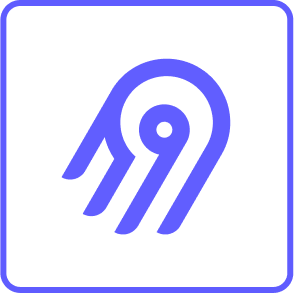

Building your pipeline or Using Airbyte
Airbyte is the only open source solution empowering data teams to meet all their growing custom business demands in the new AI era.
- Inconsistent and inaccurate data
- Laborious and expensive
- Brittle and inflexible
- Reliable and accurate
- Extensible and scalable for all your needs
- Deployed and governed your way
Start syncing with Airbyte in 3 easy steps within 10 minutes



Take a virtual tour
Demo video of Airbyte Cloud
Demo video of AI Connector Builder
What sets Airbyte Apart
Modern GenAI Workflows
Move Large Volumes, Fast
An Extensible Open-Source Standard
Full Control & Security
Fully Featured & Integrated
Enterprise Support with SLAs
What our users say
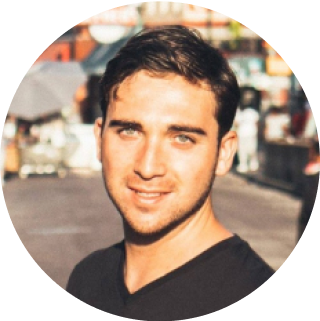
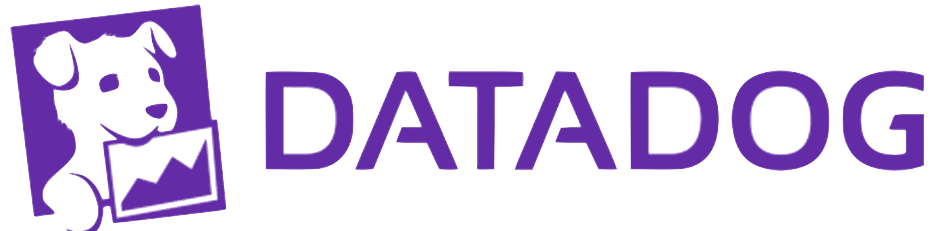
"The intake layer of Datadog’s self-serve analytics platform is largely built on Airbyte.Airbyte’s ease of use and extensibility allowed any team in the company to push their data into the platform - without assistance from the data team!"
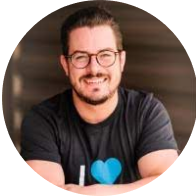
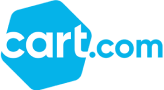
“Airbyte helped us accelerate our progress by years, compared to our competitors. We don’t need to worry about connectors and focus on creating value for our users instead of building infrastructure. That’s priceless. The time and energy saved allows us to disrupt and grow faster.”
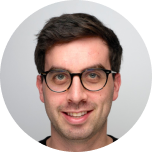

“We chose Airbyte for its ease of use, its pricing scalability and its absence of vendor lock-in. Having a lean team makes them our top criteria. The value of being able to scale and execute at a high level by maximizing resources is immense”
Before you start, familiarize yourself with the Datadog API. You’ll need to use the API to retrieve the data you want to move to SQL Server. Check the Datadog API documentation to find the appropriate endpoints and data formats. You may need to create an API key in your Datadog account to authenticate your requests.
- Install SQL Server: Make sure you have Microsoft SQL Server installed and running.
- Create a Database: Create a new database on your SQL Server instance to store the data from Datadog.
- Design the Database Schema: Define the tables and columns that will store the Datadog data, ensuring they match the structure of the data you’ll be retrieving from the API.
- Choose a Programming Language: Select a programming language that you are comfortable with and that can make HTTP requests and connect to SQL Server (e.g., Python, C#, PowerShell).
- Fetch Data: Write a script that uses the Datadog API to fetch the data you want. You’ll need to handle pagination if you’re dealing with large datasets.
- Parse the Data: Parse the JSON response from the Datadog API to extract the data you need.
- Connect to SQL Server: In the same script, establish a connection to your SQL Server database using the appropriate library for your programming language (e.g., pyodbc for Python, System.Data.SqlClient for C#).
- Prepare the Data: Transform the data into a format suitable for insertion into the SQL Server database, matching the schema you designed.
- Insert the Data: Write SQL INSERT statements to add the data to your SQL Server database. Use parameterized queries to avoid SQL injection attacks.
- Automate the Process: To keep your SQL Server database up-to-date, schedule your script to run at regular intervals (e.g., using Cron jobs on Linux or Task Scheduler on Windows).
- Error Handling: Implement error handling in your script to manage any potential issues during the data transfer process.
- Logging: Add logging to your script to keep track of the data transfer status and to troubleshoot any issues that may arise.
- Test the Script: Run the script manually to ensure that it correctly fetches data from Datadog and inserts it into your SQL Server database.
- Monitor: After deploying the script, monitor its execution and the data integrity in your SQL Server database to ensure everything is working as expected.
Document the Process: Write documentation for your data transfer process, including how the script works, the schedule, and any monitoring or alerting systems you have in place.
Example Script Outline (Python)
Here’s a very high-level outline of what a Python script might look like:
import requests
import pyodbc
# Datadog API setup
api_key = 'your_api_key'
app_key = 'your_app_key'
datadog_endpoint = 'https://api.datadoghq.com/api/v1/query'
# SQL Server connection setup
conn_str = 'DRIVER={ODBC Driver 17 for SQL Server};SERVER=your_server;DATABASE=your_db;UID=your_user;PWD=your_password'
conn = pyodbc.connect(conn_str)
cursor = conn.cursor()
# Fetch data from Datadog
response = requests.get(datadog_endpoint, params={'api_key': api_key, 'application_key': app_key, 'query': 'your_query'})
data = response.json()
# Parse and insert data into SQL Server
for entry in data['series']:
# Transform the data as needed
transformed_data = transform_data(entry)
# Insert into SQL Server
cursor.execute("INSERT INTO your_table (column1, column2) VALUES (?, ?)", transformed_data)
conn.commit()
# Close the connection
cursor.close()
conn.close()
Remember to replace placeholders like your_api_key, your_server, your_db, your_user, your_password, and your_query with your actual Datadog API keys, SQL Server connection details, and query parameters.
FAQs
What is ETL?
ETL, an acronym for Extract, Transform, Load, is a vital data integration process. It involves extracting data from diverse sources, transforming it into a usable format, and loading it into a database, data warehouse or data lake. This process enables meaningful data analysis, enhancing business intelligence.
Datadog is a monitoring and analytics tool for information technology (IT) and DevOps teams that can be used for performance metrics as well as event monitoring for infrastructure and cloud services. The software can monitor services such as servers, databases and appliances Datadog monitoring software is available for on-premises deployment or as Software as a Service (SaaS). Datadog supports Windows, Linux and Mac operating systems. Support for cloud service providers includes AWS, Microsoft Azure, Red Hat OpenShift, and Google Cloud Platform.
Datadog's API provides access to a wide range of data related to monitoring and analytics of IT infrastructure and applications. The following are the categories of data that can be accessed through Datadog's API:
1. Metrics: Datadog's API provides access to a vast collection of metrics related to system performance, network traffic, application performance, and more.
2. Logs: The API allows users to retrieve logs generated by various applications and systems, which can be used for troubleshooting and analysis.
3. Traces: Datadog's API provides access to distributed traces, which can be used to identify performance bottlenecks and optimize application performance.
4. Events: The API allows users to retrieve events generated by various systems and applications, which can be used for alerting and monitoring purposes.
5. Dashboards: Users can retrieve and manage dashboards created in Datadog, which can be used to visualize and analyze data from various sources.
6. Monitors: The API allows users to create, update, and manage monitors, which can be used to alert on specific conditions or events.
7. Synthetic tests: Datadog's API provides access to synthetic tests, which can be used to simulate user interactions with applications and systems to identify performance issues.
Overall, Datadog's API provides a comprehensive set of data that can be used to monitor and optimize IT infrastructure and applications.
What is ELT?
ELT, standing for Extract, Load, Transform, is a modern take on the traditional ETL data integration process. In ELT, data is first extracted from various sources, loaded directly into a data warehouse, and then transformed. This approach enhances data processing speed, analytical flexibility and autonomy.
Difference between ETL and ELT?
ETL and ELT are critical data integration strategies with key differences. ETL (Extract, Transform, Load) transforms data before loading, ideal for structured data. In contrast, ELT (Extract, Load, Transform) loads data before transformation, perfect for processing large, diverse data sets in modern data warehouses. ELT is becoming the new standard as it offers a lot more flexibility and autonomy to data analysts.
