

Building your pipeline or Using Airbyte
Airbyte is the only open source solution empowering data teams to meet all their growing custom business demands in the new AI era.
- Inconsistent and inaccurate data
- Laborious and expensive
- Brittle and inflexible
- Reliable and accurate
- Extensible and scalable for all your needs
- Deployed and governed your way
Start syncing with Airbyte in 3 easy steps within 10 minutes



Take a virtual tour
Demo video of Airbyte Cloud
Demo video of AI Connector Builder
What sets Airbyte Apart
Modern GenAI Workflows
Move Large Volumes, Fast
An Extensible Open-Source Standard
Full Control & Security
Fully Featured & Integrated
Enterprise Support with SLAs
What our users say

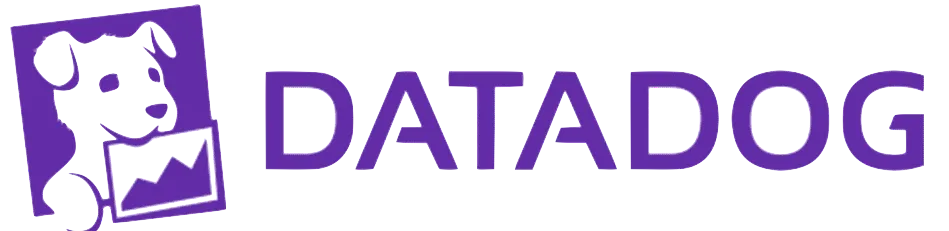
"The intake layer of Datadog’s self-serve analytics platform is largely built on Airbyte.Airbyte’s ease of use and extensibility allowed any team in the company to push their data into the platform - without assistance from the data team!"


“Airbyte helped us accelerate our progress by years, compared to our competitors. We don’t need to worry about connectors and focus on creating value for our users instead of building infrastructure. That’s priceless. The time and energy saved allows us to disrupt and grow faster.”
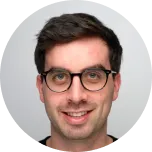

“We chose Airbyte for its ease of use, its pricing scalability and its absence of vendor lock-in. Having a lean team makes them our top criteria. The value of being able to scale and execute at a high level by maximizing resources is immense”
1. Download Kafka: Go to the Apache Kafka website and download the latest version of Kafka.
2. Extract Kafka: Unzip the downloaded file to your desired location.
3. Start ZooKeeper: Kafka uses ZooKeeper, so you need to start it before starting Kafka.
```shell
bin/zookeeper-server-start.sh config/zookeeper.properties
```
4. Start Kafka Server: Open another terminal and run the Kafka server.
```shell
bin/kafka-server-start.sh config/server.properties
```
1. Create Topic: Create a Kafka topic where the data from PostgreSQL will be published.
```shell
bin/kafka-topics.sh --create --topic postgres-data --bootstrap-server localhost:9092 --replication-factor 1 --partitions 1
```
1. Install PostgreSQL: If you haven't already, install PostgreSQL on your system.
2. Create a Database: Create a database and tables that you want to move data from.
3. Enable Logical Replication: Modify `postgresql.conf` to enable logical replication by setting `wal_level` to `logical`.
4. Create Publication: For PostgreSQL 10 and above, you can create a publication for the tables you want to watch.
```sql
CREATE PUBLICATION my_publication FOR TABLE my_table;
```
1. Set Up Your Development Environment: Make sure you have a suitable programming environment with necessary dependencies installed, such as a PostgreSQL driver and Kafka client library.
2. Database Connection: Write code to connect to your PostgreSQL database.
3. Polling or Trigger-Based Data Retrieval: Decide on a method for retrieving data from PostgreSQL. You can poll the database at intervals or use triggers to act on data changes.
4. Read Data: Write a function to read data from PostgreSQL. This could be new rows or updated rows depending on your application.
5. Format Data: Format the data into a structure suitable for sending to Kafka.
6. Kafka Producer: Write a Kafka producer in your application that connects to the Kafka cluster.
7. Send Data to Kafka: Send the formatted data to the Kafka topic you created earlier.
8. Error Handling: Implement error handling to deal with any issues during data retrieval or publishing.
9. Logging: Add logging to your application for monitoring and debugging purposes.
1. Compile and Run: Compile your application and run it.
2. Monitor: Monitor the application for any errors and ensure data is being published to Kafka successfully.
1. Consume Messages: Use a Kafka consumer to consume messages from the Kafka topic to validate that data is being moved correctly.
```shell
bin/kafka-console-consumer.sh --bootstrap-server localhost:9092 --topic postgres-data --from-beginning
```
2. Check Data Integrity: Verify that the data in Kafka matches the source data in PostgreSQL.
1. Deploy: Deploy your custom application to a suitable production environment.
2. Scale: If necessary, scale your Kafka cluster and application to handle the load.
1. Set Up Monitoring: Set up monitoring for both your Kafka cluster and custom application to ensure they are running smoothly.
2. Regular Maintenance: Perform regular maintenance checks on both PostgreSQL and Kafka.
Additional Considerations:
Security: Ensure that your Kafka cluster and PostgreSQL database are secured, using SSL/TLS and SASL if necessary.
Transaction Support: If your application requires transaction support, make sure to handle transactions properly in your custom application.
Data Serialization: Choose an appropriate data serialization format for Kafka messages (e.g., JSON, Avro, Protobuf).
Backpressure Handling: Implement backpressure handling in your application in case Kafka cannot handle the rate of incoming messages.
Remember, this approach requires a significant amount of custom development and testing. It's important to consider the trade-offs between building a custom solution and using existing third-party connectors that may offer additional features and robustness.
First, let’s see what connectors are at our disposal:
```
confluent connect plugin list
```
Look for “PostgresSource” in the output. If you don’t see it, double-check your Confluent CLI setup.
Before we configure our connector, let’s examine its properties:
```
confluent connect plugin describe PostgresSource
```
This command provides a wealth of information. Take some time to read through it – understanding these properties will help you troubleshoot issues later.
Now comes the fun part – creating your configuration file. I recommend using a text editor you’re comfortable with. Let’s call our file postgres-to-kafka.json:
```json
{
"name": "pg-to-kafka-stream",
"config": {
"connector.class": "PostgresSource",
"kafka.auth.mode": "SERVICE_ACCOUNT",
"kafka.service.account.id": "sa-abc123",
"topic.prefix": "pg_",
"connection.host": "your-db-host.com",
"connection.port": "5432",
"connection.user": "your_username",
"connection.password": "your_password",
"db.name": "your_database",
"table.whitelist": "users,orders",
"timestamp.column.name": "updated_at",
"incrementing.column.name": "id",
"output.data.format": "JSON",
"db.timezone": "UTC",
"tasks.max": "1",
"mode": "timestamp+incrementing"
}
}
```
Some notes on this configuration:
- The name field is how you’ll refer to this connector later. Choose something memorable!
- kafka.auth.mode: Using a service account is generally more secure than API keys.
- topic.prefix: This helps organize your Kafka topics. I like using short prefixes like “pg_”.
- mode: “timestamp+incrementing” is often a good choice for capturing both new and updated rows.
Remember, this is just a starting point. You may need to adjust based on your specific PostgreSQL setup.
With your configuration file ready, it’s time to create the connector:
```
confluent connect cluster create --config-file postgres-to-kafka.json
```
If successful, you’ll get a response with your connector’s ID. Save this ID – you’ll need it for monitoring.
Connecting systems can be tricky. Let’s check on our connector’s status:
```
confluent connect cluster status <your-connector-id>
```
Look for “RUNNING” in the output. If you see “FAILED”, check the error messages and revisit your configuration.
Now for the moment of truth – is data flowing into Kafka? Let’s check:
```
confluent kafka topic consume pg_users --from-beginning
```
Replace “pg_users” with whatever your topic name is based on your prefix and table name.
If you see JSON data streaming by, congratulations! You’ve successfully connected PostgreSQL to Kafka.
FAQs
What is ETL?
ETL, an acronym for Extract, Transform, Load, is a vital data integration process. It involves extracting data from diverse sources, transforming it into a usable format, and loading it into a database, data warehouse or data lake. This process enables meaningful data analysis, enhancing business intelligence.
An object-relational database management system, PostgreSQL is able to handle a wide range of workloads, supports multiple standards, and is cross-platform, running on numerous operating systems including Microsoft Windows, Solaris, Linux, and FreeBSD. It is highly extensible, and supports more than 12 procedural languages, Spatial data support, Gin and GIST Indexes, and more. Many webs, mobile, and analytics applications use PostgreSQL as the primary data warehouse or data store.
PostgreSQL gives access to a wide range of data types, including:
1. Numeric data types: This includes integers, floating-point numbers, and decimal numbers.
2. Character data types: This includes strings, text, and character arrays.
3. Date and time data types: This includes dates, times, and timestamps.
4. Boolean data types: This includes true/false values.
5. Network address data types: This includes IP addresses and MAC addresses.
6. Geometric data types: This includes points, lines, and polygons.
7. Array data types: This includes arrays of any of the above data types.
8. JSON and JSONB data types: This includes JSON objects and arrays.
9. XML data types: This includes XML documents.
10. Composite data types: This includes user-defined data types that can contain multiple fields of different data types.
Overall, PostgreSQL's API provides access to a wide range of data types, making it a versatile and powerful tool for data management and analysis.
What is ELT?
ELT, standing for Extract, Load, Transform, is a modern take on the traditional ETL data integration process. In ELT, data is first extracted from various sources, loaded directly into a data warehouse, and then transformed. This approach enhances data processing speed, analytical flexibility and autonomy.
Difference between ETL and ELT?
ETL and ELT are critical data integration strategies with key differences. ETL (Extract, Transform, Load) transforms data before loading, ideal for structured data. In contrast, ELT (Extract, Load, Transform) loads data before transformation, perfect for processing large, diverse data sets in modern data warehouses. ELT is becoming the new standard as it offers a lot more flexibility and autonomy to data analysts.
What should you do next?
Hope you enjoyed the reading. Here are the 3 ways we can help you in your data journey: