What Is Data as a Product (DaaP): Examples & Purpose
As an organization, you generate and utilize large volumes of data every day. However, when the data is in a raw format, its scope for utilization is limited. To realize its maximum potential, you must change how you treat your data within the organization.
Data as a product (DaaP) represents a shift in thinking, where you transform raw data into high-quality information products. This modifies your data strategy and empowers your employees to make smarter, more informed business decisions, helping you achieve sustainable growth in the long run.
This article provides a detailed overview of data as a product (DaaP) and explores its benefits, components, and practical examples. It also explains how DaaP and data products are different by listing the key differences.
What Is Data as a Product?
Data as a product is an approach that does not consider data as a mere byproduct of operations. It considers data a standalone asset that you curate, manage, and deliver, focusing on quality, usability, and discoverability.
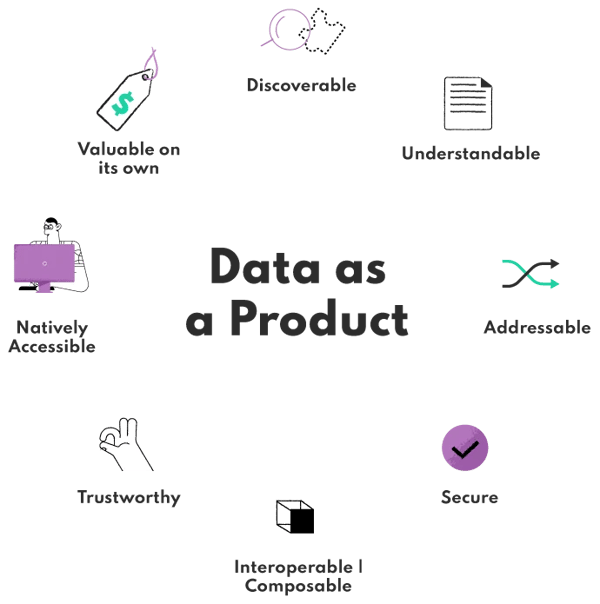
In a DaaP framework, you design data to meet specific user needs, such as internal teams, customers, or partners, and ensure it is reliable, accessible, and actionable. Implementing data as a product emphasizes the importance of data governance, metadata management, and user experience, refining data into a consumable product and fostering innovation.
Benefits of Considering Data as a Product
You can enhance your organization's ability to leverage data effectively by shifting your perspective on data from a raw resource to a valuable product. It can help you get better outcomes across various areas of data management and utilization. Below are some points that explain the benefits of considering data as a product:
Improved Data Quality
By treating data as a product, your organization prioritizes rigorous data quality control measures to maintain high standards. This includes implementing regular data audits, data cleaning and validation processes, establishing data profiling guidelines, and fostering a culture of data accuracy. Consequently, high-quality data builds trust with stakeholders, allows you to conduct more precise data analytics, and minimizes the risks associated with erroneous data.
Enhanced User Experience
The DaaP concept strongly emphasizes delivering data in a user-friendly manner. It encourages the incorporation of intuitive interfaces for exploration, easy accessibility for authorized users, and clear documentation of data sources. This enhances the user experience and makes interacting with and deriving value from data easier. Improved usability also increases data utilization and efficiency of your data teams.
Increased Data Discoverability
When you view data as a product, you must organize and catalog it systematically. It also involves creating well-structured, comprehensive metadata. Doing this ensures that your internal teams or external partners can quickly find and access the data they need, saving their time and effort. Enhanced data discoverability helps streamline your business operations and data processes.
Better Governance and Compliance
The DaaP approach ensures that data governance and compliance are integral to your data management practices. By establishing clear policies, access controls, security protocols, roles, and responsibilities, you can verify whether data is handled according to regulatory requirements and internal standards. This reduces the risk of data breaches, legal penalties, and reputational damage while promoting ethical data usage.
Improved Decision-Making
By treating data as a product, your organization can transform information into actionable insights that drive strategic choices and help mitigate risks. It leads to optimized resource allocation, increased operational efficiency, and the capability to identify new opportunities for growth and innovation, providing a competitive edge.
Data as a Product vs. Data Product vs. Data as a Service
Understanding the differences between data as a product, data product, and data as a service is crucial. Each concept has characteristics and applications that can help your organization’s data utilization strategy.
Components of a Data as a Product Strategy
A robust DaaP strategy encompasses several vital components that work together to enable you to utilize the full potential of your data. Let’s explore these components.
Data Architecture
Data architecture defines the blueprint of your organization’s data flows. It considers data sources, storage, integration and retrieval systems, processing mechanisms, and access methods. A well-structured data architecture supports scalability, flexibility, and seamless data functions throughout the data lifecycle while facilitating advanced analytics.
Data Governance
This framework outlines policies, procedures, and roles to establish data integrity, security, and compliance. Data governance ensures responsible data use, protects sensitive information, and fosters trust in the data's accuracy and relevance. It encompasses data quality standards, access controls, and regulatory adherence that empower you to manage your data ethically while protecting you from hefty fines.
Metadata Management
Effective metadata management involves creating detailed descriptions of your data sets, including definitions, formats, and usage guidelines, cataloging data assets, and maintaining data lineage. This gives you a comprehensive context and documentation of your data assets, allowing you to leverage them productively.
Data Lineage
Data lineage tracks the flow and transformation of data from its origin throughout its lifecycle. It lets you understand how data is derived and ensures traceability for analysis and troubleshooting.
With data lineage, you can also gain visibility into data processes that help you understand dependencies and changes over time. This is crucial for ensuring data accuracy, tracing errors, and maintaining compliance, as it provides a clear audit trail of data movements.
Data Catalogs
Data catalogs are centralized repositories that facilitate the organization and documentation of data assets within your organization. They provide detailed metadata, usage guidelines, and ownership details that help new team members familiarize themselves with the existing infrastructure. A comprehensive data catalog allows you to foster a data-driven culture and promote efficient data utilization.
Data Mesh and Its Relationship to DaaP
Data mesh is a modern data management architecture that helps you address the challenges of scaling data analytics and operations within your organization. It allows for decentralization and domain-oriented ownership of data. One of the four core principles of data mesh is treating data as a product, which fundamentally reshapes how you manage and utilize data.
DaaP emphasizes that each domain, like operations or marketing, is responsible for transforming its respective raw data into well-defined, high-quality datasets that cater to its needs. These data products must be reliable and easily consumable by other domains and teams across your organization. With a product-thinking mindset, you can empower your workforce to tailor data for optimal use by different teams, similar to developing regular products to meet customer needs.
