Top companies trust Airbyte to centralize their Data



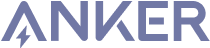
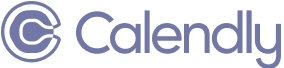
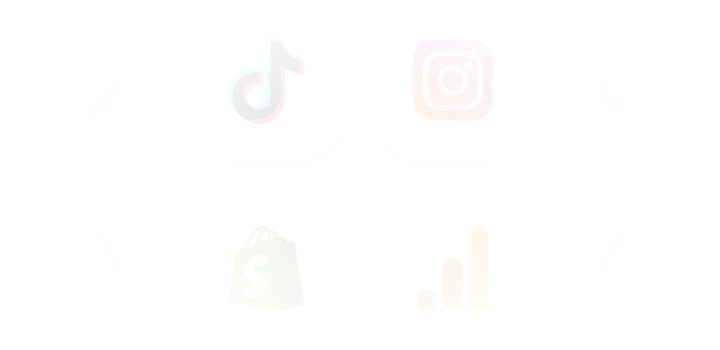
Select your source
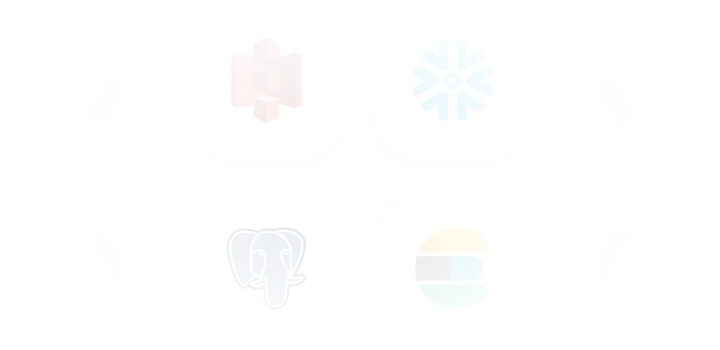
Select your destination
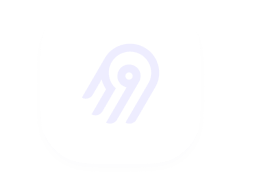
Sync your Data
Ship more quickly with the only solution that fits ALL your needs.
As your tools and edge cases grow, you deserve an extensible and open ELT solution that eliminates the time you spend on building and maintaining data pipelines
Leverage the largest catalog of connectors
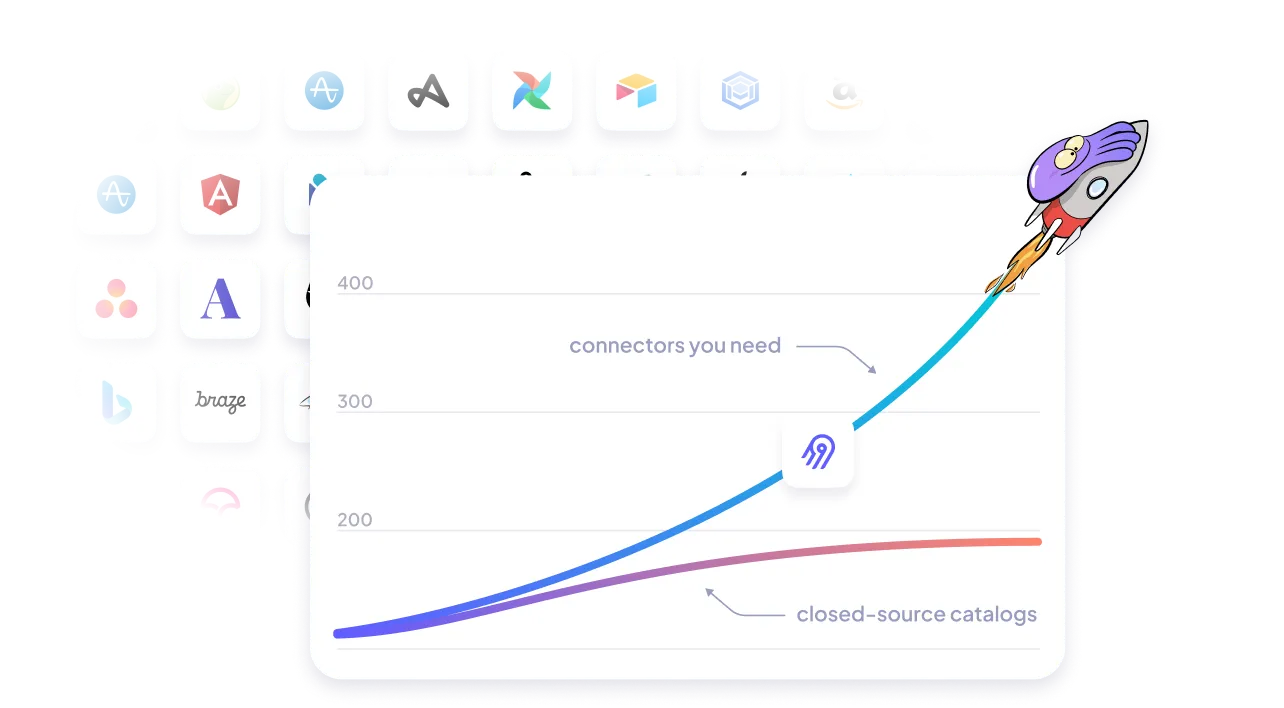
Cover your custom needs with our extensibility
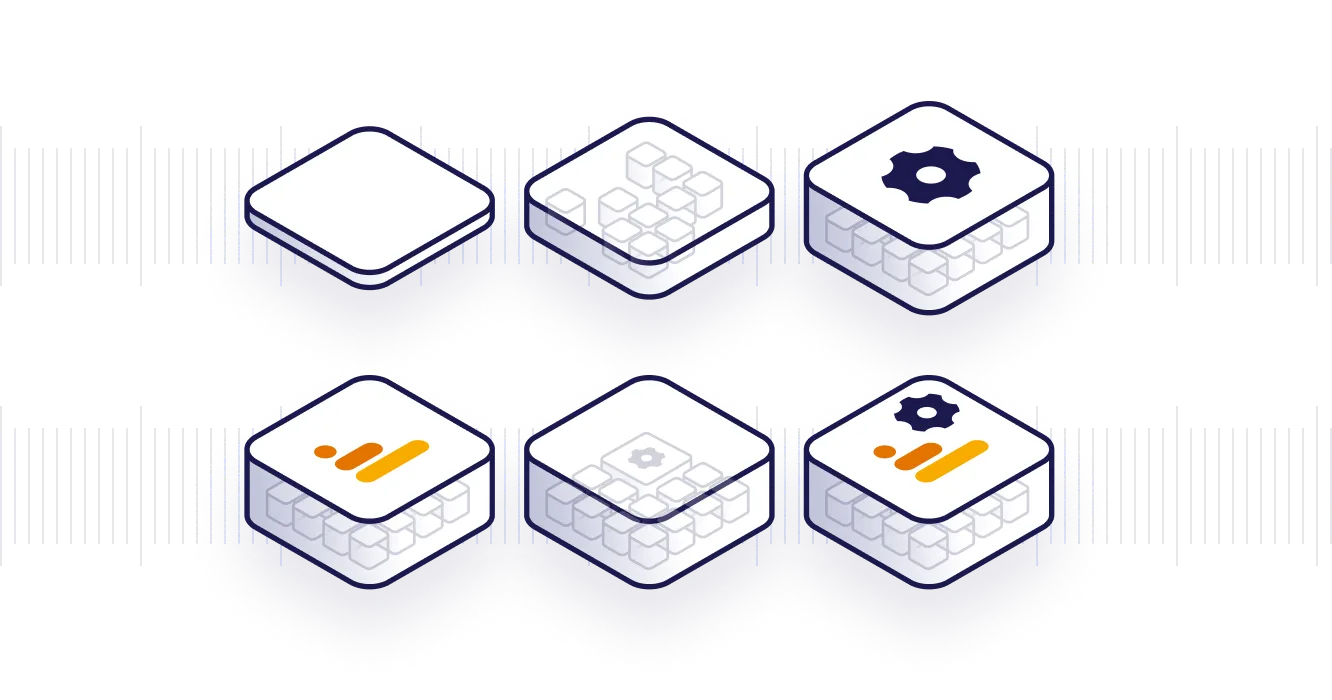
Free your time from maintaining connectors, with automation
- Automated schema change handling, data normalization and more
- Automated data transformation orchestration with our dbt integration
- Automated workflow with our Airflow, Dagster and Prefect integration

Reliability at every level

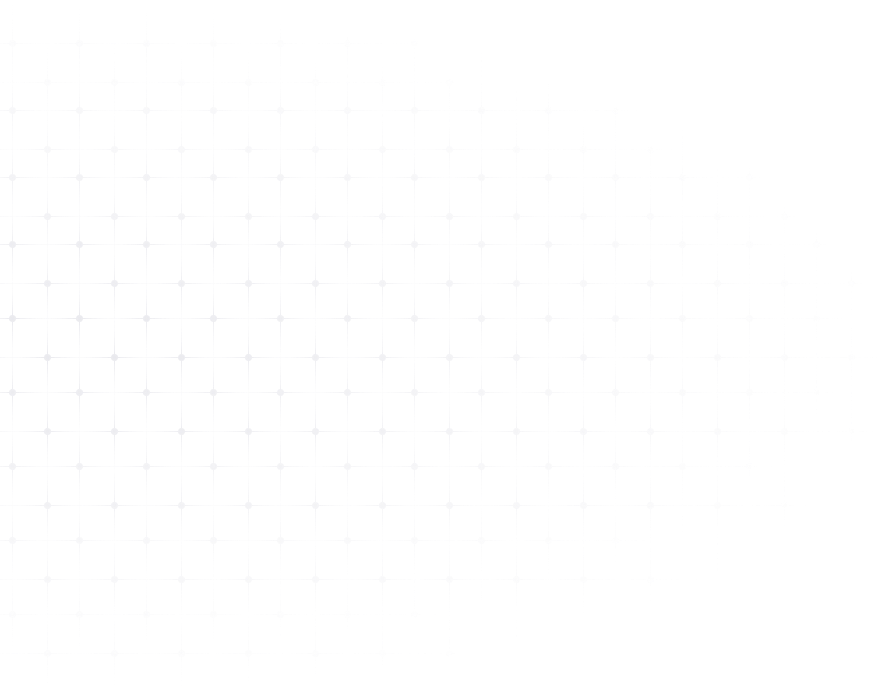
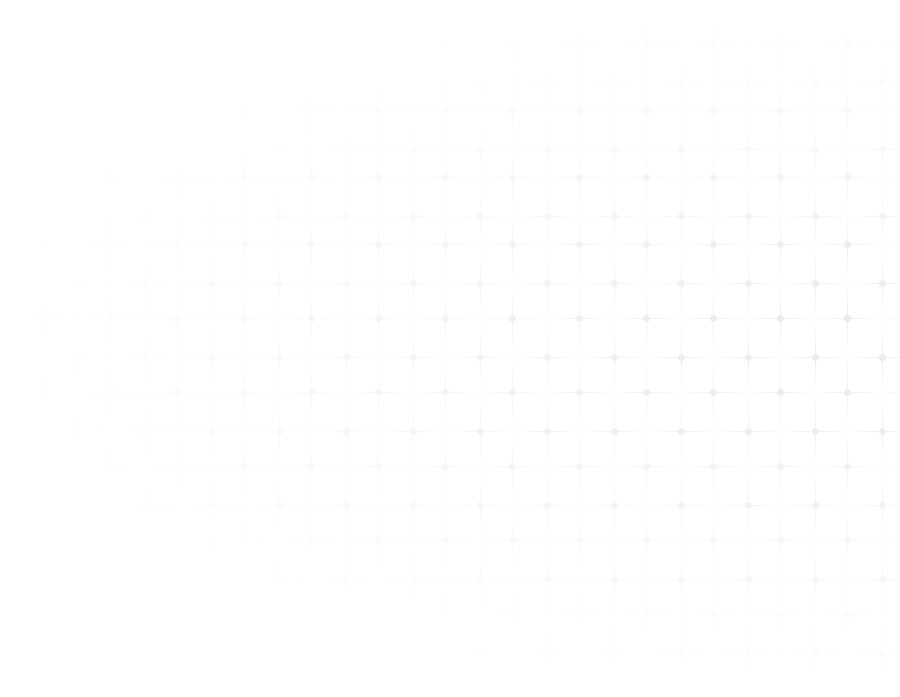

Airbyte Open Source
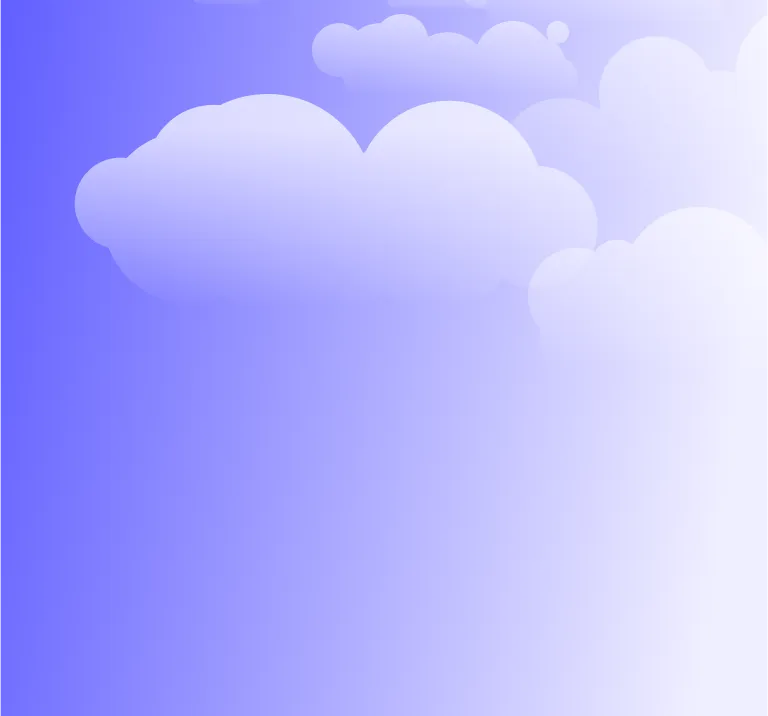
Airbyte Cloud
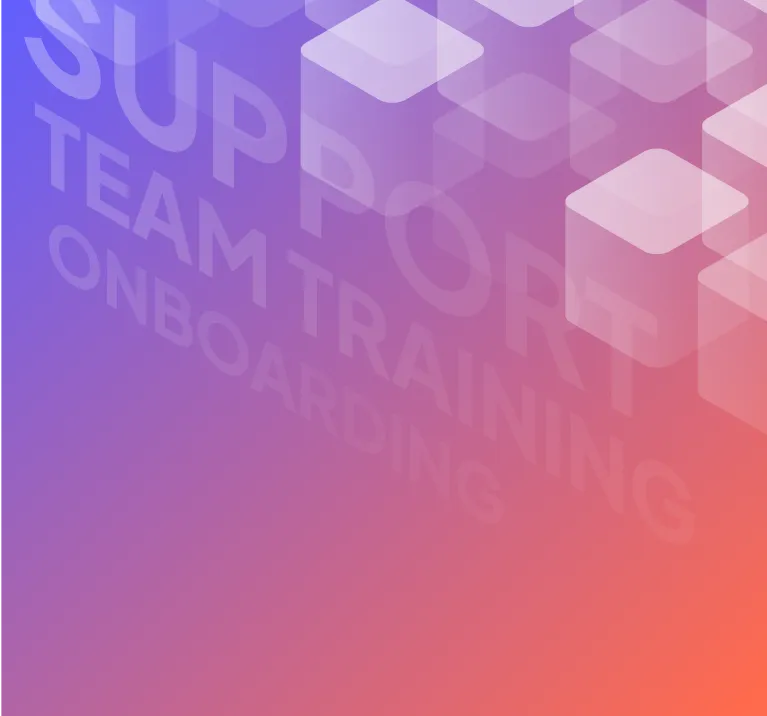
Airbyte Enterprise
Why choose Airbyte as the backbone of your data infrastructure?
Keep your data engineering costs in check
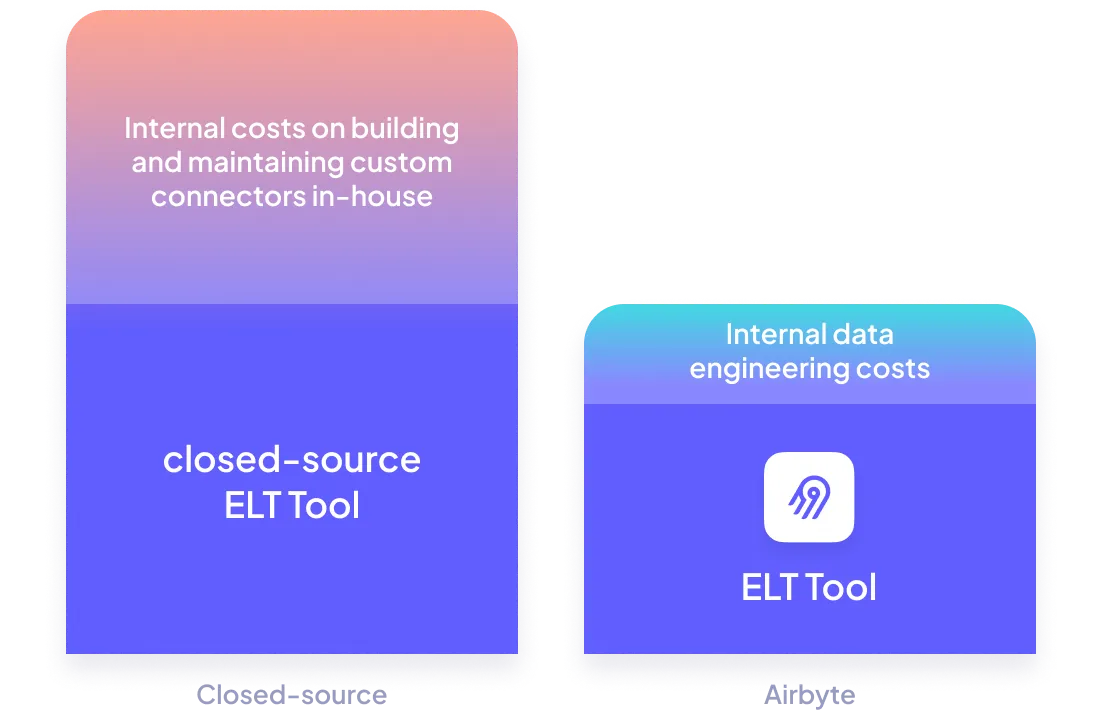
Get Airbyte hosted where you need it to be
- Airbyte Cloud: Have it hosted by us, with all the security you need (SOC2, ISO, GDPR, HIPAA Conduit).
- Airbyte Enterprise: Have it hosted within your own infrastructure, so your data and secrets never leave it.
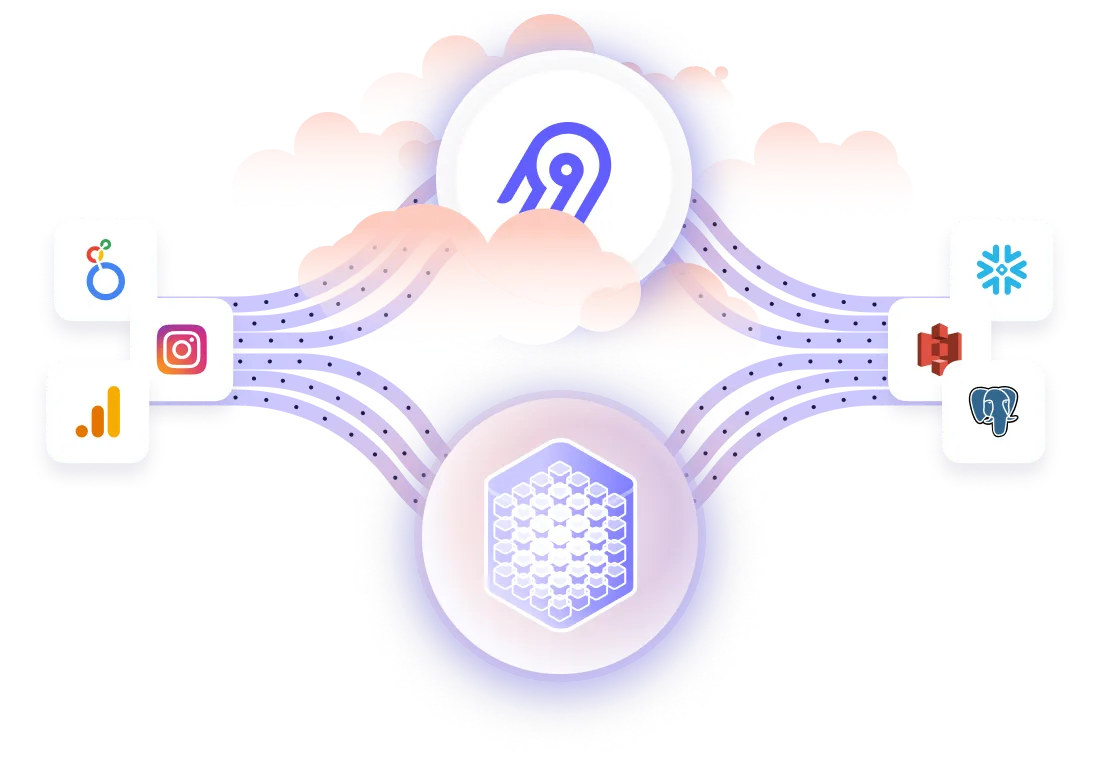
White-glove enterprise-level support
Including for your Airbyte Open Source instance with our premium support.
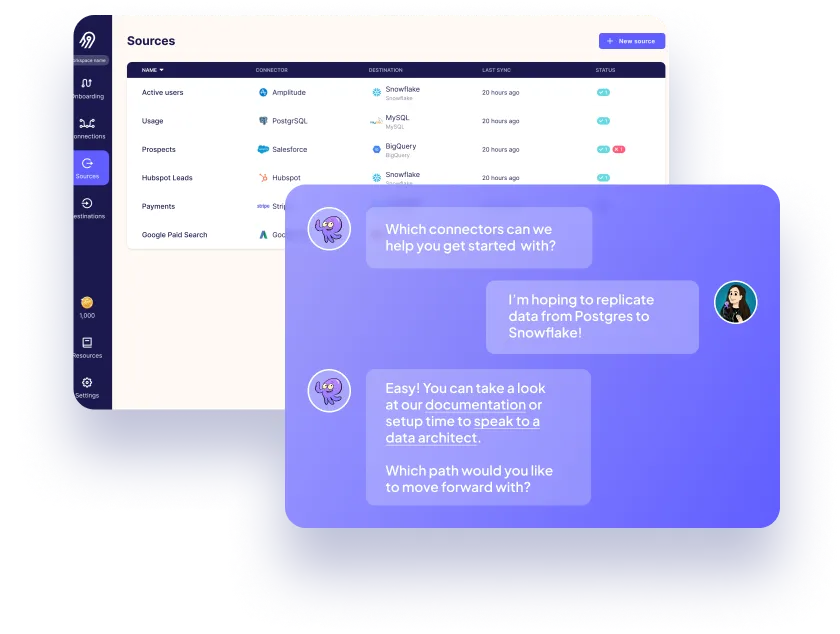
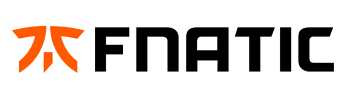
Fnatic, based out of London, is the world's leading esports organization, with a winning legacy of 16 years and counting in over 28 different titles, generating over 13m USD in prize money. Fnatic has an engaged follower base of 14m across their social media platforms and hundreds of millions of people watch their teams compete in League of Legends, CS:GO, Dota 2, Rainbow Six Siege, and many more titles every year.
Ready to get started?
FAQs
What is ETL?
ETL, an acronym for Extract, Transform, Load, is a vital data integration process. It involves extracting data from diverse sources, transforming it into a usable format, and loading it into a database, data warehouse or data lake. This process enables meaningful data analysis, enhancing business intelligence.
RKI stands for the Robert Koch Institute is continuously monitoring the situation, evaluating all available information, estimating the risk for the population in Germany. RKI Corvid provides selected information on COVID-19 available in English. In the connector source RKI Corvid we want to add streams for the states that include history data , incidence rate , cases , deaths and so on.
For huge analytical tables, Apache Iceberg is a high-performance format. Using Apache Iceberg, engines such as Spark, Trino, Flink, Presto, Hive and Impala can safely work with the same tables, at the same time, providing the reliability and simplicity of SQL tables to big data. With Apache Iceberg, you can merge new data, update existing rows, and delete specific rows. Data files can be eagerly rewritten or deleted deltas can be used to make updates faster.
The RKI Covid's API provides access to a wide range of data related to the Covid-19 pandemic in Germany. The data can be categorized into the following categories:
1. Case data: This includes information on the number of confirmed cases, deaths, and recoveries in Germany.
2. Testing data: This includes information on the number of tests conducted, the positivity rate, and the number of tests per capita.
3. Hospitalization data: This includes information on the number of hospitalizations, ICU admissions, and ventilator use.
4. Vaccination data: This includes information on the number of people vaccinated, the number of doses administered, and the percentage of the population vaccinated.
5. Geographic data: This includes information on the number of cases and deaths by state, district, and municipality.
6. Demographic data: This includes information on the age, gender, and ethnicity of Covid-19 patients.
7. Time series data: This includes information on the daily and cumulative number of cases, deaths, and vaccinations over time.
Overall, the RKI Covid's API provides a comprehensive set of data that can be used to track the spread of Covid-19 in Germany and inform public health policies and interventions.
What is ELT?
ELT, standing for Extract, Load, Transform, is a modern take on the traditional ETL data integration process. In ELT, data is first extracted from various sources, loaded directly into a data warehouse, and then transformed. This approach enhances data processing speed, analytical flexibility and autonomy.
Difference between ETL and ELT?
ETL and ELT are critical data integration strategies with key differences. ETL (Extract, Transform, Load) transforms data before loading, ideal for structured data. In contrast, ELT (Extract, Load, Transform) loads data before transformation, perfect for processing large, diverse data sets in modern data warehouses. ELT is becoming the new standard as it offers a lot more flexibility and autonomy to data analysts.