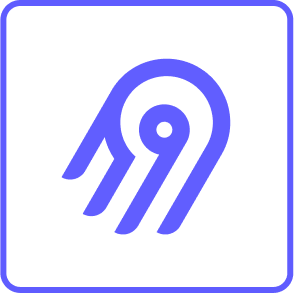

Building your pipeline or Using Airbyte
Airbyte is the only open source solution empowering data teams to meet all their growing custom business demands in the new AI era.
- Inconsistent and inaccurate data
- Laborious and expensive
- Brittle and inflexible
- Reliable and accurate
- Extensible and scalable for all your needs
- Deployed and governed your way
Start syncing with Airbyte in 3 easy steps within 10 minutes



Take a virtual tour
Demo video of Airbyte Cloud
Demo video of AI Connector Builder
What sets Airbyte Apart
Modern GenAI Workflows
Move Large Volumes, Fast
An Extensible Open-Source Standard
Full Control & Security
Fully Featured & Integrated
Enterprise Support with SLAs
What our users say
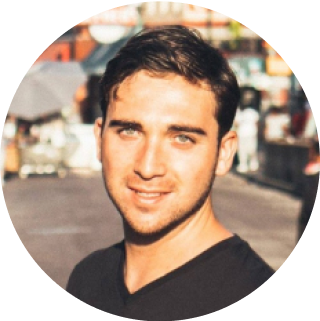
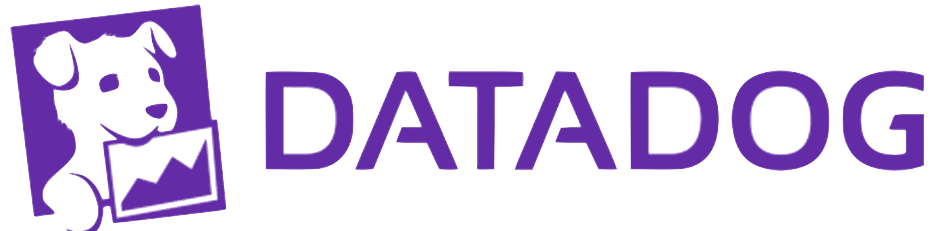
"The intake layer of Datadog’s self-serve analytics platform is largely built on Airbyte.Airbyte’s ease of use and extensibility allowed any team in the company to push their data into the platform - without assistance from the data team!"
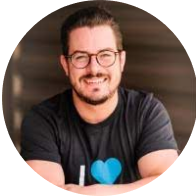
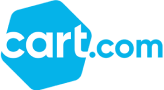
“Airbyte helped us accelerate our progress by years, compared to our competitors. We don’t need to worry about connectors and focus on creating value for our users instead of building infrastructure. That’s priceless. The time and energy saved allows us to disrupt and grow faster.”
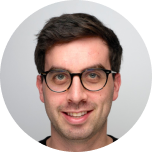

“We chose Airbyte for its ease of use, its pricing scalability and its absence of vendor lock-in. Having a lean team makes them our top criteria. The value of being able to scale and execute at a high level by maximizing resources is immense”
1. Identify the Data to Export: Decide which tables or data sets you need to export from MySQL.
2. Choose Export Format: BigQuery supports CSV, JSON, Avro, and Parquet formats. Choose a format that suits your needs (CSV is commonly used for simplicity).
3. Export the Data:
- Connect to your MySQL database using a command-line tool or a database management tool like phpMyAdmin.
- Use the `mysqldump` command to export your data. For example, to export a table to a CSV file, you can use:
```sh
SELECT * FROM your_table_name
INTO OUTFILE '/path_to_export/your_table_name.csv'
FIELDS TERMINATED BY ','
ENCLOSED BY '"'
LINES TERMINATED BY '\n';
```
- Make sure that the account used to run the `mysqldump` command has the necessary permissions to access the data and write to the file system.
1. Clean and Format Data: Ensure that the data is clean (e.g., no null bytes, properly escaped newlines, etc.) and conforms to BigQuery’s data types and format requirements.
2. Split Large Files: If you have very large CSV files, consider splitting them into smaller chunks to make the upload process more manageable and to avoid timeouts.
3. Compress Files (Optional): Compress the CSV files using GZIP to reduce upload time and storage costs in BigQuery.
1. Create a Google Cloud Storage Bucket:
- Go to the Google Cloud Console.
- Navigate to "Storage" and create a new bucket.
- Set the storage class and location according to your needs.
2. Upload Files to GCS:
- Use the `gsutil cp` command to upload your files to the GCS bucket:
```sh
gsutil cp /path_to_export/*.csv gs://your-bucket-name/
```
- Ensure that you have the necessary permissions to upload files to the GCS bucket.
1. Create a Dataset in BigQuery:
- Go to the BigQuery console.
- Create a new dataset where you will store your imported tables.
2. Create Table Schema:
- Define the schema for your BigQuery table, matching the structure of the MySQL data you exported.
- You can create the schema manually in the BigQuery UI or define it in a JSON file.
3. Load Data from GCS to BigQuery:
- In the BigQuery UI, navigate to your dataset and click on "Create table".
- Set the "Create table from" option to "Google Cloud Storage" and provide the path to your CSV files in the bucket.
- Choose the file format and specify the schema.
- Configure additional settings like field delimiter, encoding, etc., as per your CSV format.
- Click on "Create table" to start the import process.
4. Verify Data Import:
- Once the data is imported, run some queries to ensure that it looks correct and matches the source data in MySQL.
1. Remove Temporary Files:
- After confirming the successful import, you can delete the exported files from your local system and the GCS bucket to avoid unnecessary storage charges.
2. Monitor Cost and Performance:
- Check the BigQuery billing and performance to understand the cost implications of the data import and subsequent queries.
FAQs
What is ETL?
ETL, an acronym for Extract, Transform, Load, is a vital data integration process. It involves extracting data from diverse sources, transforming it into a usable format, and loading it into a database, data warehouse or data lake. This process enables meaningful data analysis, enhancing business intelligence.
MySQL is an SQL (Structured Query Language)-based open-source database management system. An application with many uses, it offers a variety of products, from free MySQL downloads of the most recent iteration to support packages with full service support at the enterprise level. The MySQL server, while most often used as a web database, also supports e-commerce and data warehousing applications and more.
MySQL provides access to a wide range of data types, including:
1. Numeric data types: These include integers, decimals, and floating-point numbers.
2. String data types: These include character strings, binary strings, and text strings.
3. Date and time data types: These include date, time, datetime, and timestamp.
4. Boolean data types: These include true/false or yes/no values.
5. Spatial data types: These include points, lines, polygons, and other geometric shapes.
6. Large object data types: These include binary large objects (BLOBs) and character large objects (CLOBs).
7. Collection data types: These include arrays, sets, and maps.
8. User-defined data types: These are custom data types created by the user.
Overall, MySQL's API provides access to a wide range of data types, making it a versatile tool for managing and manipulating data in a variety of applications.
What is ELT?
ELT, standing for Extract, Load, Transform, is a modern take on the traditional ETL data integration process. In ELT, data is first extracted from various sources, loaded directly into a data warehouse, and then transformed. This approach enhances data processing speed, analytical flexibility and autonomy.
Difference between ETL and ELT?
ETL and ELT are critical data integration strategies with key differences. ETL (Extract, Transform, Load) transforms data before loading, ideal for structured data. In contrast, ELT (Extract, Load, Transform) loads data before transformation, perfect for processing large, diverse data sets in modern data warehouses. ELT is becoming the new standard as it offers a lot more flexibility and autonomy to data analysts.
What should you do next?
Hope you enjoyed the reading. Here are the 3 ways we can help you in your data journey: