
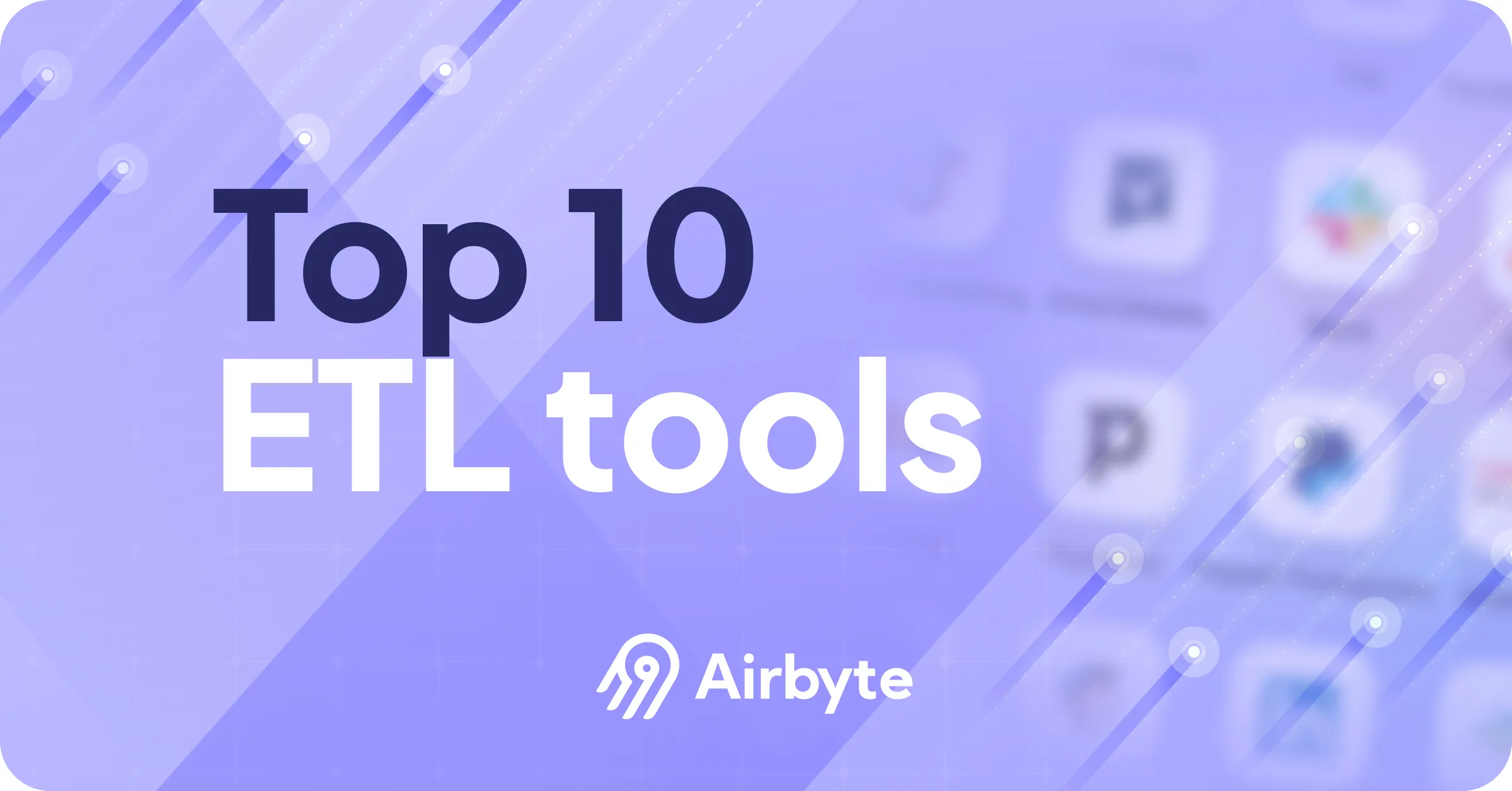
Data is the crucial asset of your organization, driving strategic initiatives, operational efficiency, and customer satisfaction. However, poor data quality can result in inaccurate insights, misguided strategies, and increased operational costs. To overcome these challenges, you can utilize data quality tools that ensure your data is reliable and trustworthy.
The data quality tools market size was USD 2.71 billion in 2024 and is expected to reach USD 4.15 billion by 2031. Investing in the right data quality tools can greatly enhance your business performance and decision-making capabilities. In this article, you'll explore the top five data quality tools.
Summary Of Data Quality Tools We Recommend
Here are the top data quality tools that can help you maintain high standards of data integrity:
- IBM InfoSphere QualityStage
- DQLabs
- Ataccama
- Informatica Data Quality
- WinPure
What are Data Quality Tools?
Data quality tools are software solutions that help you improve and maintain the quality of your data. These tools enable you to automate diverse tasks involved in data quality management, such as data profiling, cleansing, monitoring, and validation. This lets you make better use of your data assets.
Advanced data quality tools also leverage machine learning, particularly anomaly detection algorithms, to identify outliers, resulting in high-quality data. Incorporating these tools into your workflows enhances business intelligence while reducing errors and compliance risks.
5 Best Data Quality Tools Of 2025
Let’s explore the top five data quality tools:
IBM InfoSphere QualityStage

IBM QualityStage is a leading data quality management solution within the IBM InfoSphere Information Server suite. It empowers you to cleanse and manage your data, helping you maintain consistent views of key entities like customers and products. By ensuring data quality, InfoSphere QualityStage minimizes the time required to implement master data management (MDM) and other strategic customer-focused IT initiatives.
Why Do We Recommend?
We recommend QualityStage because of its rich capabilities. Here are a few of them:
Built-in Data Quality Rules: QualityStage provides over 200 built-in data quality rules. This aids in controlling the ingestion of bad data and ensures that only trusted data is loaded into target systems.
Deep Data Profiling: The platform offers deep data profiling capabilities, enabling you to understand the content, quality, and structure of data. This includes column analysis, data classification, quality scores, and relationship analysis.
Data Standardization: InfoSphere QualityStage lets you standardize data from diverse sources into a standard format. This ensures that fields like names, dates, and addresses are consistent across your systems.
Data Matching: It employs probabilistic matching techniques to identify and merge identical records, creating a single, accurate view of data entities.
Pros and Cons
Here are some of the pros and cons of InfoSphere QualityStage:
Pros:
- The platform utilizes machine learning for automatic business-term assignment, which streamlines the data preparation process.
- In addition to the built-in data quality rules, QualityStage lets you create custom rules tailored to specific business requirements.
- QualityStage includes built-in governance that helps you comply with regulations and maintain control over your data.
- The platform supports real-time data processing, enabling you to cleanse and standardize data as it is being ingested.
- It offers versatile deployment options, including on-premises and cloud, providing flexibility to meet your organization's needs.
Cons:
- The platform could be complex to set up and manage, requiring technical expertise and resources.
- Some users find the interface less intuitive than other modern data quality tools.
- Performance may degrade when handling extremely large volumes of data, requiring optimization.
Pricing
IBM InfoSphere QualityStage offers flexible subscription-based pricing. For precise information, you can contact their sales team.
DQLabs
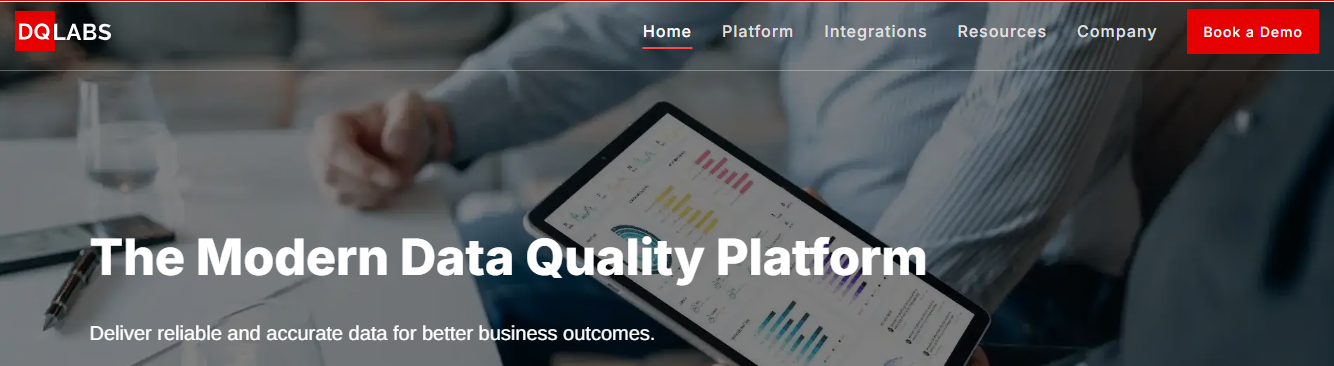
DQLabs is an AI-driven modern data quality platform designed to enhance the accuracy of data for improved business outcomes. It lets you automate quality checks and resolutions through a semantic layer, delivering reliable data for effective reporting and analytics.
Why Do We Recommend?
We suggest DQLabs due to its powerful self-service capabilities. Here are a few of them:
Out-of-the-box Quality Checks: DQLabs provides 50+ data quality measures that assess different aspects of your data, including its health, frequency, and distribution pattern. This ensures that your data is accurate.
Automated Rules Discovery: The platform automatically identifies data quality rules based on patterns and semantics, reducing manual effort and accelerating the data quality process.
End-to-End Automated Lineage: DQLabs provides complete traceability of data throughout its lifecycle, enhancing transparency and accountability.
Alerts Prioritization: DQLabs employs AI/ML-driven anomaly detection to track data quality issues. By analyzing deviations from standard data, it categorizes alerts into high, medium, and low priority levels, ensuring efficient prioritization of issues.
Pros and Cons
Let’s take a look at the pros and cons of DQLabs:
Pros:
- DQLabs offers a no-code setup for ongoing monitoring across your data pipelines.
- You can automate incident detection, impact analysis, and root cause diagnosis for end-to-end observability.
- The ML models within DQLabs analyze data patterns and automatically set appropriate thresholds for data quality checks.
- Pre-configured dashboards enable you to start quickly without extensive setup or resource requirements.
Cons:
- Handling unstructured data can be challenging.
- Customization of reporting features is limited.
Pricing
For specific details, you must contact their sales team.
Ataccama

Ataccama is an AI-powered data quality tool that ensures data accuracy while automating key processes to improve efficiency and minimize errors. It enables you to track data quality, monitor key metrics, and identify issues throughout the data lifecycle. This helps keep your data reliable and secure, enhancing overall operational efficiency.
Why Do We Recommend?
We recommend Ataccama due to its remarkable capabilities. Here are a few of them:
Data Validation: You can implement automated data validation rules to ensure that only consistent and accurate data enters your business systems.
Complete Lineage: The Ataccama lineage scanner provides a comprehensive mapping of your entire data flow, monitoring data quality and identifying anomalies along the way. This guarantees that your data remains accurate and reliable throughout its journey from source to target.
Smart Rule Generation: You can create data quality rules effortlessly with Ataccama's ONE AI agent that converts plain text into actionable rules. It also provides recommendations on where to apply these rules, enhancing workflow efficiency.
Continuous Monitoring: Ataccama lets you detect and resolve issues instantly with automated alerts and notifications, facilitating quick intervention to maintain data quality.
Pros and Cons
Here are some of the pros and cons of Ataccama:
Pros:
- The platform enables you to integrate data quality checks with your existing ETL, CI/CD pipelines, and analytics platforms.
- Ataccama offers a DQ firewall that stops bad data from entering your systems, enhancing overall data quality.
- You can save time by reusing data quality configurations across different environments and technologies.
- The tool prevents unauthorized data modifications through secure access controls, ensuring that your data remains accurate and trustworthy.
- Ataccama enables you to handle billions of records without compromising speed, delivering high performance for large-scale data operations.
Cons:
- Ataccama’s extensive feature set can make it challenging for beginners to learn and utilize effectively.
- Integrating Ataccama with existing systems can be complex and may require technical expertise.
- The tool is generally considered expensive, particularly for businesses with smaller budgets.
Pricing
Ataccama's pricing information is not publicly disclosed. For details on pricing, you can reach out to their sales team directly.
Informatica Data Quality
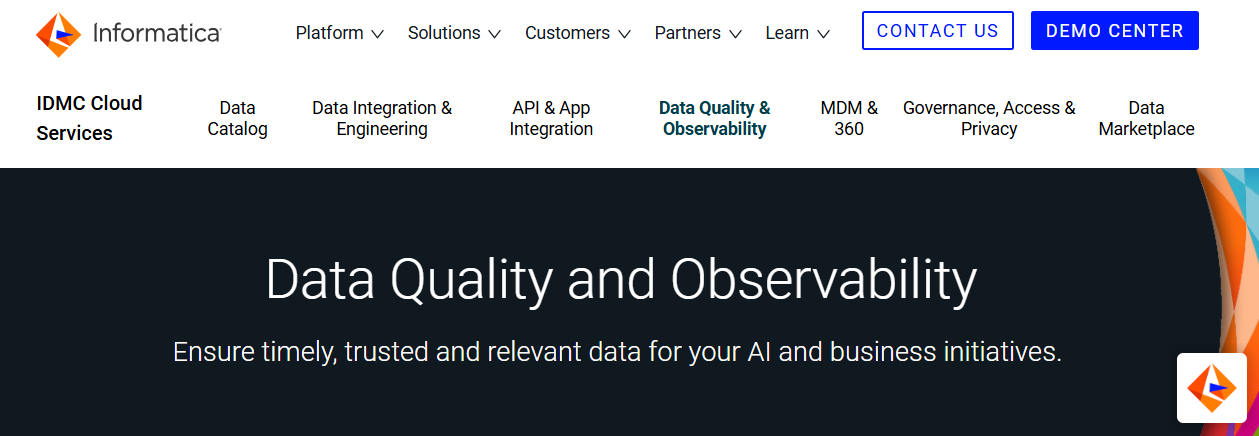
Informatica Data Quality (IDQ) is a data quality management solution that enables you to cleanse and manage the quality of your data. It ensures that your data is accurate and reliable, which is essential for trustworthy analytics and decision-making.
Why Do We Recommend?
We suggest IDQ because of its exceptional features. Here are a few of them:
Data Quality Rules: Informatica Data Quality offers pre-built quality rules that you can run or customize according to your project objectives.
Advanced Data Matching: IDQ offers AI-powered match tuning and rule recommendations that let you address data variations with high accuracy.
Smart Data Quality Insights: The platform lets you automatically profile data, apply rules, identify issues, and monitor data quality through visually engaging scorecards.
Enhanced Data Consistency: IDQ helps you standardize variations in punctuation, formatting, and spelling, removing inconsistencies. This ensures uniformity in key fields like city, state, and ZIP code.
Pros and cons
Let’s take a look at the pros and cons of Informatica Data Quality:
Pros:
- It facilitates safe and secure access to shared and trustworthy data.
- The tool lets you automatically link metadata with business context for a holistic view with greater transparency.
- You can enrich your data with AI-powered curation, adding relevant context to data assets through automated classification, association, and recommendations.
- With CLAIRE AI copilot capabilities, you can automate numerous data management tasks, reducing the risk of errors and enhancing data quality.
Cons:
- Data quality rules do not easily adapt to dynamic data. You often have to write new rules when data changes.
- Customizing and configuring IDQ to meet specific business needs can be time-consuming and resource-intensive.
- The platform's built-in reporting capabilities are limited.
Pricing
Informatica offers consumption-based pricing, where you only pay for the services you need. You can request a quote to get a sample price range based on your specific use case.
WinPure
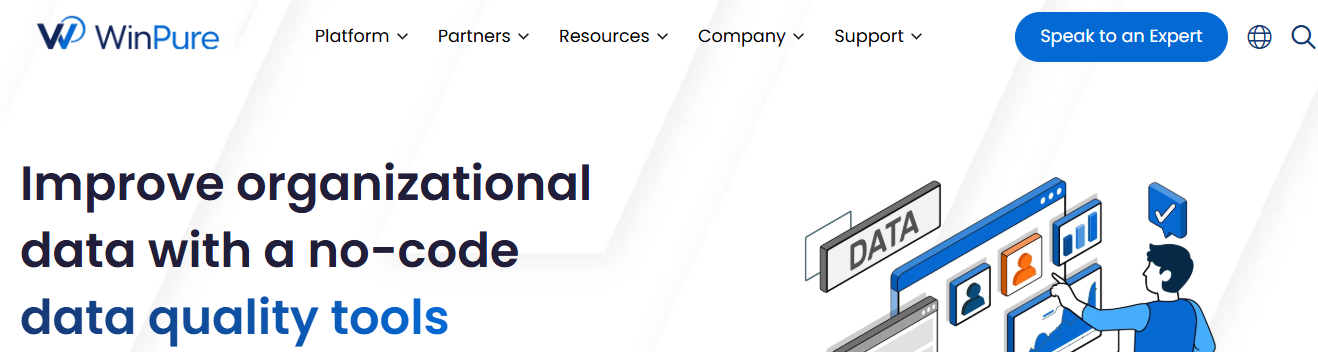
WinPure is a no-code data quality management platform that enables you to enhance the reliability of your data. It empowers you to resolve complex data issues without requiring technical expertise. With its intuitive interface, WinPure significantly reduces the time spent on manual data cleaning, enabling you to focus on strategic initiatives.
Why Do We Recommend?
We recommend WinPure for its advanced functionalities. Here are a few of them:
Custom Cleansing: WinPure offers a built-in word library that empowers you to create custom cleansing definitions. This functionality facilitates precise control over the data cleansing process.
Comprehensive Data Health Analysis: WinPure’s data profiling feature examines the 30 most frequently occurring errors, offering a detailed statistical overview of the data health. It enables you to identify columns with high data quality issues and highlights the most problematic error types.
Advanced Fuzzy Matching: The tool uses advanced fuzzy match algorithms to handle typos, abbreviations, and variations in data entry. This ensures accurate matching of records even when there are minor discrepancies in names, addresses, or other fields.
Address Parsing: WinPure includes an address parser that breaks down complex address strings into individual components (e.g., street names, city names, zip codes). This enhances the quality of address data by standardizing and normalizing elements.
Pros and Cons
Here are some of the pros and cons of WinPure:
Pros:
- WinPure facilitates integration with various popular databases and file applications, including CSV, Excel, XML, SQL Server, Oracle, MySQL, Salesforce, and more.
- You can effortlessly combine duplicate records into a unified master record, preserving all critical information. This ensures a consolidated and clear view of your data.
- WinPure’s robust automation capabilities enable you to pre-schedule data cleaning tasks. Once scheduled, the tool automatically initiates the cleaning and matching processes based on the provided data.
- It lets you streamline the complex process of managing master data by providing flexible data models and collaborative workflows.
Cons:
- WinPure does not support cross-column matching, which might lead to incomplete data analysis.
- It only detects simple patterns and parses data into a single column. This can lead to information loss and inaccurate interpretations.
Pricing
WinPure offers several pricing plans—Small Business, Pro Business, Enterprise Lite, Enterprise, and Enterprise Server.
Key Features Every Data Quality Tool Must Have
Let’s understand the essential features that a data quality tool must possess:
Data Profiling: Data profiling is the process of analyzing data to understand its structure, content, and interrelationships between data. A data quality platform should provide capabilities enabling you to explore and visualize the data, identify patterns and anomalies, and assess the quality of your data assets.
Data Cleansing: Data cleansing is the process of identifying and rectifying errors and inaccuracies in data. A data quality tool should offer comprehensive data cleansing functionalities, such as data standardization, deduplication, and enrichment. It must be flexible enough to let you apply various quality rules and automate the cleansing process to ensure that data quality is consistently maintained over time.
Data Monitoring and Validation: These are crucial components of ongoing data quality management. A data quality platform must facilitate tracking data quality metrics and validating data against established criteria. Further, these features should be customizable, enabling you to define your own data quality thresholds and validation rules according to your business requirements.
Issue Resolution Workflow: A data quality tool should include capabilities for error detection, empowering you to pinpoint data quality issues and their sources. Additionally, the platform should provide root cause analysis that helps you understand the underlying factors contributing to data quality issues.
How to Choose the Right Data Quality Tool?
Here are some tips to help you choose the right data quality tool:
Identify Your Data Quality Requirements: Identify the dimensions of data quality that are most relevant to your organization, such as accuracy, completeness, consistency, and timeliness. This lets you better evaluate and select the tool that aligns with your needs.
Evaluate Tool Capabilities: Different data quality tools offer various functionalities like data profiling, cleansing, standardization, and monitoring. To find the right one for your organization, compare these capabilities against your defined requirements.
Usability: Prioritize platforms that are user-friendly and have an intuitive interface. A tool that is easy to use will encourage adoption across your organization and let your team manage data quality effectively.
Scalability and Flexibility: Ensure the tool can scale with your organization's growth and adapt to changing data quality needs. Look for platforms that offer flexible deployment options, such as cloud-based or on-premises solutions.
Ease of Integration: Consider data quality solutions that are compatible with your existing technology stack and can be implemented without extensive modifications.
Assess Vendor Support: Evaluate the level of customer support provided by the vendor, as well as the availability of a user community or resources for troubleshooting.
Integrate Airbyte into your Data Quality Strategy
Data silos, which are isolated data repositories within your organization, significantly impact data quality. When data is stored in different places, it becomes difficult to ensure its accuracy, leading to incomplete data. To overcome these challenges, you can leverage data integration tools like Airbyte.
