers, including Google Cloud Platform (GCP), Amazon Web Services (AWS), and Microsoft Azure. Therefore, you have the flexibility to deploy on the cloud provider of your choice.
- Diverse Data Format Support: Snowflake supports structured and semi-structured data formats for data processing without using complex technologies like Hadoop or Hive. You can store data in various formats, including JSON, Avro, ORC, Parquet, and XML, using VARIANT data types, with a size limit of 16GB.
- Time-Travel: Snowflake allows you to track historical changes of data over time. With its time travel feature, you can find out how your data table looked at any time. This is essential for data auditing, data loss, and recovery from accidental change.
Why Migrate From Amplitude to Snowflake?
Both tools have their unique use cases, features, and functionalities. Therefore, you might want to migrate data from Amplitude to Snowflake for many reasons. Here are some of the key reasons:
- Dedicated Storage System: Snowflake enables you to centralize your data from products and services. Migration data from Amplitude to Snowflake can help you store historical user engagement data.
- Product Development: Bringing data from Amplitude into Snowflake allows you to combine different datasets like customer feedback and support tickets. This can collectively help you identify the bottlenecks to enhance product development.
- Machine Learning: Amplitude provides valuable insights into user behavior and product interactions. However, the environment of Snowflake is more conducive to complex data science techniques. Snowflake supports ML and allows you to seamlessly integrate with other data science platforms, such as Apache Spark and TensorFlow, to lead more precise predictive analysis and personalization strategies to optimize business performance.
Using Airbyte to Connect Amplitude to Snowflake
You can use Airbyte to automate the process of connecting Amplitude to Snowflake. Airbyte is a data integration tool that streamlines the process of extracting and loading data from different sources to a destination of your choice. With its easy-to-use interface and workflow management capabilities, you can connect Amplitude to Snowflake in a few clicks.
Here is a step-by-step guide on using Airbyte to connect both tools:
Step 1: Configure Amplitude As Source
- Sign up or log in to your Airbyte account.

- After successfully logging in, you'll be redirected to the home page of the Airbyte cloud platform.
- Click on the Sources section from the left navigation bar.
- On the Sources page, use the Search bar from the top and type in Amplitude.
- Click on the connector card appearing on the search result.

- You'll be redirected to the Create a source page. Fill in the API Key, Secret Key, and Replication Start Date there.

- Additionally, toggle the Optional fields section and configure the Data region and Request time range according to your requirements.
- Click on Set up source.
Step 2: Configure Snowflake As Destination
- After successfully setting up and configuring the source as Amplitude, click on the Destinations tab from the left navigation bar.
- Type in Snowflake in the Search field and click on the connector card you see on the search results.
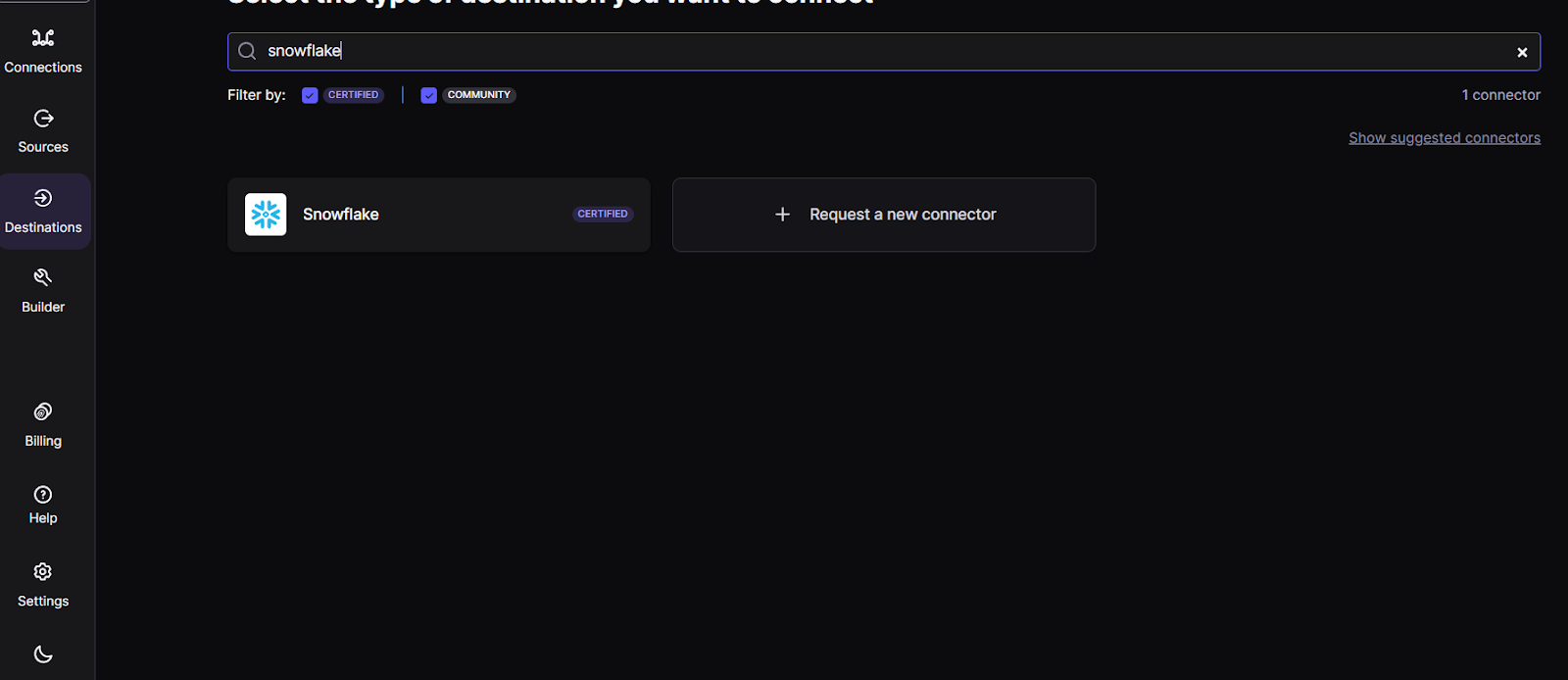
- You'll see the Create a destination page. Fill in the fields including Host, Role, Warehouse, Database, Default Schema and Username.
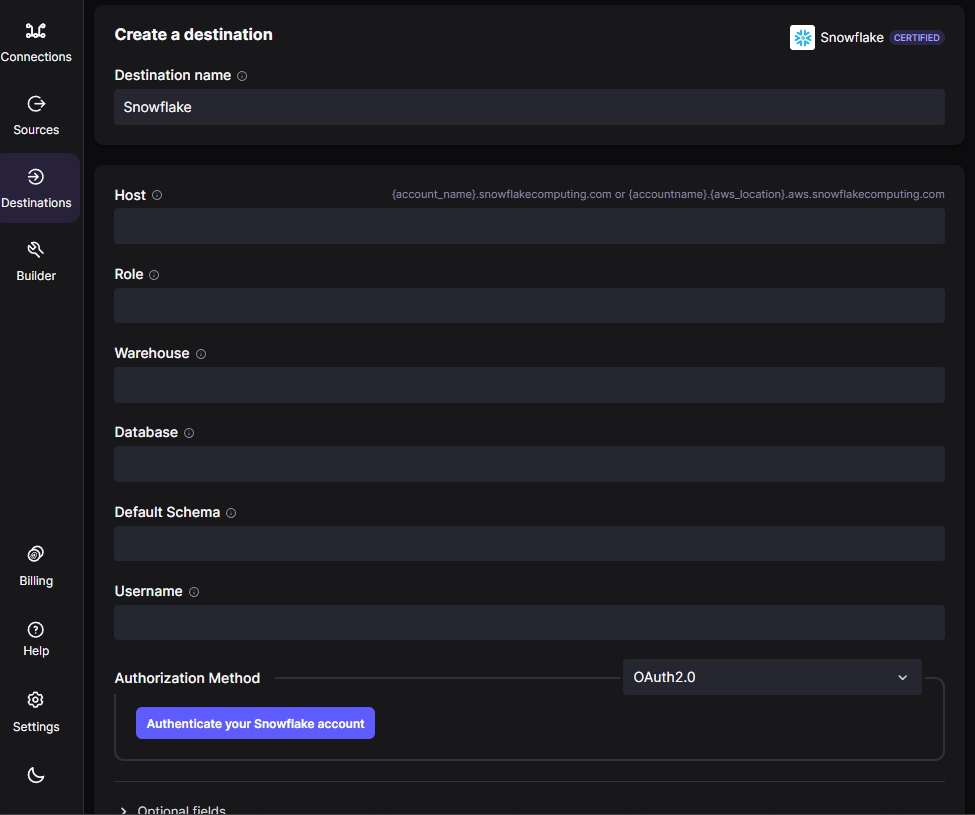
- Select the Authorization Method between OAuth2.0, Key Pair Authentication, Username, and Password.
- Click on Set up destination.
Step 3: Create a Connection Between Amplitude And Snowflake
- Now that you have created a source and destination in Airbyte, you must establish a connection between them. You can select the Create a new connection option after creating the destination or simply click on the Connections tab on the navigation bar.

- Click on Amplitude from Step 1 as the source and Snowflake from Step 2 as the destination to create a connection.
- Configure connection details and provide Connection Name. According to your requirements, you can also tweak other details, including the Streams section, sync mode, and Replication frequency.
- Click on Set up connection > sync now to start synchronization.
That's it. You have connected Amplitude to Snowflake using Airbyte without doing much manual intervention between the two tools.
Another method you can use to migrate data from Amplitude to Snowflake is using the native user interface of the Amplitude platform. The native method is somewhat similar to the method mentioned above, where you need to provide details of your destination to set up the integration. You can learn more about how to natively export data from Amplitude and import it to Snowflake here. However, it doesn’t provide the benefits that Airbyte does.
Benefits of Using Airbyte to Connect Amplitude to Snowflake
Airbyte offers a lot of benefits for migrating data from Amplitude to Snowflake. Some of the key ones are mentioned below:
- Pre-built Connectors: Airbyte provides over 350+ pre-built connectors for data integration. These connectors are templates that usually handle most of the integration and compatibility tasks for you, which reduces setup time and complexity. In addition, using the connectors, you can connect with any data source and destination of your choice and manage all your data integration requirements from one platform.
- Open source: Along with its cloud edition, Airbyte provides an open-source environment for data integration. With over 15,000+ community members and 800+ active contributors, its open-source community has a robust ecosystem to build community-driven connectors.
- Customizable Pipelines: With Airbyte, you can manage data pipelines your way. It provides three ways to deal with data pipelines: User interface, Terraform provider, and API. The user interface option allows you to manage the data pipeline with a few clicks using Airbyte's UI. The Terraform provider option provides features to programmatically build your pipelines with full control. Lastly, as its name suggests, the API option provides an option to handle pipelines via application programming interfaces (APIs).
- Diverse Solutions: It provides three enterprise solutions for your diverse requirements, including Airbyte Cloud, Airbyte Self-Managed, and Powered by Airbyte.
Conclusion
You have learned an easy way to move data from Amplitude to Snowflake using Airbyte. With its robust event-based analytics, Amplitude provides detailed insights into user behavior and product interaction. On the other hand, Snowflake provides robust data warehousing capabilities that complement the analytical strengths of Amplitude.
Airbyte's data integration capabilities further enhance the combination of both platforms. Its extensive library of pre-built connectors, open-source nature, and customizable pipelines address the diverse requirements of modern data-driven organizations, not only for Amplitude to Snowflake but for any data source and destination of your choice.
What should you do next?
Hope you enjoyed the reading. Here are the 3 ways we can help you in your data journey:
Ready to get started?
Frequently Asked Questions
Amplitude's API provides access to a wide range of data related to user behavior and engagement on digital platforms. The following are the categories of data that can be accessed through Amplitude's API:
1. User data: This includes information about individual users such as their demographics, location, and device type.
2. Event data: This includes data related to user actions such as clicks, page views, and purchases.
3. Session data: This includes information about user sessions such as the duration of the session and the number of events that occurred during the session.
4. Funnel data: This includes data related to user behavior in a specific sequence of events, such as a checkout funnel.
5. Retention data: This includes data related to user retention, such as the percentage of users who return to the platform after a certain period of time.
6. Revenue data: This includes data related to revenue generated by the platform, such as the total revenue and revenue per user.
7. Cohort data: This includes data related to groups of users who share a common characteristic, such as the date they signed up for the platform.