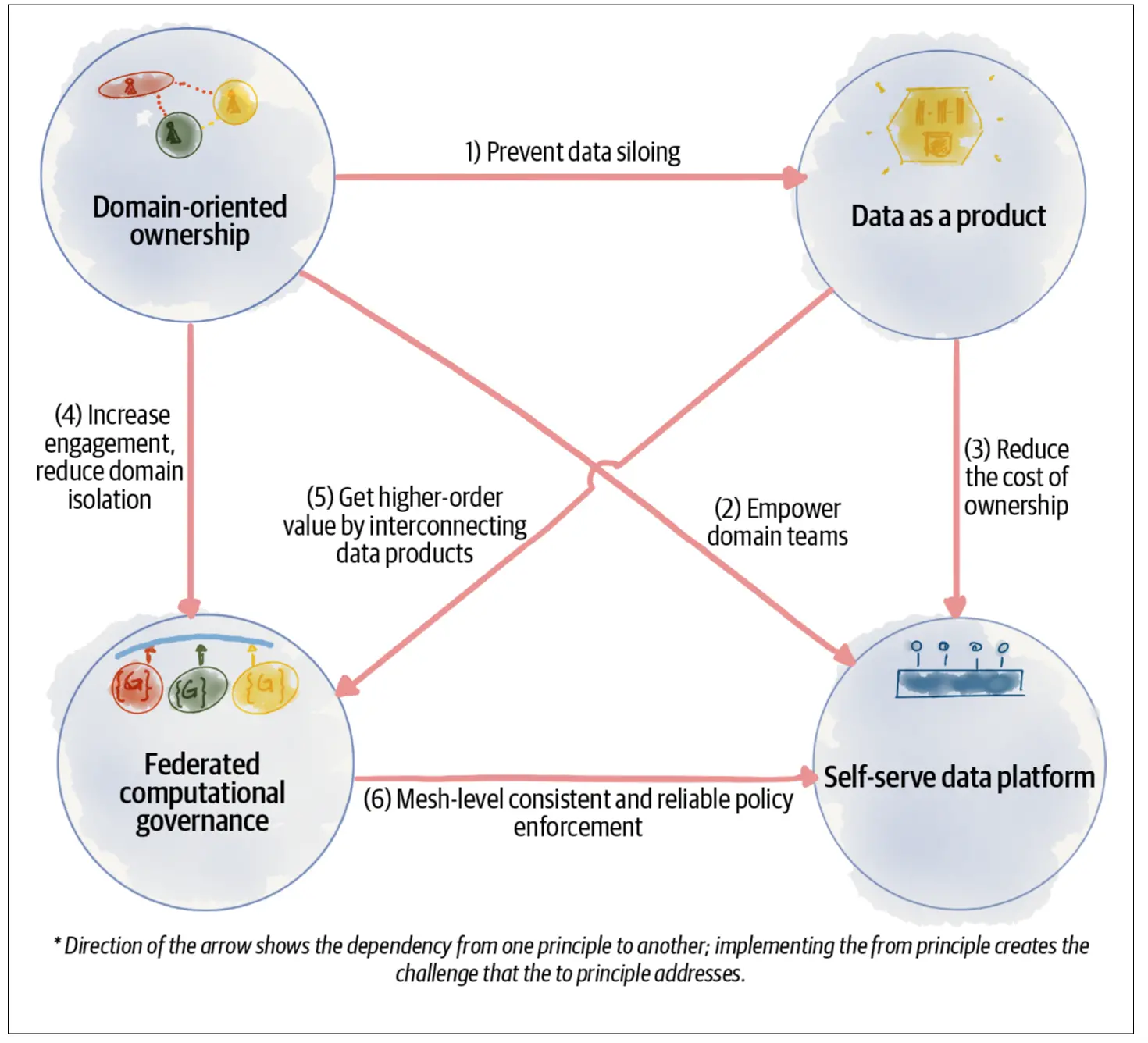
The DaaP principle within the data mesh highlights the necessity of a standardized process for making data available on a self-service basis. This helps reduce dependency on centralized data teams and allows for more effective data leveraging.
Treating data as a product also aligns with another data mesh principle: federated computational governance. This decentralized governance model enables scalability, as each domain independently manages its data products while adhering to overarching organizational standards.
To sum up, the principle of treating data as a product is integral to the success of a data mesh strategy. It fosters a decentralized, scalable, and user-centric data environment, enabling your employees across all levels to make well-informed decisions that lead to sustainable business growth.
How to Implement Data as a Product in Your Organization?
Here is a breakdown of the steps required to implement the data as a product concept in your organization:
Step 1: Shift in Mindset
Start by treating your data teams as customers and try to understand their needs and pain points. Shift your mindset from simply collecting data to prioritizing its usability for your teams and external partners. Apply product management thinking when building data as a product.
Step 2: Define Data Product Ownership and Teams
Establish dedicated teams within each business domain consisting of data engineers, analysts, and domain experts. These teams can help understand and verify their domain-specific requirements and use cases and will be accountable for their data.
Step 3: Set Clear Objectives and Metrics
Define objectives and business outcomes you want to achieve with data as a product. This could include improving decision-making, enhancing customer insights, or streamlining operations. Establish KPIs to measure impact and identify gaps for improvement.
Step 4: Develop a Robust Data Architecture
Design a flexible and scalable data architecture that supports the creation, management, and consumption of data as a product. This architecture should facilitate data integration, storage, retrieval, and interoperability across your organization.
Step 5: Implement Data Governance and Quality Standards
Implement data quality management processes, such as validation, cleansing, and auditing, to maintain high data standards. Establish comprehensive governance policies to protect your data against unauthorized access and breaches.
Step 6: Building & Delivering the Data Product
Ensure your data products are consistent, accurate, and up-to-date to provide relevant insights. Develop an intuitive interface like dashboards or APIs that require minimal technical expertise for data access and exploration.
Step 7: Create User-Centric Data Catalogs
Develop comprehensive data catalogs that provide detailed information about each of your data products, including descriptions, metadata, and usage guidelines. Ensure that data catalogs are user-friendly and searchable, enabling your teams to quickly find the datasets they need.