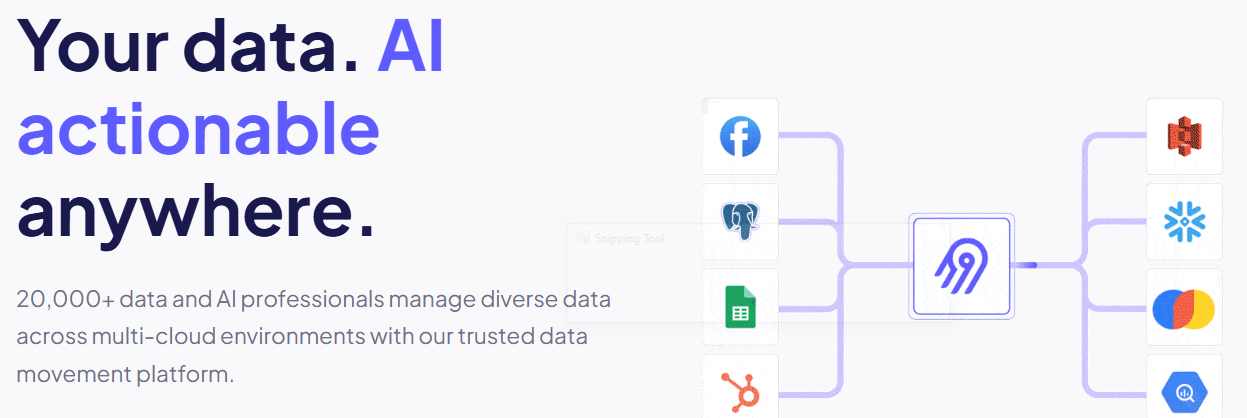
Airbyte offers an extensive catalog of 550+ pre-built connectors that enable you to consolidate data from diverse sources into your preferred destination without the need for extensive coding. In addition, you can utilize Airbyte’s AI Assistant to build custom connectors using Connector Builder and speed up the development process significantly.
Here are some of the key features of Airbyte:
GenAI Workflows: Airbyte supports integration with popular vector databases like Chroma, Pinecone, Qdrant, Milvus, and more. This enables you to simplify your AI workflows by loading semi-structured and unstructured data directly to vector store destinations.
Change Data Capture: Airbyte's CDC feature enables you to replicate only the changes made in the source into your target system. This ensures that the destination systems are continuously updated with the latest changes, reducing the risk of data inconsistencies.
Record Change History: This feature enhances the reliability of your data movement. If any record breaks the sync, Airbyte modifies it during transit, logs the changes, and ensures that the sync completes successfully.
Conclusion
Maintaining high data quality is essential to make informed decisions and drive business success. In this article, you've explored the best data quality tools. Each tool has its unique capabilities and strengths. By evaluating your specific needs and comparing these tools, you can choose the one that best fits your organization's requirements.
What should you do next?
Hope you enjoyed the reading. Here are the 3 ways we can help you in your data journey:
Frequently Asked Questions
What is ETL?
ETL, an acronym for Extract, Transform, Load, is a vital data integration process. It involves extracting data from diverse sources, transforming it into a usable format, and loading it into a database, data warehouse or data lake. This process enables meaningful data analysis, enhancing business intelligence.
This can be done by building a data pipeline manually, usually a Python script (you can leverage a tool as Apache Airflow for this). This process can take more than a full week of development. Or it can be done in minutes on Airbyte in three easy steps: set it up as a source, choose a destination among 50 available off the shelf, and define which data you want to transfer and how frequently.
The most prominent ETL tools to extract data include: Airbyte, Fivetran, StitchData, Matillion, and Talend Data Integration. These ETL and ELT tools help in extracting data from various sources (APIs, databases, and more), transforming it efficiently, and loading it into a database, data warehouse or data lake, enhancing data management capabilities.
What is ELT?
ELT, standing for Extract, Load, Transform, is a modern take on the traditional ETL data integration process. In ELT, data is first extracted from various sources, loaded directly into a data warehouse, and then transformed. This approach enhances data processing speed, analytical flexibility and autonomy.
Difference between ETL and ELT?
ETL and ELT are critical data integration strategies with key differences. ETL (Extract, Transform, Load) transforms data before loading, ideal for structured data. In contrast, ELT (Extract, Load, Transform) loads data before transformation, perfect for processing large, diverse data sets in modern data warehouses. ELT is becoming the new standard as it offers a lot more flexibility and autonomy to data analysts.