What should you do next?
Hope you enjoyed the reading. Here are the 3 ways we can help you in your data journey:
Datadog is a monitoring and analytics tool for information technology (IT) and DevOps teams that can be used for performance metrics as well as event monitoring for infrastructure and cloud services. The software can monitor services such as servers, databases and appliances Datadog monitoring software is available for on-premises deployment or as Software as a Service (SaaS). Datadog supports Windows, Linux and Mac operating systems. Support for cloud service providers includes AWS, Microsoft Azure, Red Hat OpenShift, and Google Cloud Platform.
Microsoft SQL Server is a relational database management (RDBMS) built by Microsoft. As a database server, its primary function is to store and retrieve data upon the request of other software applications, either from the same computer or a different computer across a network—including the internet. To serve the needs of different audiences and workload sizes, Microsoft offers multiple editions (at least 12) of its Microsoft SQL Server.
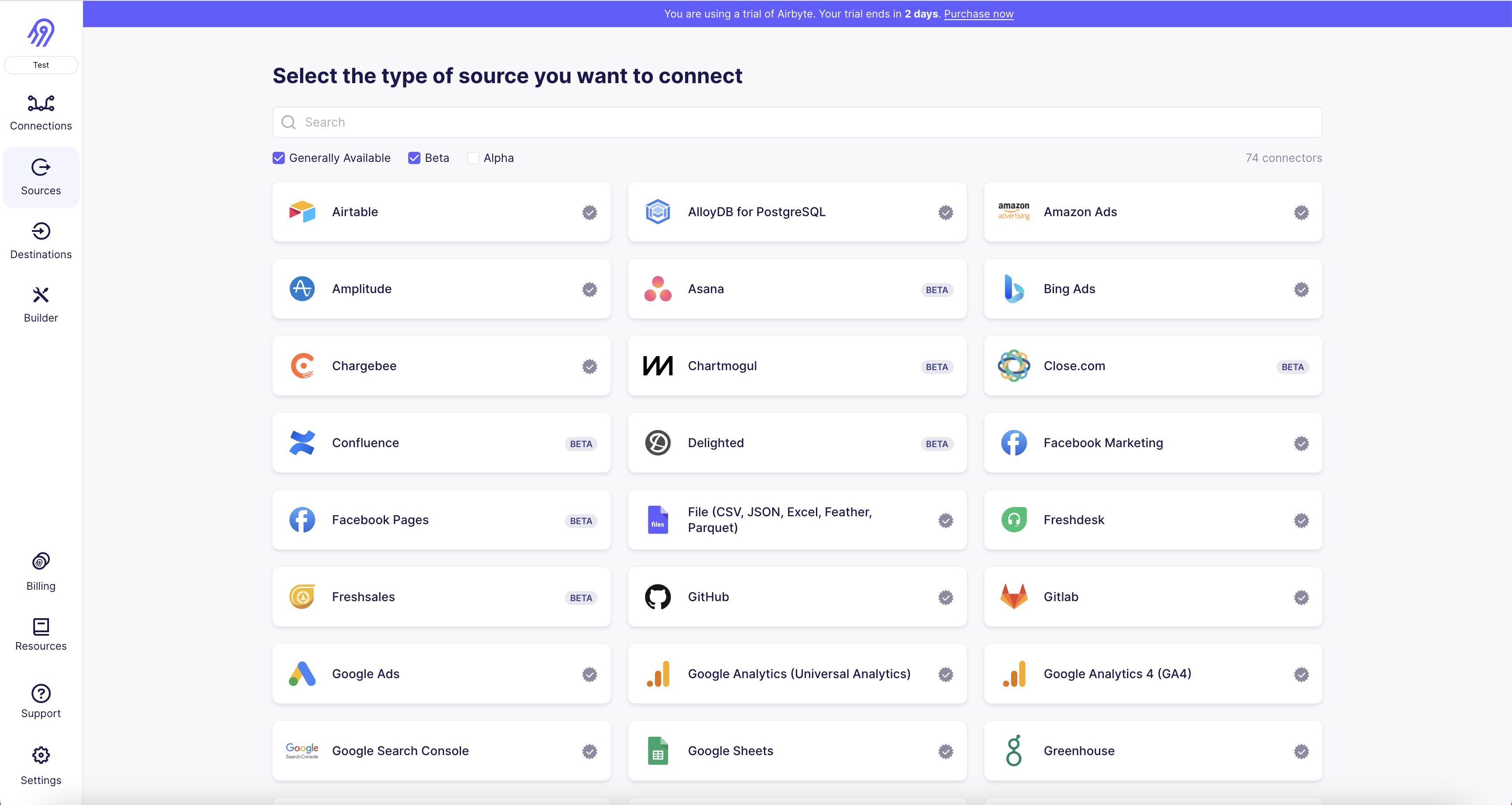
1. First, navigate to the Airbyte dashboard and click on "Sources" in the left-hand menu.
2. Click on the "New Source" button in the top right corner of the screen.
3. Select "Datadog" from the list of available sources.4. Enter a name for your Datadog source connector and click "Next".
5. Enter your Datadog API key and application key in the appropriate fields.
6. Click "Test Connection" to ensure that your credentials are correct and that Airbyte can connect to your Datadog account.
7. Once the connection is successful, click "Create" to save your Datadog source connector.
8. You can now use your Datadog source connector to create a new Airbyte pipeline or add it to an existing one.
9. To create a new pipeline, click on "Pipelines" in the left-hand menu and then click "New Pipeline".
10. Select your Datadog source connector as the source and choose your destination connector.
11. Follow the prompts to configure your pipeline and start syncing data between Datadog and your destination.

1. Open the Airbyte platform and navigate to the "Destinations" tab on the left-hand side of the screen.
2. Scroll down until you find the "MSSQL - SQL Server" connector and click on it.
3. Click on the "Create new destination" button.
4. Fill in the required information, including the destination name, host, port, database name, username, and password.
5. Click on the "Test connection" button to ensure that the connection is successful.
6. Once the connection is successful, click on the "Save" button to save the destination.
7. Navigate to the "Sources" tab on the left-hand side of the screen and select the source that you want to connect to the MSSQL - SQL Server destination.
8. Click on the "Create new connection" button.
9. Select the MSSQL - SQL Server destination that you just created from the drop-down menu.
10. Fill in the required information for the source, including the source name, host, port, database name, username, and password.
11. Click on the "Test connection" button to ensure that the connection is successful.
12. Once the connection is successful, click on the "Save" button to save the connection.13. You can now start syncing data from your source to your MSSQL - SQL Server destination.

With Airbyte, creating data pipelines take minutes, and the data integration possibilities are endless. Airbyte supports the largest catalog of API tools, databases, and files, among other sources. Airbyte's connectors are open-source, so you can add any custom objects to the connector, or even build a new connector from scratch without any local dev environment or any data engineer within 10 minutes with the no-code connector builder.
We look forward to seeing you make use of it! We invite you to join the conversation on our community Slack Channel, or sign up for our newsletter. You should also check out other Airbyte tutorials, and Airbyte’s content hub!
What should you do next?
Hope you enjoyed the reading. Here are the 3 ways we can help you in your data journey:
What should you do next?
Hope you enjoyed the reading. Here are the 3 ways we can help you in your data journey:
Ready to get started?
Frequently Asked Questions
Datadog's API provides access to a wide range of data related to monitoring and analytics of IT infrastructure and applications. The following are the categories of data that can be accessed through Datadog's API:
1. Metrics: Datadog's API provides access to a vast collection of metrics related to system performance, network traffic, application performance, and more.
2. Logs: The API allows users to retrieve logs generated by various applications and systems, which can be used for troubleshooting and analysis.
3. Traces: Datadog's API provides access to distributed traces, which can be used to identify performance bottlenecks and optimize application performance.
4. Events: The API allows users to retrieve events generated by various systems and applications, which can be used for alerting and monitoring purposes.
5. Dashboards: Users can retrieve and manage dashboards created in Datadog, which can be used to visualize and analyze data from various sources.
6. Monitors: The API allows users to create, update, and manage monitors, which can be used to alert on specific conditions or events.
7. Synthetic tests: Datadog's API provides access to synthetic tests, which can be used to simulate user interactions with applications and systems to identify performance issues.
Overall, Datadog's API provides a comprehensive set of data that can be used to monitor and optimize IT infrastructure and applications.