Step 8: Invest in Training & Change Management
Provide training and implement change management strategies to facilitate the transition toward utilizing data as a product and ensure organization-wide understanding and adoption of DaaP practices.
Streamlining Data Integration for DaaP
A critical aspect of DaaP is ensuring high-quality data flows are used for your applications and advanced analytics models. To achieve this, you need to integrate your organization’s data residing in multiple sources and create a unified view, which can be a complex and time-consuming process. Airbyte, a data integration and replication platform, can help you streamline the data movement between multiple sources and a destination.
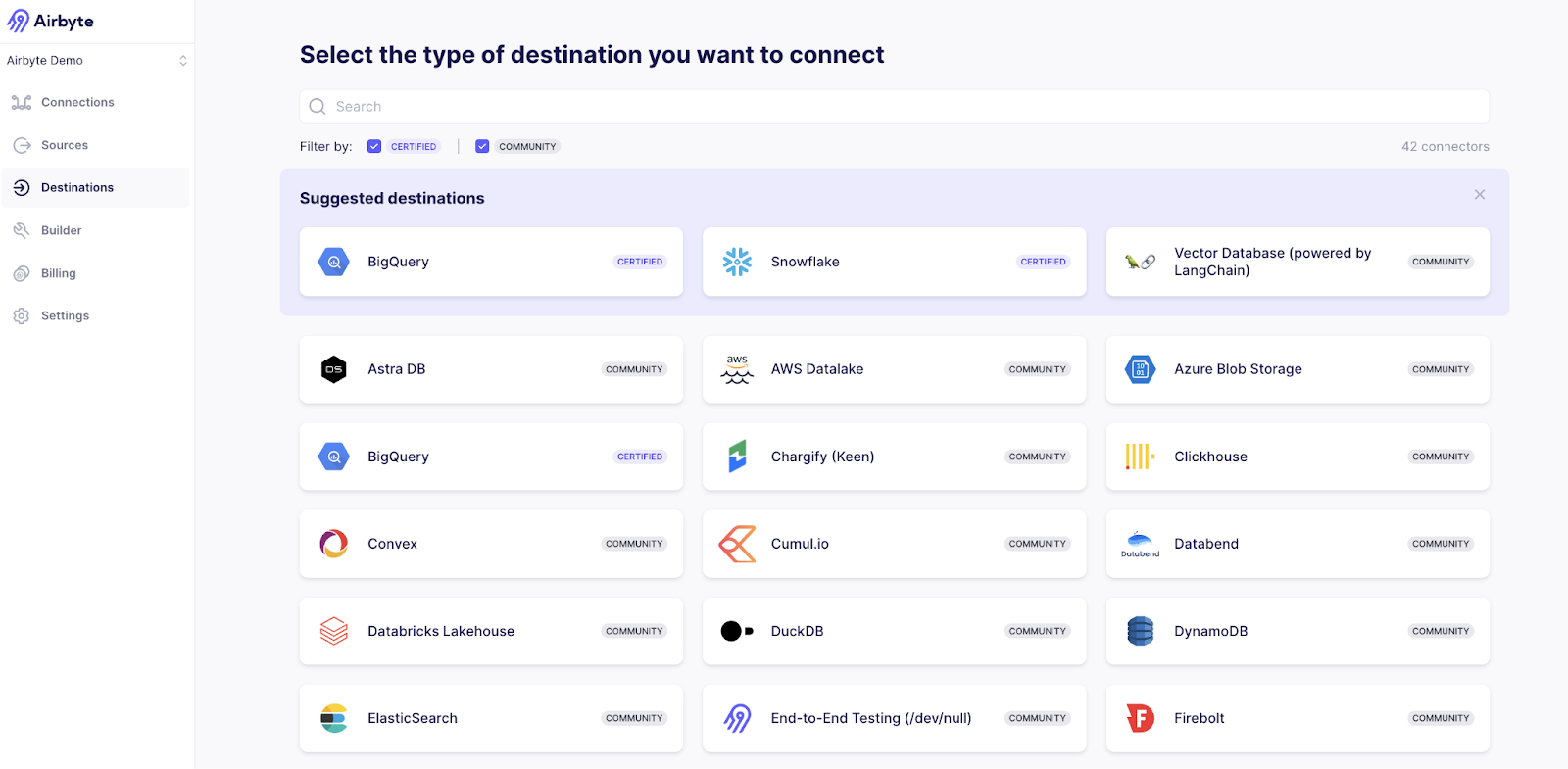
Here's how Airbyte empowers your data as a product approach:
- Simplifies Data Acquisition: Airbyte's extensive library of over 350 pre-built connectors allows you to extract data from a wide range of sources effortlessly. It also provides you the flexibility to build custom connectors using the no-code Connector Development Kit (CDK).
- GenAI Workflows: You can effortlessly ingest unstructured data into leading vector stores like Pinecone, Weaviate, or Milvus and simplify your AI workflows. LangChain's advanced capabilities for chunking and embedding, powered by OpenAI, Cohere, and other providers, can help you enhance your RAG transformations.
- Flexible Pipeline Management: Airbyte allows you to create and manage data pipelines easily using an intuitive interface, powerful APIs, Terraform Provider, or PyAirbyte.
- Supports Complex Data Transformations: With Airbyte, you can easily integrate with dbt (Data Build Tool) and transform your data into a suitable format based on your requirements.
- Change Data Capture and Incremental Sync: Airbyte’s Change Data Capture (CDC) feature helps you detect and capture the data changes made at the source. This ensures you have access to the latest data. Airbyte also supports full refreshes and incremental sync based on your needs.
- Schema Change Management: Based on your configured settings for detecting and propagating schema changes, Airbyte automatically reflects or ignores the changes in source data schemas. This ensures accurate and efficient data syncs with minimized errors.
- Scalability and Flexibility: Airbyte can scale up or down based on your organization’s data volume. Its cloud-based and self-managed service easily fits into your existing data infrastructure, which is crucial for handling changing workloads.
- Security and Governance: Your sensitive data remains protected with Airbyte’s security measures, such as data encryption, audit trails, monitoring, SSO, and RBAC. It ensures compliance by adhering to industry standards like ISO 27001, SOC 2, GDPR, and HIPAA.
Airbyte automates most of its processes, which helps ensure the accuracy, reliability, and high quality of your data pipelines. Its no-code, user-intuitive interface allows you to explore data independently with minimal technical expertise, aligning with the DaaP concept.
You can also connect with the expert community of over 2000 data engineers. They have built 7000+ custom connectors in minutes using the low-code/no-code or AI Connector Builder and would be happy to help with your Airbyte projects.
To learn more about Airbyte, you can refer to its official documentation.
Examples of Data as a Product
Let's take a look at some real-world data as a product examples to illustrate how this approach applies across industries:
- Mayo Clinic leverages data as a product to personalize medical care and improve diagnoses and treatment plans. This involves integrating and analyzing patient data from various sources, including genomics, medical history, and wearable devices.
- Netflix utilizes the DaaP concept to enhance user experience by analyzing data on watched content, ratings, and browsing behavior. This information flows into recommendation algorithms, increasing engagement and subscriber retention.
- JP Morgan Chase uses DaaP to prevent financial fraud by continuously monitoring real-time transactional data and identifying fraudulent activities. This helps safeguard customers and reduce the risk of financial losses.
- Siemens implements data as a product by collecting and analyzing sensor data from machines and production lines. This enables the company to perform predictive maintenance, prevent downtime, and optimize production processes.
Key Takeaways
The concept of data as a product empowers you to create high-quality, user-centric datasets tailored to meet the needs of your data teams, end-users, or partners. This not only enhances decision-making but also fosters a data-driven culture that brings new possibilities for growth.
Implementing DaaP requires a strategic shift. By following the steps outlined in this guide and investing in data architecture that supports DaaP, you can improve the operational efficiency of most of your data processes.
Continuously monitor your needs, refine your approach, and educate your employees accordingly. Adopting the DaaP approach can help you transform your existing data into a strategic asset that fuels innovation and drives results.
FAQs
Why treat data as a product?
Treating data as a product necessitates prioritizing the maintenance of high-quality, trustworthy data that drives valuable insights and improved decision-making.
What is the principle of data as a product?
The “data as a product” principle applies product management thinking to data. Data is seen as a valuable asset, prioritizing usability, clear ownership, and the needs of your data teams.
What is the difference between data as an asset and data as a product?
Data as an asset is valuable information, but it might be unprocessed, undocumented, or siloed. However, data as a product is a refined version of that asset. It is well-organized, documented, and designed to meet specific user needs.