AI and machine learning (ML) are revolutionizing data integration by offering scalable, intelligent solutions through artificial intelligence (AI) and machine learning (ML) technologies. These platforms use machine learning algorithms, natural language processing, and computer vision to optimize data processing, detect anomalies, recommend transformations, and train models effectively.
This guide presents ten leading data integration platforms that harness AI models and ML capabilities for enhanced performance. Whether handling batch ETL jobs or real-time cloud processing, these tools offer a competitive advantage, ensuring efficient data integration with minimal effort.
What Is AI/ML-Enabled Data Integration?
AI/ML-enabled data integration platforms use artificial intelligence and machine learning to manage data ingestion, transformation, monitoring, and governance efficiently. AI is designed to mimic human intelligence, distinguishing it from machine learning, which focuses on teaching machines to perform specific tasks by identifying patterns. These systems learn from training data, adapting to new sources and challenges without explicit programming.
Key features include:
- Anomaly detection using machine learning models
- Schema evolution tracking for system changes
- Predictive analytics for performance and resource management
- Generative AI for auto-generated SQL and transformation logic
- Self-healing workflows for autonomous error recovery
- Data pre-processing for preparing data for various tasks like classification and regression
Some platforms offer natural language interfaces, simplifying data flow creation for non-technical users while enabling data scientists to focus on complex tasks. AI-driven integration also covers image and video data, providing insights through computer vision and image processing technologies across various industries.
Benefits of AI/ML in Data Integration Tools
AI and machine learning artificial intelligence streamline data integration, offering smarter pipelines that reduce complexity. Advantages include:
- Automated Tasks: AI tools handle repetitive tasks like schema mapping, reducing the need for domain expertise.
- Improved Data Quality: ML models ensure data consistency and validation, enhancing analytics.
- Faster Development: Natural language interfaces and rapid prototyping speed up workflow creation.
- Resource Optimization: AI efficiently manages computational power, especially in cloud environments like Google Cloud.
- Resilience and Flexibility: AI models adapt pipelines to new data sources and formats.
- Advanced Monitoring: ML tools provide real-time tracking and model evaluation.
- Efficient handling of large datasets, ensuring scalability and performance.
These AI/ML solutions enhance technical performance and support strategic tasks like fraud detection. Whether you’re training models or deploying models, these tools unlock your data’s full potential, especially as you accumulate more data.
How to Choose the Right AI/ML Data Integration Tool
With a growing number of vendors claiming AI capabilities, it’s critical to look past the marketing and evaluate real-world impact. Consider whether the tool integrates expert systems for tailored AI solutions. Evaluate how the tool augments human intelligence by providing actionable insights from complex data. Use the following criteria to assess whether a tool fits your organization’s goals, team composition, and technical maturity.
1. AI/ML Features
Are you looking for ML tools that assist with development (e.g., SQL generation, data transformation) or platforms that fully automate model training, pipeline recovery, and anomaly resolution? Look for platforms that simplify the application of machine learning technology through user-friendly ml algorithms. Also consider support for custom models, reinforcement learning, or tools that integrate with open source software libraries like scikit-learn or TensorFlow. Consider tools that include AI systems capable of mimicking human intelligence for more accurate and responsive solutions.
2. Data Ecosystem Compatibility
Your chosen solution should integrate easily with your current data warehouses, data lakes, and orchestration tools. Look for platforms that support structured, unstructured, and visual data from a broader range of data sources, including APIs, IoT devices, and streaming platforms.
3. User Friendliness vs. Customizability
Some tools offer low-code interfaces ideal for analysts and operations teams. Others provide developer-first experiences with SDKs, CLI tools, and API extensibility. The best platforms balance user-friendly nature with advanced technology for control and customization for advanced users.
4. Scalability and Performance
Choose tools that scale with your data and offer AI/ML-powered optimization, such as predictive analytics, workload-aware resource allocation, and auto-scaling in cloud environments like Google Cloud Services. Choose tools that leverage numerical computation for advanced data analysis and algorithm development.
5. Monitoring, Observability, and Resilience
Look for platforms that include deep learning for anomaly detection, automated pipeline recovery, and real-time monitoring of model performance and pipeline health. Look for platforms that efficiently process data for real-time monitoring and anomaly detection.
6. Vendor Transparency and Roadmap
Check if the vendor offers clear documentation on AI applications, AI models, and future plans. Avoid platforms with unclear AI lifecycle processes. Check if the vendor is involved in ongoing research and development to stay ahead of technological advancements.
Define your needs to find AI solutions with the right mix of automation, flexibility, and performance. These tools enable rapid deployment, smarter data analysis, and scalable infrastructure, turning raw data into business insights efficiently.
Utilizing machine learning, artificial intelligence, deep learning, and ML tools, these platforms enhance data preprocessing, decision-making, and operational efficiency, ensuring high performance and adaptability.
1. Airbyte
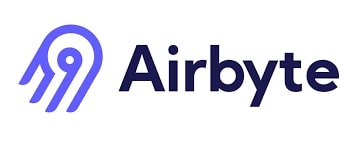
Overview: Airbyte is an open-source software library for data integration, offering flexibility, modular architecture, and a strong community. It supports over 550 sources and destinations, making it ideal for scalable data pipelines.
How it Uses AI/ML
Airbyte leverages AI tools and machine learning (ML) models to enhance pipeline resilience, data quality, and performance optimization. Its features include anomaly detection, schema drift handling, and automated sync monitoring, reducing operational overhead.
Key Features:
- 550+ connectors with open-source extensibility
- AI-driven connector generation and dev assistance
- Modular design for custom workflows
- Native support for change data capture (CDC) and orchestration tools
- Cloud-managed and self-hosted options
Best for: Teams seeking a flexible, AI-aware integration platform with strong community support, combining custom engineering with intelligent automation.
2. StreamSets

Overview: StreamSets is a data integration platform designed for smart data pipelines across hybrid and multi-cloud environments, supporting both batch and real-time processing. It is known for robust data observability and DevOps-friendly features.
How it Uses AI/ML
StreamSets uses machine learning for anomaly detection, pipeline drift monitoring, and dataflow diagnostics. Its ML models enable machines to analyze historical pipeline performance and metadata to identify abnormal patterns, schema changes, and potential data integrity issues before they cause failures. This enhances pipeline resilience and efficiency, making it ideal for fraud detection in financial services. By incorporating generative AI, StreamSets can automatically generate transformation logic, allowing it to perform tasks with greater efficiency and minimal human intervention.
Key Features:
- ML-based anomaly detection for intelligent monitoring
- Schema evolution tracking with drift alerts
- Hybrid support for cloud, on-prem, and containerized environments
- Built-in versioning and lineage tracking
- Visual low-code pipeline interface with code extensibility
Best for: Enterprise teams managing complex, high-stakes data flows needing strong observability and predictive resilience powered by machine learning and generative AI to perform tasks efficiently.
3. AWS Glue

Overview: AWS Glue is a serverless data integration service within the AWS ecosystem, designed for scalable data preparation, transformation, and loading. It integrates with services like S3, Redshift, Athena, and SageMaker.
How it Uses AI/ML
AWS Glue leverages machine learning for its Data Catalog, automatically classifying datasets, inferring schemas, and tracking data lineage. It offers ML Transforms for deduplication and entity matching, streamlining the task of data structure management. Additionally, it supports deep learning tasks for complex models. These features enhance data quality and streamline data structure management.
Key Features:
- ML-driven data classification and schema inference
- Built-in transforms for deduplication
- Scalable ETL engine with Apache Spark
- Seamless AWS integration
- Visual and code-first pipeline interfaces
Best for: Organizations within the AWS ecosystem seeking serverless scalability and ML-powered automation for data management.
4. Nexla
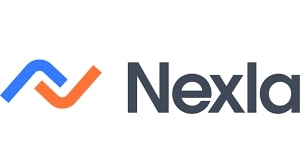
Overview: Nexla is a data operations platform that enables teams to integrate, prepare, and deliver data across systems with minimal engineering effort. It supports both real-time and batch pipelines, with a strong emphasis on usability across technical and non-technical users.
How it Uses AI/ML
Nexla integrates artificial intelligence and machine learning to create Nexsets, intelligent data objects that manage schema recognition and metadata inference. This enables automatic detection of data changes and mapping suggestions, minimizing manual effort. AI-driven dimensionality reduction techniques further simplify data analysis, allowing pipelines to adapt seamlessly to structural or scale changes while helping teams stay informed with minimal human intervention.
Key Features:
- ML-generated Nexsets for adaptive schema management
- Automated data mapping, enrichment, and validation
- Unified interface for engineers, analysts, and business users
- Supports both real-time streaming and batch ingestion
- Built-in monitoring and role-based access controls
Best for: Organizations that need to operationalize data across many teams—without requiring everyone to write code—and want a platform that can intelligently manage change under the hood.
5. IBM DataStage

Overview: IBM DataStage is a robust ETL platform for scalable data integration across on-prem and cloud environments, part of IBM’s broader data and AI ecosystem.
How it Uses AI/ML
IBM DataStage utilizes Watson AI and machine learning models for predictive workload optimization, resource tuning, and error resolution. Neural networks enhance its capabilities by automating decisions on job scheduling and execution, improving efficiency and minimizing failures.
Key Features:
- AI-assisted workload balancing and failure prediction
- Integration with IBM Cloud Pak for Data
- Graphical and code-based pipeline authoring
- Metadata-driven governance and lineage tracking
- Designed for complex, high-scale enterprise environments
Best for: Large enterprises needing a mature ETL engine with AI-driven performance optimization and strong governance standards.
6. Gathr

Overview: Gathr is a unified platform for data engineering, streaming analytics, and ML ops. It brings low-code data pipeline development to technical and non-technical teams alike, while embedding ML to streamline backend processing.
How it Uses AI/ML
Gathr uses machine learning for auto-scaling, job tuning, and pipeline health diagnostics. Its platform analyzes usage patterns, resource consumption, and performance metrics to dynamically allocate compute and recommend improvements—helping teams avoid overprovisioning and reduce costs.
Key Features:
- ML-powered workload prediction and resource optimization
- Unified support for streaming, batch, and event-based data
- Drag-and-drop interface with code extensibility
- Built-in connectors to cloud warehouses and ML platforms
- Integrated monitoring and performance insights
Best for: Teams that want a hybrid low-code experience with strong back-end optimization—and need to manage diverse data workloads without micromanaging infrastructure.
7. Zuar
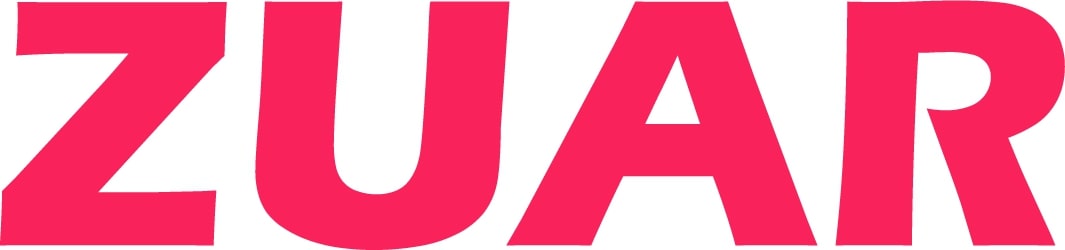
Overview: Zuar offers a lightweight but powerful data integration and automation platform tailored to analytics workflows. It simplifies data ingestion, transformation, and delivery into BI tools and dashboards, leveraging generative AI for enhanced data processing.
How it Uses AI/ML
Zuar incorporates AI-assisted data prep to help users clean, transform, and structure data faster. It also includes smart alerting and monitoring features that use historical usage and performance data to surface anomalies and potential pipeline issues before they break reports. These AI solutions, powered by generative AI, ensure data integrity and operational efficiency.
Key Features:
- Lightweight deployment and quick setup for SMBs and mid-market
- ML-based monitoring for data freshness and sync anomalies
- Prebuilt integrations with BI tools like Tableau and Power BI
- Pipeline templates and reusable transformation blocks
- White-labeling options for embedded analytics delivery
Best for: Teams focused on analytics enablement who want a lightweight, ML-enhanced integration layer that connects cleanly to downstream visualization tools, with the added benefits of generative AI and robust AI solutions.
8. Coalesce.io
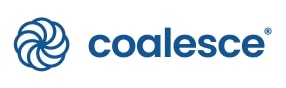
Overview: Coalesce is a column-aware transformation platform purpose-built for data teams working inside cloud data warehouses like Snowflake. It combines a structured UI with full SQL flexibility and metadata-driven modeling, utilizing artificial intelligence and natural language processing to streamline complex data tasks.
How it Uses AI/ML
Coalesce is integrating ML tools and generative AI to auto-generate transformation logic, suggest model structures, and accelerate column-level lineage creation. These AI solutions, enhanced by artificial intelligence and natural language processing, reduce time spent on repetitive modeling work and improve confidence in downstream reporting accuracy.
Key Features:
- AI-assisted modeling and transformation templates
- Visual interface paired with editable SQL
- Column-level metadata and lineage tracking
- Versioning, git integration, and reusable components
- Optimized for Snowflake and scalable data warehousing
Best for: Analytics and data engineering teams building complex warehouse models who want the efficiency of AI-driven modeling with the control of SQL and metadata-first design, supported by artificial intelligence and natural language processing.
9. Hevo Data

Overview: Hevo is a no-code data pipeline platform focused on reliability and ease of use. It helps teams ingest and sync data from SaaS apps and databases to cloud warehouses in real time.
How it Uses AI/ML
Hevo uses machine learning to power its auto-recovery features, intelligent alerting, and pipeline monitoring. ML models help detect sync anomalies, diagnose root causes, and suggest corrective actions, all aimed at improving pipeline reliability without manual babysitting.
Key Features:
- ML-powered error detection and auto-resolution
- Prebuilt integrations with 150+ sources
- Real-time syncs and low-latency pipelines
- Visual interface for transformation and routing
- Built-in observability and alerting system
Best for: Data teams at startups and mid-market companies looking for a plug-and-play integration platform with built-in reliability features and ML-powered support.
10. Etleap

Overview: Etleap is a managed ETL platform that combines the flexibility of custom pipelines with the simplicity of a fully managed service. It’s built for teams who want powerful transformation workflows without the overhead of maintaining infrastructure.
How it Uses AI/ML
Etleap uses machine learning to detect and adapt to schema changes, optimize transformation execution, and provide automated pipeline health checks. Its AI-enhanced features are designed to prevent common integration failures and minimize downtime, especially in fast-changing data environments.
Key Features:
- Schema evolution detection with automated pipeline updates
- ML-based health monitoring and alerting
- Full support for Redshift, Snowflake, and other warehouse targets
- Managed infrastructure with detailed observability
- Strong emphasis on data accuracy and uptime
Best for: Teams that want a managed ETL solution with just enough AI to reduce risk and improve day-to-day pipeline performance—without needing to manage the backend.
Final Thoughts: Smarter Integration Starts Here
AI and machine learning, including generative AI and AI applications, are transforming the way modern teams think about data integration—from building pipelines to keeping them healthy over time. These technologies are applied in real-world scenarios, including smart devices like Google Nest, which utilize machine learning to perform tasks such as providing estimated commute times, thereby improving user interaction through technology. Whether you’re working with streaming data, evolving schemas, or just looking to eliminate manual grunt work, the tools in this list are paving the way for a smarter, more adaptive future.
AI tools and ML tools are essential for modern data integration, providing the intelligence needed to build and maintain efficient, scalable pipelines. AI is designed to mimic human intelligence, distinguishing it from ML, which focuses on teaching machines to perform specific tasks by identifying patterns.
If you’re looking for a platform that balances developer control, extensibility, and AI-powered automation, Airbyte is a strong place to start. With 550+ connectors, a growing suite of AI and ML features, and the flexibility of open-source or managed deployment, Airbyte helps you build resilient, scalable pipelines—without the overhead.
Ready to see what intelligent integration looks like? Start building with Airbyte today.